Using amide proton transfer-weighted magnetic resonance imaging (MRI) in peritumor tissue to predict parametrial infiltration of cervical cancer: a case-control study
Introduction
As one of the most common gynecological malignancies, cervical cancer (CC) has the fourth highest incidence rate and mortality among female cancers (1). The prognosis and recurrence factors of CC include tumor size, staging, pathological subtypes, grading, parametrial infiltration (PMI), and lymph node metastasis. Of these PMI is considered a decisive factor in the treatment of patients with CC (2). Patients with PMI usually undergo chemotherapy and radiotherapy first, otherwise they can choose surgical treatment. If PMI is found after surgery, radiotherapy and chemotherapy are still necessary, which are associated with certain side effects (3). Therefore, an accurate assessment of the preoperative state of the patient’s parauterine tissue is crucial, because it is not only related to the prognosis but also affects the clinical decision-making.
At present, the decision to perform surgery is still based on the Federation of Gynecology and Obstetrics (FIGO) staging, as early infiltration is usually difficult to detect. Magnetic resonance imaging (MRI) has higher accuracy in detecting PMI than clinical physical examination (4). T2 weighted image (T2WI), as a commonly used MRI sequence, can reflect the anatomical structure information of the corresponding tissues. Based on diffusion-weighted imaging (DWI), the obtained apparent diffusion coefficient (ADC) sequence, which describes the speed of diffusion of water molecule in tissues, has been widely studied in the differentiation of benign and malignant cervical lesions (5), differentiation of CC (6), and prognostic efficacy (7). Although some studies have supported the role of MRI in the diagnosis of PMI (8,9), a significant limitation of MRI evaluation of PMI is that some tumors can cause interstitial edema through tumor compression or inflammation, which may mask the true tumor boundary, thereby overestimating MRI’s evaluation of PMI accuracy and increasing the false positive rate (10).
Amide proton transfer-weighted (APTw) imaging is a type of non-invasive novel molecular technology, and is based on the principle of exchange saturation transfer (11). It can be used to detect the content of endogenous free proteins and peptides in tissues, reflecting cellular metabolism and pathophysiological information (12). APTw is mainly used in the central nervous system (13,14) and has gradually been applied in the diagnosis and treatment of CC in recent years (15). In 2019, Li et al. reported that APTw imaging may be a potential predictive technique for the histologic grading of squamous cell carcinoma of the cervix (SCCC) and can provide helpful information to assist in SCCC diagnosis (16). Recently, a published APTw study on 70 SCCC patients showed that APTw and dynamic contrast-enhanced magnetic resonance imaging (DCE-MRI) quantitative parameters can help preoperatively predict the occurrence of deep stromal invasion (DSI) and/or lymphovascular space invasion (LVSI) (17). Furthermore, relatively fewer studies have focused on the quantitative values and microenvironment changes of peritumor tissues, to our knowledge, thus far, APTw imaging has not been applied to measure APTw values in peritumor tissue to predict PMI in patients with CC.
Therefore, this study aimed to investigate the potential of APTw in predicting PMI of CC, and to further evaluate whether the APTw parameters in peritumor tissue can add diagnostic value to DWI-MRI in CC. We present this article in accordance with the STROBE reporting checklist (available at https://qims.amegroups.com/article/view/10.21037/qims-24-412/rc).
Methods
Patients
The study was conducted in accordance with the Declaration of Helsinki (as revised in 2013). The study was approved by the Institutional Ethics Committee of the Second Hospital of Jilin University (No. 2022-230) and individual consent for this retrospective analysis was waived. The inclusion criteria were as follows: (I) a tumor size >1 cm in diameter; (II) had not received treatments such as radiotherapy or chemotherapy; (III) pelvic MRI scan was performed within 2 weeks before surgery. The exclusion criteria were (I) incomplete pathologic information; (II) inadequate image quality for analysis. Initially, 131 patients diagnosed with CC through histopathological at our hospital from March 2021 to December 2022 were included. All patients underwent MRI (including APTw MRI and DWI). Eventually, 81 of these patients (negative PMI on clinical physical examination and radical hysterectomy with postoperative pathological confirmation of CC) were included in the study. These 81 patients were divided into two groups: PMI group (n=22, mean age =51.27±11.15 years, age range =31–66 years) and non-PMI group (n=59, mean age =48.02±10.26 years, age range =19–68 years).
