Artificial intelligence improves the diagnosis of human leukocyte antigen (HLA)-B27-negative axial spondyloarthritis based on multi-sequence magnetic resonance imaging and clinical features
Introduction
Axial spondyloarthritis (axSpA) is a chronic inflammatory disease that primarily affects the sacroiliac joint (SIJ) and spine, with a prevalence ranging from 0.3% to 1.4% (1,2). Human leukocyte antigen-B27 (HLA-B27) serves as a significant genetic marker strongly associated with axSpA. The presence of HLA-B27 provides important evidence for rheumatologists in diagnosis (3). However, previous studies have shown that a notable proportion, ranging from 42% to 57%, of suspected axSpA cases lack HLA-B27 (4). Patients with HLA-B27-negative axSpA often experience prolonged diagnostic delays, and the pathogenesis and symptoms of their condition remain inadequately understood (5). Delayed diagnosis hampers early intervention, leading to suboptimal treatment outcomes and unnecessary side effects (6). Persistent disease activity exacerbates symptoms, contributing to pain, irreversible structural damage, functional impairment, and heightened cardiovascular risks (7,8). Therefore, it is imperative to increase the focus on HLA-B27-negative axSpA to address the issues of delayed diagnosis, thereby improving treatment response and prognosis.
Detection of sacroiliitis on imaging modalities plays a pivotal role in diagnosing HLA-B27-negative axSpA (9). However, conventional radiographs, as defined by the 1984 modified New York (mNY) criteria (10), may not adequately capture early structural damage and inflammatory lesions, leading to delayed axSpA diagnosis (11). In contrast, magnetic resonance imaging (MRI) is highly sensitive in detecting early changes in SIJs, such as bone marrow edema, bone erosion, ankyloses, and so on (12). Hence, the Assessment of SpondyloArthritis International Society (ASAS) classification criteria [2009] incorporated a positive SIJ MRI scan, in addition to 11 clinical features, to classify axSpA (13). However, it is worth noting that positive MRI findings can also occur in other conditions, such as infection, degenerative arthritis, and osteitis condensans, potentially leading to misdiagnosis of axSpA (14,15). Moreover, HLA-B27-negative axSpA often exhibits atypical manifestations, including less involvement of the SIJs and less symmetric and marginal syndesmophytes (16). These variations pose challenges in distinguishing axSpA from its mimic diseases. In addition, the interpretations of SIJ MRI depend on radiologists’ personal experiences, which may introduce subjective bias, especially in basic-level hospitals (17). Given these challenges, new and effective methods are urgently required to assist clinicians in accurately interpreting SIJ MRI and improve the diagnosis of HLA-B27-negative axSpA.
Recently, artificial intelligence (AI) with deep learning (DL) has emerged as a great power for uncovering disease characteristics on MRI that may not be captured by the naked eye (18). DL techniques have been successfully applied to classify various types of arthritis and detect changes in SIJs indicative of axSpA (19-21). These studies have demonstrated the remarkable capability of AI in automatically analyzing SIJ MRI. However, the development of AI models for diagnosing HLA-B27-negative axSpA faces inevitable challenges: (I) small datasets: the MRI dataset for suspected HLA-B27-negative axSpA is small-size due to factors including the low incidence of the condition, delayed diagnosis, and challenges in data collection, which may not guarantee a robust population-level model training; (II) data differences: variations in image artifacts and unusual imaging appearances can reduce the generalization ability of AI models on unseen datasets. Further investigations are still needed to solve these issues.
In this study, we aimed to develop an AI model, named NegSpA-AI, which integrates SIJ MRI with clinical SpA features to provide more accurate diagnoses of axSpA in HLA-B27-negative patients, thereby improving clinical decision-making and patient outcomes. We present this article in accordance with the TRIPOD-AI reporting checklist (available at https://qims.amegroups.com/article/view/10.21037/qims-24-729/rc).
Methods
Study samples
The study was conducted in accordance with the Declaration of Helsinki (as revised in 2013). The study was approved by the Regional Institutional Review Board of the Third Affiliated Hospital of Southern Medical University and followed by Nanhai Hospital (No. 201501003). The requirement to obtain informed consent from patients in the retrospective cohorts was waived. Patients in the prospective cohort provided written informed consent that clearly stated that all the collected information would be used for publication by the investigator. Patients’ protected health information was removed from Digital Imaging and Communications in Medicine (DICOM) data in accordance with US (HIPAA), European (GDPR), or other relevant legal requirements (22). The data related to patients used in this study can be obtained from the corresponding author upon reasonable request.
We consecutively and retrospectively enrolled HLA-B27-negative patients with low back pain and axSpA from the Third Affiliated Hospital of Southern Medical University and Nanhai Hospital between January 2010 and August 2021. Following previous studies (23,24), HLA-B27-negative patients with low back pain but without axSpA (non-axSpA) were also recruited as a control group. Detailed disease subtypes of non-axSpA patients are described in Table S1. Figure 1 outlines the overall inclusion and exclusion criteria. The inclusion criteria for both groups were as follows: (I) HLA-B27 negative; (II) chronic low back pain (duration over 3 months); (III) available SIJ MRI and clinical data within 2 weeks after the initial examination and before any treatment. The exclusion criteria were as follows: (I) less than 2 clinical data; (II) incomplete MRI data; (III) poor MRI quality severely impeding observation of SIJs. The collected clinical data included age, sex, disease duration, and 11 SpA features as per the ASAS criteria (2009) (13). The clinical SpA features comprised inflammatory back pain, arthritis, heel enthesitis, uveitis, dactylitis, psoriasis, Crohn’s disease or ulcerative colitis, good response to nonsteroidal anti-inflammatory drugs (NSAIDs), family history of axSpA, HLA-B27 status, and C-reactive protein (CRP) levels. Clinical data were gathered from the electronic medical record system. Structural damage status was assessed by 2 musculoskeletal radiologists (Caolin Liu and Yangyang Shao) with 11 and 3 years of experience in SIJ MRI interpretation. The structural damage status was reported as positive if erosions, sclerosis, joint space narrowing/widening, or ankyloses were observed; it was otherwise reported as negative (3). Patients from center 1 recruited before April 2020 were allocated as the training set for model development using a 5-fold cross-validation, and patients recruited after April 2020 constituted an internal test set. Patients from center 2 were used as an independent external test set.
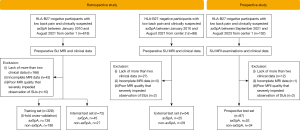
Next, we consecutively enrolled 87 HLA-B27-negative patients with low back pain and clinically suspected axSpA from center 1 between September 2021 and August 2023 to constitute a prospective test set for testing the developed model. These patients underwent SIJ MRI examinations including T1-weighted (T1W), T2-weighted (T2W), and fat suppression (FS) sequences, and their clinical data were collected. Patients were excluded from the prospective test set if they met any of the above exclusion criteria.
