Deep-learning radiomics based on ultrasound can objectively evaluate thyroid nodules and assist in improving the diagnostic level of ultrasound physicians
Introduction
Thyroid nodules present a growing concern in the medical community. Due to advancements in medical technology, the detection rate of thyroid nodules has increased significantly over the past 30 years (1). Ultrasound (US) is the recommended initial imaging modality for assessing palpable thyroid nodules (1). The detection rate of thyroid nodules in the general population can reach up to 65% through US examination, and approximately 90% of thyroid nodules are benign and 95% are asymptomatic (2). The determination of the nature of thyroid nodules has become an important clinical issue after thyroid screening. Both puncture and surgical pathology are invasive methods. Therefore, the safe and non-invasive identification of benign and malignant thyroid nodules is essential to achieve reasonable management and prevent excessive interventions and treatment (3,4).
The Thyroid Imaging, Reporting, and Data System (TI-RADS) is a commonly used method for evaluating thyroid nodules based on the US semantic features of conventional US images. This method evaluates thyroid nodules based on the composition, echogenicity, shape, margin, and echogenic foci. However, this evaluation method is largely influenced by the subjective judgment of physicians. Artificial intelligence (AI) can improve diagnostic performance and interobserver agreement in thyroid cancer diagnosis, especially in less-experienced physicians (5). Compared with the subjective qualitative reasoning of imaging physicians, AI can extract a large number of features from traditional imaging that cannot be observed by the naked eye for the objective quantitative evaluation of clinical tasks, increasing the accuracy and repeatability of diagnosis (6). Thus, the in-depth analysis of US images using radiomics technology could greatly facilitate the diagnosis and evaluation of thyroid nodules. The potential of radiomics to improve the ability of physicians with different levels of experience to diagnose thyroid malignant nodules is crucial and also needs to be explored (7).
Radiomics analysis, both handcrafted and through deep-learning (DL), has received significant attention in recent years due to advancements in image information mining technology (8). This approach uses a vast array of features to develop predictive models for diagnosis, prognosis, and treatment planning. Radiomics analysis has shown good potential in distinguishing between benign and malignant thyroid nodules, and the area under the receiver operating characteristic curve of this method has been reported to reach 0.97 (9). However, some of the limitations of previous studies include small sample sizes (10), the absence of multicenter evidence (11), and the failure to include readily accessible basic clinical data (e.g., age and sex) and US semantic features as evaluation factors (12). In addition, few studies have compared the ability of physicians across all levels of experience and DL to differentiate between benign and malignant thyroid nodules.
A nomogram is a visual model that establishes scoring criteria based on the regression coefficients of all predictive indicators, assigning a score to each value level of each predictive indicator to optimize the accuracy and intuitiveness of predictions (13).
This study sought to develop a nomogram based on multicenter handcrafted radiomics combined with DL to study the potential of traditional US to distinguish between benign and malignant thyroid nodules. It also sought to examine the ability of DL radiomics to improve the diagnostic ability of physicians with different levels of experience, and to confirm the reliability and applicability of this technology as a practical tool for thyroid nodule diagnosis and treatment planning. We present this article in accordance with the TRIPOD reporting checklist (available at https://qims.amegroups.com/article/view/10.21037/qims-23-1597/rc).
Methods
The study was conducted in accordance with the Declaration of Helsinki (as revised in 2013). The study was approved by the Ethics Committee of Ordos Central Hospital (No. 2024-100). The Ethics Committee waived the requirement for individual consent for this retrospective analysis.
Patients
This study retrospectively collected the data of 1,645 thyroid nodules from 1,031 patients diagnosed and treated between January 2016 and January 2022. The nodule data were collected from three institutions (institution 1, Ordos Central Hospital; institution 2, North China University of Science and Technology Affiliated Hospital; institution 3, The Affiliated Taizhou People’s Hospital of Nanjing Medical University). The development set comprised data from institution 1, while the external validation sets 1 and 2 comprised data from institutions 2 and 3, respectively (Figure 1).
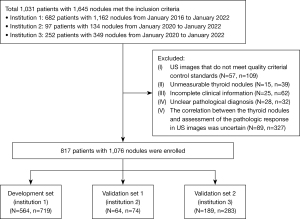
To be eligible for inclusion in this study, the patients had to meet the following inclusion criteria: (I) be aged over 18 years; (II) have nodules with pathological results obtained by fine-needle aspiration or surgical excision; and (III) have undergone a conventional US examination within one month before the pathological examination. Patients were excluded from the study if they met any of the following exclusion criteria: (I) had US images that did not meet image quality control standards; (II) had unmeasurable thyroid nodules; (III) had incomplete clinical information; (IV) had an unclear pathological diagnosis (according to the Bethesda System for Reporting Thyroid Cytopathology, under which the nodules were classified as Bethesda I, III, or IV, but there were no final pathological results); and/or (V) the correlation between the thyroid nodules and assessment of the pathologic response in the US images was uncertain. Table 1 illustrates the process of the study population enrollment.
