DLCNBC-SA: a model for assessing axillary lymph node metastasis status in early breast cancer patients
Introduction
Breast cancer is one of the most common malignant tumors in women worldwide, among whom its incidence is increasing annually (1,2). Early detection and effective intervention can reduce treatment costs and improve the survival rate and quality of life of patients. Currently, there are many detection techniques for breast cancer, such as X-ray (3), ultrasound (4), magnetic resonance imaging (MRI) (5), positron emission tomography/computed tomography (PET/CT) (6), and pathological examination (7).
Among these methods, pathological examination is considered the gold standard for the diagnosis of benign and malignant breast cancer (8,9). Pathological examination of breast cancer typically involves assessing the lymph node metastasis status, which plays a pivotal role in both prognosis and tumor dissemination (10). Particularly, the evaluation of axillary lymph nodes (ALN) metastasis holds significant importance in guiding treatment strategies for breast cancer patients (11). Therefore, preoperative prediction of lymph node metastasis can offer valuable insights for developing adjuvant therapies and surgical plans, facilitating informed decision-making prior to treatment initiation (12).
With the rapid advancement of digital imaging technology, whole slide image/imaging (WSI) has emerged as a widely adopted technique in digital pathology, gaining increasing popularity (13,14). WSI employs automated microscopes or optical magnification systems equipped with digital slide acquisition devices to scan and capture conventional glass-based histological sections at high resolution and large scale (15,16). This technology enables pathologists to perform comprehensive and detailed examinations of samples, facilitating more accurate determination of lymph node metastasis in breast cancer. However, manual analysis of WSI heavily relies on the pathologist’s expertise and diagnostic experience for precise diagnosis. Subjective interpretation by pathologists may impact diagnostic outcomes and prolonged observation of WSI might divert their attention. A study investigating single breast biopsy section interpretation based on WSI demonstrated a concordance rate of 75.3% between individual pathologists’ interpretations and reference diagnoses derived from expert consensus (17). Moreover, smaller hospitals lacking experienced pathologists may face time constraints that impede timely diagnosis and treatment for breast cancer patients. By employing artificial intelligence (AI) methods, the burden associated with WSI diagnosis can be alleviated while enhancing efficiency and accuracy (Acc) in diagnostics, ultimately providing more prompt medical services for patients.
AI has begun to assist doctors in making a diagnosis by helping them analyze medical images, thereby improving the diagnostic efficiency of doctors to a certain extent and reducing the treatment cost for patients (18,19). The main methods used in combination with AI for medical image analysis include detection (20), segmentation (21,22), registration (23), and noise reduction (24,25). These applications play a significant role in early diagnosis, lesion localization, individualized treatment, and comprehensive tumor evaluation. Qiao et al. (26) explored the application of digital pathology in medical diagnosis and treatment in their research on the combination of digital pathology and AI technology, emphasized the great potential of AI in data analysis, and showed that digital pathology provides prospects for the development of precision medicine and has the importance of transforming medical information into clinical knowledge. Amgad et al. (27) proposed the NuCLS model by modifying the mask regions with convolutional neural network (CNN) features (Mask R-CNN) architecture and successfully completed the specific task of nucleus detection on WSIs, which is highly important for computer-aided diagnosis of pathology and exploration of new quantitative morphological biomarkers. By establishing a high-precision deep learning platform composed of multiple CNNs, Li et al. (28) successfully achieved high Acc in the classification of human diffuse large B-cell lymphoma (DLBCL) via pathological images. In the future, the clinical application of these methods for reducing the workload of pathologists and for classifying DLBCL subtypes and other hematopoietic malignancies is expected to increase rapidly.
Digital pathology combined with AI is expected to transform high-quality and efficient pathological data into clinically actionable knowledge. By leveraging deep learning techniques, pathologists can employ computational analysis of pathological images to facilitate cancer classification (29-31). Moreover, deep learning combined with WSI technology has shown the potential to automatically extract features from images and effectively classify different subclasses of breast cancer samples, thereby promoting the progress of computer-aided diagnosis and prognosis in digital pathology (13,32,33). Deep learning models have the capability to extract intricate features from vast amounts of data, facilitating precise determination of lesion characteristics. Moreover, they are progressively emerging as a novel tool for pathologists, gradually replacing conventional optical microscopes (16). Deep learning is regarded as a promising asset in pathology (34); however, further research is imperative to fully harness its potential in this field.
The objective of this study is to employ deep learning techniques for solving the binary classification problem of ALN metastasis status in early breast cancer (EBC) patients (35), specifically classifying it as negative or positive. Considering the availability of numerous CNN models suitable for binary classification problems in deep learning research, such as EfficientNet (36), MobileNet (37), DenseNet121 (38), ResNet50 (39), and VGG16 (40), we have chosen these well-established CNNs as feature extractors. By comparing their performance during our investigation, our aim is to identify the most effective feature extractor. Additionally, we incorporate attention mechanisms to enhance the model’s ability to analyze features and integrate clinical data for determining ALN metastasis status. This approach of integrating deep learning models with WSI analysis and clinical data aims to improve Acc and efficiency in determining ALN metastasis status. Consequently, we propose an image classification model that combines mature CNNs as feature extractors along with attention mechanisms. The primary goal of this model is to assist medical professionals in lesion localization and determination of ALN status by analyzing WSI and clinical data from EBC patients.