Diagnosis and labeling of patients
Each patient underwent a standardized diagnostic flowchart (see Figure S1) to be diagnosed and labeled as axSpA or non-axSpA. Firstly, 2 experienced musculoskeletal radiologists (Shisi Li and Rui Zhang), each with over 10 years of experience, independently interpreted the SIJ MRI following the criteria in the ASAS MRI working group (25) to report whether the MRI was suggestive of axSpA. The 2 radiologists discussed cases that had controversial reports to reach a consensus. Subsequently, 2 seasoned rheumatologists (X.H. and X.S.), each possessing over 10 years of experience, independently conducted a thorough analysis of all available information to provide a differential diagnosis between axSpA and non-axSpA. Rheumatologists analyzed the imaging findings on SIJ MRI from the radiologists, status of clinical SpA features, laboratory determinations, physician measurements, infection indicators, rheumatoid factors, pregnancy status, and the incidence of axSpA in the local area (26,27). Rheumatologists referred to both the ASAS classification criteria [2009] and the mNY criteria [1984] during the diagnostic process. In cases where there were controversial diagnoses or uncertainties, the 2 rheumatologists, along with another experienced rheumatologist (Y.H.) with over 15 years of experience, discussed them together to reach a consensus diagnosis. The final diagnoses were used as the ground-truth diagnoses to evaluate the performance of AI models and clinicians in the reader study. All clinicians participating in making the ground-truth diagnoses were isolated from the reader study.
Acquisition and annotation of MR images
MRI examinations of patients were conducted at 2 centers utilizing MRI scanners with field strengths of 1.5-T (Achieva; Philips Healthcare, Amsterdam, Netherlands) and 3.0-T (Ingenia; Philips Healthcare) employing both body coils and bed spine coil. The MRI protocol included T1W, T2W, and FS sequences. For T1W and T2W sequences, the axial plane images were primarily used if they were available, otherwise the coronal plane images were used. The FS sequences encompassed various sequences, such as spectral attenuated inversion recovery (SPAIR) T2W, proton density-weighted (PDW) SPAIR, and short tau inversion recovery (STIR), owing to differences in sequence acquisition protocols. Specifically, axial SPAIR sequences were preferred when they were available, otherwise the coronal SPAIR or STIR sequences were used. Further parameters of the MRI sequences are detailed in Table S2. All MRIs were downloaded from the Picture Archiving and Communications Systems (PACS) of participating centers and stored as DICOM format files at their original sizes and resolutions.
We introduce a 6-step annotation method for clinicians to annotate rectangular volumes of interest (RVOIs) containing bilateral SIJs on MRI (Appendix 1 and Figure S2). Firstly, 2 musculoskeletal radiologists (Xiaqing Chen and Rui Zhang) with 3 and 15 years of experience performed the 6-step annotation method to annotate RVOIs for each MRI. Both the radiologists were blinded to the actual diagnoses of the patients during the annotation. The intersection over union (IoU) between the annotated RVOIs was calculated to measure the inter-reader reliability. Then, RVOIs from the 2 radiologists were merged using the union function under the MATLAB (MathWorks, Natick, MA, USA) environment to generate a final RVOI for each MRI. The final RVOIs were used to develop the AI model.
Development and validation of NegSpA-AI
To avoid the potential impact of image differences on the model performance, we performed standard preprocessing procedures on all the MRIs, including intensity normalization, slice selection, and data augmentation. The detailed image preprocessing procedures are described in Appendix 2 and Figure S3. After preprocessing, each MRI was divided into 2 sub-images containing the left-side and right-side SIJs, which were used as input for the DL models. Then, 5 basic convolutional neural networks, including VGG16_bn (28) and various members of the ResNet family (29) (ResNet18, ResNet34, ResNet50, and Resnet101), were established and adapted into tri-input frameworks. These tri-input frameworks simultaneously take images from T1W, T2W, and FS sequences as inputs and predict the patients as axSpA or non-axSpA. Following validation and comparison, the tri-input framework exhibiting the best performance was chosen as the MRI-based DL model. Next, the clinical SpA features were integrated into the MRI-based DL model to develop NegSpA-AI (Figure 2). During model training, a data augmentation method named MixCut was proposed and a 2-stage transfer learning approach was employed. Moreover, visualization analysis using the gradient-weighted class activation mapping (Grad-CAM) method and clinical stratification analysis based on age, sex, disease duration, and structural damage were conducted for NegSpA-AI. Detailed development, validation, and analysis of NegSpA-AI are outlined in Appendix 2. All models were implemented using PyTorch (version 1.6) with Python (version 3.6) under a Linux system (Ubuntu, version 18.0; Canonical, London, UK) on NVIDIA GPUs (GeForce RTX 2080 Ti; NVIDIA, Santa Clara, CA, USA) with the CUDA platform (version 11.0). The source code can be found online at https://github.com/MedImgPro/ASMixCut. This code can be deployed in regular PACS systems and personal computers with GPU hardware to enable the accessibility of NegSpA-AI.
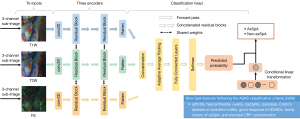
Reader study
On the internal and external test sets, 4 musculoskeletal radiologists (Rad1, Rad2, Rad3, and Rad4 with 4, 5, 16, and 31 years of experience, respectively) independently interpreted SIJ MRI following the ASAS MRI Working group guidelines (25) to report whether the MRI was suggestive of axSpA or not. On the prospective test set, Rad2, Rad3, and 2 rheumatologists (Rheu1 and Rheu2 with 4 and 12 years of experience, respectively) participated. Rad2 and Rad3 were tasked with interpreting MRIs similar to the process on the internal and external test sets. The rheumatologists were tasked with providing a differential diagnosis between axSpA and non-axSpA by thoroughly evaluating all available information, adhering to the ASAS classification criteria (2009) (13) and modified New York criteria (1984) (10). All clinicians participating in the reader study were blinded to the diagnostic outcomes and had no prior involvement in patient labelling, image assessment, or annotation. Clinicians with over 10 years of experience were categorized as seniors, whereas those with less than 5 years of experience were categorized as juniors. After a 3-month washout period since the first evaluation, all clinicians repeated their assessments with AI assistance following the same criteria and process in the first assessment. The AI assistance comprised prediction scores generated by NegSpA-AI and activation heatmaps on MR images from T1W, T2W, and FS sequences. The performance of NegSpA-AI was compared with that of both junior and senior clinicians to verify the clinical interpretation and diagnostic ability of AI. In addition, the performance of independent and AI-assisted clinicians was compared to investigate the contribution value of AI in clinical decision-making.
Statistical analysis
The normal distribution of all the clinical features was tested. We found that all the clinical features were not normally distributed. The statistical distribution of skewed variable, namely, disease duration, was present using median (Q1, Q3). Then, we applied the non-parametric Kruskal-Wallis H test for continuous variables and the non-parametric chi-square test for categorical variables to evaluate their statistical differences among different data sets. The inter-reader reliability of image annotations was evaluated using IoU, where IoU >0.5 indicated reliability. The distinguishing performance was evaluated using F1-score, area under the curve (AUC), accuracy, sensitivity, and specificity with 95% confidence intervals (CIs). The Delong test was performed to compare AUC differences between different MRI-based DL models. The Wilcoxon rank sum test was employed to compare model performance in the stratification analysis. The exact Fisher-Yates test was used to compare the performance of clinicians with and without AI assistance. Two-sided P values <0.05 statistically significant. All statistical analyses were implemented using the Python Scikit-learn library (Python version 3.6; Python Software Foundation, Wilmington, DE, USA).