Table 1
Variables | Development set (n=719) | Validation set 1 (n=74) | Validation set 2 (n=283) | ||||||||
---|---|---|---|---|---|---|---|---|---|---|---|
Benign nodule (n=411) | Malignant nodule (n=308) | P value | Benign nodule (n=8) | Malignant nodule (n=66) | P value | Benign nodule (n=175) | Malignant nodule (n=108) | P value | |||
Age, years | 55.06±10.55 | 46.08±10.75 | <0.001 | 53.50±12.25 | 53.09±12.09 | 0.928 | 57.41±9.90 | 51.20±9.86 | <0.001 | ||
Gender | 0.089 | 0.807 | 0.393 | ||||||||
Female | 315 (76.64) | 253 (82.14) | 6 (75.00) | 42 (63.64) | 120 (68.57) | 80 (74.07) | |||||
Male | 96 (23.36) | 55 (17.86) | 2 (25.00) | 24 (36.36) | 55 (31.43) | 28 (25.93) | |||||
Transverse dimension (mm) | 27.90±17.54 | 10.64±7.47 | <0.001 | 24.34±9.74 | 9.74±9.72 | <0.001 | 21.56±14.91 | 9.93±6.06 | <0.001 | ||
Longitudinal dimension (mm) | 21.84±14.42 | 9.39±5.52 | <0.001 | 14.35±6.17 | 8.31±6.11 | <0.001 | 12.55±8.68 | 7.62±3.97 | <0.001 | ||
Shape | <0.001 | <0.001 | <0.001 | ||||||||
Width greater than height | 392 (95.38) | 195 (63.31) | 8 (100.00) | 36 (54.55) | 172 (98.29) | 89 (82.41) | |||||
Height greater than width | 19 (4.62) | 113 (36.69) | 0 | 30 (45.45) | 3 (1.71) | 19 (17.59) | |||||
Location | 0.202 | 0.383 | 0.581 | ||||||||
Right lobe | 213 (51.82) | 165 (53.57) | 2 (25.00) | 33 (50.00) | 88 (50.29) | 48 (44.44) | |||||
Left lobe | 186 (45.26) | 127 (41.23) | 5 (62.50) | 29 (43.94) | 80 (45.71) | 54 (50.00) | |||||
Isthmus | 10 (2.43) | 16 (5.19) | 1 (12.50) | 4 (6.06) | 7 (4.00) | 6 (5.56) | |||||
Left lobe and isthmus | 2 (0.49) | 0 | 0 | 0 | 0 | 0 | |||||
Composition | <0.001 | 0.034 | <0.001 | ||||||||
Mixed cystic and solid | 199 (48.42) | 15 (4.87) | 3 (37.50) | 2 (3.03) | 46 (26.29) | 0 | |||||
Cystic or almost completed cystic | 36 (8.76) | 1 (0.32) | 0 | 0 | 23 (13.14) | 0 | |||||
Solid or almost completed solid | 176 (42.82) | 292 (94.81) | 5 (62.50) | 64 (96.97) | 106 (60.57) | 108 (100) | |||||
Echogenicity | <0.001 | <0.001 | <0.001 | ||||||||
Hypoechoic | 81 (19.71) | 256 (83.12) | 3 (37.50) | 58 (87.88) | 77 (44.00) | 104 (96.30) | |||||
Isoechoic | 36 (8.76) | 1 (0.32) | 0 | 0 | 25 (14.29) | 0 | |||||
Anechoic | 2 (0.49) | 11 (3.57) | 0 | 0 | 0 | 1 (0.93) | |||||
Very hypoechoic | 286 (69.59) | 36 (11.69) | 5 (62.50) | 6 (9.09) | 65 (37.14) | 2 (1.85) | |||||
Hyperechoic | 6 (1.46) | 4 (1.30) | 0 | 2 (3.03) | 8 (4.57) | 1 (0.93) | |||||
Echogenic foci | <0.001 | 0.312 | <0.001 | ||||||||
None | 2 (0.49) | 0 | 0 | 0 | 1 (0.57) | 1 (0.93) | |||||
Punctate echogenic foci | 357 (86.86) | 160 (51.95) | 6 (75.00) | 33 (50.00) | 150 (85.71) | 49 (45.37) | |||||
Macrocalcifications | 24 (5.84) | 117 (37.99) | 2 (25.00) | 22 (33.33) | 12 (6.86) | 43 (39.81) | |||||
Peripheral calcifications | 28 (6.81) | 31 (10.06) | 0 | 11 (16.67) | 12 (6.86) | 15 (13.89) | |||||
Margin | <0.001 | 1 | <0.001 | ||||||||
Smooth | 388 (94.40) | 180 (58.44) | 0 | 0 | 159 (90.86) | 40 (37.04) | |||||
Lobulated or irregular | 1 (0.24) | 1 (0.32) | 0 | 0 | 0 | 1 (0.93) | |||||
Indistinct border | 22 (5.35) | 127 (41.23) | 8 (100.00) | 66 (100.00) | 16 (9.14) | 67 (62.04) |
Data are presented as N (%) or mean ± standard deviation. n indicates the number of nodules.
Ultimately, 1,076 nodules from 817 patients were included in the study, of which 594 were benign and 482 were malignant. The development set comprised the data of 564 patients with 719 nodules, while validation set 1 comprised the data of 64 patients with 74 thyroid nodules, and validation set 2 comprised the data of 189 patients with 283 thyroid nodules. The pathological results for all the nodules were obtained by puncture or surgery. In the development set, there were 308 malignant nodules and 411 benign nodules. In validation set 1, there were 66 malignant nodules and 8 benign nodules. In validation set 2, there were 108 malignant nodules and 175 benign nodules. The pathological classification of the thyroid nodules is shown in Table S1.