Image acquisition
All patients were examined using a 3.0T MRI system (Ingenia 3.0 T CX; Philips Healthcare, Best, the Netherlands). A combined abdominopelvic phased array coil was used. A conventional MRI plain + enhancement sequence was performed. Patients were placed in the supine position with a moderately filled bladder. The scan range was from the patient’s umbilicus to the level of the pubic symphysis. If the tumor was large and involved too extensively, the examination range was appropriately expanded. Routine MRI scan sequences included axial T1WI/T2WI sequences, sagittal T2WI sequences, axial fat-suppressed T2WI sequences, DCE-MRI sequences, and DWI. The APT value was calculated as the percentage of magnetization transfer asymmetry (MTRasym) at the frequency offset of +3.5 ppm as:
In which S-Δω and SΔω represent signals at frequency offset -Δω and Δω (Δω = +3.5 ppm), respectively; S0 indicates the signal without RF saturation (18). After scanning was completed, APTw images were automatically generated on the console (19). ADC maps were generated from the DWI images on the scanner console. The detailed information of MRI imaging and APTw imaging parameters are shown in Table 1.
Table 1
Parameter | Axial T1WI | Axial T2WI | Sag T2WI | DWI | DCE-MRI | APTw |
---|---|---|---|---|---|---|
TR (ms) | 500 | 2,500 | 3,500 | 4,000 | 500 | 90 |
TE (ms) | 13.13 | 110 | 110 | 56.3 | 1.31 | – |
FOV (cm) | 30 | 40 | 30 | 38 | 36 | 8 |
Matrix | 332×289 | 400×400 | 300×300 | 128×126 | 300×300 | 160×229 |
Layer thickness (mm) | 4 | 4 | 4 | 5 | 1.2 | 2 |
Layer spacing (mm) | 1 | 1 | 1 | 2 | 3 | 1 |
Scanning time (s) | 47 | 92 | 77 | 60 | 270 | 190 |
T1WI, T1 weighted image; T2WI, T2 weighted image; DWI, diffusion weighted imaging; DCE-MRI, dynamic contrast-enhanced magnetic resonance imaging; APTw, amide proton transfer-weighted; TR, repetition time; TE, echo time; FOV, field of view.
Image processing
Patients’ T2WI and APTw sequences were uploaded to the Philips post-processing workstation. The images were independently reviewed by two radiologists (8 and 12 years of experience in gynecological MRI) without knowledge of the patient’s clinical and pathologic information to map the regions of interest (ROI). According to the previous method described by Mori et al. (20), three circular ROIs (mean area, 20 mm2) were then drawn along the tumor margin where the APTw values visually appeared to be most increased on the peritumoral zone for measuring the APTw values of the individual ROIs, and the average values were taken. For intratumoral measurements, ROIs were placed inside the tumor that contained the largest tumor area, while blood vessels, regions of necrosis, and tumor stalk were carefully avoided by referring to T2-weighted images. APTw-t, the average APTw values of tumor; APTw-p, the average APTw values in peritumor tissues; ADC, the average ADC values of tumor.
In addition, two radiologists blindly reviewed the morphological and signal characteristics of T2WI sequence images of 81 patients without knowing the pathological results, in case of disagreement, a consensus was reached through consultation and discussion, and the diagnostic efficiency was calculated.
Statistical analysis
Statistical analysis was performed using standard statistical software SPSS 25.0 (IBM Corporation, Armonk, NY, USA), and intra-class correlation coefficients (ICC) were used to analyze the consistency of parameter values obtained from two observers using a double-blind method with multiple measurements. The ICC <0.40, 0.40≤ ICC <0.75 and ICC ≥0.75 respectively indicated as poor, medium, and good consistency. The Kolmogorov-Smirnov test was used to assess whether the variables were normally distributed. Variables that exhibited a normal distribution were represented as mean ± standard deviation. Otherwise, it was represented as the median (first quartile and third quartile). Independent samples t-test was used for normally distributed data, Mann-Whitney U test was used for non-normally distributed data, and Chi-squared test was used for categorical variables. The different parameter values of APTw and ADC for the PMI and non-PMI groups were included in the binary logistic regression analysis to establish a combined parameter model for predicting the PMI group, non-PMI group, the performance of the above parameters on the diagnostic aspects of the different groups was analyzed using the receiver operating characteristic curve, and the curve was calculated. The area under the curve (AUC) was calculated and the corresponding sensitivity and specificity of the parameters were determined according to the maximum Youden index. For all statistical tests, P<0.05 was considered to indicate statistically significant differences.