Results
Patient characteristics
Overall, we analyzed 541 patients from the Third Affiliated Hospital of Southern Medical University and Nanhai Hospital, including 261 (48.2%) axSpA and 280 (51.8%) non-axSpA. The training set contained 328 patients (144 females; mean age: 36.9 years), among whom 138 (42.1%) were diagnosed with axSpA. The internal test set included 72 patients (30 females; mean age: 36.7 years) with 45 (62.5%) diagnosed with axSpA. The external test set comprised 54 patients (16 females; mean age: 36.6 years), where 25 (46.3%) were diagnosed with axSpA. The prospective test set consisted of 87 patients (51 females; mean age: 38.2 years) with 53 (60.9%) diagnosed with axSpA. Patient characteristics are listed in Table 1 and Tables S3,S4. The inter-reader reliability of the RVOI annotations reached an average IoU of 0.906±0.090 in the retrospective cohorts and 0.867±0.105 in the prospective cohort, which was deemed reliable and thus had a low risk of introducing bias in the model training.
Table 1
Characteristics | All sets (n=541) | Training set (n=328) | Internal test set (n=72) | External test set (n=54) | Prospective test set (n=87) | P value |
---|---|---|---|---|---|---|
Age (years) | 37.0±16.0 | 36.9±17.0 | 36.7±15.1 | 36.6±12.7 | 38.2±14.4 | 0.732 |
Sex | 0.007 | |||||
Female | 241 (44.5) | 144 (44.0) | 30 (41.7) | 16 (29.6) | 51 (58.6) | |
Male | 300 (55.5) | 184 (56.0) | 42 (58.3) | 38 (70.4) | 36 (41.4) | |
Disease duration# | 12.0 (5.0, 48.0) | 18.5 (6.0, 60.0) | 12.0 (4.5, 60.0) | 7.0 (1.5, 36.0) | 12.0 (4.2, 48.0) | 0.136 |
Structural damage | 0.336 | |||||
Positive | 303 (56.0) | 177 (53.9) | 39 (54.2) | 36 (66.7) | 51 (58.6) | |
Negative | 238 (44.0) | 151 (46.1) | 33 (45.8) | 18 (33.3) | 36 (41.4) | |
SpA features | ||||||
Arthritis | 179 (33.1) | 95 (28.9) | 17 (23.6) | 41 (75.9) | 26 (29.9) | <0.001 |
Heel enthesitis | 31 (5.7) | 0 | 0 | 31 (57.4) | 0 | <0.001 |
Uveitis | 1 (0.1) | 0 | 0 | 1 (1.9) | 0 | 0.029 |
Dactylitis | 14 (2.6) | 0 | 0 | 12 (22.2) | 2 (2.3) | <0.001 |
Psoriasis | 3 (0.6) | 1 (0.3) | 1 (1.4) | 0 | 1 (1.1) | 0.544 |
Crohn’s disease or UC | 4 (0.7) | 2 (0.6) | 0 | 1 (1.9) | 1 (1.1) | 0.632 |
Good response to NSAIDs | 334 (61.7) | 234 (71.3) | 58 (80.5) | 14 (25.9) | 28 (32.2) | <0.001 |
Family history of axSpA | 58 (10.7) | 27 (8.2) | 18 (25.0) | 8 (14.8) | 5 (5.7) | <0.001 |
Elevated CRP concentration | 181 (33.5) | 114 (34.8) | 22 (30.6) | 32 (59.3) | 13 (14.9) | <0.001 |
Classification | ||||||
AxSpA | 261 (48.2) | 138 (42.1) | 45 (62.5) | 25 (46.3) | 53 (60.9) | |
Non-axSpA | 280 (51.8) | 190 (57.9) | 27 (37.5) | 29 (53.7) | 34 (39.1) |
Data are presented as median (Q1, Q3), mean ± standard deviation or number (%). P values represent the statistical differences among the training, internal, external, and prospective test sets. In the SpA features, data are numbers of positive patients with percentages in parentheses. M, months; UC, ulcerative colitis; NSAIDs, nonsteroidal anti-inflammatory drugs; axSpA, axial spondyloarthritis; CRP, C-reactive protein.
Performance of various MRI-based DL models
When using common data augmentations, Tri-ResNet50 demonstrated the most superior performance among all the MRI-based single-input, dual-input, and tri-input networks. On the internal test set, Tri-ResNet50 attained an AUC of 0.779 (95% CI: 0.663–0.895), F1 score of 0.778, and accuracy of 72.2% (95% CI: 60.4–82.1%). On the external test set, Tri-ResNet50 achieved the highest AUC of 0.778 (95% CI: 0.648–0.908) with P values <0.05, F1 score of 0.731 with accuracy of 74.1% (95% CI: 60.3–85.0%) (Table 2, Tables S5,S6, and Figures S4,S5). The implementation of MixCut further improved the performance of Tri-ResNet50. Specifically, on the internal test set, MixCut increased the AUC, F1 score, accuracy, sensitivity, and specificity of Tri-ResNet50 by 0.091 (P=0.004), 0.092, 11.1%, 11.1%, and 11.1%, respectively. On the external test set, MixCut led to enhancements of 0.062 in AUC (P=0.037), 0.084 in F1 score, 7.4% in accuracy, 12.0% in sensitivity, and 3.5% in specificity (Table 2). Additionally, Figure S6 visually illustrates that MixCut can create MRI samples with more diverse lesions for model training. Consequently, the Tri-ResNet50 with MixCut was selected for the subsequently development of NegSpA-AI.