US examination
The parameters and inspection methods of the US instrument are shown in Appendix 1. The clinical information of the patients, including their gender and age, was obtained from the Hospital Information System.
Extraction and selection of clinical US semantic signature
For the development of the clinical US semantic signature, the transverse dimension, longitudinal dimension, orientation, composition, echogenicity, echogenic foci, and margin of the nodules were evaluated independently by two experts, A and B, with 11 and 14 years of experience in diagnosing thyroid nodules using US, respectively. The two experts evaluated the thyroid nodules without knowledge of the pathological results, and any disputes over the results were resolved by negotiation. The features with significant differences between the groups were identified and used as the predictive variables. A univariate logistic regression analysis was used to select the risk factors with a P value <0.05. The selected factors were imported into the multivariate regression analysis, and the clinical US semantic signature (C_US_sig) was constructed by the retaining features with a P value <0.05.
Segmentation images
The regions of interest (ROIs) were manually segmented using ITK-SNAP software (version 3.8.0, http://www.itksnap.org). This process resulted in a manually segmented ROI for each nodule. Two US physicians (C and D) randomly selected 30 cases for blind tumor segmentation, and used the intraclass correlation coefficient (ICC) to evaluate the consistency of the extracted radiomics features. An ICC >0.75 indicated that the image features had good repeatability (14). Physician C segmented the remaining 1,046 images. It is important to note that this study did not require exclusion of necrotic or cystic areas within the ROIs.
Radiomics signature (Rad_sig)
For the radiomics features, the PyRadiomics package in the Python platform was used to extract features from the ROI. The radiomics features were categorized into three groups according to their geometry, intensity, and texture. The geometry features describe the shape properties of the ROI, while the intensity features describe the statistical distribution of the voxel intensities in the ROI, and the texture features describe the patterns and spatial distributions of the intensities using various methods, including the gray-level co-occurrence matrix (GLCM), gray-level run-length matrix (GLRLM), gray-level size-zone matrix (GLSZM), and neighborhood gray-tone difference matrix (NGTDM). In total, 1,561 features were calculated for each ROI, using these different categories and methods.
Regarding the radiomics features, the data were first normalized, after which the redundant features were reduced using the t-test and a Pearson correlation analysis (15). If a strong correlation with a Spearman correlation coefficient >0.85 was detected between two features, the feature with the higher absolute value was selected for further analysis. Subsequently, least absolute shrinkage and selection operator (LASSO) regression with 10-fold cross-validation was employed to select features with non-zero coefficients (16). Finally, the Rad_sig was created by linearly combining these selected features based on the LASSO regression coefficients.
DL features
We used six commonly used DL models (densenet201, densenet121, resnet50, resnet101, inception_v3, and vgg 19), and the model with the best area under the curve (AUC) performance in the validation sets was selected for the DL feature construction. The segmented US images were preprocessed by normalizing the gray values to the range (–1, 1) using minimum-maximum transformation. The normalized images were then resized to 224×224 pixels with the nearest interpolation. The processed images served as the input for the optimal model, which was then fine-tuned using the development set. Finally, the predicted probability of the optimal DL model was taken as the signature (DL_sig). Guided gradient-weighted class activation mapping was employed to visualize the output of the last convolutional layer in the convolutional neural networks (CNNs), highlighting the specific subregions that played a crucial role in generating the DL features (17).
Nomogram construction and effectiveness evaluation
The C-US_sig, Rad_sig, and DL_sig were combined to construct a comprehensive nomogram for distinguishing between benign and malignant thyroid nodules. Figure 2 shows a workflow diagram that depicts the development and validation of the signatures and nomogram in this study. Calibration curves were used to evaluate the consistency between the estimated probability and actual probability. A decision curve analysis (DCA) was used to assess the clinical usefulness of the nomogram by estimating the net benefit in the threshold probabilities (18).
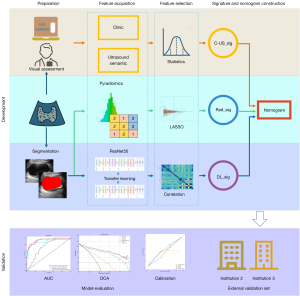
Evaluation of thyroid nodules by physicians with different levels of experience
In validation set 2, three junior physicians, three senior physicians, and three experts each differentiated the benign and malignant thyroid nodules based on Chinese TI-RADS (C-TI-RADS) (19) with solid composition, microcalcifications, markedly hypoechoic, ill-defined or irregular margins, and vertical orientation as the malignant US features. Each malignant feature was scored 1 point, and a score of ≥4 points indicated malignant nodules. The obtained results were compared with the puncture or postoperative pathological results. Next, these nine physicians re-interpreted the results based on the Rad_sig and DL_sig to obtain the AUCs, and the diagnostic efficacy of the physicians was compared before and after DL radiomics assistance using the Delong test. Calibration curves were used to evaluate the consistency between the estimated probability and actual probability. A DCA was used to assess the clinical usefulness by estimating the net benefit in the threshold probabilities.