Results
Comparison of the patient characteristics and conventional MRI features between PMI group and non-PMI group
Table 2 presents the patient characteristics and conventional MRI features of the PMI group and non-PMI group. There was a significant statistical difference in maximum tumor diameter (MTD), squamous cell carcinoma antigen (SCC-Ag) level, platelet count (PLT), white blood cell count (WBC) and red blood cell (RBC) (P<0.05). However, no significant difference in age, menopausal status, Pathological type and alkaline phosphatase (ALP) was found (P>0.05). A typical case is shown in Figure 1.
Table 2
Characteristic | PMI group (n=22) | Non-PMI group (n=59) | P value |
---|---|---|---|
Age (years) | 51.27±11.15 | 48.02±10.26 | 0.218 |
Menopausal status | 0.451 | ||
No | 11 (50.0%) | 35 (59.3%) | |
Yes | 11 (50.0%) | 24 (40.7%) | |
Pathological type | 0.593 | ||
CSC | 20 (90.9%) | 49 (83.1%) | |
CA | 2 (9.10%) | 10 (16.9%) | |
MTD | 0.003 | ||
≤3 cm | 5 (22.7%) | 35 (59.3%) | |
>3 cm | 17(77.3%) | 24 (40.7%) | |
SCC-Ag level | 0.004 | ||
≤1.5 ng/mL | 6 (27.3%) | 37 (62.7%) | |
>1.5 ng/mL | 16 (72.7%) | 22 (37.3%) | |
ALP (U/L) | 78.50 (67.25, 101.50) | 78.33 (59.00, 101.00) | 0.276 |
PLT (109/L) | 296.16±82.34 | 262.99±54.38 | 0.038 |
WBC (109/L) | 7.60 (6.65, 9.15) | 5.40 (4.90, 6.80) | <0.001 |
RBC (109/L) | 4.44 (4.06, 4.61) | 4.62 (4.36, 4.88) | 0.012 |
Data are expressed as mean ± standard deviation, median (first quartile and third quartile) or number (percentage). PMI, parametrial infiltration; CSC, cervical squamous carcinoma; CA, cervical adenocarcinoma; MTD, maximum tumor diameter; SCC-Ag, squamous cell carcinoma antigen; ALP, alkaline phosphatase; PLT, platelet count; WBC, white blood cell count; RBC, red blood cell.
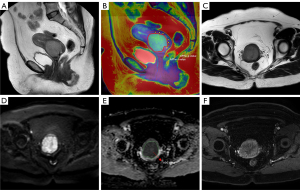
Data consistency between the two observers
The intra-class agreements between the two observers were good for the APTw-t, APTw-p and ADC values in both groups (all ICC ≥0.75, Table 3).
Table 3
Parameter | Group | Observer 1 | Observer 2 | ICC |
---|---|---|---|---|
APTw-t (%) | PMI group | 3.77±0.30 | 3.78±0.31 | 0.919 |
Non-PMI group | 3.42±0.29 | 3.44±0.29 | 0.918 | |
APTw-p (%) | PMI group | 2.62±0.19 | 2.74±0.17 | 0.911 |
Non-PMI group | 2.11±0.43 | 2.15±0.41 | 0.898 | |
ADC (×10−3 mm2/sec) | PMI group | 0.89±0.08 | 0.87±0.07 | 0.867 |
Non-PMI group | 1.00±0.14 | 0.99±0.13 | 0.947 |
Data are expressed as mean ± standard deviation. ICC, intra-class correlation coefficients; APTw-t, APTw values of tumor; PMI, parametrial infiltration; APTw-p, APTw values in peritumor tissues; APTw, amide proton transfer-weighted; ADC, apparent diffusion coefficient.