Table 2
Framework | Aug. | Internal test set | External test set | |||||||||||
---|---|---|---|---|---|---|---|---|---|---|---|---|---|---|
AUC | P value | Accuracy | Sensitivity | Specificity | F1 | AUC | P value | Accuracy | Sensitivity | Specificity | F1 | |||
Tri-ResNet18 | Com. | 0.750 (0.635–0.863) |
0.374 | 69.4 (57.5–79.8) |
71.1 (55.7–83.6) |
66.7 (46.0–83.5) |
0.744 | 0.754 (0.616–0.893) |
0.056 | 66.7 (52.5,78.9) |
80.0 (59.3–93.2) |
55.2 (35.7– 73.6) |
0.690 | |
Tri-ResNet34 | Com. | 0.757 (0.636–.879) |
0.269 | 72.2 (60.4–82.1) |
68.9 (53.4–81.8) |
77.8 (57.7–91.4) |
0.756 | 0.755 (0.616–0.896) |
0.041 | 66.7 (52.5,78.9) |
72.0 (50.6–87.9) |
62.1 (42.3–79.3) |
0.667 | |
Tri-ResNet50 | Com. | 0.779 (0.663–0.895)† |
NA | 72.2 (60.4–82.1)† |
77.8 (62.9–88.8)† |
63.0 (42.4,80.6) |
0.778† | 0.778 (0.648–0.908)† |
NA | 74.1 (60.3–85.0)† |
76.0 (54.9–90.6)† |
72.4 (52.8–87.3)† |
0.731† | |
Tri-ResNet101 | Com. | 0.755 (0.638–0.871) |
0.352 | 69.4 (57.5–79.8) |
62.2 (46.5–76.2) |
81.5 (61.9–93.7) |
0.718 | 0.748 (0.600–0.894) |
0.080 | 68.5 (46.5–85.1) |
68.0 (46.5–85.1) |
69.0 (49.2–84.7) |
0.667 | |
Tri-VGG16 | Com. | 0.759 (0.647–0.871) |
0.028 | 65.3 (53.1–76.1) |
64.4 (48.8–78.1) |
66.7 (46.0–84.5) |
0.699 | 0.706 (0.551–0.861) |
0.010 | 63.0 (48.7–75.7) |
60.0 (38.7–78.9) |
65.5 (45.7–82.1) |
0.600 | |
Tri-ResNet50 | Mixup | 0.835 (0.720–0.933) |
0.015 | 79.2 (68.0–87.8) |
77.8 (62.9–88.8) |
81.5 (61.9–93.7) |
0.824 | 0.825 (0.712–0.938) |
0.149 | 77.8 (64.4–88.0) |
84.0 (63.9–95.5) |
72.4 (52.8–87.3) |
0.778 | |
Tri-ResNet50 | MixCut* | 0.870 (0.742–0.952)* |
0.004* | 83.3 (72.7–91.1)* |
88.9 (76.0–96.3)* |
74.1 (53.7–8.9) |
0.870* | 0.840 (0.730–0.950)* |
0.037* | 81.5 (68.6–90.8)* |
88.0 (68.8–97.5)* |
75.9 (56.5–89.7)* |
0.815* |
Accuracy, sensitivity, and specificity are expressed as percentages. Data in brackets are 95% confidence intervals. P values represent statistical AUC differences between Tri-ResNet50 model using the common data augmentations and other models. When fixing common data augmentations, the unique best performance of the optimal framework was shown in ‘†’; when fixing the optimal framework, the unique best performance of MixCut was shown in ‘*’. MRI, magnetic resonance imaging; Aug., data augmentation method; AUC, the area under the curve; F1, F1 score; Com., common; NA, not available.
Performance of NegSpA-AI
On the internal and external test sets, NegSpA-AI exhibited robust performance, achieving AUCs of 0.878 (95% CI: 0.786–0.971) and 0.870 (95% CI: 0.771–0.970), along with F1 scores of 0.891 and 0.830, respectively (Table 3). On the prospective test set, NegSpA-AI continued to demonstrate commendable performance, with an F1 score of 0.819, an AUC of 0.815 (95% CI: 0.714–0.915), and a sensitivity of 81.1% (95% CI: 68.0–90.6%). Moreover, our stratification analysis revealed that NegSpA-AI achieved more accurate diagnoses for patients in specific subgroups such as those younger than 28 years, with disease durations longer than 24 months, or exhibiting positive structural damage, compared to their respective comparative subgroups (refer to Figure 3A-3C). Interestingly, NegSpA-AI demonstrated higher accuracy in diagnosing females than males (Figure 3D).
Table 3
Data sets | AUC | Accuracy | Sensitivity | Specificity | F1-score |
---|---|---|---|---|---|
Internal test set | 0.878 (0.786–0.971) | 86.1 (75.9–93.1) | 91.1 (78.8–97.5) | 81.8 (57.7–91.4) | 0.891 |
External test set | 0.870 (0.771–0.970) | 83.3 (70.7–92.1) | 88.0 (68.8–97.5) | 80.3 (60.3–92.0) | 0.830 |
Prospective test set | 0.815 (0.714–0.915) | 79.2 (68.0–86.3) | 81.1 (68.0–90.6) | 79.5 (55.6–87.1) | 0.819 |
Accuracy, sensitivity, and specificity are expressed as percentages. Data in brackets are 95% confidence intervals. NegSpA-AI, an artificial intelligence tool to improve the diagnosis of axSpA in HLA-B27-negative patients; AUC, area under the curve.
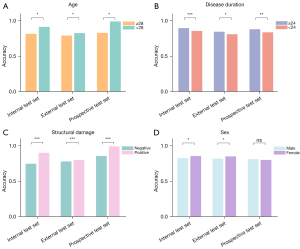
AI assistance for radiologists and rheumatologists
NegSpA-AI demonstrated superior performance compared to the junior rheumatologist (Figure 4), and most of the patients with accurate AI predictions but inaccurate clinician classifications were female and ≥28 years of age (see Table S7 for details). With AI assistance, the discriminating accuracy, sensitivity, and specificity of independent junior radiologists were respectively increased by 9.7–11.1%, 11.1–13.3%, and 7.4–7.4%, respectively, on the internal test set, and 7.4–7.5%, 1.0–4.0%, and 10.3–14.5%, respectively, on the external test set (all P<0.05; as depicted in Table 4 and Figure 4). AI assistance also enhanced the accuracy of independent senior radiologists by 5.5–5.6% and 7.4–11.1% on the internal and external test sets, respectively (all P<0.05). On the prospective test set, NegSpA-AI helped to significantly improve the diagnostic accuracy, sensitivity, and specificity of the junior rheumatologist by 7.7%, 7.7%, and 6.9%, respectively (independent vs. AI-assisted: accuracy 76.2% vs. 83.9%; sensitivity 77.2% vs. 84.9%; specificity 76.5% vs. 83.4%; all P<0.01).