The paper is organized as follows: The “Methods” section introduces the data sets, model structure, and comparative experiments and ablation experiments designed for this study. Subsequently, the “Results” section presents graphical representations of the conducted comparative experiments and ablation experiments. Finally, in the “Discussion” section, we analyze the obtained results, evaluate the computational efficiency of our model, discuss limitations of our research method proposed, and suggest potential research directions. We present this article in accordance with the TRIPOD-AI reporting checklist (available at https://qims.amegroups.com/article/view/10.21037/qims-24-257/rc).
Methods
Datasets
The EBC core-needle biopsy WSI dataset, provided by BCNB (41), was employed in this study to train and validate our proposed Deep Learning Convolutional Neural Network-Based Classification Model for the Prediction of ALN Metastasis. This dataset contains WSIs in JPG format and the corresponding clinical data of 1,058 EBC patients between May 2010 and August 2020. Clinical data encompasses the patient’s age, tumor size, tumor type, estrogen receptor (ER) status, progesterone receptor (PR) status, human epidermal growth factor receptor 2 (HER2) status and expression, as well as the number of lymph node metastases (LNM). We divided the WSIs and clinical data of these patients into a training cohort (840 patients) and an independent test cohort (218 patients), with N0 serving as the negative criterion and N(+) as the positive criterion. In this dataset, the mean age of the patients was 57.58 years, the mean tumor size was 2.234 cm, and the mean number of LNM was 1.2. Additional detailed characteristics, including tumor category and ER status, are shown in Figure 1. The study was conducted in accordance with the Declaration of Helsinki (as revised in 2013).
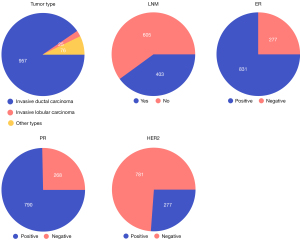
Model architecture and module building
The DLCNBC-SA model proposed in this paper is a deep learning-based image binary classification model, as illustrated in Figure 2. Prior to training the model, the feature extractor was pre-trained using the CIFAR10 dataset (42) to enhance the model’s generalization capability, expedite training speed, and optimize data utilization.
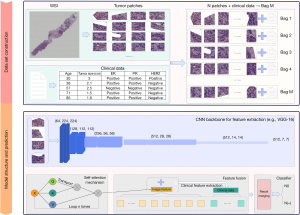
First, each WSI was divided into N patches of size 256×256 pixels, and M bags were built for them. The data processing step also included preprocessing each patient’s clinical data, which were entered into the same bag as that of the patient’s segmented WSIs. To improve the generalizability of the model and solve the overfitting problem, a data augmentation technique was applied to the images in the bag, and the shape of the image was 3, 224, 224.
Subsequently, the CNN model was used to extract features from the images inside the bag, and the sizes of the feature maps and were obtained. The feature map size, denoted as , should be transformed into the shape of by using a flattening operation. The obtained feature map is subsequently fed into a densely connected layer for further feature extraction, resulting in the output . To enhance the model’s understanding of the input information and effectively address the complexity and variability in the input data, the self-attention mechanism (43) was used to improve the model’s ability to represent and understand the image information and improve its performance and generalizability. The core computation of this attention mechanism is expressed as follows:
The specific values of , , and are obtained by directly assigning the input , where represents the second dimension of the input . This attention method retains the original features in the tensor for feature weighting and enhances the expression ability of the data. Finally, the tensor processed by the attention mechanism was combined with the clinical data of the corresponding patient to form a tensor , and the final result was obtained through the classifier.
Training
For this experiment, we opted to develop the model using PyCharm (JetBrains, Prague, Czechia) and set the default number of training rounds to 200. To enhance the model’s robustness, we employed a random rotation technique for dataset augmentation.
In terms of optimizer selection, we believe that the stochastic gradient descent (SGD) (44) optimizer possesses certain advantages. By solely considering the gradient of the current sample during parameter updates, it facilitates escaping local minimum points to some extent and enhances the likelihood of finding the global optimal solution. Moreover, SGD incurs relatively low computational overhead, which is particularly advantageous in resource-constrained scenarios. Considering these benefits alongside the optimizer employed in the DLCNBC-WS (45) model, we have opted for SGD as our chosen optimizer with a default learning rate set at 0.001. To control the impact of previous gradients on newly updated parameters, we set momentum to 0.3; additionally, weight decay was assigned a value of 0.001 to effectively regulate parameter decay rates using regularization techniques. The optimization formula is presented below:
Specifically, represents the model parameters after rounds of iteration, denotes the learning rate during the training process, signifies the weight decay parameter, indicates the momentum parameter, represents the momentum from the previous round , and symbolizes the gradient of loss function with respect to parameter . Regarding our choice of loss function, we employed cross-entropy (46), which can be calculated as follows:
The variable represents the true label of the sample, whereas denotes the predicted score assigned to the sample by the model. Additionally, and correspond to distinct weights allocated to different sample losses, with representing the total number of samples.