Comparison of parameters between the two groups
The APTw and ADC values for evaluating PMI group and non-PMI group are shown in Table 4 and Figure 2. The APTw-t and APTw-p values (%) of PMI group were higher than those of non-PMI group [3.71 (interquartile range, IQR, 3.60–3.98) and 2.75 (IQR, 2.68–2.77) vs. 3.33 (IQR, 3.24–3.60) and 1.98 (IQR, 1.82–2.36); P<0.001]. The ADC values of PMI group were lower than those of non-PMI group [0.88 (IQR, 0.83–0.94) ×10−3 vs. 0.95 (IQR, 0.88–1.04)×10−3 mm2/sec; P<0.001].
Table 4
Parameter | PMI group | Non-PMI group | P value |
---|---|---|---|
APTw-t (%) | 3.71 (3.60–3.98) | 3.33 (3.24–3.60) | <0.001 |
APTw-p (%) | 2.75 (2.68–2.77) | 1.98 (1.82–2.36) | <0.001 |
ADC (×10−3 mm2/sec) | 0.88 (0.83–0.94) | 0.95 (0.88–1.04) | <0.001 |
Data are expressed as median (first quartile–third quartile). PMI, parametrial infiltration; APTw-t, APTw values of tumor; APTw-p, APTw values in peritumor tissues; APTw, amide proton transfer-weighted; ADC, apparent diffusion coefficient.
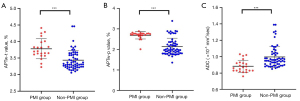
Diagnostic performance of T2WI sequence
Two radiologists diagnosed 23 cases with PMI and 58 cases without PMI through T2WI sequence. The difference between the pathological diagnosis and T2WI sequence diagnosis was statistically significant (P<0.001). The AUC value of T2WI sequence was 0.867, accuracy was 88.89%, sensitivity was 78.26%, and specificity was 93.10%, and there were 4 false negative cases and 5 false positive cases (Table 5).
Table 5
Pathological diagnosis results | T2WI diagnostic results | Total | |
---|---|---|---|
PMI group | Non-PMI group | ||
PMI group | 18 | 4 | 22 |
Non-PMI group | 5 | 54 | 59 |
Total | 23 | 58 | 81 |
T2WI, T2 weighted image; PMI, parametrial infiltration.
Diagnostic performance of each parameter and their combination for diagnostic efficacy
The results of receiver operating characteristic curve analysis are shown in Table 6 and Figure 3. The AUCs of APTw-t, APTw-p and ADC value for PMI diagnosis were 0.810, 0.831 and 0.806 respectively. The combined APTw-t + ADC and APTw-p + ADC values significantly improved the diagnostic performance (AUC =0.869, 0.918). The AUC value of APTw-p + ADC was optimal, with a sensitivity and specificity of 95.50% and 74.60% respectively.
Table 6
Parameter | AUC (95% CI) | Cut-off value* | Sensitivity | Specificity |
---|---|---|---|---|
APTw-t | 0.810 (0.711–0.910) | 3.55 | 86.40% | 69.50% |
APTw-p | 0.831 (0.738–0.923) | 2.44 | 95.50% | 81.40% |
ADC | 0.806 (0.690–0.921) | 0.92 | 77.30% | 69.50% |
APTw-t + ADC | 0.869 (0.778–0.960) | – | 81.80% | 79.70% |
APTw-p + ADC | 0.918 (0.858–0.977) | – | 95.50% | 74.60% |
*, the threshold corresponding to the maximum Youden index. This threshold can be used as the optional classification threshold. PMI, parametrial infiltration; AUC, area under the curve; CI, confidence interval; APTw-t, APTw values of tumor; APTw-p, APTw values in peritumor tissues; APTw, amide proton transfer-weighted; ADC, apparent diffusion coefficient.