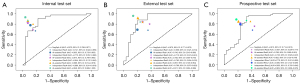
Table 4
Data sets | Clinician | Accuracy | Sensitivity | Specificity | ||||||||
---|---|---|---|---|---|---|---|---|---|---|---|---|
Independent | AI-assisted | P value | Independent | AI-assisted | P value | Independent | AI-assisted | P value | ||||
Internal test set | Rad1 | 72.2 (60.4–82.1) | 81.9 (71.1–90.0) | 0.0003 | 66.7 (51.0–80.0) | 77.8 (62.9–88.8) | 0.0007 | 81.5 (62.0–93.7) | 88.9 (70.8–97.6) | 0.007 | ||
Rad2 | 75.0 (63.4–84.5) | 86.1 (75.9–93.1) | 0.0002 | 68.9 (53.4–81.8) | 82.2 (68.0–92.0) | 0.0006 | 85.2 (66.3–95.8) | 92.6 (75.7–99.1) | 0.042 | |||
Rad3 | 83.3 (70.0–90.1) | 88.9 (79.3–95.1) | 0.008 | 80.0 (65.4–90.4) | 86.7 (73.2–95.0) | 0.032 | 85.0 (62.1–96.8) | 92.6 (75.7–99.1) | 0.008 | |||
Rad4 | 88.9 (79.3–95.1) | 94.4 (86.4–98.5) | 0.033 | 86.7 (73.2–94.9) | 93.3 (81.7–98.6) | 0.047 | 92.6 (75.7–99.1) | 96.3 (81.0–99.9) | 0.17 | |||
External test set | Rad1 | 66.7 (52.5–78.9) | 74.1 (60.3–85.0) | 0.0004 | 68.0 (46.5–85.1) | 69.0 (49.2–84.7) | 0.008 | 65.5 (45.7–82.1) | 80.0 (59.3–93.2) | 0.0006 | ||
Rad2 | 70.3 (56.4–82.0) | 77.8 (64.4–88.0) | 0.0002 | 80.0 (59.3–93.2) | 84.0 (63.9–95.5) | 0.01 | 62.1 (42.3–79.3) | 72.4 (52.7–87.3) | 0.009 | |||
Rad3 | 77.8 (64.4–88.0) | 85.2 (72.9–93.4) | 0.008 | 84.0 (63.9–95.5) | 92.0 (74.0–99.0) | 0.023 | 72.4 (52.8–87.3) | 79.3 (60.3–92.0) | 0.027 | |||
Rad4 | 79.6 (66.5–89.4) | 90.7 (79.7–96.9) | 0.039 | 84.0 (63.9–95.5) | 96.0 (79.6–99.9) | 0.28 | 75.9 (56.5–89.7) | 86.2 (68.3–96.1) | 0.041 | |||
Prospective test set | Rad2 | 67.8 (56.9–77.4) | 79.3 (69.3–87.3) | 0.016 | 75.5 (61.7–86.2) | 81.1 (68.0–90.6) | 0.022 | 55.9 (37.9–72.8) | 76.5 (58.8–89.3) | 0.031 | ||
Rad3 | 75.9 (65.5–84.4) | 82.8 (73.2–90.0) | 0.007 | 84.9 (72.4–93.3) | 84.9 (72.4–93.3) | 0.040 | 61.8 (43.6–77.8) | 79.4 (62.1–91.3) | 0.19 | |||
Rheu1 | 76.2 (68.0–86.3) | 83.9 (74.5–91.0) | 0.006 | 77.2 (65.9–89.2) | 84.9 (72.4–93.3) | 0.006 | 76.5 (58.8–89.3) | 83.4 (65.5–93.2) | 0.005 | |||
Rheu2 | 86.2 (77.1–92.7) | 88.5 (79.9–96.9) | 0.044 | 88.7 (77.0–95.7) | 90.6 (79.3–96.9) | 0.53 | 82.4 (65.5–93.2) | 85.3 (68.9–95.0) | 0.36 |
Accuracy, sensitivity, and specificity are expressed as percentages. Data in brackets are 95% confidence intervals. P<0.05 was considered significant. Rad1 and Rad2 are junior radiologists. Rad3 and Rad4 are senior radiologists. Rheu1 and Rheu2 are junior and senior rheumatologists, respectively. AI, artificial intelligence.
Visualization of NegSpA-AI
Grad-CAM heatmaps on representative MRI demonstrated that NegSpA-AI effectively focused on analyzing critical lesions, such as bone marrow edema, fat deposition, sclerosis, and bone erosion, thereby making accurate predictions (Figure 5A,5B). Nevertheless, despite its efficacy, NegSpA-AI misidentified locations on SIJ MRI and consequently made incorrect predictions for certain patients (Figure 5C,5D).
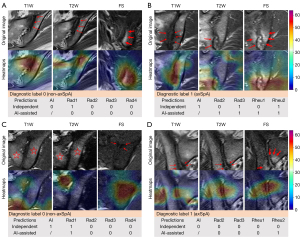
Discussion
In this multicenter study, we developed NegSpA-AI, an advanced semi-automatic AI model that integrates SIJ MRI and clinical SpA features to diagnose axSpA in HLA-B27-negative patients. NegSpA-AI concordantly demonstrated favorable diagnostic performance on the internal, external, and prospective test sets. The reader study highlighted NegSpA-AI’s significant contribution in offering valuable assistance to radiologists in interpreting SIJ MRI and rheumatologists in diagnosing axSpA in HLA-B27-negative patients. The promising outcomes suggest that NegSpA-AI has the potential to enhance clinical decision-making and facilitate appropriate treatment strategies in real-world clinical practice.
Accurate interpretation of SIJ MRI is crucial for diagnosing HLA-B27-negative axSpA. However, even experienced radiologists encounter challenges in precisely interpreting the atypical and confusing presentations of these MRIs (30). Previous studies have shown that interpretations of SIJ MRIs by well-trained musculoskeletal radiologists achieved only modest diagnostic performance, with the highest AUC reported at 0.736 for the diagnosis of non-radiographic HLA-B27 negative axSpA (31). With the rapid development of AI techniques, several investigations have applied machine learning to objectively analyze SIJ MRI in rheumatic diseases (21). For instance, Ye et al. constructed a clinical-radiomics nomogram model to differentiate axSpA from non-axSpA in patients with low back pain, achieving a validation AUC of 0.90 (23). Others have developed DL models to detect specific lesions on MRI of axSpA patients, for example, bone marrow edema, bone erosion, and active inflammatory sacroiliitis (32-34). Nevertheless, most existing models were designed using MRI to identify axSpA in general populations, without specifically addressing the diagnostic challenges associated with HLA-B27-negative axSpA. Furthermore, many of these models have not undergone real-world prospective testing to validate their performance. Therefore, their findings may have limited values in accurately interpreting SIJ MRI to enable the timely interpretation of HLA-B27-negative axSpA.
Rheumatologists have been reported to exhibit uncertainty of approximately 30% in the baseline diagnosis of axSpA among all suspected patients (24). The diagnostic uncertainty is further compounded in HLA-B27-negative populations (5). Therefore, this study focused specifically on HLA-B27-negative populations to distinguish axSpA from non-axSpA. Our NegSpA-AI model surpassed the current diagnostic performance of rheumatologists, demonstrating good robustness and generalization on the internal (AUC: 0.878; accuracy: 86.1%), external (AUC: 0.870; accuracy: 83.3%), and prospective test sets (AUC: 0.815; accuracy: 79.2%). Specifically, we found that the demographic diversities have an impact on the performance of NegSpA-AI. In the stratification analysis, NegSpA-AI obtained a higher diagnostic accuracy in the young, long-disease-duration, or positive-structural-damage patient subgroups compared to older, shorter-disease-duration, or negative-structural-damage subgroups (Figure 3A-3C), consistent with findings from previous studies (35). Notably, although previous studies have highlighted the diagnostics challenges in female patients due to their higher rates of negative SIJ MRI findings (36), our results revealed that NegSpA-AI achieved more accurate diagnosis of HLA-B27-negative axSpA in females than in males (Figure 3D). This discrepancy can be attributed to variations in patients’ HLA-B27 status (37). Previous studies have included over half of HLA-B27-positive patients with active disease courses, which were more prevalent in males. In contrast, our analysis focused solely on HLA-B27-negative patients, who typically have milder disease courses and a higher proportion of females (16). As a result, the developed NegSpA-AI effectively improves the diagnoses of previously difficult-to-diagnose HLA-B27-negative axSpA, which can be used to guide the timely intervention and ultimately benefit patients’ health-related quality of life and outcomes.