Statistical analysis
The categorical variables were compared using the Chi-square test or Fisher test, while the quantitative variables were compared using the t-test or Mann-Whitney U-test to assess the differences among the groups. All the data analyses were performed using Python (version 3.7.12; https://pyradiomics.readthedocs.io/) on the OnekeyAI platform (version 3.1.8). For the statistical analysis, we used version 0.13.2 of the statistical model. We used the radiomics package (version 3.0.1) to extract the radiomics features. Machine-learning algorithms, such as support vector machines, were implemented using scikit-learn (version1.0.2). DL models were developed based on the torch 1.11.0 version, including cuda 11.3.1 and cudnn8.2.1.
Results
Clinical characteristics
Table 1 sets out the clinical characteristics of the entire dataset. Significant distinctions were observed between the benign and malignant nodules in terms of age, transverse dimension, longitudinal dimension, orientation, echogenic foci, margin, echogenicity, and composition (all P<0.001). The Pearson correlation coefficients of the features were calculated, and the results showed a strong correlation between the transverse dimension and longitudinal dimension, while the correlations between other features were not significant (Figure S1).
Signature and nomogram construction
Based on the univariate and multivariate analyses, age, transverse dimension, orientation, echogenic foci, margin, echogenicity, and composition were identified as independent risk factors for predicting malignant thyroid nodules (P<0.05). These factors constituted the C_US_sig (Table S2).
In total, 1,561 features were initially analyzed using the t-test and Spearman correlation analysis, resulting in the retention of 371 features. Subsequently, LASSO regression was applied, which identified 45 features with non-zero coefficients. These 45 features were then linearly combined based on their respective coefficients to form the Rad_sig. These 45 features comprised one original image feature, one logarithm filter feature, 24 wavelet transform filter features, seven local binary pattern features, four exponential filter features, two gradient filter features, three square root filter features, and three-square filter features (Table S3).
Among the six DL models, resnet50 was chosen as the pre-trained CNN model. Feature heatmaps of two patient examples generated from the ResNet50 were visualized in Figure 3. All the models were pre-trained on the ILSVRC-2012 dataset. The specific parameters for DL fine tuning are provided in Appendix 2. The predicted probability of the DL model was directly used as the DL_sig. Additionally, C_US_sig, Rad_sig, and DL_sig were integrated in a comprehensive nomogram (Figure 4).
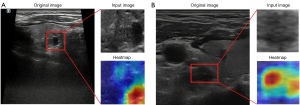
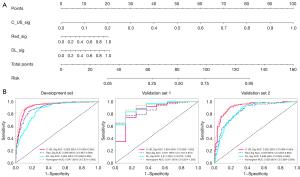
Model performance and evaluation
The AUCs of the C_US_sig, Rad_sig, DL_sig, and comprehensive nomogram models in the development set were 0.926 [95% confidence interval (CI): 0.906–0.946], 0.908 (95% CI: 0.887–0.929), 0.864 (95% CI: 0.838–0.890), and 0.947 (95% CI: 0.931–0.963), respectively, with corresponding accuracy values of 0.879, 0.840, 0.791, and 0.890. In validation set 1, the AUCs were 0.854 (95% CI: 0.695–1.000), 0.871 (95% CI: 0.770–0.973), 0.911 (95% CI: 0.813–1.000), and 0.917 (95% CI: 0.824–1.000), respectively, with respective accuracy values of 0.865, 0.689, 0.838, and 0.865. In validation set 2, the AUCs were 0.922 (95% CI: 0.889–0.954), 0.849 (95% CI: 0.803–0.894), 0.848 (95% CI: 0.804–0.893), and 0.929 (95% CI: 0.899–0.959), respectively, (Figure 4), with corresponding accuracy values 0.851, 0.745, 0.755, and 0.858. The specific performance results for each model is presented in Table 2. The comprehensive nomogram had the highest AUC and accuracy among all the datasets. Based on the DCA results, the nomogram had the best clinical net benefit. The calibration curves indicated good calibration for all models (Figure 5).
Table 2
Data set | Model | AUC (95% CI) | Accuracy | Sensitivity | Specificity |
---|---|---|---|---|---|
Development set | C-US_sig | 0.926 (0.906–0.946) | 0.879 | 0.905 | 0.859 |
Rad_sig | 0.908 (0.887–0.929) | 0.840 | 0.84 | 0.839 | |
DL_sig | 0.864 (0.838–0.890) | 0.791 | 0.886 | 0.720 | |
Nomogram | 0.947 (0.931–0.963) | 0.890 | 0.895 | 0.886 | |
Validation set 1 | C-US_sig | 0.854 (0.695–1.000) | 0.865 | 0.879 | 0.750 |
Rad_sig | 0.871 (0.770–0.973) | 0.689 | 0.652 | 1.000 | |
DL_sig | 0.911 (0.813–1.000) | 0.838 | 0.833 | 0.875 | |
Nomogram | 0.917 (0.824–1.000) | 0.865 | 0.864 | 0.875 | |
Validation set 2 | C-US_sig | 0.922 (0.889–0.954) | 0.851 | 0.907 | 0.817 |
Rad_sig | 0.849 (0.803–0.894) | 0.745 | 0.925 | 0.634 | |
DL_sig | 0.848 (0.804–0.893) | 0.755 | 0.916 | 0.657 | |
Nomogram | 0.929 (0.899–0.959) | 0.858 | 0.935 | 0.811 |
The nomogram integrates the C-US_sig, Rad_sig, and DL_sig. AUC, area under the curve; CI, confidence interval; C_US_sig, clinical ultrasound semantic signature; Rad_sig, radiomics signature; DL_sig, deep-learning signature.