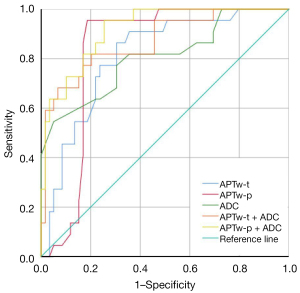
Discussion
MRI is the most sensitive imaging modality for the diagnosis of CC, which is significantly more accurate than both clinical and computed tomography (CT) examinations. New MRI techniques are constantly being attempted to improve the accuracy of diagnosing PMI. Our study evaluated the diagnostic potential of APTw values of tumor (APTw-t), APTw values in peritumor tissues (APTw-p) and ADC value at MRI for differentiating PMI. The results showed that PMI group exhibited higher APTw values than the non-PMI group. The ADC values of PMI group were lower than those of non-PMI group. The AUCs of APTw-t, APTw-p and ADC value for PMI diagnosis were 0.810, 0.831 and 0.806 respectively. In addition, the combination of APTw and DWI improved the diagnostic performance compared with individual analysis of either technique, among them, the AUC value (0.918) of APTw-p + ADC was optimal, with a sensitivity and specificity of 95.50% and 74.60% respectively. Our research results indicated that the combination of APTw value and ADC value could better grade diseases before surgery, which is consistent with previous research results on other tumors (21-23). Wang et al. investigated the use of APTw and ADC in estimating histologic grade of bladder cancer, indicating they may serve as potential noninvasive biomarkers in predicting histologic grade of bladder cancer (23). Moreover, a recent study also reported that APT and ADC have complementary effects on the sensitivity and specificity for identifying different grades of prostate cancer, a combination model of APTw and ADC could improve the diagnostic efficacy of prostate cancer (22). In addition, PMI group had lower ADC values, which is consistent with previous research findings on CC (5).
In this study, APTw-t values were higher in the PMI group than that in the non-PMI group for the following possible reasons. Tumors with higher staging exhibit more aggressive biological behavior, mainly including large tumor size, high nuclear grading, increased cell density, and high percentage of gland formation, all of which lead to an increase in intracellular proteins and lipids, which in turn leads to higher APTw signal intensity (24,25). In addition, as tumors become more malignant, the number of cytoplasmic ribosomes increase, leading to an exuberant metabolism and subsequent production of more proteins associated with tumor invasion and metastasis, resulting in higher APTw values.
Moreover, APTw-t values of tumors in both groups were significantly higher than APTw-p values. We speculate that α-enolase ENO1 (a multifunctional protein), transcription factors Pokemon and proteins show high expression in tumor tissues (26,27), hence, tumor tissues with higher protein content and APTw show higher signal intensity than peritumor tissues. Research has shown that vascular endothelial growth factor (VEGF) and microvessel density (MVD) are significantly higher in tumor tissues than in peritumor tissues (28), with increased tumor neovascularization and high concentrations of hemoglobin and albumin in the blood, which in turn leads to higher APTw signal intensity.
In addition, we found that the APTw-p in the PMI group were higher than those in the non-PMI group, and the diagnostic efficacy of peritumor tissues was also higher than that of the tumor itself, which may be due to the more significant changes in the microenvironment components of tumors with PMI. The tumor surrounding tissues mainly include fibroblasts, capillaries, lymphatic vessels, neural tissue, and lymphocytes. Fibroblasts are an important component of the tumor stromal microenvironment. When the tumor infiltrates, proliferates, and invades the surrounding tissues, the number of fibroblasts continues to increase, meanwhile, fibroblasts produce rich angiogenic promoting factors, such as vascular endothelial growth factor A (VEGFA) and platelet derived growth factor (PDGF), which further leads to different protein contents in the tumor microenvironment at different stages (29). Lymphatic vessels have been shown to be involved in tumor development. When tumor tissue infiltrates the lymphatic vessels of peritumor tissue, it leads to lymphatic vessel remodeling and expansion, and a large number of cancer cells enter them. Lymphangiogenic factors such as VEGF also induce lymphatic vessel expansion and weaken lymphatic connections, which further promotes lymphatic vessel invasion by tumor cells and a significant increase in protein content in peritumor tissue (30,31). So we speculate that in the peritumor tissue with PMI, a large number of lymphocytes are seen, and such infiltrated lymphocytes are rich in cytoplasm and have a high degree of tumor heterogeneity, which is of great significance in diagnosing early PMI.
