Assessment of lumbar paraspinal muscle morphology using mDixon Quant magnetic resonance imaging (MRI): a cross-sectional study in healthy subjects
Introduction
Lumbar paraspinal muscles (LPM) are an important part of the lumbar extensor muscles and the deep spinal (core) stabilisation system. They are composed of two main groups: the transversospinalis muscle group, including the multifidus muscle (MF); and more laterally, the erector spinae muscle (ES), which consists of the longissimus and iliocostalis muscles. The lumbar extensor muscular system plays an important role in stabilising the lumbar spine and trunk. Inadequate function of the lumbar extensor muscles and poor coordination of the core muscles are thought to be important aetiological factors in low back pain (1-3). Several neuromuscular diseases are also characterised by dysfunction of the trunk muscles including the LPM, for example motor neuron diseases and axial myopathies (4,5). In terms of macroscopical morphological assessment, two signs of muscle damage are usually described: a decrease in muscle size [expressed either as a cross-sectional area (CSA) or total muscle volume (TMV)] and an increase in the amount of fatty deposits [expressed as a fat fraction (FF)] (6-8). Another biomarker, functional muscle volume (FMV), is easily derived from two biomarkers described above (TMV and FF) and is a preferred parameter over TMV (7).
The morphology of LPM may be influenced by several physiological variables, mostly by age, sex, and anthropometric parameters (9-12), but also by the level of physical activity of the individual and by present comorbidities, such as diabetes mellitus. Moreover, previous studies have shown that individual parameters of paraspinal muscles differ in individual levels of the lumbar spine (9,11-15). Further, the degree of intramuscular fat infiltration seems to differ depending on the particular muscle. However, there is not yet a unified view of which paraspinal muscle has greater fat infiltration (9,13,16).
Morphological studies of paraspinal muscles vary widely in the literature, presumably due to different imaging modalities (9-13,17-19). Prior studies have predominantly used T1- or T2-weighted sequences for semi-quantitative assessments of FF (19-22). Recent research has demonstrated the usefulness of the Dixon technique for the quantitative assessment of muscle fat (9,11), with particularly accurate quantitative measurements achieved using the mDixon Quant magnetic resonance imaging (MRI) or similar sequences (12,18,23,24).
The aims of this study were: (I) to develop a methodology for LPM assessment using advanced MRI methods, including a manual segmentation process; (II) to confirm the reliability of the measurements; (III) to evaluate the values of LPM morphological parameters [FF and muscle volume (MV)] in a healthy population; (IV) to study the influence of physiological factors (age, sex, anthropometric parameters, physical activity) on muscle morphology; (V) to build equations to predict LPM morphological parameters in a healthy population. We present this article in accordance with the STROBE reporting checklist (available at https://qims.amegroups.com/article/view/10.21037/qims-23-1796/rc).
Methods
This was a prospective cross-sectional observational comparative single-centre study. The Ethics Committee of the University Hospital Brno approved the study protocol (No. 05-090621/EK), and written informed consent was obtained from all participants. The study was conducted in accordance with the Declaration of Helsinki (as revised in 2013).
Participants
Personal recruitment by experienced staff was used to gather volunteers from the Caucasian population of the South Moravian region in south-eastern Czech Republic. The recruitment was carried out from April 2021 to March 2023. The guidelines included an established quota of subjects, intended to obtain a proportional representation of four age categories (18–29, 30–39, 40–49, 50–70 years) and both sexes. Figure 1 summarises the subject recruitment.
Exclusion criteria were: age <18 years, acute low back pain, chronic low back pain (of duration over 12 weeks) in the medical history, presence of lumbosacral radicular pain in the medical history with residual signs of nerve root dysfunction (sensory impairment and/or absent or diminished reflexes and/or weakness) in clinical neurological examination and manual muscle testing of the lower extremities, previous surgery of the lumbar spine, vertebral fracture, spine infection or tumour, scoliosis, degenerative changes in the lumbar spine (the presence of lumbar spinal stenosis (Schizas grading scale above A4) and lumbar disc herniation), presence of myopathy, comorbid conditions affecting the overall mobility (e.g., post-stroke paresis, heart failure leading to limited mobility), confirmed pregnancy, general MRI contraindications, and the presence of metal material in the lumbar spine, even MRI-compatible metal, as artefacts can influence measurement accuracy.
Medical history and neurological clinical evaluation
Each subject underwent a detailed neurological clinical evaluation, including medical history, performed by an experienced neurologist (BA 28 working years, PK 6 working years) to confirm suitability for the study. Before the MRI assessment, each participant filled in a two-part questionnaire covering basic demographic and anthropometric data (age, sex, weight, and height) and the short form of the International Physical Activity Questionnaire (IPAQ) to quantify the level of physical activity. Based on the IPAQ, a total physical activity MET-minutes a week was calculated for each participant (MET is metabolic equivalent) (25).
MRI of the lumbar spine and LPM
The Philips Ingenia MRI system, with a 3T magnetic field and anterior and posterior receiving coils, was used for the morphological evaluation. The examination included standard MRI sequences for lumbar spine assessment (turbo spin echo T2, T1, and STIR in the sagittal plane and T2 in the axial and coronal planes). Furthermore, we utilised a 6-point Dixon gradient echo sequence with multi-fat-peak compensation (seven) as well as eddy current correction (labelled mDixon Quant by the Philips company) for creating water, fat, in-phase, out-phase, and FF images (23). The mDixon Quant sequence was acquired in the axial plane for the bilateral assessment of parameters of the LPM (MF and ES) and psoas muscles (PS) (as a control muscle that is located in the lumbar region but is not a paraspinal muscle). Parameters of MRI sequences are shown in Table 1. The minimal sequence coverage was from intervertebral disc Th12/L1 to L5/S1. The whole duration of the MRI examination was approximately 30 minutes.
Table 1
Parameter | Sag T2 | Sag STIR | Sag T1 | Cor T2 | Ax T2 | Ax Quant |
---|---|---|---|---|---|---|
FOV (mm) | 260×260 | 260×260 | 260×260 | 260×260 | 180×180 | 380×380 |
#Slices | 22 | 16 | 22 | 25 | 9/disc | 47 |
Slice thick. (mm) | 3 | 4 | 3 | 3 | 3 | 5 |
Acq. Vox. size (mm × mm) | 0.8×0.85 | 0.9×1 | 0.7×1 | 0.7×1 | 0.6×0.75 | 1.5×1.5 |
Rec. Vox. size (mm × mm) | 0.32×0.32 | 0.45×0.45 | 0.35×0.35 | 0.32×0.32 | 0.41×0.41 | 1.19×1.19 |
TR (ms) | 3,900 | 2,500 | 650 | 4,700 | 4,000 | 14 |
TE (ms) | 90 | 70 | 9 | 90 | 100 | 1.32 |
ETL/#Echoes | 23/– | 19/– | 3/– | 23/– | 24/– | –/6 |
TI/delta TE (ms) | – | 210/– | – | – | – | –/1.1 |
NSA | 1 | 1 | 1 | 1 | 2 | 2 |
SENSE | 1.5 | 1.7 | 1.5 | 1.8 | 2 | 2 |
Acq. time (m:s) | 2:38 | 3:10 | 2:39 | 2:07 | 6:26 | 4:48 |
MRI, magnetic resonance imaging; Sag, sagittal; STIR, short tau inversion recovery; Cor, coronal; Ax, axial; Ax Quant, mDIXON quant sequence; FOV, field of view; #Slices, number of slices; thick., thickness; Acq. Vox. size, acquisition voxel size; Rec. Vox. size, reconstruction voxel size; TR, repetition time; TE, time to echo; ETL, echo train length; #Echoes, number of echoes; TI, inversion time; NSA, number of signal averages; SENSE, sensitivity encoding parallel technique; Acq. time, acquisition time.
All MRI images were assessed by an experienced blinded radiologist (RG 7 working years, MS 4 working years, MK 21 working years) to exclude any pathology within the study exclusion criteria.
Segmentation of selected muscles
Image analysis of selected muscles (MF, ES, and PS bilaterally) was performed manually on all slices without any interpolation methods using the software application ITK-SNAP (26). The segmentation methodology and the defining of the regions of interest (ROIs) were based on recommendations by Crawford et al. (MF and ES) and on the description of healthy PS by Weinreb (27,28).
All three muscles (MF, ES, and PS) were segmented primarily in the water image; the other images (fat, in-phase, and out-phase) were used for detailed correction of the segmentation masks at all slices between the first axial MRI image above the superior endplate of L1 to the second axial MRI image below the inferior endplate of L5 (Figure 2).