In this experiment, our specific computer environment is presented in Table 1. Unless otherwise specified, the optimizer, data augmentation method, and loss function employed in this study were all selected based on the aforementioned descriptions.
Table 1
Categorization | Reconfiguration |
---|---|
Computer system | Ubuntu 18.04.6 LTS |
CPU | Intel(R) Xeon(R) Silver 4214R CPU @ 2.40GHz |
GPU | NVIDIA Geforce RTX 3090 Founders Edition |
Frameworks for deep learning | Torch-1.12.1+cu116 |
CPU, central processing unit; GPU, graphics processing unit.
Comparative experiment
In addition to selecting the batch-normalized VGG16 (VGG16_BN) as the feature extractor for our CNN, we introduced and adapted an attention mechanism specifically tailored for our model in order to enhance performance and generalization by emphasizing key image components. The inclusion of attention mechanisms aims to improve model performance by directing focus towards important regions within the image.
To ensure accurate and comparable results, we maintained consistency with state-of-the-art (SOTA) models on the same dataset during experimental setup. By comparing our experimental outcomes with those of SOTA models, we can comprehensively evaluate the impact of our proposed attention mechanism on overall model performance. Additionally, we conducted experiments and comparisons using four baseline models incorporating Transform techniques, namely ViT (47), RegionViT (48), BiFormer (49), and GPViT (50), further validating the effectiveness of our proposed method.
Ablation studies
We conducted a series of ablation experiments to investigate the impact of different feature extractors, data augmentation techniques, and optimizers on the performance of the DLCNBC-SA model. Our objective was to identify the optimal combination that maximizes the performance of the DLCNBC-SA model by gradually replacing these factors. Initially, we replaced the feature extractor and compared model performance using different options such as VGG16_BN and ResNet50. Subsequently, various data augmentation techniques including random rotation and random cropping were employed to evaluate their influence on model performance. Finally, we examined different optimizers such as SGD and Adam (51) to determine an optimal choice for optimizing the DLCNBC-SA model’s performance. Through these ablation experiments, we aimed to gain a comprehensive understanding of each factor’s contribution towards enhancing the DLCNBC-SA model’s performance while providing valuable guidance and recommendations.
Results
To ensure the rigor of the study, the general metrics used to evaluate the performance of classifiers included accuracy (Acc), sensitivity (Sens), specificity (Spec), positive predictive value (PPV), negative predictive value (NPV), F1 score, and area under the curve (AUC).
Comparative analysis of models in experimental studies
Table 2 presents comprehensive information on the proposed model, along with 4 models incorporating the Transform mechanism and the SOTA model using the same dataset. The experimental results clearly demonstrate that our proposed model outperforms other models in terms of AUC, Spec, and PPV. However, for Acc, Sens, NPV, and F1-Score metrics, the SOTA model exhibits superior performance on this dataset. Furthermore, Figure 3 showcases the confusion matrices employed in this experiment for each model; these matrices are crucial tools for evaluating classification model performance. The inclusion of confusion matrices further emphasizes how well each model predicts different categories and provides a solid foundation for detailed analysis of their performance.
Table 2
Method | AUC | Acc | Sens | Spec | PPV | NPV | F1-Score |
---|---|---|---|---|---|---|---|
ViT | 0.829 | 0.739 | 0.786 | 0.709 | 0.629 | 0.841 | 0.698 |
RegionViT | 0.841 | 0.757 | 0.643 | 0.828 | 0.701 | 0.787 | 0.671 |
BiFormer | 0.621 | 0.633 | 0.25 | 0.873 | 0.553 | 0.65 | 0.344 |
GPViT | 0.839 | 0.743 | 0.548 | 0.866 | 0.719 | 0.753 | 0.622 |
DLCNBC-WS | 0.862 | 0.803 | 0.833 | 0.784 | 0.707 | 0.882 | 0.765 |
Proposed | 0.882 | 0.780 | 0.560 | 0.918 | 0.810 | 0.769 | 0.662 |
DLCNBC-SA, a deep learning-based network specifically tailored for core needle biopsy and clinical data feature extraction, which integrates a self-attention mechanism; ALN, axillary lymph nodes; AUC, area under the curve; Acc, accuracy; Sens, sensitivity; Spec, specificity; PPV, positive predictive value; NPV, negative predictive value.
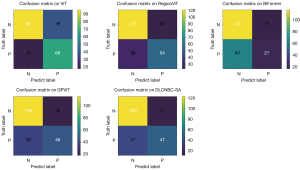
Experiment on ablation of feature extractor
We assessed the performance of 10 distinct CNN models enlisted in Table 3 as feature extractors and summarized their weight training experimental outcomes on the same evaluation metrics for the test set, as presented in Table 3. The corresponding AUC bar chart is depicted in Figure 4. The findings demonstrated that utilizing VGG16_BN as a feature extractor yielded superior performance, achieving an AUC value of 0.882. However, apart from Acc and PPV, this model did not exhibit significant advantages across other indicators and necessitates further refinement.