The main technical strengths of NegSpA-AI lie in its 2-stage transfer learning strategy and the MixCut method, which address challenges related to small training datasets and data differences and ensure fairness of the model. The tri-input structure enables NegSpA-AI to simultaneously learn different axSpA lesions from the T1W, T2W, and FS sequences. However, this architecture may encounter convergence difficulties and unstable performance. Previous studies have utilized transfer learning with pre-trained models to mitigate these issues (38,39). Building upon this, we implemented an improved 2-stage transfer learning strategy introduced by Howard (40) to fine-tune our tri-input framework. Ablation analysis showed that images from the T1W sequence were critical for distinguishing axSpA from non-axSpA in HLA-B27-negative patients, whereas our tri-input framework robustly fused complementary information of images from the T1W and the other 2 sequences to achieve superior performance (Tables S5,S6). Furthermore, we proposed a novel data augmentation method called MixCut to alleviate the potential bias in model training with limited training data. Although common data augmentations have been widely used to increase training sample size, they often struggle with out-of-distribution images (41). To enhance model generalization, Mixup (42) and Cutmix (43) were proposed to create new training samples by globally mixing 2 images, albeit without considering local contextual information. In contrast, MixCut considers the saliency of both input and mixed samples to create virtual MRI samples with diverse local lesions (as illustrated in Figure S6), thereby improving AUCs of common data augmentations by 0.062–0.091 (P<0.05) and Mixup by 0.015–0.035 on the test sets (Table 2). Finally, these advantages enhanced the performance of NegSpA-AI to surpass that of junior rheumatologists.
The issue of whether AI techniques can provide support for clinical decision-making remains a crucial concern (44). Several studies have demonstrated the reliability of interactive AI systems in aiding clinical diagnosis, particularly in the context of cancer (45,46). In this study, AI was more accurate than clinicians in classifying cases who were female and are older than 28 years (Table S7). Our reader study confirmed that the heatmaps and prediction scores generated from NegSpA-AI helped clinicians make decisions more accurately and confidently in the above cases. Junior radiologists are prone to confuse axSpA with its mimickers; the heatmaps can indicate small lesions (e.g., bone erosion and osteosclerosis) for them. For example, a 30-year-old woman with non-axSpA (osteitis condensans ilii) who was initially misclassified as having axSpA by junior Radiologist 1 (Rad1) was corrected to non-axSpA upon observing highlighted sclerosis areas on the heatmaps and a low prediction score of axSpA (Figure 5A). Senior radiologists have usually consolidated their routine MRI assessment procedures (47), and NegSpA-AI primarily assisted them in identifying symptomatic lesions on SIJ MRI to exclude non-axSpA in ambiguous cases. Our rheumatologists achieved accuracies ranging from 76.2% to 86.2% in their independent diagnoses of HLA-B27-negative axSpA on the prospective test set, surpassing those reported in previous studies (24). This improvement may be attributed to the clear instruction provided to our rheumatologists to perform a differential diagnosis between axSpA and non-axSpA, giving them favorable hints in the diagnoses. In this context, AI assistance still significantly increased the diagnostic accuracy, sensitivity, and specificity of the junior rheumatologist by 7.7%, 7.7%, and 6.9%, respectively (all P<0.01). Taken together, NegSpA-AI facilitated a more accurate interpretation of SIJ MRI and diagnosis of axSpA in HLA-B27-negative patients, which is expected to alleviate the current clinical problem of delayed diagnosis of HLA-B27-negative axSpA, especially in females and those older than 28 years.
This study has several limitations. First, all enrolled patients are of the same race, and patients with several non-axSpA diseases (e.g., bone tuberculosis and chondrosarcoma) were not included, which may have introduced selection bias and affected the model’s widespread applicability. Second, we did not analyze patients with a positive spine MRI, which may have hindered the diagnosis of axSpA affecting only the spine. Since the spine is one of the blind spots in the diagnosis of axSpA, further training and implementation of NegSpA-AI will be needed on spine MRI to pave the way for a better diagnosis of those cases. Third, structural changes assessed by radiologists on computed tomography (CT) scans are usually accepted as the gold standard. However, due to the limited number of patients with low back pain who underwent CT, we only evaluated structural damages on MRI, which may have led to biased assessments of structural damages. Fourth, there is no standard sequence for FS, whereas several FS sequences on different MRI devices constituted the FS sequences. Fifth, the number of patients from the external general hospital was smaller than that of those from the internal orthopedic hospital, which also resulted in different male-to-female ratios. Further work will include larger external datasets for validation. Sixth, NegSpA-AI was developed as a semi-automatic model as it requires manual annotations by clinicians. We will work on adding a RVOI detection task to extend NegSpA-AI to a fully automatic model.
Conclusions
This study successfully constructed a semi-automatic AI model that integrates SIJ MRI and clinical SpA features to enhance the diagnosis of axSpA in HLA-B27-negative patients. Using a 2-stage transfer learning and the novel MixCut method, the constructed NegSpA-AI demonstrated good robustness and generalization across both retrospective and prospective test sets. By assisting both radiologists and rheumatologists in the more accurate interpretation and diagnosis of HLA-B27-negative axSpA, NegSpA-AI holds the potential to facilitate timely diagnosis and improve treatment outcomes for patients with axSpA.
Acknowledgments
We thank Caolin Liu, MS from Nanhai Hospital, and Shisi Li, MD from the Third Affiliated Hospital of Southern Medical University, for their help in collection and quality control of data. Professional English language editing support was provided by AsiaEdit (asiaedit.com).
Funding: This work was supported by
Footnote
Reporting Checklist: The authors have completed the TRIPOD-AI reporting checklist. Available at https://qims.amegroups.com/article/view/10.21037/qims-24-729/rc
Conflicts of Interest: All authors have completed the ICMJE uniform disclosure form (available at https://qims.amegroups.com/article/view/10.21037/qims-24-729/coif). The authors have no conflicts of interest to declare.
Ethical Statement: The authors are accountable for all aspects of the work in ensuring that questions related to the accuracy or integrity of any part of the work are appropriately investigated and resolved. The study was conducted in accordance with the Declaration of Helsinki (as revised in 2013). The study was approved by the Regional Institutional Review Board of the Third Affiliated Hospital of Southern Medical University and followed by Nanhai Hospital (No. 201501003). The requirement for the informed consent of patients in the retrospective cohorts was waived. Patients in the prospective cohort provided written informed consent that clearly stated that all the collected information would be used for publication by the investigator.
Open Access Statement: This is an Open Access article distributed in accordance with the Creative Commons Attribution-NonCommercial-NoDerivs 4.0 International License (CC BY-NC-ND 4.0), which permits the non-commercial replication and distribution of the article with the strict proviso that no changes or edits are made and the original work is properly cited (including links to both the formal publication through the relevant DOI and the license). See: https://creativecommons.org/licenses/by-nc-nd/4.0/.