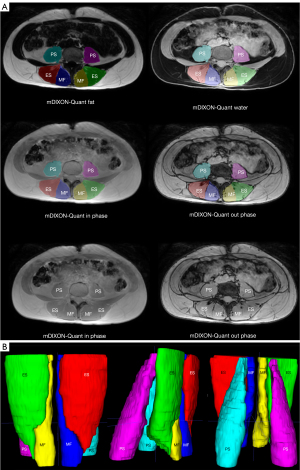
The definition of ROI from MRI for individual muscles is described below. The medial boundary of the MF extends from the spinal process to the lamina, with the anterior border defined by the mamillary process and the zygapophyseal joint. The lateral border is the fascial line between the MF and ES, and the posterior border follows the MF’s epimysium, distinguishable from nearby thoracolumbar fascia and subcutaneous adipose tissue (27).
For the ES, its medial boundary is formed by the fascial line between the MF and ES muscle, adjacent to the mamillary process and zygapophyseal joint. The anterior border extends along the lateral transverse process and adjacent muscles (quadratus lumborum muscle). The lateral and posterior borders follow the fascia surrounding the muscles, distinct from the thoracolumbar fascia and subcutaneous adipose tissue (27).
The segmentation of the PS utilised the well-visible fascia of the muscle as its outer border, starting from its origin and continuing to the L5/S1 vertebral level. The iliac muscle was not included in the segmentation; it was not part of the defined ROI (28).
Manual segmentation was allocated between three trained raters [a radiologist (rater M.S.) and two neurologists (raters P.K. and V.K.) with spine disease experience] blinded to the participants’ demographic, anthropometric, and clinical data. Segmentation masks of individual muscles were used to obtain FF, TMV, and FMV. Reconstruction algorithms used by mDixon Quant can produce voxels with negative FF or FF above 100%. In these cases, the value of voxels below 0 were set as 0 and the value of voxels over 100% were set as 100%. The final FF was calculated as the average of FF in all muscle voxels bilaterally, expressed as a percentage. TMV for a given muscle was determined for each individual by summing TMV values obtained for the right and left muscles. To estimate the FMV, we adopted the approach of Carlier et al. (7) and it was calculated as follows (Eq. [1]):
Data analysis
For the statistical analysis, we used IBM SPSS Statistics 28.0, Statistica 12 (StatSoft) and R (V4.1.1) software. The descriptive statistical analysis respected the type of data and the distribution of the values. Statistical significance in all statistical tests was set at two-sided P<0.05.
Prior to this study, the power analysis was conducted for estimating the minimum number of patients with chronic low back pain needed to detect differences in parameters of the LPM compared to healthy controls, which resulted in a requirement of 47 patients in each group. As for the healthy controls, we were aware of the need for a larger sample size, especially for matching purposes, and for reliable description of values covering the full range of healthy subjects. Therefore, we opted for a sample of 90 healthy controls (45 females, 45 males of all ages), which allowed us to adequately describe the variability of parameters in healthy subjects and was consistent with our capabilities.
The reliabilities of the segmentation masks and the FF and TMV of muscles (ES, MF, PS) were evaluated. To assess intra-rater reliability, a subsample of five randomly selected MRIs was re-segmented (always under a different name/ID to increase blindness) and evaluated by all three raters (M.S., P.K., V.K.), a total of three times, always three weeks apart. To assess inter-rater reliability, a subsample of 15 randomly selected volunteers was independently segmented and assessed by all three data-blinded raters. Reliability was assessed separately for the right and left muscles.
Inter- and intra-rater reliability was assessed using Dice coefficients for mask agreement, Hausdorff distance (HD), and average HD for error deviation calculated using the EvaluateSegmentation Tool (29). The Kruskal-Wallis test was used to detect any significant variation in the reliability of FF and volume measurements among raters, muscles, or right-left asymmetry. To assess measurement agreement, Pearson correlation coefficients (r, ∥r∥<0.3, weak; 0.3≤∥r∥<0.6, moderate; 0.6≤∥r∥<0.9, strong; ∥r∥≥0.9, perfect) and intraclass correlation coefficients (ICCs, ICC <0.5, poor; 0.5≤ ICC <0.75, moderate; 0.75≤ ICC <0.9, good; ICC ≥0.9, excellent) were calculated (30,31), as were absolute (Eq. [2]) and relative (Eq. [3]) differences of FF and TMV between raters.
Where X is FF or TMV and index 1 or 2 means two different measurements (inter or intra).
The statistical significance of differences in FF and MV among individual muscles were analysed with the Kruskal-Wallis rank sum test with the Wilcoxon post hoc test.
To assess the effect of the demographic and anthropometric factors and physical activity on FF, TMV, and FMV, multivariable linear regression models were built. Prior to the regression analysis, the normality of the variables’ distribution was tested utilising Shapiro-Wilk test and visually assessed by plotting histograms. Variables with non-normal distributions, i.e., FF, TMV, FMV, and MET-min/week, were log-transformed to approximate normality. Firstly, we built univariable linear regression models with FF, TMV, and FMV separately for each muscle as dependent variables, and age, height, weight, BMI, sex, and MET-min/week (log scaled) separately as explanatory variables. To assess the importance of a given factor on the dependent variables, R-squared (a percentage of model variability explained by the factor), was calculated together with the statistical significance of the factor/covariate. Multivariable linear regression models were built based on the significance of individual explanatory parameters in the univariable models. Graphs were generated for each muscle showing the predicted FF, TMV, and FMV with 95% confidence intervals and their dependence on the statistically significant factors from the multivariable linear regression models. If any of the factors were not found to be significant for a given morphological parameter, the predicted values for one representative factor/covariate value were displayed in the graph. That is, if sex was not significant, the predicted values of the morphological parameter were shown for males; if BMI was not significant, BMI 25 kg/m2 was used; and if age was not significant, age 40 years was chosen. Finally, based on multivariable linear regression models, equations were built to calculate the predicted FF in each muscle and their prediction interval bounds for an individual with particular age, sex, and BMI, alongside the predicted TMV and FMV in each muscle with prediction interval bounds for an individual with particular age, weight and sex.
Results
Basic characteristics of participants
Ninety-one healthy volunteers underwent clinical examination and MRI of the lumbar spine and LPM (Figure 1). One volunteer was excluded due to the finding of an asymptomatic spinal intramedullary ependymal cyst at the level of the L1 vertebral body on MRI. A total of 90 subjects were included in the analysis (45 men, 45 women). Each of the four age categories included 10 to 12 subjects of both sexes. The essential characteristics of these subjects, including age, sex, height, weight, BMI, and level of physical activity, are comprehensively outlined in Table 2.
Table 2
Variable | Sex | Total | 18–29 years | 30–39 years | 40–49 years | 50–70 years |
---|---|---|---|---|---|---|
Sex (N) | Male | 45 | 11 | 12 | 11 | 11 |
Female | 45 | 12 | 12 | 11 | 10 | |
Age (years) | Male | 38.0 (30.0, 48.0) | 27.0 (24.0, 28.0) | 32.0 (31.0, 37.0) | 43.0 (40.0, 48.0) | 53.0 (50.0, 55.0) |
Female | 36.0 (28.0, 46.0) | 26.0 (24.0, 27.0) | 35.0 (32.0, 36.0) | 45.0 (41.0, 46.0) | 57.0 (51.0, 58.0) | |
Height (cm) | Male | 183.0 (178.0, 186.0) | 183.0 (174.0, 186.0) | 184.0 (180.0, 190.0) | 182.0 (180.0, 183.0) | 182.0 (178.0, 186.0) |
Female | 168.0 (164.0, 174.0) | 167.0 (161.0, 175.0) | 168.0 (166.0, 173.0) | 171.0 (164.0, 176.0) | 168.0 (162.0, 174.0) | |
Weight (kg) | Male | 87.0 (78.0, 93.0) | 80.0 (73.0, 88.0) | 88.0 (78.5, 91.5) | 89.0 (78.0, 99.0) | 92.0 (83.0, 96.0) |
Female | 63.0 (59.0, 69.0) | 60.0 (57.0, 65.0) | 63.5 (58.5, 70.0) | 63.0 (62.0, 65.0) | 70.0 (55.0, 78.0) | |
BMI (kg/m2) | Male | 25.5 (23.6, 27.2) | 24.8 (22.9, 26.5) | 24.9 (23.3, 26.2) | 25.4 (23.6, 30.6) | 26.3 (25.4, 29.8) |
Female | 21.9 (20.8, 24.5) | 21.7 (20.7, 22.6) | 21.7 (20.7, 24.8) | 22.8 (20.7, 24.7) | 23.8 (20.8, 28.7) | |
Total physical activity MET-minutes/week | Male | 1,866.0 (1,182.0, 2,815.0) |
2,622.0 (1,999.0, 3,920.0) |
2,043.0 (1,236.5, 2,849.5) |
1,280.0 (1,104.0, 1,566.0) |
1,533.0 (876.0, 5,239.0) |
Female | 1,680.0 (1,114.0, 2,527.0) |
1,781.5 (1,321.5, 2,513.5) |
1,692.5 (1,022.5, 2,229.0) |
1,893.0 (820.0, 3,000.0) |
1,459.5 (558.0, 2,106.0) |
Data are expressed as median (25th percentile, 75th percentile) for continuous data and as absolute frequency for categorical data. N, number of individuals; BMI, body mass index; MET, metabolic equivalent.