Table 3
Backbone | AUC | Acc | Sens | Spec | PPV | NPV | F1-Score |
---|---|---|---|---|---|---|---|
EfficientNet-B0 | 0.783 | 0.702 | 0.345 | 0.925 | 0.744 | 0.693 | 0.472 |
EfficientNet-B3 | 0.798 | 0.684 | 0.333 | 0.903 | 0.683 | 0.684 | 0.448 |
EfficientNet-B5 | 0.786 | 0.734 | 0.571 | 0.836 | 0.686 | 0.757 | 0.623 |
MobileNetV3-Large | 0.544 | 0.587 | 0.191 | 0.836 | 0.421 | 0.622 | 0.262 |
DenseNet121 | 0.793 | 0.734 | 0.548 | 0.851 | 0.697 | 0.750 | 0.613 |
ResNet50 | 0.783 | 0.688 | 0.452 | 0.836 | 0.633 | 0.709 | 0.528 |
VGG-11 BN | 0.811 | 0.771 | 0.679 | 0.828 | 0.713 | 0.804 | 0.695 |
VGG-13 BN | 0.867 | 0.757 | 0.607 | 0.851 | 0.718 | 0.776 | 0.658 |
VGG-16 BN | 0.882 | 0.780 | 0.560 | 0.918 | 0.810 | 0.769 | 0.662 |
VGG-19 BN | 0.869 | 0.748 | 0.893 | 0.657 | 0.620 | 0.907 | 0.732 |
ALN, axillary lymph nodes; DLCNBC-SA, a deep learning-based network specifically tailored for core needle biopsy and clinical data feature extraction, which integrates a self-attention mechanism; AUC, area under the curve; Acc, accuracy; Sens, sensitivity; Spec, specificity; PPV, positive predictive value; NPV, negative predictive value; VGG, visual geometry group; BN, batch normalization.
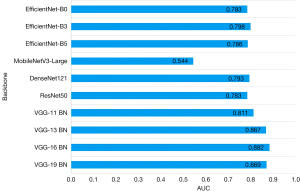
Data augmentation techniques for thermal melting experiments
The primary objective of employing data augmentation techniques is to enhance the diversity of training data by transforming and expanding it, thereby improving the model’s generalization capability and mitigating the risk of overfitting. Prior to model training, we applied 7 distinct data augmentation strategies as listed in Table 4 to augment the dataset and subsequently evaluated their corresponding AUCs using the assessment metrics summarized in Table 4. As depicted in Figure 5, random rotation exhibited superior performance with respect to AUC (0.882), Spec (0.918), and precision (0.810). Conversely, random affine transformation demonstrated optimal Acc (0.789), Sens (0.738), NPV (0.833), and F1 score (0.730). Consequently, for training our DLCNBC-SA model, random rotation and random affine transformation are deemed more suitable compared to the remaining 5 data augmentation strategies.
Table 4
Augmentation | AUC | Acc | Sens | Spec | PPV | NPV | F1-Score |
---|---|---|---|---|---|---|---|
No | 0.835 | 0.748 | 0.762 | 0.739 | 0.647 | 0.832 | 0.670 |
RandomRotation | 0.882 | 0.780 | 0.560 | 0.918 | 0.810 | 0.769 | 0.662 |
RandomAffine | 0.877 | 0.789 | 0.738 | 0.820 | 0.720 | 0.833 | 0.730 |
ColorJitter | 0.840 | 0.762 | 0.643 | 0.836 | 0.711 | 0.789 | 0.675 |
RandomVerticalFlip | 0.862 | 0.775 | 0.737 | 0.799 | 0.697 | 0.830 | 0.717 |
RandomHorizontalFlip | 0.844 | 0.757 | 0.655 | 0.821 | 0.696 | 0.791 | 0.675 |
All | 0.819 | 0.729 | 0.595 | 0.813 | 0.667 | 0.762 | 0.629 |
DLCNBC-SA, a deep learning-based network specifically tailored for core needle biopsy and clinical data feature extraction, which integrates a self-attention mechanism; ALN, axillary lymph nodes; AUC, area under the curve; Acc, accuracy; Sens, sensitivity; Spec, specificity; PPV, positive predictive value; NPV, negative predictive value.
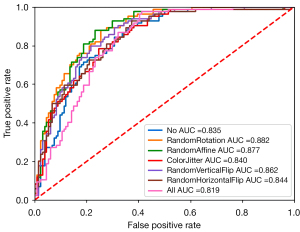
Revising the experiment on optimizer ablation
The performance of the model is influenced by different optimizers due to their distinct parameter update strategies. In the training process of the DLCNBC-SA model, we sequentially employed 4 different optimizers (listed in Table 5) to optimize its parameters and plotted the corresponding AUC results in Figure 6. It is evident from the table that the AdaDelta (53) optimizer with adaptive learning rate consistently outperforms the other 3 optimizers across almost all indicators, except for a slightly lower Spec value than its maximum of 0.008. Moreover, other indicators exhibit excellent performance.