The integrity of the low signal loop located outside the cervical parenchyma on T2WI plays an important role in determining PMI of CC. Currently, there are two difficulties in MRI diagnosis. On the one hand, it is false negatives caused by micro infiltration or discontinuous lesions in the peritumor tissue. Bleker et al. reported that among 120 patients with negative parauterine MRI examination, 5 cases were pathologically confirmed to have PMI after surgery (32). On the other hand, inflammation or peritumoral edema around cervical lesions can lead to false positives. Kong TW et al. found that in cases where the low signal ring around the cervix is invaded, 66.7% of cases have postoperative pathological confirmation of PMI (33). In our study, 4 false negative cases and 5 false positive cases were detected through T2WI diagnosis. However, after measuring the APTw-t, APTw-p and ADC value and comparing them with the cut-off value, it was found that 7 patients could avoid misdiagnosis or missed diagnosis, indicating that the values have guiding significance for clinical diagnosis and treatment.
There are some limitations to the present study. First, it is a single-center, retrospective study with a small data set, and most patients included in the study were early-stage patients, which may affect the accuracy of the research results to some extent. Second, we utilized the theory of microenvironment to explain the relatively high values of APTw in tumors and peritumor tissues with PMI, therefore, further molecular experimental research is still needed to validate our findings.
Conclusions
APTw is a promising imaging marker for preoperative clinical prediction of CC. This study found that the values of APTw in tumors and peritumor tissues has certain potential and value in the evaluation of PMI, especially in peritumor tissues, which can provide additional information to improve the results of diffusion-weighted MRI, thereby assisting further clinical decision-making and improving patient prognosis.
Acknowledgments
Funding: This work was supported by
Footnote
Reporting Checklist: The authors have completed the STROBE reporting checklist. Available at https://qims.amegroups.com/article/view/10.21037/qims-24-412/rc
Conflicts of Interest: All authors have completed the ICMJE uniform disclosure form (available at https://qims.amegroups.com/article/view/10.21037/qims-24-412/coif). The authors have no conflicts of interest to declare.
Ethical Statement: The authors are accountable for all aspects of the work in ensuring that questions related to the accuracy or integrity of any part of the work are appropriately investigated and resolved. The study was conducted in accordance with the Declaration of Helsinki (as revised in 2013). The study was approved by the Institutional Ethics Committee of the Second Hospital of Jilin University (No. 2022-230) and individual consent for this retrospective analysis was waived.
Open Access Statement: This is an Open Access article distributed in accordance with the Creative Commons Attribution-NonCommercial-NoDerivs 4.0 International License (CC BY-NC-ND 4.0), which permits the non-commercial replication and distribution of the article with the strict proviso that no changes or edits are made and the original work is properly cited (including links to both the formal publication through the relevant DOI and the license). See: https://creativecommons.org/licenses/by-nc-nd/4.0/.
References
- Sung H, Ferlay J, Siegel RL, Laversanne M, Soerjomataram I, Jemal A, Bray F. Global Cancer Statistics 2020: GLOBOCAN Estimates of Incidence and Mortality Worldwide for 36 Cancers in 185 Countries. CA Cancer J Clin 2021;71:209-49. [Crossref] [PubMed]
- Patel-Lippmann K, Robbins JB, Barroilhet L, Anderson B, Sadowski EA, Boyum J. MR Imaging of Cervical Cancer. Magn Reson Imaging Clin N Am 2017;25:635-49. [Crossref] [PubMed]
- Chung HH, Kang SB, Cho JY, Kim JW, Park NH, Song YS, Kim SH, Lee HP. Can preoperative MRI accurately evaluate nodal and parametrial invasion in early stage cervical cancer? Jpn J Clin Oncol 2007;37:370-5. [Crossref] [PubMed]
- Bourgioti C, Chatoupis K, Rodolakis A, Antoniou A, Tzavara C, Koutoulidis V, Moulopoulos LA. Incremental prognostic value of MRI in the staging of early cervical cancer: a prospective study and review of the literature. Clin Imaging 2016;40:72-8. [Crossref] [PubMed]
- Lin Y, Li H, Chen Z, Ni P, Zhong Q, Huang H, Sandrasegaran K. Correlation of histogram analysis of apparent diffusion coefficient with uterine cervical pathologic finding. AJR Am J Roentgenol 2015;204:1125-31. [Crossref] [PubMed]
- Zhang B, Guan Y, Huang S, Ge Y, Huang X, Cao Y, Jin J, He J. Whole-lesion Histogram Analysis of Apparent Diffusion Coefficient for Distinguishing Cervical Cancers with Different Differentiation. 2016 IEEE 10th International Conference on Nano/Molecular Medicine and Engineering (NANOMED), Macau, China, 2016:27-31.