Assessment of reliability
Reliability of segmentation
The inter-rater mask agreement, expressed in terms of the Dice coefficient—an index of spatial mask overlap—showed values of around 92% for ES and PS. For MF, the inter-rater Dice coefficients varied between 84% and 88%, which was within an acceptable range. The maximum HD between the segmentation masks was on average 5 to 8 mm, corresponding to 4 to 7 voxels. The median of average HD ranged from 0.1 to 0.3 mm (Figure 3). Statistically significantly (P<0.001) lower inter-rater reliability was found for MF segmentation masks (based on the Dice coefficient and average HD) and higher inter-rater reliability was found for PS (HD, P<0.001).
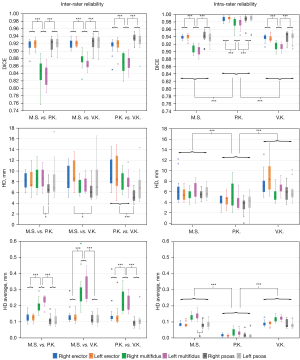
Intra-rater reliability of the segmentation masks showed robustness, as indicated by Dice coefficients greater than 90% (ranged from 91% to 99%) across raters, suggesting a substantial level of agreement. The maximum HD between the segmentation masks was on average 4 to 8 mm and the median of average HD ranged from 0.01 to 0.13 mm. The Dice coefficient (P<0.001) and average HD (P=0.048) showed statistically significantly lower intra-rater reliability for the MF segmentation masks. The data are presented in Figure 3.
Reliability of FF assessment
The ICC for inter-rater reliability of FF assessment ranged between 0.97 and 0.99 (Table 3); a subsequent Kruskal-Wallis analysis did not prove statistically significant differences (P>0.05) in FF between raters nor left-right dependency. The excellent reliability was also supported by the perfect correlation of FF values of individual muscles among raters (Pearson correlation coefficients exceeded values of 0.96); the low mean values of the absolute differences of FF between raters were 1.73%, and the low mean values of the relative differences of FF between raters were 16.22% (Table 3).
Table 3
Parameter | Raters | ||
---|---|---|---|
M.S. vs. P.K. | M.S. vs. V.K. | P.K. vs. V.K. | |
Fat fraction | |||
ICC (95% CI) | 0.968* (0.952–0.979) | 0.990* (0.984–0.993) | 0.976* (0.963–0.984) |
Mean abs (rel) difference (%) | 1.80 (17.82) | 1.50 (16.51) | 1.90 (14.34) |
Pearson r | 0.965* | 0.980* | 0.973* |
Total muscle volume | |||
ICC (95% CI) | 0.993* (0.989–0.995) | 0.995* (0.991–0.997) | 0.995* (0.988–0.997) |
Mean abs (cm3) [rel (%)] difference | 12.38 (7.63) | 10.21 (5.31) | 10.53 (6.25) |
Pearson r | 0.990* | 0.994* | 0.992* |
*, P values <0.001. M.S., P.K., V.K., initials of the raters. ICC, intraclass correlation coefficient; CI, confidence interval; abs, absolute; rel, relative.
Intra-rater reliability showed an even slightly higher agreement when assessing the FF of individual muscles. ICC exceeded the value of 0.99 (Table 4) and we did not prove any statistically significant differences (P>0.05) between raters nor right-left asymmetry. This internal consistency was further confirmed by the values of Pearson correlation coefficients (r≥0.99), indicating a perfect correlation between measurements; very low mean values of the absolute differences of FF between repeated measurements were on average 0.75%, and the low mean values of the relative differences of FF between repeated measurements were on average 6.46% (Table 4).
Table 4
Parameter | Raters | ||
---|---|---|---|
M.S. | P.K. | V.K. | |
Fat fraction | |||
ICC (95% CI) | 0.996* (0.993–0.998) | 0.997* (0.995–0.999) | 0.995* (0.992–0.998) |
Mean abs (rel) difference (%) | 0.82 (8.21) | 0.53 (4.14) | 0.89 (7.04) |
Pearson r | 0.990* | 0.990* | 0.986* |
Total muscle volume | |||
ICC (95% CI) | 0.999* (0.998–0.999) | 1.000* (0.999–1.000) | 0.998* (0.997–0.999) |
Mean abs (cm3) [rel (%)] difference | 5.40 (2.68) | 2.97 (1.68) | 7.11 (3.26) |
Pearson r | 0.998* | 0.999* | 0.996* |
*, P values <0.001. M.S., P.K., V.K., initials of the raters. ICC, intraclass correlation coefficient; CI, confidence interval; abs, absolute; rel, relative.
Reliability of TMV assessment
There was a very high level of inter-rater agreement for the volumetric assessment of each muscle, as confirmed by ICC (values greater than 0.99) and Pearson correlation coefficient (r≥0.99) (Table 3). A Kruskal-Wallis analysis did not prove any statistical differences (P>0.05) between raters nor right-left asymmetry. The mean values of absolute differences of volumes between raters were 11.04 cm3; the mean values of relative differences of volumes between raters were 6.40% (Table 3).
Excellent intra-rater agreement was again found for the volumetric analysis of individual muscles (ICC greater than 0.99) (Table 4) and was confirmed by Pearson correlation coefficient values (r higher than 0.99). A Kruskal-Wallis analysis again did not prove any statistical differences (P>0.05) between raters nor right-left asymmetry. The mean values of absolute differences of volumes between raters were 5.16 cm3; the mean values of relative differences of volumes between raters were 2.54% (Table 4).
Assessment of LPM morphological parameters
Table 5 shows the FF, TMV and FMV values for each muscle. The FF values for individual muscles varied significantly (P<0.001), with the lowest values found for the PS (median 6.1%), higher for the ES (median 8.3%), and the highest value found for the MF (median 11.1%). Regarding MV, the individual muscles again differed significantly (P<0.001). The smallest volumes were in MF (median 211 cm3 for TMV and 184 cm3 for FMV), followed by PS (median 374 cm3 for TMV and 342 cm3 for FMV) and the largest volumes were in ES (median 555 cm3 for TMV and 478 cm3 for FMV). The values of the individual parameters (FF, TMV, FMV) in relation to sex and four age categories (18–29, 30–39, 40–49, 50–70 years) can be found in the online supplementary material (Table S1).
Table 5
Characteristic | Erector spinae muscleA (N=90) | Multifidus muscleB (N=90) |
Psoas muscleC (N=90) |
P value | |||
---|---|---|---|---|---|---|---|
All# | A vs. B | B vs. C | A vs. C | ||||
Fat fraction (%) | 8.3 (5.8, 10.4) | 11.1 (7.0, 16.7) | 6.1 (5.2, 7.1) | <0.001* | <0.001* | <0.001* | <0.001* |
Total muscle volume (cm3) | 555 (430, 700) | 211 (184, 243) | 374 (272, 486) | <0.001* | <0.001* | <0.001* | <0.001* |
Functional muscle volume (cm3) | 478 (385, 610) | 184 (153, 211) | 342 (248, 452) | <0.001* | <0.001* | <0.001* | <0.001* |
Data are presented as median (interquartile range). The muscle volume is the sum of the right and left sides. #, Kruskal-Wallis rank sum test; Wilcoxon post-hoc P values with Holm adjustment method. *, statistically significant. N, number of individuals.
Factors influencing LPM morphological parameters
FF
The results of the univariable linear regression analysis, indicating how individual factors (age, sex, height, weight, BMI, and MET-min/week) contribute to FF variability in all three muscles, are summarised in Table 6. Age consistently showed a significant effect on FF in all muscles (P<0.001). Moreover, models with age as an explanatory variable had the highest percentage of FF variability explained (ranging from 39.6% to 44.8%) compared to other variables. Among anthropometric parameters, weight and BMI significantly affected FF of ES (for weight P=0.047, for BMI P=0.005) and PS (for weight P=0.001, for BMI P<0.001). Since weight and BMI are related covariates, BMI as a variable with higher R-squared value was used in the multivariable analysis. In contrast to anthropometric parameters, sex significantly contributed to FF variability in MF (P=0.026). MET-min/week, as a parameter reflecting physical activity, showed a borderline significant effect on FF (P=0.034), but this effect was only observed for one muscle (ES) and only when considering the logarithmic value of this parameter. Because of the marginal significance of this effect and the subjective nature of MET-min/week, we decided not to include this factor in the subsequent multivariable analysis.