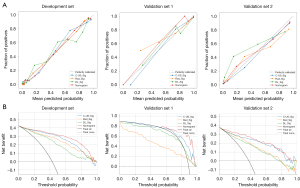
Evaluation of thyroid nodules by physicians with different levels of experience
In validation set 2, the AUCs of the C_US_sig models for three junior physicians were as follows: 0.752 (95% CI: 0.700–0.804), 0.735 (95% CI: 0.682–0.788), and 0.714 (95% CI: 0.659–0.769). In contrast, the AUCs of the three senior physicians were higher, at 0.824 (95% CI: 0.779–0.869), 0.802 (95% CI: 0.754–0.849), and 0.740 (95% CI: 0.687–0.793). Among all groups, the three experts performed the best, with AUCs of 0.908 (95% CI: 0.874–0.942), 0.891 (95% CI: 0.855–0.927), and 0.901 (95% CI: 0.865–0.936), demonstrating their outstanding ability in evaluating the model. After reinterpreting using DL radiomics, the AUCs of the three models constructed by junior physicians in validation set 2 significantly increased, reaching 0.858 (95% CI: 0.817–0.899), 0.875 (95% CI: 0.834–0.915), and 0.923 (95% CI: 0.890–0.955), respectively. Similarly, the AUCs of the model established by senior physicians have also increased, reaching 0.888 (95% CI: 0.850–0.925), 0.937 (95% CI: 0.907–0.967), and 0.944 (95% CI: 0.916–0.973), respectively. Among experts, their three models demonstrated excellent performance, with AUCs of 0.919 (95% CI: 0.88–0.951), 0.912 (95% CI: 0.879–0.945), and 0.917 (95% CI: 0.85–0.948), indicating that DL radiomics has a significant impact on improving model accuracy (Figure 6). The Delong test showed a statistically significant difference in the diagnostic efficacy of the junior and senior physicians in diagnosing thyroid nodules before and after DL radiomics assistance (P<0.05). However, no statistically significant difference was observed in the diagnostic efficacy of the experts in diagnosing thyroid nodules (Figure S2). Simultaneously, the calibration curve and DCA showed that the models with DL radiomics assistance had good performance in terms of the calibration and clinical net benefits (Figure S3).
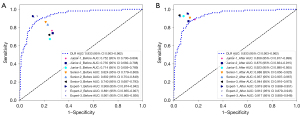
Discussion
This multicenter study combined clinical semantic features, Rad_sig, and DL features to develop and validate a combined nomogram for distinguishing between benign and malignant thyroid nodules. The results showed that the nomogram developed in this study outperformed the standalone use of either the clinical US semantic model or the Rad_sigs in terms of the AUC and accuracy. This nomogram had the highest AUC and accuracy across all the datasets, as well as the highest clinical utility as revealed by the DCA results. The discriminative performance of our nomogram was superior or at least comparable to that of previous models (5-10). Meanwhile, DL radiomics assistance improved the ability of junior and senior physicians to differentiate between benign and malignant thyroid nodules.
US is the preferred imaging method for screening thyroid nodules. Semantic features are the most commonly used method for evaluating benign and malignant thyroid nodules; composition, echogenicity, shape, margin, and echogenic foci, play a crucial role in the diagnosis of malignant thyroid nodules (20,21). Our research suggests that a younger age, smaller transition dimension, vertical orientation, predominately solid composition, hypoechogenicity, microcalculations, and an ill-defined margin are independent predictors of malignant thyroid nodules. The C_US_sig had good diagnostic performance in the two validation sets with AUCs of 0.854 and 0.922, respectively. These results strongly align with the diagnostic value observed in the study conducted by Chen et al. (22) in which age and US signs (such as margin, shape, echogenic foci, and echogenicity) were used to accurately identify malignant thyroid nodules. Consequently, our findings reinforce the importance of conventional US features in the identification of both benign and malignant thyroid nodules.
TI-RADS provides an effective evaluation method by optimizing the points assigned to each US semantic feature to improve system performance, preventing unnecessary needle biopsies and surgeries (23). Wildman-Tobriner et al. (24) concluded that applying machine learning to TI-RADS may optimize system performance. Radiomics analysis is a quantitative and objective computer-based image analysis technique that provides additional information and thus facilitates the diagnosis of thyroid nodules, and is widely used in the diagnosis, grading, staging, and prognosis prediction of organ diseases such as thyroid, breast, chest and lungs, liver, kidney, and gynecology (25).
We constructed radiomics models using different machine-learning methods to predict benign and malignant thyroid nodules, and demonstrated good performance using the two validation sets (AUCs: 0.871 and 0.849). Several single-center studies have shown that incorporating radiomics modality can further improve the basic diagnostic performance of models when combined with clinical and US information (26-28). The strengths of our research lie in the use of multicenter data with a relatively large sample size. These characteristics enhanced the stability and generalizability of our model. However, traditional radiomics features are pre-set and selected based on the professional knowledge of US physicians, and are limited by the development of medical imaging technology and the different levels of experience of doctors (29).