Table 5
Optimizer | AUC | Acc | Sens | Spec | PPV | NPV | F1-Score |
---|---|---|---|---|---|---|---|
Adam | 0.541 | 0.587 | 0.286 | 0.776 | 0.444 | 0.634 | 0.348 |
AdaDelta | 0.892 | 0.817 | 0.667 | 0.910 | 0.824 | 0.813 | 0.737 |
RMSProp (52) | 0.573 | 0.569 | 0.298 | 0.739 | 0.417 | 0.627 | 0.347 |
SGD | 0.882 | 0.780 | 0.560 | 0.918 | 0.810 | 0.769 | 0.662 |
DLCNBC-SA, a deep learning-based network specifically tailored for core needle biopsy and clinical data feature extraction, which integrates a self-attention mechanism; ALN, axillary lymph nodes; AUC, area under the curve; Acc, accuracy; Sens, sensitivity; Spec, specificity; PPV, positive predictive value; NPV, negative predictive value; Adam, adaptive moment estimation; AdaDelta, an adaptive learning rate optimizer; RMSProp, root mean square pro; SGD, stochastic gradient descent.
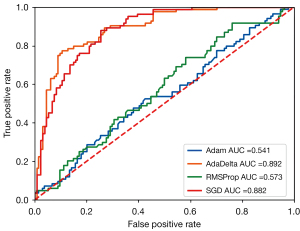
Discussion
The objective of this study was to analyze the prognostic significance of ALN metastasis status in the WSIs of EBC patients. The proposed DLCNC-SA model comprises the VGG16_BN, which exhibits exceptional performance in image classification due to its simple structure, and incorporates a self-attention mechanism that effectively enhances contextual information within the image. This model demonstrates outstanding performance in detecting ALN metastasis in EBC patient WSI and enables rapid inference of ALN status as positive or negative. In this study, to explore the influencing factors of the model performance, we conducted experiments by using different feature extractors, data augmentation operations, and optimizers (37,47).
Table 6 presents the floating point operations per second (FLOPs) and model complexity of all models in the comparative experiments. Upon comparison, it is observed that the proposed method exhibits a computational cost of 154.27 G, slightly surpassing RegionViT’s 127.89 G and BiFormer’s 93.70 G, yet still falling within the realm of relatively efficient computational costs. In terms of computation efficiency, the computational cost of the proposed method closely aligns with that of DLCNBC-WS, which stands at 154.07 G and represents the SOTA approach. Additionally, the proposed method boasts a parameter count of 41.37 M, fewer than both RegionViT (72.10 M) and BiFormer (56.40 M), while remaining within a comparably smaller range for parameters count. Although slightly higher than DLCNBC-WS’ parameter count of 21.17 M, it remains within an acceptable range.
Table 6
Indicator | ViT | RegionViT | BiFormer | GPViT | DLCNBC-WS | Proposed |
---|---|---|---|---|---|---|
FLOPs (G) | 168.63 | 127.89 | 93.70 | 312.42 | 154.07 | 154.27 |
Params (M) | 86.01 | 72.10 | 56.40 | 200.67 | 21.17 | 41.37 |
FLOPs, floating point operations per second; G, giga; M, mega.
Based on Figure 1, we identified a class imbalance issue in the BCNB dataset. To comprehensively consider the trade-off between true negative and false negative examples, we utilized weights in the loss function to mitigate the loss of positive and negative instances. However, upon examining Sens and Spec values from Table 2 to Table 5, it became evident that our model exhibited a bias towards classifying inputs as negative. Therefore, in this paper, we adopted AUC as the primary evaluation metric for assessing model performance due to its independence from class imbalance and ability to evaluate different models by considering both true positive rate and false positive rate. By analyzing data presented in Tables 2,5, it is apparent that our proposed model outperforms the SOTA model on the same dataset with a performance gap of 0.03. This demonstrates superior classification capability and improved overall performance of our proposed approach. In medical imaging applications where high prediction Acc is crucial, an AUC value of 0.892 may seem satisfactory; however, even minor errors can have significant consequences on patient health within the field of medical diagnosis. Henceforth, we emphasize the importance of achieving higher Acc levels while acknowledging that further validation and evaluation are necessary to ensure robustness, reliability, and effectiveness when deploying these models in real clinical environments. Given that decisions made within the medical domain often involve patients’ lives and well-being at stake, continuous efforts should be made to enhance model performance to reduce misdiagnosis rates along with missed diagnoses while minimizing clinical risks.
This study has several limitations. First, limited by the hardware, our network model cannot directly process WSIs; therefore, WSIs need to be divided and assembled into packages before they can be used for training and testing, which will cause image information to be lost. Second, our model was trained and tested on only the BCNB dataset and not on other publicly available datasets, which may lead to our model overfitting to the specific features and noise of the current dataset and ignoring the possible diversity and characteristics of other datasets. This can lead to poor performance on unseen data and potentially unstable performance in real-world applications.
Conclusions
In this paper, we used the DLCNBC-SA model to perform binary classification of ALN status [N0 vs. N(+)] for predicting preoperative ALN metastasis status in EBC patients. We found that using the self-attention mechanism can help the model simultaneously focus on the information of all elements in the WSI, capture the WSI global information, and distinguish the importance of different parts. In addition, we found that appropriate data augmentation operations on the data and selection of an appropriate optimizer can improve the classification performance of the model. In particular, when the random rotation method is used in preprocessing and AdaDelta is used as the optimizer in training, the AUC is better than that of the SOTA model by 0.03.