Table 6
Variable | Erector spinae muscle | Multifidus muscle | Psoas muscle | |||||||||||
---|---|---|---|---|---|---|---|---|---|---|---|---|---|---|
Beta | 95% CI | P value | R2 (%) | Beta | 95% CI | P value | R2 (%) | Beta | 95% CI | P value | R2 (%) | |||
Age | 0.027 | 0.021 to 0.034 | <0.001* | 44.8 | 0.030 | 0.022 to 0.037 | <0.001* | 39.6 | 0.016 | 0.012 to 0.019 | <0.001* | 42.8 | ||
Height | −0.001 | −0.011 to 0.009 | 0.858 | 0.0 | −0.004 | −0.015 to 0.008 | 0.510 | 0.5 | 0.003 | −0.003 to 0.009 | 0.299 | 1.2 | ||
Weight | 0.006 | 0.000 to 0.012 | 0.047* | 4.4 | 0.001 | −0.006 to 0.008 | 0.725 | 0.1 | 0.006 | 0.002 to 0.009 | 0.001* | 11.0 | ||
BMI | 0.036 | 0.011 to 0.062 | 0.005* | 8.7 | 0.013 | −0.016 to 0.043 | 0.375 | 0.9 | 0.027 | 0.012 to 0.041 | <0.001* | 13.7 | ||
Sex | 0.236 | 1.6 | 0.026* | 5.5 | 0.420 | 0.7 | ||||||||
Male | – | – | – | – | – | – | ||||||||
Female | 0.117 | −0.078 to 0.311 | 0.249 | 0.031 to 0.467 | −0.046 | −0.160 to 0.067 | ||||||||
MET-100 minutes/week | −0.006 | −0.013 to 0.000 | 0.057 | 4.0 | −0.006 | −0.013 to 0.001 | 0.114 | 2.8 | −0.003 | −0.007 to 0.001 | 0.136 | 2.5 | ||
MET-minutes/week (log scale) | −0.098 | −0.189 to −0.007 | 0.034* | 5.0 | −0.074 | −0.179 to 0.032 | 0.168 | 2.1 | −0.044 | −0.097 to 0.009 | 0.103 | 3.0 |
*, statistically significant. CI, confidence interval; R2, R-squared; BMI, body mass index; MET, metabolic equivalent.
The results of the multivariable regression analysis fitting the most significant explanatory variables from the univariable models are summarised in Table 7. Same as the univariable analysis, age showed the highest effect on FF that was positive in all muscles, i.e., FF significantly increases (P<0.001) with age (Figure 4). Similarly, the FF ascends with increasing BMI (for PS and ES) and is higher for females than for males (for MF and ES) (Figure 4).
Table 7
Variable | Erector spinae muscle | Multifidus muscle | Psoas muscle | ||||||||
---|---|---|---|---|---|---|---|---|---|---|---|
Beta | 95% CI | P value | Beta | 95% CI | P value | Beta | 95% CI | P value | |||
(Intercept) | −4.336 | −4.890 to −3.781 | <0.001* | −3.698 | −4.370 to −3.026 | <0.001* | −3.729 | −4.063 to −3.395 | <0.001* | ||
Age | 0.025 | 0.019 to 0.031 | <0.001* | 0.029 | 0.021 to 0.037 | <0.001* | 0.014 | 0.010 to 0.018 | <0.001* | ||
BMI | 0.031 | 0.009 to 0.052 | 0.005* | 0.008 | −0.018 to 0.034 | 0.534 | 0.017 | 0.004 to 0.029 | 0.012* | ||
Sex | |||||||||||
Male | – | – | – | – | – | – | |||||
Female | 0.217 | 0.062 to 0.372 | 0.007* | 0.276 | 0.088 to 0.464 | 0.004* | 0.008 | −0.085 to 0.101 | 0.865 | ||
R2 (%) | 51.1 | 45.4 | 47.5 |
*, statistically significant. CI, confidence interval; BMI, body mass index; R2, R-squared.
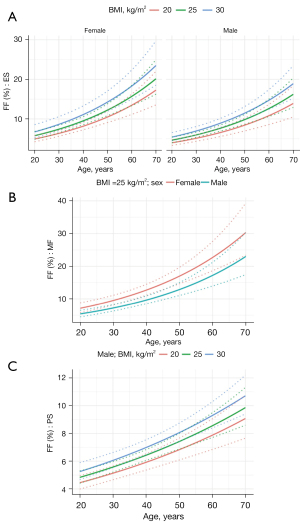
Based on the multivariable models, equation (Eq. [4]) was built to calculate the predicted FF (FFpred) for each muscle in an individual of a given age, BMI, and sex:
Where β0 is the model intercept, β1 is the beta coefficient for age in years, β2 is the beta coefficient for BMI in kg/m2, β3 is the beta coefficient for sex (1 = female, 0 = male). Based on values of individual beta coefficients and 95% prediction interval bounds, we can calculate the predicted range of FF in healthy individuals as (Eq. [5]):
Where σ is the average variance of the prediction interval.
MV
The results of the univariable linear regression analysis showing how individual physiological factors contribute to the variability of TMV for all three muscles are shown in Table 8. Surprisingly, age failed to reach a significant effect on TMV of LPM and PS in the univariable linear regression models. However, as age is an important demographic factor, we included it in the subsequent multivariable analysis (see below). Among anthropometric parameters, weight demonstrated the highest R-squared values, explaining a substantial portion of the TMV variability, ranging from 36.3% to 67.6% (P<0.001). Therefore, weight was included as an anthropometric parameter in multivariable models (TMV increased with weight). Sex showed significant and consistent effects on TMV of all three muscles (lower TMV in women) (P<0.001). Similar to FF, MET-min/week showed no significant effect on TMV. Thus, MET-min/week was again excluded from subsequent multivariable analysis.
Table 8
Variable | Erector spinae muscle | Multifidus muscle | Psoas muscle | |||||||||||
---|---|---|---|---|---|---|---|---|---|---|---|---|---|---|
Beta | 95% CI | P value | R2 (%) | Beta | 95% CI | P value | R2 (%) | Beta | 95% CI | P value | R2 (%) | |||
Age | 0.002 | −0.003 to 0.007 | 0.485 | 0.6 | 0.003 | −0.002 to 0.007 | 0.230 | 1.6 | −0.006 | −0.012 to 0.001 | 0.086 | 3.3 | ||
Height | 0.022 | 0.018 to 0.026 | <0.001* | 55.1 | 0.015 | 0.011 to 0.020 | <0.001* | 37.1 | 0.023 | 0.017 to 0.029 | <0.001* | 40.1 | ||
Weight | 0.015 | 0.013 to 0.017 | <0.001* | 67.6 | 0.009 | 0.007 to 0.012 | <0.001* | 36.3 | 0.016 | 0.012 to 0.019 | <0.001* | 47.6 | ||
BMI | 0.045 | 0.031 to 0.058 | <0.001* | 33.7 | 0.025 | 0.012 to 0.038 | <0.001* | 14.8 | 0.046 | 0.028 to 0.064 | <0.001* | 23.4 | ||
Sex | <0.001* | 62.8 | <0.001* | 24.7 | <0.001* | 57.1 | ||||||||
Male | — | — | — | — | — | — | ||||||||
Female | −0.456 | −0.531 to −0.382 | −0.243 | −0.333 to −0.153 | −0.538 | −0.637 to −0.439 | ||||||||
MET-100 minutes/week | 0.000 | −0.004 to 0.004 | 0.888 | 0.0 | 0.001 | −0.002 to 0.005 | 0.452 | 0.6 | 0.000 | −0.005 to 0.005 | 0.959 | 0.0 | ||
MET-minutes/week (log scale) | −0.037 | −0.095 to 0.020 | 0.199 | 1.9 | −0.007 | −0.057 to 0.042 | 0.768 | 0.1 | −0.036 | −0.107 to 0.036 | 0.322 | 1.1 |
*, statistically significant. CI, confidence interval; R2, R-squared; BMI, body mass index; MET, metabolic equivalent.
The results of the multivariable analysis with age, weight, and sex as explanatory variables are shown in Table 9. Weight evinced significant effects (P<0.001) on TMV of all muscles, while TMV increased with increasing weight (Figure 5). The effect of age on TMV was found only in PS (decrease with age), sex influenced TMV of ES and PS (lower volume in women). We calculated the FMV (MV without fat) and repeated the regression analysis; the results of both the univariable and multivariable regression analyses are shown in Tables 10,11. In multivariable analysis, weight, age and sex significantly influenced FMV in all 3 muscles (ES, MF and PS), increasing with weight, greater in men and decreasing with age (Figure 6).
Table 9
Variable | Erector spinae muscle | Multifidus muscle | Psoas muscle | ||||||||
---|---|---|---|---|---|---|---|---|---|---|---|
Beta | 95% CI | P value | Beta | 95% CI | P value | Beta | 95% CI | P value | |||
(Intercept) | 5.697 | 5.457 to 5.937 | <0.001* | 4.757 | 4.422 to 5.092 | <0.001* | 5.644 | 5.299 to 5.990 | <0.001* | ||
Age | −0.001 | −0.004 to 0.002 | 0.570 | 0.001 | −0.003 to 0.005 | 0.718 | −0.008 | −0.012 to −0.004 | <0.001* | ||
Weight | 0.010 | 0.007 to 0.013 | <0.001* | 0.008 | 0.004 to 0.012 | <0.001* | 0.010 | 0.005 to 0.014 | <0.001* | ||
Sex | |||||||||||
Male | – | – | – | – | – | – | |||||
Female | −0.240 | −0.325 to −0.154 | <0.001* | −0.075 | −0.194 to 0.044 | 0.214 | −0.330 | −0.453 to −0.207 | <0.001* | ||
R2 (%) | 76.8 | 37.4 | 68.5 |
*, statistically significant. CI, confidence interval; R2, R-squared.