DL is an evolution from artificial neural networks. It uses a hierarchical structure to separate and automatically learn features from shallow to deep data layers to build models. Its feature selection and learning are spontaneous and do not require manual intervention, which significantly reduces the subjective impact of evaluators. Due to its strong learning ability, there are already many diagnostic models based on DL networks in the field of medical imaging (30).
Currently, DL is widely used in the feature extraction stage of radiomics. We performed a radiomics analysis in our cohort using both DL and handcrafted methods. Notably, the diagnostic performance of the DL features was not superior to that of the radiomics features, which is not consist with previous research findings (31,32). Several factors might have contributed to the suboptimal performance of the DL features. First, the margin between the benign thyroidal tissue and malignant tissue might have appeared unclear or blurry on the US imaging, making it challenging for the CNN model to accurately extract textural features, thereby affecting the model’s performance (33). Second, the selection of only the two-dimensional ROI slice of the nodule for the DL network analysis might have resulted in the insufficient extraction of nodule information, subsequently affecting the CNN model’s recognition ability. Third, handcrafted features primarily capture image texture, voxel intensity, and shape characteristics, while CNN-based DL features employ hierarchical neural networks to extract multi-level features from images (34). Fourth, handcrafted features are more dependent on the doctor’s subjectivity, which is inconsistent with DL algorithms. Thus, the inconsistency between the physical meanings of handcrafted and DL features could be one of the most significant reasons for the unsatisfactory diagnostic performance of the DL features.
In addition, we used DL radiomics to assist junior physicians, senior physicians, and experts to distinguish between benign and malignant thyroid nodules. We found that DL radiomics effectively improved the diagnostic level of the primary and intermediate physicians (P<0.05), and enhanced the practical work of thyroid nodule diagnosis and risk stratification. These advantages were particularly beneficial for newly diagnosed thyroid nodule patients and those undergoing monitoring. For experts, the effectiveness of DL radiomics improvement was not very significant, indicating that our model requires further optimization and performance improvement.
This study had several limitations. First, our research was only based on the differentiation of benign and malignant thyroid nodules, and refined risk stratification based on the pathological types of malignant thyroid nodules is an important direction for future research. Second, not enough was done to reduce the noise and improve the image resolution of the US images, and further research is needed under the guidance of relevant research and methods in the future (35,36). Third, the selection of imaging biomarkers based on radiomics and DL models lack biological validation, which limits their widespread clinical application.
Conclusions
Our comprehensive nomogram combining US semantic features, radiomics features, and DL features has high predictive power for benign and malignant thyroid nodules. DL radiomics can assist junior and senior physicians to improve their ability to distinguish between thyroid nodules. It is expected to have auxiliary value for clinical practice in practical work.
Acknowledgments
We would like to express our thanks to the OnekeyAI platform and its developers, as well as all of the individuals who participated in this study and each of the researchers and technicians who made this work possible.
Funding: This study was supported by
Footnote
Reporting Checklist: The authors have completed the TRIPOD reporting checklist. Available at https://qims.amegroups.com/article/view/10.21037/qims-23-1597/rc
Conflicts of Interest: All authors have completed the ICMJE uniform disclosure form (available at https://qims.amegroups.com/article/view/10.21037/qims-23-1597/coif). The authors have no conflicts of interest to declare.
Ethical Statement: The authors are accountable for all aspects of the work in ensuring that questions related to the accuracy or integrity of any part of the work are appropriately investigated and resolved. The study was conducted in accordance with the Declaration of Helsinki (as revised in 2013). The study was approved by the Ethics Committee of Ordos Central Hospital (No. 2024-100). The Ethics Committee waived the requirement for individual consent for this retrospective analysis.
Open Access Statement: This is an Open Access article distributed in accordance with the Creative Commons Attribution-NonCommercial-NoDerivs 4.0 International License (CC BY-NC-ND 4.0), which permits the non-commercial replication and distribution of the article with the strict proviso that no changes or edits are made and the original work is properly cited (including links to both the formal publication through the relevant DOI and the license). See: https://creativecommons.org/licenses/by-nc-nd/4.0/.