Acknowledgments
Funding: This work was supported by
Footnote
Reporting Checklist: The authors have completed the TRIPOD-AI reporting checklist. Available at https://qims.amegroups.com/article/view/10.21037/qims-24-257/rc
Conflicts of Interest: All authors have completed the ICMJE uniform disclosure form (available at https://qims.amegroups.com/article/view/10.21037/qims-24-257/coif). The authors have no conflicts of interest to declare.
Ethical Statement: The authors are accountable for all aspects of the work in ensuring that questions related to the accuracy or integrity of any part of the work are appropriately investigated and resolved. The study was conducted in accordance with the Declaration of Helsinki (as revised in 2013).
Open Access Statement: This is an Open Access article distributed in accordance with the Creative Commons Attribution-NonCommercial-NoDerivs 4.0 International License (CC BY-NC-ND 4.0), which permits the non-commercial replication and distribution of the article with the strict proviso that no changes or edits are made and the original work is properly cited (including links to both the formal publication through the relevant DOI and the license). See: https://creativecommons.org/licenses/by-nc-nd/4.0/.
References
- Ginsburg O, Yip CH, Brooks A, Cabanes A, Caleffi M, Dunstan Yataco JA, et al. Breast cancer early detection: A phased approach to implementation. Cancer 2020;126:2379-93. [Crossref] [PubMed]
- Sung H, Ferlay J, Siegel RL, Laversanne M, Soerjomataram I, Jemal A, Bray F. Global Cancer Statistics 2020: GLOBOCAN Estimates of Incidence and Mortality Worldwide for 36 Cancers in 185 Countries. CA Cancer J Clin 2021;71:209-49. [Crossref] [PubMed]
- Sakai T, Ozkurt E, DeSantis S, Wong SM, Rosenbaum L, Zheng H, Golshan M. National trends of synchronous bilateral breast cancer incidence in the United States. Breast Cancer Res Treat 2019;178:161-7. [Crossref] [PubMed]
- Yan M, Yao J, Zhang X, Xu D, Yang C. Machine learning-based model constructed from ultrasound radiomics and clinical features for predicting HER2 status in breast cancer patients with indeterminate (2+) immunohistochemical results. Cancer Med 2024;13:e6946. [Crossref] [PubMed]
- Skaane P, Bandos AI, Gullien R, Eben EB, Ekseth U, Haakenaasen U, Izadi M, Jebsen IN, Jahr G, Krager M, Niklason LT, Hofvind S, Gur D. Comparison of digital mammography alone and digital mammography plus tomosynthesis in a population-based screening program. Radiology 2013;267:47-56. [Crossref] [PubMed]
- Mori M, Fujioka T, Katsuta L, Tsuchiya J, Kubota K, Kasahara M, Oda G, Nakagawa T, Onishi I, Tateishi U. Diagnostic performance of time-of-flight PET/CT for evaluating nodal metastasis of the axilla in breast cancer. Nucl Med Commun 2019;40:958-64. [Crossref] [PubMed]
- Zeng H, Qiu S, Zhuang S, Wei X, Wu J, Zhang R, Chen K, Wu Z, Zhuang Z. Deep learning-based predictive model for pathological complete response to neoadjuvant chemotherapy in breast cancer from biopsy pathological images: a multicenter study. Front Physiol 2024;15:1279982. [Crossref] [PubMed]
- Zhang Z, Chen P, McGough M, Xing F, Wang C, Bui M, Xie Y, Sapkota M, Cui L, Dhillon J, Ahmad N, Khalil FK, Dickinson SI, Shi X, Liu F, Su H, Cai J, Yang L. Pathologist-level interpretable whole-slide cancer diagnosis with deep learning. Nat Mach Intell 2019;1:236-45. [Crossref]
- Zhu J, Liu M, Li X. Progress on deep learning in digital pathology of breast cancer: a narrative review. Gland Surg 2022;11:751-66. [Crossref] [PubMed]
- Chatterjee G, Pai T, Hardiman T, Avery-Kiejda K, Scott RJ, Spencer J, Pinder SE, Grigoriadis A. Molecular patterns of cancer colonisation in lymph nodes of breast cancer patients. Breast Cancer Res 2018;20:143. [Crossref] [PubMed]
- du Bois H, Heim TA, Lund AW. Tumor-draining lymph nodes: At the crossroads of metastasis and immunity. Sci Immunol 2021;6:eabg3551. [Crossref] [PubMed]
- Tafreshi NK, Kumar V, Morse DL, Gatenby RA. Molecular and functional imaging of breast cancer. Cancer Control 2010;17:143-55. [Crossref] [PubMed]
- Jahn SW, Plass M, Moinfar F. Digital Pathology: Advantages, Limitations and Emerging Perspectives. J Clin Med 2020;9:3697. [Crossref] [PubMed]
- Volynskaya Z, Evans AJ, Asa SL. Clinical Applications of Whole-slide Imaging in Anatomic Pathology. Adv Anat Pathol 2017;24:215-21. [Crossref] [PubMed]