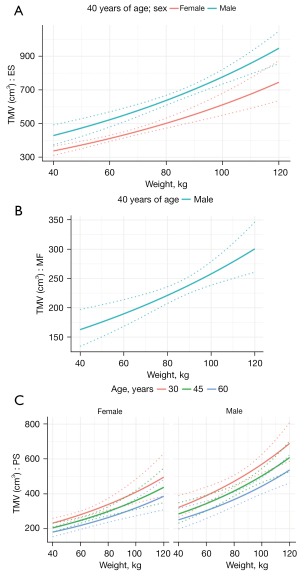
Table 10
Variable | Erector spinae muscle | Multifidus muscle | Psoas muscle | |||||||||||
---|---|---|---|---|---|---|---|---|---|---|---|---|---|---|
Beta | 95% CI | P value | R2 (%) | Beta | 95% CI | P value | R2 (%) | Beta | 95% CI | P value | R2 (%) | |||
Age | −0.002 | −0.007 to 0.004 | 0.569 | 0.4 | −0.002 | −0.007 to 0.003 | 0.377 | 0.9 | −0.007 | −0.013 to 0.000 | 0.038* | 4.8 | ||
Height | 0.022 | 0.018 to 0.027 | <0.001* | 54.2 | 0.016 | 0.012 to 0.020 | <0.001* | 42.2 | 0.023 | 0.017 to 0.029 | <0.001* | 39.1 | ||
Weight | 0.014 | 0.012 to 0.017 | <0.001* | 57.7 | 0.009 | 0.007 to 0.012 | <0.001* | 34.9 | 0.015 | 0.012 to 0.019 | <0.001* | 44.6 | ||
BMI | 0.039 | 0.025 to 0.053 | <0.001* | 25.0 | 0.022 | 0.009 to 0.035 | 0.001* | 11.6 | 0.044 | 0.026 to 0.062 | <0.001* | 21.1 | ||
Sex | <0.001* | 64.1 | <0.001* | 33.3 | <0.001* | 56.0 | ||||||||
Male | – | – | – | – | – | – | ||||||||
Female | −0.469 | −0.543 to −0.394 | −0.280 | −0.364 to −0.196 | −0.534 | −0.635 to −0.434 | ||||||||
MET-100 minutes/week | 0.001 | −0.003 to 0.005 | 0.530 | 0.4 | 0.002 | −0.001 to 0.006 | 0.156 | 2.3 | 0.000 | −0.005 to 0.005 | 0.966 | 0.0 | ||
MET-minutes/week (log scale) | −0.022 | −0.081 to 0.037 | 0.455 | 0.6 | 0.007 | −0.042 to 0.056 | 0.771 | 0.1 | −0.032 | −0.104 to 0.039 | 0.371 | 0.9 |
*, statistically significant. CI, confidence interval; R2, R-squared; BMI, body mass index; MET, metabolic equivalent.
Table 11
Variable | Erector spinae muscle | Multifidus muscle | Psoas muscle | ||||||||
---|---|---|---|---|---|---|---|---|---|---|---|
Beta | 95% CI | P value | Beta | 95% CI | P value | Beta | 95% CI | P value | |||
Intercept | 5.856 | 5.597 to 6.116 | <0.001* | 4.897 | 4.579 to 5.214 | <0.001* | 5.656 | 5.306 to 6.005 | <0.001* | ||
Age | −0.004 | −0.007 to −0.001 | 0.011* | −0.004 | −0.008 to 0.000 | 0.037* | −0.009 | −0.013 to −0.005 | <0.001* | ||
Weight | 0.008 | 0.005 to 0.011 | <0.001* | 0.007 | 0.003 to 0.010 | <0.001* | 0.009 | 0.005 to 0.013 | <0.001* | ||
Sex | |||||||||||
Male | – | – | – | – | – | – | |||||
Female | −0.285 | −0.377 to −0.193 | <0.001* | −0.132 | −0.245 to −0.019 | 0.022* | −0.336 | −0.461 to −0.212 | <0.001* | ||
R2 (%) | 73.8 | 43.0 | 68.1 |
*, statistically significant. CI, confidence interval; R2, R-squared.
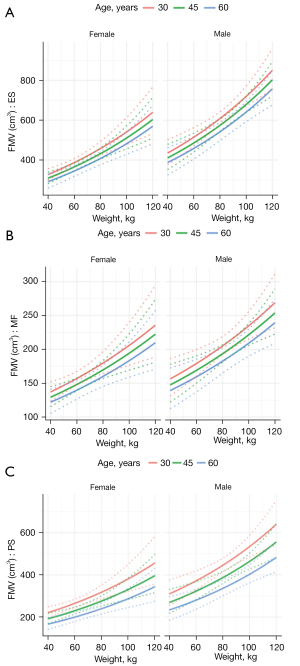
Based on the multivariable models, equations (Eqs. [6,7]) were built to calculate the predicted TMV and FMV (TMVpred, FMVpred) for an individual with particular age, weight and sex:
Where β0 is the model intercept, β1 is the beta coefficient for age in years, β2 is the beta coefficient for weight in kilograms, β3 is the beta coefficient for sex (1 = female, 0 = male). Based on values of individual beta coefficients and 95% prediction interval bounds the predicted range of TMV and FMV in healthy individuals can be calculated as (Eqs. [8,9]):
Where σ is the average variance of the prediction interval.
The calculator for predicted FF, TMV and FMV values of individual muscles (ES, MF, PS) with prediction intervals is available in https://cdn.amegroups.cn/static/public/qims-23-1796-1.xlsx.
Discussion
In this study, we developed a method to assess morphology of LPM (ES and MF) and the control muscle (PS) using an advanced MRI method (6-point Dixon gradient echo sequence). This method was found to be reliable for creating segmentation masks and for assessing FF and TMV. The values of basic morphological parameters (biomarkers, i.e., FF and MV) of these muscles in our cohort of healthy volunteers are presented and the main physiological factors influencing these parameters were determined. The FF was influenced the most by age; the MV was influenced most by the weight and sex of the individual. We developed equations to calculate the predicted FF, TMV and FMV values for each muscle as a function of the individual’s independent physiological variables.
The agreement of the segmentation masks in our study reached high values for the Dice coefficient, low values (high agreement) for the average HD in the case of inter-rater reliability, and, according to the assumption, an even better agreement was shown in the case of intra-rater reliability. Both of these parameters are very sensitive to the number of voxels in the segmented masks. This could be one reason MF had worse results than other bigger muscles. In addition to the size of the muscle, the LPM (ES and MF) are not encapsulated by their own independent layer of epimysium, complicating their identification and delineation (27). When we examined the reproducibility of FF and MV, we observed excellent ICC values (ICC >0.96) and perfect correlations among all raters and between the raters themselves (r>0.96), which is in line with the results of previous research on this topic (12,18,32,33). The chosen segmentation methodology is tedious; nevertheless, this step was necessary for the future creation of a fully automated machine learning-based method, which is a challenge in this anatomical field. Several works on this topic exist and this approach could improve the already high reproducibility of segmentation and save hours of physician work (34,35).
The reproducibility is high but the accuracy of quantification of FF is an unresolved issue. Several different approaches can be found, each with advantages and disadvantages. We decided to use the mDixon Quant sequence because its accuracy has been evaluated in previous studies with very good results (36,37). For a good segmentation, a high resolution and tissue contrast is necessary; for a good quantification of fat, a high signal-to-noise ratio is also necessary; these are two contradictory conditions. For this reason, we decided not to perform data acquisition by fast acquisition with breath held as in some other publications (9,12). Since we are primarily interested in the area of muscles located around the spine, we chose the direction of phase encoding right-to-left with sufficient oversampling. This ensures that breathing artefacts remain primarily in the anterior region of the image, and we can capture the image longer (more signal averaging), with higher resolution, sufficient signal, and minimal motion artefacts in the ROI.
Comparing values of FF and MV between studies is challenging due to methodological differences and variations in study cohorts, including sex, age, height, and weight, which can significantly affect MV and FF measurements. For MV, the range of lumbar levels assessed is also crucial. Our study is notable for using a precise 6-point Dixon method and manual segmentation to assess the morphological parameters of the individual muscles across their entire volume in L1 to L5 levels, as opposed to single level assessments since parameters vary at different vertebral levels (9).