References
- Wang B, Wan Z, Zhang M, Gong F, Zhang L, Luo Y, Yao J, Li C, Tian W. Diagnostic value of a dynamic artificial intelligence ultrasonic intelligent auxiliary diagnosis system for benign and malignant thyroid nodules in patients with Hashimoto thyroiditis. Quant Imaging Med Surg 2023;13:3618-29. [Crossref] [PubMed]
- Durante C, Grani G, Lamartina L, Filetti S, Mandel SJ, Cooper DS. The Diagnosis and Management of Thyroid Nodules: A Review. JAMA 2018;319:914-24. [Crossref] [PubMed]
- Grani G, Sponziello M, Pecce V, Ramundo V, Durante C. Contemporary Thyroid Nodule Evaluation and Management. J Clin Endocrinol Metab 2020;105:2869-83. [Crossref] [PubMed]
- Gharib H, Papini E, Garber JR, Duick DS, Harrell RM, Hegedüs L, Paschke R, Valcavi R, Vitti P. AACE/ACE/AME Task Force on Thyroid Nodules. American Association of Clinical Endocrinologists, American College of Endocrinology, and Associazione Medici Endocrinologi Medical Guidelines for Clinical Practice for the Diagnosis and Management of Thyroid Nodules--2016 Update. Endocr Pract 2016;22:622-39. [Crossref] [PubMed]
- Ha EJ, Lee JH, Lee DH, Moon J, Lee H, Kim YN, Kim M, Na DG, Kim JH. Artificial Intelligence Model Assisting Thyroid Nodule Diagnosis and Management: A Multicenter Diagnostic Study. J Clin Endocrinol Metab 2024;109:527-35. [Crossref] [PubMed]
- Summers RM. Artificial Intelligence of COVID-19 Imaging: A Hammer in Search of a Nail. Radiology 2021;298:E162-4. [Crossref] [PubMed]
- Cleere EF, Davey MG, O'Neill S, Corbett M, O'Donnell JP, Hacking S, Keogh IJ, Lowery AJ, Kerin MJ. Radiomic Detection of Malignancy within Thyroid Nodules Using Ultrasonography-A Systematic Review and Meta-Analysis. Diagnostics (Basel) 2022;12:794. [Crossref] [PubMed]
- Yang WT, Ma BY, Chen Y. A narrative review of deep learning in thyroid imaging: current progress and future prospects. Quant Imaging Med Surg 2024;14:2069-88. [Crossref] [PubMed]
- Zhou H, Jin Y, Dai L, Zhang M, Qiu Y, Wang K, Tian J, Zheng J. Differential Diagnosis of Benign and Malignant Thyroid Nodules Using Deep Learning Radiomics of Thyroid Ultrasound Images. Eur J Radiol 2020;127:108992. [Crossref] [PubMed]
- Prochazka A, Gulati S, Holinka S, Smutek D. Classification of Thyroid Nodules in Ultrasound Images Using Direction-Independent Features Extracted by Two-Threshold Binary Decomposition. Technol Cancer Res Treat 2019;18:1533033819830748. [Crossref] [PubMed]
- Colakoglu B, Alis D, Yergin M. Diagnostic Value of Machine Learning-Based Quantitative Texture Analysis in Differentiating Benign and Malignant Thyroid Nodules. J Oncol 2019;2019:6328329. [Crossref] [PubMed]
- Angell TE, Maurer R, Wang Z, Kim MI, Alexander CA, Barletta JA, Benson CB, Cibas ES, Cho NL, Doherty GM, Doubilet PM, Frates MC, Gawande AA, Krane JF, Marqusee E, Moore FD, Nehs MA, Larsen PR, Alexander EK. A Cohort Analysis of Clinical and Ultrasound Variables Predicting Cancer Risk in 20,001 Consecutive Thyroid Nodules. J Clin Endocrinol Metab 2019;104:5665-72. [Crossref] [PubMed]
- Balachandran VP, Gonen M, Smith JJ, DeMatteo RP. Nomograms in oncology: more than meets the eye. Lancet Oncol 2015;16:e173-80. [Crossref] [PubMed]
- Huang Y, Zhu T, Zhang X, Li W, Zheng X, Cheng M, Ji F, Zhang L, Yang C, Wu Z, Ye G, Lin Y, Wang K. Longitudinal MRI-based fusion novel model predicts pathological complete response in breast cancer treated with neoadjuvant chemotherapy: a multicenter, retrospective study. EClinicalMedicine 2023;58:101899. [Crossref] [PubMed]
- Wang T, She Y, Yang Y, Liu X, Chen S, Zhong Y, Deng J, Zhao M, Sun X, Xie D, Chen C. Radiomics for Survival Risk Stratification of Clinical and Pathologic Stage IA Pure-Solid Non-Small Cell Lung Cancer. Radiology 2022;302:425-34. [Crossref] [PubMed]
- Wang JC, Fu R, Tao XW, Mao YF, Wang F, Zhang ZC, Yu WW, Chen J, He J, Sun BC. A radiomics-based model on non-contrast CT for predicting cirrhosis: make the most of image data. Biomark Res 2020;8:47. [Crossref] [PubMed]
- Selvaraju RR, Cogswell M, Das A, Vedantam R, Parikh D, Batra D. Grad-CAM: Visual Explanations from Deep Networks via Gradient-Based Localization. 2017 IEEE International Conference on Computer Vision (ICCV), Venice, Italy, 2017:618-26.