- Chen J, Hua H, Pang J, Shi X, Bi W, Li Y, Xu W. The Value of Diffusion-Weighted Magnetic Resonance Imaging in Predicting the Efficacy of Radiation and Chemotherapy in Cervical Cancer. Open Life Sci 2018;13:305-11. [Crossref] [PubMed]
- Park BK, Kim TJ. Useful MRI Findings for Minimally Invasive Surgery for Early Cervical Cancer. Cancers (Basel) 2021;13:4078. [Crossref] [PubMed]
- Merz J, Bossart M, Bamberg F, Eisenblaetter M. Revised FIGO Staging for Cervical Cancer - A New Role for MRI. Rofo 2020;192:937-44. [Crossref] [PubMed]
- Sala E, Rockall AG, Freeman SJ, Mitchell DG, Reinhold C. The added role of MR imaging in treatment stratification of patients with gynecologic malignancies: what the radiologist needs to know. Radiology 2013;266:717-40. [Crossref] [PubMed]
- Wáng YXJ, Dou W, Shen Z, Zhang Y. An update on liver chemical exchange saturation transfer imaging with a focus on clinical translation. Quant Imaging Med Surg 2023;13:4057-76. [Crossref] [PubMed]
- Zhou J, Payen JF, Wilson DA, Traystman RJ, van Zijl PC. Using the amide proton signals of intracellular proteins and peptides to detect pH effects in MRI. Nat Med 2003;9:1085-90. [Crossref] [PubMed]
- Togao O, Yoshiura T, Keupp J, Hiwatashi A, Yamashita K, Kikuchi K, Suzuki Y, Suzuki SO, Iwaki T, Hata N, Mizoguchi M, Yoshimoto K, Sagiyama K, Takahashi M, Honda H. Amide proton transfer imaging of adult diffuse gliomas: correlation with histopathological grades. Neuro Oncol 2014;16:441-8. [Crossref] [PubMed]
- Park JE, Kim HS, Park KJ, Choi CG, Kim SJ. Histogram Analysis of Amide Proton Transfer Imaging to Identify Contrast-enhancing Low-Grade Brain Tumor That Mimics High-Grade Tumor: Increased Accuracy of MR Perfusion. Radiology 2015;277:151-61. [Crossref] [PubMed]
- Li S, Liu J, Zhang Z, Wang W, Lu H, Lin L, Zhang Y, Cheng J. Added-value of 3D amide proton transfer MRI in assessing prognostic factors of cervical cancer: a comparative study with multiple model diffusion-weighted imaging. Quant Imaging Med Surg 2023;13:8157-72. [Crossref] [PubMed]
- Li B, Sun H, Zhang S, Wang X, Guo Q. Amide proton transfer imaging to evaluate the grading of squamous cell carcinoma of the cervix: A comparative study using 18 F FDG PET. J Magn Reson Imaging 2019;50:261-8. [Crossref] [PubMed]
- Song Q, Tian S, Ma C, Meng X, Chen L, Wang N, Lin L, Wang J, Song Q, Liu A. Amide proton transfer weighted imaging combined with dynamic contrast-enhanced MRI in predicting lymphovascular space invasion and deep stromal invasion of IB1-IIA1 cervical cancer. Front Oncol 2022;12:916846. [Crossref] [PubMed]
- Zhou J, Heo HY, Knutsson L, van Zijl PCM, Jiang S. APT-weighted MRI: Techniques, current neuro applications, and challenging issues. J Magn Reson Imaging 2019;50:347-64. [Crossref] [PubMed]
- He YL, Li Y, Lin CY, Qi YF, Wang X, Zhou HL, Yang JJ, Xiang Y, Xue HD, Jin ZY. Three-dimensional turbo-spin-echo amide proton transfer-weighted mri for cervical cancer: A preliminary study. J Magn Reson Imaging 2019;50:1318-25. [Crossref] [PubMed]
- Mori N, Mugikura S, Takasawa C, Miyashita M, Shimauchi A, Ota H, Ishida T. kasajima A, Takase K, Kodama T, Takahashi S. Peritumoral apparent diffusion coefficients for prediction of lymphovascular invasion in clinically node-negative invasive breast cancer. Eur Radiol 2016;26:331-9. [Crossref] [PubMed]