Comparing our results with the study by Crawford et al. (9), our research reported FF values for MF and ES that were on average one-third to one-half lower. TMV in our research was approximately 15% lower for MF, and TMV for ES was about 28% higher. This discrepancy may be due to the use of the less accurate 2-point Dixon sequences and different segmentation methods with a semi-automated approach by Crawford et al. (9), and most importantly, a different segmentation range, which may contribute to the variation between studies. Valentin et al. (33) also reported higher FF values for LPM muscles, probably due to differences in measurement methods. To analyse FF, the study by Valentin et al. (33) used a T1 sequence and relied on relative comparisons with subcutaneous fat areas, making values from this work incomparable with those obtained in other studies. The study by Baum et al. (38) reported FF values for the PS of 4.1% (an average for both sides), which is relatively similar to our results (6.1%), although our values are up to half as high due to the low FF values in PS. However, Baum et al. (38) included only 10 participants with a significantly younger average age (29 years), which may explain the lower FF values observed. A study by Sollmann et al. (12) achieved comparable FF values for PS (men: 5.1%, women: 6.0%) to our study, with a closely matched cohort in terms of age and BMI. However, like Baum et al. (38), Sollmann et al. (12) combined MF and ES into a single muscle, which affect direct comparisons of the values. Using the same imaging modality (MRI), Modesto et al. (39) showed TMV values for PS that were approximately 30% lower than ours due to differences in volume assessment methodology, age composition (healthy volunteers in our cohort were younger) and, in particular, different segmentation ranges.
Many studies have investigated the influence of physiological factors (age, sex, weight, height, BMI, and physical activity) on muscle morphological parameters, but the results are inconsistent and often contradictory. In this study, we found that age was the main factor influencing muscle FF in adults, accounting for about 40–45% of the FF variability. We have demonstrated that FF of LPM and also PS increases with age, which is in line with most of the previous studies (9-17,40-42). Among the anthropometric factors, FF of PS and ES was most influenced by BMI, and FF increased with increasing BMI. However, we could not demonstrate an effect of BMI on FF in MF. Sollmann et al. (12) showed that LPM FF (ES and MF were evaluated as a single muscle) at level L3–L5 was independent of BMI, which was not the case for the other segments (T12–L2 or T9–T11) or PS. No significant correlation between BMI and FF of LPM was demonstrated in other studies (9,10). In line with the findings of this study, the proportion of FF has been described as higher among women in LPM (9,11,16,17,43). On the other hand, Burian et al., like us, did not find any significant differences between men and women in FF values of PS (43). It has been repeatedly described that the association between the level of physical activity in daily life and the paraspinal fat replacement is negligible or even non-existent (9,10), which we also confirmed in our study. The fact that LPM are minimally affected by general physical activity is also supported by the results of our previous study, which found no correlation between physical activity and muscle strength and muscular endurance of the lower back extensor muscles, which include ES and MF (44). The reason for this may be due to the different representation of muscle fibres; the feature of LPM is the predominance of slow‐twitch fibres (type 1) compared to the limb muscles (including PS) with predominant fast-twitch fibres (type 2) (45,46). Slow‐twitch fibres determine the postural function of LPM; fast-twitch fibres determine phasic movements of the limb muscles.
Ordinary physical activities and sports involve mostly phasic movements, which do not target slow-twitch fibres. Only certain types of exercise appear to involve paraspinal muscles in their postural function (3,47). It can be assumed that those exercises that specifically target the deep spinal stabilisation system are needed to improve the conditioning of LPM in primary and secondary prevention, which was confirmed in our previous study (48).
Very few studies have evaluated MV as a biomarker of muscle damage, with most preferring muscle CSA as it is easier to measure and does not require time-consuming muscle segmentation (9,32,33). However, the values for muscle CSA are dependent on the lumbar level at which they are assessed (CSA of ES decreases at lower segments) (15). Thus, MV appears to be a much more reliable marker than CSA, and three-dimensional volumetric measures are expected to be more closely related to muscle function than two-dimensional measures (32). It was reported that TMV of MF and ES are lower in women than in men (9), which we confirmed for PS and ES, but not for MF (no effect of sex on TMV was found for this muscle in the multivariable analysis). Another study evaluating factors influencing lumbar spine muscle TMV concluded that height was a highly significant factor influencing PS, MF, and ES volume (33), which was also demonstrated in the present study. However, our study showed a slightly greater effect of weight on TMV compared to height. The results of the analysis of the effect of age on MV of LPM vary widely among studies; Crawford et al. concluded that TMV of MF and ES are independent of age (9), and Valentin et al. described the effect of age only on TMV of MF (volume decreased with increasing age), but not on TMV of ES and PS (33). In our study, there was no significant effect of age on TMV of LPM, but TMV of PS decreased with age. It is known that ageing causes significant changes in skeletal muscle composition, with a marked increase in non-contractile tissue (intramuscular fat and connective tissue) and a decrease in contractile tissue; the consequence is loss of intrinsic muscle strength typically observed at older age (49). Such a remodelling process causes a disproportionate age-associated decrease of force compared to that of TMV (49). Significant atrophy of LPM can occur without a reduction in the total CSA within the muscles’ fascial boundaries, so paraspinal muscle atrophy is described in terms of the replacement of muscle with fat and fibrous tissue, resulting in reduced functional contractility of muscle (50-52). Therefore, a measure of the FMV would be a better indicator of the muscle’s contractile ability and could reveal the real atrophy of the muscle (53). In our study, there was a significant negative effect of age on FMV in both LPM and PS. The graphs of predicted FMV show that the FMV for all the muscles evaluated is lowest at age 60, higher at age 45, and highest at age 30. This correlates with previously published data on natural ageing and sarcopenia which suggests that the age of 30–40 years is the point at which a slow but continuous loss of muscle mass (FMV) becomes significant, accelerating by the age of 60–70 years (54-56). FMV was also significantly influenced by weight (increasing with weight) and gender (higher values in men) for both LPM and PS in our study. Few studies have used regression models to analyse the relationship between paraspinal muscle morphology and demographic, anthropometrics factors, and level of physical activity. In concordance with our results, no association between level of physical activity and paraspinal muscle morphology was found (33,42) and FF increased with increasing age and was higher in females (11). However, comparison with our study is rather complex due to substantial differences in demographic differences in subjects screened and the methodology used. Khil et al. did not differentiate MF and ES, calculated CSA and not MV, and the mean age of subjects screened was lower (34.3 years) than in our study (11). Fortin et al. obtained data only in men, only in L3–4 and L5–S1 segments, and calculated CSA instead of MV (42); similar to the study by Valentin et al. (33), muscle fat infiltration was computed as percentage of pixel intensity in fat tissue. Moreover, Valentin et al. used BMI and height, which are covariates of interest, in one multivariable regression model, which could lead to distorted results (33). In our study, the multivariable regression models were used to establish equations to calculate predicted FF, TMV and FMV of individual muscles based on the physiological factors of the individual. We believe that these equations will help to simplify the detection of morphological abnormalities in LPM and we anticipate their use in routine clinical practice.
The present study has some limitations. The main limitation is the limited number of subjects enrolled. For this reason, the values presented for the morphological parameters of individual muscles cannot be considered as norms, but only as values found in our cohort of healthy subjects, and the calculation of predicted values is based only on this healthy cohort. In a second step, we also need to test the established parameters in individuals where we expect LPM involvement, such as patients with chronic low back pain or neuromuscular diseases. However, to the best of our knowledge, this study is among those with the largest number of healthy subjects, selected according to strict criteria to exclude parameters that could influence muscle parameters. Equal representation of males and females was maintained in the recruitment of individuals, as was the accurate age stratification of the cohort. The strengths of this study are the use of a 6-point Dixon gradient echo sequence, the evaluation of physiological variables that influence the morphological parameters of LPM, and the provision of equations for calculating the FF, TMV and FMV values of given muscle based on the individual’s characteristics. We also believe that the improvement of the manual segmentation methodology for individual muscles will be useful in developing a methodology for automatic segmentation of these muscles using artificial intelligence. We are already working on this. The knowledge of paraspinal muscle biomarker values (also in relation to the physiological characteristics of the individual) determined by MRI will be used in the diagnosis of LPM damage in various diseases, including low back pain and neuromuscular diseases. It can also be used to monitor the dynamics of disease and the effect of treatment, as well as to select the optimal rehabilitation programme and exercises. Furthermore, assessing LPM in patients with low back pain will help to understand the pathophysiology of this very common condition.
Conclusions
A methodology has been developed to assess the morphological parameters of LPM (ES and MF) using a 6-point Dixon gradient echo sequence, including the segmentation of these muscles. The high level of reliability of this methodology has been confirmed. An analysis of the physiological factors that have an influence on the FF and MV of these muscles was carried out. The FF of LPM was most influenced by age and MV was most influenced by the weight and sex of the individual. The results of this study suggest the expected values of LPM morphological parameters in a healthy population, thus helping to detect their abnormalities. Equations were developed to calculate the predicted FF of LPM in relation to age, BMI and sex, and the predicted TMV and FMV of LPM in relation to age, weight and sex of the individual. We believe that the results of this study could improve our understanding of the pathophysiology of low back pain, in which LPM are thought to play an important role, as well as the possible involvement of these muscles in some neuromuscular diseases.