- Kumar N, Gupta R, Gupta S. Whole Slide Imaging (WSI) in Pathology: Current Perspectives and Future Directions. J Digit Imaging 2020;33:1034-40. [Crossref] [PubMed]
- Veta M, Pluim JP, van Diest PJ, Viergever MA. Breast cancer histopathology image analysis: a review. IEEE Trans Biomed Eng 2014;61:1400-11. [Crossref] [PubMed]
- Elmore JG, Longton GM, Carney PA, Geller BM, Onega T, Tosteson AN, Nelson HD, Pepe MS, Allison KH, Schnitt SJ, O'Malley FP, Weaver DL. Diagnostic concordance among pathologists interpreting breast biopsy specimens. JAMA 2015;313:1122-32. [Crossref] [PubMed]
- Wang Y, Coudray N, Zhao Y, Li F, Hu C, Zhang YZ, Imoto S, Tsirigos A, Webb GI, Daly RJ, Song J. HEAL: an automated deep learning framework for cancer histopathology image analysis. Bioinformatics 2021;37:4291-5. [Crossref] [PubMed]
- Litjens G, Kooi T, Bejnordi BE, Setio AAA, Ciompi F, Ghafoorian M, van der Laak JAWM, van Ginneken B, Sánchez CI. A survey on deep learning in medical image analysis. Med Image Anal 2017;42:60-88. [Crossref] [PubMed]
- Adegun A, Viriri S. Deep learning techniques for skin lesion analysis and melanoma cancer detection: a survey of state-of-the-art. Artif Intell Rev 2021;54:811-41. [Crossref]
- Baldeon Calisto M, Lai-Yuen SK. AdaEn-Net: An ensemble of adaptive 2D-3D Fully Convolutional Networks for medical image segmentation. Neural Netw 2020;126:76-94. [Crossref] [PubMed]
- Milletari F, Ahmadi S, Kroll C, Plate A, Rozanski V, Maiostre J, Levin J, Dietrich O, Ertl-Wagner B, Boetzel K, Navab N. Hough-CNN: Deep learning for segmentation of deep brain regions in MRI and ultrasound. Comput Vis Image Underst 2017;164:92-102. [Crossref]
- El-Gamal FEA, Elmogy M, Atwan A. Current trends in medical image registration and fusion. Egypt Inform J 2016;17:99-124. [Crossref]
- Augustin AM, Kesavadas C, Sudeep PV. An Improved Deep Persistent Memory Network for Rician Noise Reduction in MR Images. Biomed Signal Process Control 2022;77:103736. [Crossref]
- Kidoh M, Shinoda K, Kitajima M, Isogawa K, Nambu M, Uetani H, Morita K, Nakaura T, Tateishi M, Yamashita Y, Yamashita Y. Deep Learning Based Noise Reduction for Brain MR Imaging: Tests on Phantoms and Healthy Volunteers. Magn Reson Med Sci 2020;19:195-206. [Crossref] [PubMed]
- Qiao Y, Zhao L, Luo C, Luo Y, Wu Y, Li S, Bu D, Zhao Y. Multi-modality artificial intelligence in digital pathology. Brief Bioinform 2022;23:bbac367. [Crossref] [PubMed]
- Amgad M, Atteya LA, Hussein H, Mohammed KH, Hafiz E, Elsebaie MAT, Mobadersany P, Manthey D, Gutman DA, Elfandy H, Cooper LAD. Explainable nucleus classification using Decision Tree Approximation of Learned Embeddings. Bioinformatics 2022;38:513-9. [Crossref] [PubMed]
- Li D, Bledsoe JR, Zeng Y, Liu W, Hu Y, Bi K, Liang A, Li S. A deep learning diagnostic platform for diffuse large B-cell lymphoma with high accuracy across multiple hospitals. Nat Commun 2020;11:6004. [Crossref] [PubMed]
- Oba K, Adachi M, Kobayashi T, Takaya E, Shimokawa D, Fukuda T, Takahashi K, Yagishita K, Ueda T, Tsunoda H. Deep learning model to predict Ki-67 expression of breast cancer using digital breast tomosynthesis. Breast Cancer 2024; Epub ahead of print. [Crossref] [PubMed]
- Campanella G, Hanna MG, Geneslaw L, Miraflor A, Werneck Krauss Silva V, Busam KJ, Brogi E, Reuter VE, Klimstra DS, Fuchs TJ. Clinical-grade computational pathology using weakly supervised deep learning on whole slide images. Nat Med 2019;25:1301-9. [Crossref] [PubMed]
- Coudray N, Ocampo PS, Sakellaropoulos T, Narula N, Snuderl M, Fenyö D, Moreira AL, Razavian N, Tsirigos A. Classification and mutation prediction from non-small cell lung cancer histopathology images using deep learning. Nat Med 2018;24:1559-67. [Crossref] [PubMed]
- Bhargava R, Madabhushi A. Emerging Themes in Image Informatics and Molecular Analysis for Digital Pathology. Annu Rev Biomed Eng 2016;18:387-412. [Crossref] [PubMed]
- Komura D, Ishikawa S. Machine Learning Methods for Histopathological Image Analysis. Comput Struct Biotechnol J 2018;16:34-42. [Crossref] [PubMed]
- Serag A, Ion-Margineanu A, Qureshi H, McMillan R, Saint Martin MJ, Diamond J, O'Reilly P, Hamilton P. Translational AI and Deep Learning in Diagnostic Pathology. Front Med (Lausanne) 2019;6:185. [Crossref] [PubMed]
- Yang CM. Diagnosis and management of early breast cancer. Journal of Dalian Medical University 2013;35:1-7.