- Huang Q, Wang Y, Meng X, Li J, Shen Y, Hu X, Feng C, Li Z, Kamel I. Amide Proton Transfer-Weighted Imaging Combined with ZOOMit Diffusion Kurtosis Imaging in Predicting Lymph Node Metastasis of Cervical Cancer. Bioengineering (Basel) 2023;10:331. [Crossref] [PubMed]
- Qin X, Mu R, Zheng W, Li X, Liu F, Zhuang Z, Yang P, Zhu X. Comparison and combination of amide proton transfer magnetic resonance imaging and the apparent diffusion coefficient in differentiating the grades of prostate cancer. Quant Imaging Med Surg 2023;13:812-24. [Crossref] [PubMed]
- Wang HJ, Cai Q, Huang YP, Li MQ, Wen ZH, Lin YY, Ouyang LY, Qian L, Guo Y. Amide Proton Transfer-weighted MRI in Predicting Histologic Grade of Bladder Cancer. Radiology 2022;305:E59. [Crossref] [PubMed]
- Jiang Y, You K, Qiu X, Bi Z, Mo H, Li L, Liu Y. Tumor volume predicts local recurrence in early rectal cancer treated with radical resection: A retrospective observational study of 270 patients. Int J Surg 2018;49:68-73. [Crossref] [PubMed]
- Chen W, Li L, Yan Z, Hu S, Feng J, Liu G, Liu B, Liu X. Three-dimension amide proton transfer MRI of rectal adenocarcinoma: correlation with pathologic prognostic factors and comparison with diffusion kurtosis imaging. Eur Radiol 2021;31:3286-96. [Crossref] [PubMed]
- Zhao ZH, Wang SF, Yu L, Wang J, Chang H, Yan WL, Fu K, Zhang J. Expression of transcription factor Pokemon in non-small cell lung cancer and its clinical significance. Chin Med J (Engl) 2008;121:445-9. [Crossref] [PubMed]
- Cui J, Wang Q, Wang HB, Wang B, Li L. Protein and DNA evidences of HCMV infection in primary breast cancer tissues and metastatic sentinel lymph nodes. Cancer Biomark 2018;21:769-80. [Crossref] [PubMed]
- Liu Y, Hong K, Weng W, Huang S, Zhou T. Association of vascular endothelial growth factor (VEGF) protein levels and gene polymorphism with the risk of chronic kidney disease. Libyan J Med 2023;18:2156675. [Crossref] [PubMed]
- Wu P, Tang Y, Gong Y, Tao X, Guan Y, Yang L, Xia X, Yi X, Xie C. Tracking tumor-specific mutations in plasma, saliva and para-carcinoma tissues from patients with head and neck squamous cell carcinomas. J Clin Oncol 2018;36:abstr 6056.
- Ma Q, Dieterich LC, Detmar M. Multiple roles of lymphatic vessels in tumor progression. Curr Opin Immunol 2018;53:7-12. [Crossref] [PubMed]
- Vaahtomeri K, Alitalo K. Lymphatic Vessels in Tumor Dissemination versus Immunotherapy. Cancer Res 2020;80:3463-5. [Crossref] [PubMed]
- Bleker SM, Bipat S, Spijkerboer AM, van der Velden J, Stoker J, Kenter GG. The negative predictive value of clinical examination with or without anesthesia versus magnetic resonance imaging for parametrial infiltration in cervical cancer stages IB1 to IIA. Int J Gynecol Cancer 2013;23:193-8. [Crossref] [PubMed]
- Kong TW, Kim J, Son JH, Kang SW, Paek J, Chun M, Chang SJ, Ryu HS. Preoperative nomogram for prediction of microscopic parametrial infiltration in patients with FIGO stage IB cervical cancer treated with radical hysterectomy. Gynecol Oncol 2016;142:109-14. [Crossref] [PubMed]