Acknowledgments
Funding: The study was supported by
Footnote
Reporting Checklist: The authors have completed the STROBE reporting checklist. Available at https://qims.amegroups.com/article/view/10.21037/qims-23-1796/rc
Conflicts of Interest: All authors have completed the ICMJE uniform disclosure form (available at https://qims.amegroups.com/article/view/10.21037/qims-23-1796/coif). P.K., V.K., D.V., K.M. and B.A. report the funding from the Ministry of Health of the Czech Republic Project for Conceptual Development in Research Organizations (No. 65269705) (University Hospital Brno, Brno, Czech Republic) and the project (MUNI/A/1186/2022). And they are members of the European Reference Network for Neuromuscular Diseases (No. 870177). M.D., M.K., M.S., R.G. also report the funding from the Ministry of Health of the Czech Republic project for conceptual development in research organizations (No. 65269705) (University Hospital Brno, Brno, Czech Republic). P.O. reports the employment relationship with the Institute of Biostatistics and Analyses Ltd., Brno, Czech Republic. The authors have no other conflicts of interest to declare.
Ethical Statement: The authors are accountable for all aspects of the work in ensuring that questions related to the accuracy or integrity of any part of the work are appropriately investigated and resolved. The Ethics Committee of the University Hospital Brno approved the study protocol (No. 05-090621/EK), and written informed consent was obtained from all participants. The study was conducted in accordance with the Declaration of Helsinki (as revised in 2013).
Open Access Statement: This is an Open Access article distributed in accordance with the Creative Commons Attribution-NonCommercial-NoDerivs 4.0 International License (CC BY-NC-ND 4.0), which permits the non-commercial replication and distribution of the article with the strict proviso that no changes or edits are made and the original work is properly cited (including links to both the formal publication through the relevant DOI and the license). See: https://creativecommons.org/licenses/by-nc-nd/4.0/.
References
- Panjabi MM. A hypothesis of chronic back pain: ligament subfailure injuries lead to muscle control dysfunction. Eur Spine J 2006;15:668-76. [Crossref] [PubMed]
- Freeman MD, Woodham MA, Woodham AW. The role of the lumbar multifidus in chronic low back pain: a review. PM R 2010;2:142-6; quiz 1 p following 167.
- Steele J, Bruce-Low S, Smith D. A reappraisal of the deconditioning hypothesis in low back pain: review of evidence from a triumvirate of research methods on specific lumbar extensor deconditioning. Curr Med Res Opin 2014;30:865-911. [Crossref] [PubMed]
- Diamanti L, Paoletti M, Vita UD, Muzic SI, Cereda C, Ballante E, Pichiecchio A. MRI study of paraspinal muscles in patients with Amyotrophic Lateral Sclerosis (ALS). J Clin Med 2020;9:934. [Crossref] [PubMed]
- Witting N, Andersen LK, Vissing J. Axial myopathy: an overlooked feature of muscle diseases. Brain 2016;139:13-22. [Crossref] [PubMed]
- Kalichman L, Hodges P, Li L, Guermazi A, Hunter DJ. Changes in paraspinal muscles and their association with low back pain and spinal degeneration: CT study. Eur Spine J 2010;19:1136-44. [Crossref] [PubMed]
- Carlier PG, Marty B, Scheidegger O, Loureiro de Sousa P, Baudin PY, Snezhko E, Vlodavets D. Skeletal Muscle Quantitative Nuclear Magnetic Resonance Imaging and Spectroscopy as an Outcome Measure for Clinical Trials. J Neuromuscul Dis 2016;3:1-28. [Crossref] [PubMed]
- Barnard AM, Willcocks RJ, Triplett WT, Forbes SC, Daniels MJ, Chakraborty S, Lott DJ, Senesac CR, Finanger EL, Harrington AT, Tennekoon G, Arora H, Wang DJ, Sweeney HL, Rooney WD, Walter GA, Vandenborne K. MR biomarkers predict clinical function in Duchenne muscular dystrophy. Neurology 2020;94:e897-909. [Crossref] [PubMed]
- Crawford RJ, Filli L, Elliott JM, Nanz D, Fischer MA, Marcon M, Ulbrich EJ. Age- and Level-Dependence of Fatty Infiltration in Lumbar Paravertebral Muscles of Healthy Volunteers. AJNR Am J Neuroradiol 2016;37:742-8. [Crossref] [PubMed]
- Dahlqvist JR, Vissing CR, Hedermann G, Thomsen C, Vissing J. Fat Replacement of Paraspinal Muscles with Aging in Healthy Adults. Med Sci Sports Exerc 2017;49:595-601. [Crossref] [PubMed]
- Khil EK, Choi JA, Hwang E, Sidek S, Choi I. Paraspinal back muscles in asymptomatic volunteers: quantitative and qualitative analysis using computed tomography (CT) and magnetic resonance imaging (MRI). BMC Musculoskelet Disord 2020;21:403. [Crossref] [PubMed]
- Sollmann N, Zoffl A, Franz D, Syväri J, Dieckmeyer M, Burian E, Klupp E, Hedderich DM, Holzapfel C, Drabsch T, Kirschke JS, Rummeny EJ, Zimmer C, Hauner H, Karampinos DC, Baum T. Regional variation in paraspinal muscle composition using chemical shift encoding-based water-fat MRI. Quant Imaging Med Surg 2020;10:496-507. [Crossref] [PubMed]
- Lee SH, Park SW, Kim YB, Nam TK, Lee YS. The fatty degeneration of lumbar paraspinal muscles on computed tomography scan according to age and disc level. Spine J 2017;17:81-7. [Crossref] [PubMed]
- Takayama K, Kita T, Nakamura H, Kanematsu F, Yasunami T, Sakanaka H, Yamano Y. New Predictive Index for Lumbar Paraspinal Muscle Degeneration Associated With Aging. Spine (Phila Pa 1976) 2016;41:E84-90. [Crossref] [PubMed]
- Urrutia J, Besa P, Lobos D, Andia M, Arrieta C, Uribe S. Is a single-level measurement of paraspinal muscle fat infiltration and cross-sectional area representative of the entire lumbar spine? Skeletal Radiol 2018;47:939-45. [Crossref] [PubMed]
- Huang R, Pan F, Kong C, Lu S. Age- and sex-dependent differences in the morphology and composition of paraspinal muscles between subjects with and without lumbar degenerative diseases. BMC Musculoskelet Disord 2022;23:734. [Crossref] [PubMed]
- Sasaki T, Yoshimura N, Hashizume H, Yamada H, Oka H, Matsudaira K, Iwahashi H, Shinto K, Ishimoto Y, Nagata K, Teraguchi M, Kagotani R, Muraki S, Akune T, Tanaka S, Kawaguchi H, Nakamura K, Minamide A, Nakagawa Y, Yoshida M. MRI-defined paraspinal muscle morphology in Japanese population: The Wakayama Spine Study. PLoS One 2017;12:e0187765. [Crossref] [PubMed]
- Zhang Y, Zhou Z, Wang C, Cheng X, Wang L, Duanmu Y, Zhang C, Veronese N, Guglielmi G. Reliability of measuring the fat content of the lumbar vertebral marrow and paraspinal muscles using MRI mDIXON-Quant sequence. Diagn Interv Radiol 2018;24:302-7. [Crossref] [PubMed]
- Han G, Jiang Y, Zhang B, Gong C, Li W. Imaging Evaluation of Fat Infiltration in Paraspinal Muscles on MRI: A Systematic Review with a Focus on Methodology. Orthop Surg 2021;13:1141-8. [Crossref] [PubMed]
- Goutallier D, Postel JM, Bernageau J, Lavau L, Voisin MC. Fatty muscle degeneration in cuff ruptures. Pre- and postoperative evaluation by CT scan. Clin Orthop Relat Res 1994;78-83.