- Dong S, Zhao Y, Chen Z. Remote Sensing Road Segmentation Based on Feature Extraction Optimization and Skeleton Detection Optimization. In: Wang L, Wu Y, Gong J. editors. Proceedings of the 7th China High Resolution Earth Observation Conference (CHREOC 2020). Lecture Notes in Electrical Engineering, Springer, Singapore, 2022;757:77-86.
.Howard AG Zhu M Chen B Kalenichenko D Wang W Weyand T Andreetto M Adam H MobileNets: Efficient convolutional neural networks for mobile vision applications. arXiv: 1704.04861.2017 . .Huang G Liu Z van der Maaten L Weinberger KQ Densely Connected Convolutional Networks. arXiv: 1608.06993.2016 .- Sharma AK, Nandal A, Dhaka A, Polat K, Alwadie R, Alenezi F, Alhudhaif A. HOG transformation based feature extraction framework in modified Resnet50 model for brain tumor detection. Biomed Signal Process Control 2023;84:104737. [Crossref]
- Etehadtavakol M, Etehadtavakol M, Ng EYK. Enhanced thyroid nodule segmentation through U-Net and VGG16 fusion with feature engineering: A comprehensive study. Comput Methods Programs Biomed 2024;251:108209. [Crossref] [PubMed]
- Xu F, Zhu C, Tang W, Wang Y, Zhang Y, Li J, Jiang H, Shi Z, Liu J, Jin M. Predicting Axillary Lymph Node Metastasis in Early Breast Cancer Using Deep Learning on Primary Tumor Biopsy Slides. Front Oncol 2021;11:759007. [Crossref] [PubMed]
- Krizhevsky A, Hinton G. Learning Multiple Layers of Features from Tiny Images. Technical Report, University of Toronto 2009.
- Sun Q, Cheng L, Meng A, Ge S, Chen J, Zhang L, Gong P. SADLN: Self-attention based deep learning network of integrating multi-omics data for cancer subtype recognition. Front Genet 2022;13:1032768. [Crossref] [PubMed]
- Zhou Y, Liang Y, Zhang H. Understanding generalization error of SGD in nonconvex optimization. Mach Learn 2022;111:345-75. [Crossref]
- Shkembi G, Muller JP, Li Z, Breininger K, Schuffler P, Kainz B. Whole Slide Multiple Instance Learning for Predicting Axillary Lymph Node Metastasis. In: Bhattarai B, Ali S, Rau A, Nguyen A, Namburete A, Caramalau R, Stoyanov D. Data Engineering in Medical Imaging. DEMI 2023. Lecture Notes in Computer Science, Springer, 2023;14314:11-20.
- Szegedy C, Vanhoucke V, Ioffe S, Shlens J, Wojna Z. Rethinking the Inception Architecture for Computer Vision. Proceedings of the IEEE Conference on Computer Vision and Pattern Recognition (CVPR), 2016:2818-26.
Dosovitskiy A Beyer L Kolesnikov A Weissenborn D Zhai X Unterthiner T Dehghani M Minderer M Heigold G Gelly S Uszkoreit J Houlsby N. An Image is Worth 16x16 Words: Transformers for Image Recognition at Scale. arXiv: 2010.11929.2021 .Chen C Panda R Fan Q. RegionViT: Regional-to-Local Attention for Vision Transformers. arXiv: 2106.02689.2022 .- Zhu L, Wang X, Ke Z, Zhang W, Lau R. BiFormer: Vision Transformer with Bi-Level Routing Attention. Proceedings of the IEEE/CVF Conference on Computer Vision and Pattern Recognition (CVPR), 2023:10323-33.
.Yang C Xu J De Mello S Crowley EJ Wang X GPViT: A High Resolution Non-Hierarchical Vision Transformer with Group Propagation. arXiv: 2212.06795.2022 . .Kingma DP Ba J Adam: A Method for Stochastic Optimization. arXiv: 1412.6980.2015 .- Xu D, Zhang S, Zhang H, Mandic DP. Convergence of the RMSProp deep learning method with penalty for nonconvex optimization. Neural Netw 2021;139:17-23. [Crossref] [PubMed]
- Sveleba S, Katerynchuk I, Kunyo I, Brygilevych V, Kotsun V, Sidelnyk Y. Dynamics of the Learning Process of a Multilayer Neural Network when using the AdaDelta Optimization Method. 2023 24th International Conference on Computational Problems of Electrical Engineering (CPEE), Grybów, Poland, 2023:1-4.