- Fitzgerald M, Saville BR, Lewis RJ. Decision curve analysis. JAMA 2015;313:409-10. [Crossref] [PubMed]
- Zhou J, Yin L, Wei X, Zhang S, Song Y, Luo B, et al. 2020 Chinese guidelines for ultrasound malignancy risk stratification of thyroid nodules: the C-TIRADS. Endocrine 2020;70:256-79. [Crossref] [PubMed]
- Anil G, Hegde A, Chong FH. Thyroid nodules: risk stratification for malignancy with ultrasound and guided biopsy. Cancer Imaging 2011;11:209-23. [Crossref] [PubMed]
- Pang T, Huang L, Deng Y, Wang T, Chen S, Gong X, Liu W. Logistic regression analysis of conventional ultrasonography, strain elastosonography, and contrast-enhanced ultrasound characteristics for the differentiation of benign and malignant thyroid nodules. PLoS One 2017;12:e0188987. [Crossref] [PubMed]
- Chen L, Zhang J, Meng L, Lai Y, Huang W. A new ultrasound nomogram for differentiating benign and malignant thyroid nodules. Clin Endocrinol (Oxf) 2019;90:351-9. [Crossref] [PubMed]
- Tessler FN, Middleton WD, Grant EG, Hoang JK, Berland LL, Teefey SA, Cronan JJ, Beland MD, Desser TS, Frates MC, Hammers LW, Hamper UM, Langer JE, Reading CC, Scoutt LM, Stavros AT. ACR Thyroid Imaging, Reporting and Data System (TI-RADS): White Paper of the ACR TI-RADS Committee. J Am Coll Radiol 2017;14:587-95. [Crossref] [PubMed]
- Wildman-Tobriner B, Buda M, Hoang JK, Middleton WD, Thayer D, Short RG, Tessler FN, Mazurowski MA. Using Artificial Intelligence to Revise ACR TI-RADS Risk Stratification of Thyroid Nodules: Diagnostic Accuracy and Utility. Radiology 2019;292:112-9. [Crossref] [PubMed]
- Guiot J, Vaidyanathan A, Deprez L, Zerka F, Danthine D, Frix AN, Lambin P, Bottari F, Tsoutzidis N, Miraglio B, Walsh S, Vos W, Hustinx R, Ferreira M, Lovinfosse P, Leijenaar RTH. A review in radiomics: Making personalized medicine a reality via routine imaging. Med Res Rev 2022;42:426-40. [Crossref] [PubMed]
- Tong Y, Li J, Huang Y, Zhou J, Liu T, Guo Y, Yu J, Zhou S, Wang Y, Chang C. Ultrasound-Based Radiomic Nomogram for Predicting Lateral Cervical Lymph Node Metastasis in Papillary Thyroid Carcinoma. Acad Radiol 2021;28:1675-84. [Crossref] [PubMed]
- Hu HT, Wang Z, Huang XW, Chen SL, Zheng X, Ruan SM, Xie XY, Lu MD, Yu J, Tian J, Liang P, Wang W, Kuang M. Ultrasound-based radiomics score: a potential biomarker for the prediction of microvascular invasion in hepatocellular carcinoma. Eur Radiol 2019;29:2890-901. [Crossref] [PubMed]
- Wang X, Agyekum EA, Ren Y, Zhang J, Zhang Q, Sun H, Zhang G, Xu F, Bo X, Lv W, Hu S, Qian X. A Radiomic Nomogram for the Ultrasound-Based Evaluation of Extrathyroidal Extension in Papillary Thyroid Carcinoma. Front Oncol 2021;11:625646. [Crossref] [PubMed]
- Fu J, Singhrao K, Zhong X, Gao Y, Qi SX, Yang Y, Ruan D, Lewis JH. An Automatic Deep Learning-Based Workflow for Glioblastoma Survival Prediction Using Preoperative Multimodal MR Images: A Feasibility Study. Adv Radiat Oncol 2021;6:100746. [Crossref] [PubMed]
- Shafiee MJ, Chung AG, Khalvati F, Haider MA, Wong A. Discovery radiomics via evolutionary deep radiomic sequencer discovery for pathologically proven lung cancer detection. J Med Imaging (Bellingham) 2017;4:041305. [Crossref] [PubMed]
- Refaee T, Salahuddin Z, Frix AN, Yan C, Wu G, Woodruff HC, Gietema H, Meunier P, Louis R, Guiot J, Lambin P. Diagnosis of Idiopathic Pulmonary Fibrosis in High-Resolution Computed Tomography Scans Using a Combination of Handcrafted Radiomics and Deep Learning. Front Med (Lausanne) 2022;9:915243. [Crossref] [PubMed]
- Yang X, Wu L, Zhao K, Ye W, Liu W, Wang Y, Li J, Li H, Huang X, Zhang W, Huang Y, Chen X, Yao S, Liu Z, Liang C. Evaluation of human epidermal growth factor receptor 2 status of breast cancer using preoperative multidetector computed tomography with deep learning and handcrafted radiomics features. Chin J Cancer Res 2020;32:175-85. [Crossref] [PubMed]
- Li H, Weng J, Shi Y, Gu W, Mao Y, Wang Y, Liu W, Zhang J. An improved deep learning approach for detection of thyroid papillary cancer in ultrasound images. Sci Rep 2018;8:6600. [Crossref] [PubMed]
- Yamashita R, Nishio M, Do RKG, Togashi K. Convolutional neural networks: an overview and application in radiology. Insights Imaging 2018;9:611-29. [Crossref] [PubMed]
- Cammarasana S, Nicolardi P, Patanè G. Real-time denoising of ultrasound images based on deep learning. Med Biol Eng Comput 2022;60:2229-44. [Crossref] [PubMed]
- Kumar V, Webb J, Gregory A, Meixner DD, Knudsen JM, Callstrom M, Fatemi M, Alizad A. Automated Segmentation of Thyroid Nodule, Gland, and Cystic Components From Ultrasound Images Using Deep Learning. IEEE Access 2020;8:63482-96.