- Mercuri E, Counsell S, Allsop J, Jungbluth H, Kinali M, Bonne G, Schwartz K, Bydder G, Dubowitz V, Muntoni F. Selective muscle involvement on magnetic resonance imaging in autosomal dominant Emery-Dreifuss muscular dystrophy. Neuropediatrics 2002;33:10-4. [Crossref] [PubMed]
- Solbakken G, Bjørnarå B, Kirkhus E, Nguyen B, Hansen G, Frich JC, Ørstavik K. MRI of trunk muscles and motor and respiratory function in patients with myotonic dystrophy type 1. BMC Neurol 2019;19:135. [Crossref] [PubMed]
- Perkins TG, Duijndam A, Eggers H, Weerdt E, Rijckaert YHE. The next generation fat-free imaging. 2015. Available online: https://philipsproductcontent.blob.core.windows.net/assets/20170523/77840f58014b4ea8bc44a77c015697b7.pdf
- Burian E, Rohrmeier A, Schlaeger S, Dieckmeyer M, Diefenbach MN, Syväri J, Klupp E, Weidlich D, Zimmer C, Rummeny EJ, Karampinos DC, Kirschke JS, Baum T. Lumbar muscle and vertebral bodies segmentation of chemical shift encoding-based water-fat MRI: the reference database MyoSegmenTUM spine. BMC Musculoskelet Disord 2019;20:152. [Crossref] [PubMed]
- Sjostrom M, Ainsworth B, Bauman A, Bull F, Hamilton-Craig C, Sallis J. Guidelines for data processing analysis of the International Physical Activity Questionnaire (IPAQ) - Short and long forms. 2005. Available online: https://www.semanticscholar.org/paper/Guidelines-for-data-processing-analysis-of-the-and-Sjostrom-Ainsworth/efb9575f5c957b73c640f00950982e618e31a7be
- Yushkevich PA, Piven J, Hazlett HC, Smith RG, Ho S, Gee JC, Gerig G. User-guided 3D active contour segmentation of anatomical structures: significantly improved efficiency and reliability. Neuroimage 2006;31:1116-28. [Crossref] [PubMed]
- Crawford RJ, Cornwall J, Abbott R, Elliott JM. Manually defining regions of interest when quantifying paravertebral muscles fatty infiltration from axial magnetic resonance imaging: a proposed method for the lumbar spine with anatomical cross-reference. BMC Musculoskelet Disord 2017;18:25. [Crossref] [PubMed]
- Weinreb JC, Cohen JM, Maravilla KR. Iliopsoas muscles: MR study of normal anatomy and disease. Radiology 1985;156:435-40. [Crossref] [PubMed]
- Taha AA, Hanbury A. Metrics for evaluating 3D medical image segmentation: analysis, selection, and tool. BMC Med Imaging 2015;15:29. [Crossref] [PubMed]
- Akoglu H. User's guide to correlation coefficients. Turk J Emerg Med 2018;18:91-3. [Crossref] [PubMed]
- Koo TK, Li MY. A Guideline of Selecting and Reporting Intraclass Correlation Coefficients for Reliability Research. J Chiropr Med 2016;15:155-63. [Crossref] [PubMed]
- Rummens S, Bosch S, Dierckx S, Vanmechelen A, Peeters R, Brumagne S, Desloovere K, Peers K. Reliability and agreement of lumbar multifidus volume and fat fraction quantification using magnetic resonance imaging. Musculoskelet Sci Pract 2022;59:102532. [Crossref] [PubMed]
- Valentin S, Licka T, Elliott J. Age and side-related morphometric MRI evaluation of trunk muscles in people without back pain. Man Ther 2015;20:90-5. [Crossref] [PubMed]
- Dourthe B, Shaikh N, Pai S A, Fels S, Brown SHM, Wilson DR, Street J, Oxland TR. Automated Segmentation of Spinal Muscles From Upright Open MRI Using a Multiscale Pyramid 2D Convolutional Neural Network. Spine (Phila Pa 1976) 2022;47:1179-86. [Crossref] [PubMed]
- Li H, Luo H, Liu Y. Paraspinal Muscle Segmentation Based on Deep Neural Network. Sensors (Basel) 2019;19:2650. [Crossref] [PubMed]
- Kise Y, Chikui T, Yamashita Y, Kobayashi K, Yoshiura K. Clinical usefulness of the mDIXON Quant the method for estimation of the salivary gland fat fraction: comparison with MR spectroscopy. Br J Radiol 2017;90:20160704. [Crossref] [PubMed]
- Kukuk GM, Hittatiya K, Sprinkart AM, Eggers H, Gieseke J, Block W, Moeller P, Willinek WA, Spengler U, Trebicka J, Fischer HP, Schild HH, Träber F. Comparison between modified Dixon MRI techniques, MR spectroscopic relaxometry, and different histologic quantification methods in the assessment of hepatic steatosis. Eur Radiol 2015;25:2869-79. [Crossref] [PubMed]
- Baum T, Lorenz C, Buerger C, Freitag F, Dieckmeyer M, Eggers H, Zimmer C, Karampinos DC, Kirschke JS. Automated assessment of paraspinal muscle fat composition based on the segmentation of chemical shift encoding-based water/fat-separated images. Eur Radiol Exp 2018;2:32. [Crossref] [PubMed]
- Modesto AE, Stuart CE, Cho J, Ko J, Singh RG, Petrov MS. Psoas muscle size as a magnetic resonance imaging biomarker of progression of pancreatitis. Eur Radiol 2020;30:2902-11. [Crossref] [PubMed]
- Kasukawa Y, Hongo M, Ebina T, Chiba T, Kudo D, Kimura R, Shimada Y, Miyakoshi N. Quantitative Evaluation of Fat Composition in Lumbar Vertebral Body and Paraspinal Muscle by Proton Density Fat Fraction with MRI. Open Journal of Orthopedics 2022;12:85-96.
- Shahidi B, Parra CL, Berry DB, Hubbard JC, Gombatto S, Zlomislic V, Allen RT, Hughes-Austin J, Garfin S, Ward SR. Contribution of Lumbar Spine Pathology and Age to Paraspinal Muscle Size and Fatty Infiltration. Spine (Phila Pa 1976) 2017;42:616-23. [Crossref] [PubMed]
- Fortin M, Videman T, Gibbons LE, Battié MC. Paraspinal muscle morphology and composition: a 15-yr longitudinal magnetic resonance imaging study. Med Sci Sports Exerc 2014;46:893-901. [Crossref] [PubMed]
- Burian E, Inhuber S, Schlaeger S, Dieckmeyer M, Klupp E, Franz D, Weidlich D, Sollmann N, Löffler M, Schwirtz A, Rummeny EJ, Zimmer C, Kirschke JS, Karampinos DC, Baum T. Association of thigh and paraspinal muscle composition in young adults using chemical shift encoding-based water-fat MRI. Quant Imaging Med Surg 2020;10:128-36. [Crossref] [PubMed]
- Vlažná D, Krkoška P, Kuhn M, Dosbaba F, Batalik L, Vlčková E, Voháňka S, Adamová B. Assessment of Lumbar Extensor Muscles in the Context of Trunk Function, a Pilot Study in Healthy Individuals. Appl Sci 2021;11:9518.
- Mannion AF. Fibre type characteristics and function of the human paraspinal muscles: normal values and changes in association with low back pain. J Electromyogr Kinesiol 1999;9:363-77. [Crossref] [PubMed]
- Arbanas J, Klasan GS, Nikolic M, Jerkovic R, Miljanovic I, Malnar D. Fibre type composition of the human psoas major muscle with regard to the level of its origin. J Anat 2009;215:636-41. [Crossref] [PubMed]
- Steele J, Fisher J, Perrin C, Conway R, Bruce-Low S, Smith D. Does change in isolated lumbar extensor muscle function correlate with good clinical outcome? A secondary analysis of data on change in isolated lumbar extension strength, pain, and disability in chronic low back pain. Disabil Rehabil 2019;41:1287-95. [Crossref] [PubMed]
- Krkoska P, Vlazna D, Sladeckova M, Minarikova J, Barusova T, Batalik L, Dosbaba F, Vohanka S, Adamova B. Adherence and Effect of Home-Based Rehabilitation with Telemonitoring Support in Patients with Chronic Non-Specific Low Back Pain: A Pilot Study. Int J Environ Res Public Health 2023;20:1504. [Crossref] [PubMed]
- Csapo R, Malis V, Sinha U, Du J, Sinha S. Age-associated differences in triceps surae muscle composition and strength - an MRI-based cross-sectional comparison of contractile, adipose and connective tissue. BMC Musculoskelet Disord 2014;15:209. [Crossref] [PubMed]
- Kader DF, Wardlaw D, Smith FW. Correlation between the MRI changes in the lumbar multifidus muscles and leg pain. Clin Radiol 2000;55:145-9. [Crossref] [PubMed]
- Parkkola R, Rytökoski U, Kormano M. Magnetic resonance imaging of the discs and trunk muscles in patients with chronic low back pain and healthy control subjects. Spine (Phila Pa 1976) 1993;18:830-6. [Crossref] [PubMed]
- Hu ZJ, He J, Zhao FD, Fang XQ, Zhou LN, Fan SW. An assessment of the intra- and inter-reliability of the lumbar paraspinal muscle parameters using CT scan and magnetic resonance imaging. Spine (Phila Pa 1976) 2011;36:E868-74. [Crossref] [PubMed]
- Ranson CA, Burnett AF, Kerslake R, Batt ME, O'Sullivan PB. An investigation into the use of MR imaging to determine the functional cross sectional area of lumbar paraspinal muscles. Eur Spine J 2006;15:764-73. [Crossref] [PubMed]
- Kim TN, Choi KM. Sarcopenia: definition, epidemiology, and pathophysiology. J Bone Metab 2013;20:1-10. [Crossref] [PubMed]
- Wiedmer P, Jung T, Castro JP, Pomatto LCD, Sun PY, Davies KJA, Grune T. Sarcopenia - Molecular mechanisms and open questions. Ageing Res Rev 2021;65:101200. [Crossref] [PubMed]
- Volpi E, Nazemi R, Fujita S. Muscle tissue changes with aging. Curr Opin Clin Nutr Metab Care 2004;7:405-10. [Crossref] [PubMed]