References
- Poddubnyy D, Sieper J, Akar S, Muñoz-Fernández S, Haibel H, Hojnik M, Ganz F, Inman RD. Characteristics of patients with axial spondyloarthritis by geographic regions: PROOF multicountry observational study baseline results. Rheumatology (Oxford) 2022;61:3299-308. [Crossref] [PubMed]
- Bakland G, Alsing R, Singh K, Nossent JC. Assessment of SpondyloArthritis International Society criteria for axial spondyloarthritis in chronic back pain patients with a high prevalence of HLA-B27. Arthritis Care Res (Hoboken) 2013;65:448-53. [Crossref] [PubMed]
- Navarro-Compán V, Sepriano A, El-Zorkany B, van der Heijde D. Axial spondyloarthritis. Ann Rheum Dis 2021;80:1511-21. [Crossref] [PubMed]
- van Lunteren M, van der Heijde D, Sepriano A, Berg IJ, Dougados M, Gossec L, Jacobsson L, Ramonda R, Rudwaleit M, Sieper J, Landewé R, van Gaalen FA. Is a positive family history of spondyloarthritis relevant for diagnosing axial spondyloarthritis once HLA-B27 status is known? Rheumatology (Oxford) 2019;58:1649-54. [Crossref] [PubMed]
- Coates LC, Baraliakos X, Blanco FJ, Blanco-Morales EA, Braun J, Chandran V, Fernandez-Sueiro JL, FitzGerald O, Gallagher P, Gladman DD, Gubar E, Korotaeva T, Loginova E, Lubrano E, Mulero J, Pinto-Tasende J, Queiro R, Sanz Sanz J, Szentpetery A, Helliwell PS. The Phenotype of Axial Spondyloarthritis: Is It Dependent on HLA-B27 Status? Arthritis Care Res (Hoboken) 2021;73:856-60. [Crossref] [PubMed]
- Mauro D, Forte G, Poddubnyy D, Ciccia F. The Role of Early Treatment in the Management of Axial Spondyloarthritis: Challenges and Opportunities. Rheumatol Ther 2024;11:19-34. [Crossref] [PubMed]
- Ferraz-Amaro I, Rueda-Gotor J, Genre F, Corrales A, Blanco R, Portilla V, et al. Potential relation of cardiovascular risk factors to disease activity in patients with axial spondyloarthritis. Ther Adv Musculoskelet Dis 2021;13:1759720X211033755.
- Inman RD, Garrido-Cumbrera M, Chan J, Cohen M, de Brum-Fernandes AJ, Gerhart W, Haroon N, Jovaisas AV, Major G, Mallinson MG, Rohekar S, Leclerc P, Rahman P. Work-Related Issues and Physical and Psychological Burden in Canadian Patients With Axial Spondyloarthritis: Results From the International Map of Axial Spondyloarthritis. J Rheumatol 2023;50:625-33. [Crossref] [PubMed]
- Ye L, Liu Y, Xiao Q, Dong L, Wen C, Zhang Z, Jin M, Brown MA, Chen D. MRI compared with low-dose CT scanning in the diagnosis of axial spondyloarthritis. Clin Rheumatol 2020;39:1295-303. [Crossref] [PubMed]
- van der Linden S, Valkenburg HA, Cats A. Evaluation of diagnostic criteria for ankylosing spondylitis. A proposal for modification of the New York criteria. Arthritis Rheum 1984;27:361-8. [Crossref] [PubMed]
- Protopopov M, Proft F, Wichuk S, Machado PM, Lambert RG, Weber U, Juhl Pedersen S, Østergaard M, Sieper J, Rudwaleit M, Baraliakos X, Maksymowych WP, Poddubnyy D. Comparing MRI and conventional radiography for the detection of structural changes indicative of axial spondyloarthritis in the ASAS cohort. Rheumatology (Oxford) 2023;62:1631-5. [Crossref] [PubMed]
- Robinson PC, van der Linden S, Khan MA, Taylor WJ. Axial spondyloarthritis: concept, construct, classification and implications for therapy. Nat Rev Rheumatol 2021;17:109-18. [Crossref] [PubMed]
- Rudwaleit M, van der Heijde D, Landewé R, Listing J, Akkoc N, Brandt J, et al. The development of Assessment of SpondyloArthritis international Society classification criteria for axial spondyloarthritis (part II): validation and final selection. Ann Rheum Dis 2009;68:777-83. [Crossref] [PubMed]
- Maksymowych WP. The role of imaging in the diagnosis and management of axial spondyloarthritis. Nat Rev Rheumatol 2019;15:657-72. [Crossref] [PubMed]
- Pohlner T, Deppe D, Ziegeler K, Proft F, Protopopov M, Rademacher J, Rios Rodriguez V, Torgutalp M, Braun J, Diekhoff T, Poddubnyy D. Diagnostic accuracy in axial spondyloarthritis: a systematic evaluation of the role of clinical information in the interpretation of sacroiliac joint imaging. RMD Open 2024;10:e004044. [Crossref] [PubMed]
- Deodhar A, Gill T, Magrey M. Human Leukocyte Antigen B27-Negative Axial Spondyloarthritis: What Do We Know? ACR Open Rheumatol 2023;5:333-44. [Crossref] [PubMed]
- Ulas ST, Radny F, Ziegeler K, Eshed I, Greese J, Deppe D, Stelbrink C, Biesen R, Haibel H, Rios Rodriguez V, Rademacher J, Protopopov M, Proft F, Poddubnyy D, Diekhoff T. Self-reported diagnostic confidence predicts diagnostic accuracy in axial spondyloarthritis imaging. Rheumatology (Oxford) 2023; Epub ahead of print. [Crossref]
- Wei L, Niraula D, Gates EDH, Fu J, Luo Y, Nyflot MJ, Bowen SR, El Naqa IM, Cui S. Artificial intelligence (AI) and machine learning (ML) in precision oncology: a review on enhancing discoverability through multiomics integration. Br J Radiol 2023;96:20230211. [Crossref] [PubMed]
- Folle L, Bayat S, Kleyer A, Fagni F, Kapsner LA, Schlereth M, Meinderink T, Breininger K, Tascilar K, Krönke G, Uder M, Sticherling M, Bickelhaupt S, Schett G, Maier A, Roemer F, Simon D. Advanced neural networks for classification of MRI in psoriatic arthritis, seronegative, and seropositive rheumatoid arthritis. Rheumatology (Oxford) 2022;61:4945-51. [Crossref] [PubMed]
- Bressem KK, Adams LC, Proft F, Hermann KGA, Diekhoff T, Spiller L, Niehues SM, Makowski MR, Hamm B, Protopopov M, Rios Rodriguez V, Haibel H, Rademacher J, Torgutalp M, Lambert RG, Baraliakos X, Maksymowych WP, Vahldiek JL, Poddubnyy D. Deep Learning Detects Changes Indicative of Axial Spondyloarthritis at MRI of Sacroiliac Joints. Radiology 2022;305:655-65. [Crossref] [PubMed]
- Adams LC, Bressem KK, Ziegeler K, Vahldiek JL, Poddubnyy D. Artificial intelligence to analyze magnetic resonance imaging in rheumatology. Joint Bone Spine 2024;91:105651. [Crossref] [PubMed]
- Harvey H, Glocker B. A standardised approach for preparing imaging data for machine learning tasks in radiology. Artificial intelligence in medical imaging: opportunities, applications and risks. Artificial Intelligence in Medical Imaging 2019:61-72.
- Ye L, Miao S, Xiao Q, Liu Y, Tang H, Li B, Liu J, Chen D. A predictive clinical-radiomics nomogram for diagnosing of axial spondyloarthritis using MRI and clinical risk factors. Rheumatology (Oxford) 2022;61:1440-7. [Crossref] [PubMed]
- Marques ML, Ramiro S, van Lunteren M, Stal RA, Landewé RB, van de Sande M, Fagerli KM, Berg IJ, van Oosterhout M, Exarchou S, Ramonda R, van der Heijde D, van Gaalen FA. Can rheumatologists unequivocally diagnose axial spondyloarthritis in patients with chronic back pain of less than 2 years duration? Primary outcome of the 2-year SPondyloArthritis Caught Early (SPACE) cohort. Ann Rheum Dis 2024;83:589-98. [Crossref] [PubMed]
- Maksymowych WP, Lambert RG, Østergaard M, Pedersen SJ, Machado PM, Weber U, et al. MRI lesions in the sacroiliac joints of patients with spondyloarthritis: an update of definitions and validation by the ASAS MRI working group. Ann Rheum Dis 2019;78:1550-8. [Crossref] [PubMed]
- Ziade N, Maroof A, Elzorkany B, Abdullateef N, Adnan A, Abogamal A, Saad S, El Kibbi L, Alemadi S, Ansari A, Abi Najm A, Younan T, Kharrat K, Sebaaly A, Rachkidi R, Witte T, Baraliakos X. What is the best referral strategy for axial spondyloarthritis? A prospective multicenter study in patients with suspicious chronic low back pain. Joint Bone Spine 2023;90:105579. [Crossref] [PubMed]
- Jamal M, van Delft ETAM, den Braanker H, Kuijper TM, Hazes JMW, Lopes Barreto D, Weel AEAM. Increase in axial spondyloarthritis diagnoses after the introduction of the ASAS criteria: a systematic review. Rheumatol Int 2023;43:639-49. [Crossref] [PubMed]
.Simonyan K Zisserman A Very deep convolutional networks for large-scale image recognition. arXiv: 14091556,2014 .- He K, Zhang X, Ren S, Sun J. Deep residual learning for image recognition. 2016 IEEE Conference on Computer Vision and Pattern Recognition (CVPR), Las Vegas, NV, USA, 2016:770-8.
- van den Berg R, Lenczner G, Thévenin F, Claudepierre P, Feydy A, Reijnierse M, Saraux A, Rahmouni A, Dougados M, van der Heijde D. Classification of axial SpA based on positive imaging (radiographs and/or MRI of the sacroiliac joints) by local rheumatologists or radiologists versus central trained readers in the DESIR cohort. Ann Rheum Dis 2015;74:2016-21. [Crossref] [PubMed]
- Lu CC, Huang GS, Lee TS, Chao E, Chen HC, Guo YS, Chu SJ, Liu FC, Kao SY, Hou TY, Chen CH, Chang DM, Lyu SY. MRI contributes to accurate and early diagnosis of non-radiographic HLA-B27 negative axial spondyloarthritis. J Transl Med 2021;19:298. [Crossref] [PubMed]
- Castro-Zunti R, Park EH, Choi Y, Jin GY, Ko SB. Early detection of ankylosing spondylitis using texture features and statistical machine learning, and deep learning, with some patient age analysis. Comput Med Imaging Graph 2020;82:101718. [Crossref] [PubMed]
- Lee KH, Choi ST, Lee GY, Ha YJ, Choi SI. Method for Diagnosing the Bone Marrow Edema of Sacroiliac Joint in Patients with Axial Spondyloarthritis Using Magnetic Resonance Image Analysis Based on Deep Learning. Diagnostics (Basel) 2021;11:1156. [Crossref] [PubMed]
- Lin KYY, Peng C, Lee KH, Chan SCW, Chung HY. Deep learning algorithms for magnetic resonance imaging of inflammatory sacroiliitis in axial spondyloarthritis. Rheumatology (Oxford) 2022;61:4198-206. [Crossref] [PubMed]
- Marzo-Ortega H, Navarro-Compán V, Akar S, Kiltz U, Clark Z, Nikiphorou E. The impact of gender and sex on diagnosis, treatment outcomes and health-related quality of life in patients with axial spondyloarthritis. Clin Rheumatol 2022;41:3573-81. [Crossref] [PubMed]
- Lorenzin M, Cozzi G, Scagnellato L, Ortolan A, Vio S, Striani G, Scapin V, De Conti G, Doria A, Ramonda R. Relationship between sex and clinical and imaging features of early axial spondyloarthritis: results from a 48 month follow-up (Italian arm of the SPondyloArthritis Caught Early (SPACE) study). Scand J Rheumatol 2023;52:519-29. [Crossref] [PubMed]
- Mariani FM, Alunno A, Di Ruscio E, Altieri P, Ferri C, Carubbi F. Human Leukocyte Antigen B*27-Negative Spondyloarthritis: Clinical, Serological, and Radiological Features of a Single-Center Cohort. Diagnostics (Basel) 2023;13:3550. [Crossref] [PubMed]
- Tas NP, Kaya O, Macin G, Tasci B, Dogan S, Tuncer T. ASNET: A Novel AI Framework for Accurate Ankylosing Spondylitis Diagnosis from MRI. Biomedicines 2023;11:2441. [Crossref] [PubMed]
- Anaya-Isaza A, Mera-Jiménez L, Verdugo-Alejo L, Sarasti L. Optimizing MRI-based brain tumor classification and detection using AI: A comparative analysis of neural networks, transfer learning, data augmentation, and the cross-transformer network. Eur J Radiol Open 2023;10:100484. [Crossref] [PubMed]
- Howard J, Thomas R, Gugger S. fast. ai. GitHub. 2018.
- Panfilov E, Tiulpin A, Klein S, Nieminen MT, Saarakkala S. Improving Robustness of Deep Learning Based Knee MRI Segmentation: Mixup and Adversarial Domain Adaptation. 2019 IEEE/CVF International Conference on Computer Vision Workshops (ICCVW), 2019:450-9.
- Zhang H, Cisse M, Dauphin YN, Lopez-Paz D. mixup: Beyond Empirical Risk Minimization. 2018 International Conference on Learning Representations (Proceedings of Machine Learning Research, Stockholmsmässan, Stockholm, Sweden), 2018.
- Yun S, Han D, Chun S, Oh SJ, Yoo Y, Choe J. CutMix: Regularization Strategy to Train Strong Classifiers With Localizable Features. Proceedings of the IEEE/CVF International Conference on Computer Vision (ICCV), 2019:6023-32.
- Hamon R, Junklewitz H, Sanchez I, Malgieri G, De Hert P. Bridging the gap between AI and explainability in the GDPR: towards trustworthiness-by-design in automated decision-making. IEEE Computational Intelligence Magazine 2022;17:72-85.
- Schaffter T, Buist DSM, Lee CI, Nikulin Y, Ribli D, Guan Y, et al. Evaluation of Combined Artificial Intelligence and Radiologist Assessment to Interpret Screening Mammograms. JAMA Netw Open 2020;3:e200265. [Crossref] [PubMed]
- Hamm CA, Baumgärtner GL, Biessmann F, Beetz NL, Hartenstein A, Savic LJ, Froböse K, Dräger F, Schallenberg S, Rudolph M, Baur ADJ, Hamm B, Haas M, Hofbauer S, Cash H, Penzkofer T. Interactive Explainable Deep Learning Model Informs Prostate Cancer Diagnosis at MRI. Radiology 2023;307:e222276. [Crossref] [PubMed]
- Yoon SY, Lee KS, Bezuidenhout AF, Kruskal JB. Spectrum of Cognitive Biases in Diagnostic Radiology. Radiographics 2024;44:e230059. [Crossref] [PubMed]