The value of radiomics features of the spleen as surrogates for differentiating subtypes of common pediatric lymphomas
Introduction
Lymphoma, a hematological disease, has a complex set of subtypes and is one of the most common malignancy among both adult and pediatric patients (1,2). However, children and adults with lymphoma have significantly different pathologic subtypes in clinical practice. For example, Hodgkin lymphoma exhibits a bimodal distribution, peaking between the ages of 15 and 30 years and again in patients aged >55 years; however, it is rare among pediatric patients under the age of 5 years (3). Diffuse large B-cell lymphoma (DLBCL) is a non-Hodgkin lymphoma (NHL) and has the highest incidence among adult patients (4). Burkitt lymphoma (BL) and lymphoblastoid lymphoma (LBL) are the two most prevalent subtypes in pediatric patients (5), and these two subtypes have distinctly different therapy protocols in clinic (6,7). Therefore, accurate diagnosis of pathological subtypes of lymphoma is critical to effective clinical practice.
Image examinations play an important role in the staging of lymphoma (8). Enhanced computed tomography (CT) is the most commonly used imaging modality for directly visualizing enlarged lymph nodes and extranodal organ involvement. Although different subtypes are associated with different typical imaging features (9,10), accurate diagnostic pathologic typing from images remain challenging. For instance, in some cases, the patients do not manifest enlarged lymph nodes, and in some CT images, the scanning range does not contain enlarged lymph nodes, which makes it even more difficult to diagnose lymphoma on CT.
The spleen is the major lymphatic organ in the human body, and most of the studies on the spleen in those with lymphoma have focused on examining the involvement of spleen (11-14). Although the spleen in some adult patients with lymphoma exhibits no obvious abnormalities on conventional images, radiomic features of the spleen can still be used to differentiate common subtypes of adult lymphoma (15). Therefore, we believe that CT images of the spleen hold the potential to yield more comprehensive insights into lymphoma. Radiomics, as an emerging technology, can reflect tissue heterogeneity in conventional images (16) by extracting a massive number of features images for high-throughput, quantitative analysis (17). Therefore, the purpose of this study was to identify LBL from BL using radiomics features of the spleen. We present this article in accordance with the TRIPOD reporting checklist (available at https://qims.amegroups.com/article/view/10.21037/qims-24-122/rc).
Methods
This study was conducted in accordance with the Declaration of Helsinki (as revised in 2013) and was approved by the Ethics Committee of the Children’s Hospital of Chongqing Medical University (issue No. 386[2023]; date: 2023.8.20). The requirement of individual consent for this retrospective analysis was waived.
Patients
A retrospective analysis of patients with BL and LBL who attended the Children’s Hospital of Chongqing Medical University was conducted. We collected the data of 279 patients with pediatric lymphoma treated from 2012 to 2023. The inclusion criteria were as follows: (I) pathologically confirmed BL or and LBL and (II) complete noncontrast, arterial-phase, and venous-phase CT images. Meanwhile, the exclusion criteria were as follows: (I) incomplete spleen on CT images, (II) incomplete images or poor image quality, and (III) previous treatment. Data from 61 patients with BL and 48 patients with LBL treated from 2012 to 2023 were collected. The oldest patient at the time of the initial consultation was no more than 18 years old. The mean imaging date for patients with LBL was August 15, 2018, and that for those with BL was June 2, 2019. The flowchart of patient inclusion is shown in Figure 1.
CT image acquisition
Transverse-axis scanning was performed using a LightSpeed VCT 64-row spiral CT machine (GE HealthCare, Chicago, IL, USA) and a Brilliance iCT 256-row spiral CT machine (Philips, Amsterdam, the Netherlands) under the following parameters: tube voltage, 80–110 kV; automatic adjustment of tube current; layer thickness, 5.0 mm; and layer spacing, 5.0 mm. As an uncooperative patient can result in lower image quality, we sedated any patients who were unable to cooperate after obtaining consent from the patient’s parents using 10% chloral hydrate (0.5 mL/kg) administered orally or intramuscular phenobarbital sodium injection (5 mg/kg).
Segmentation, preprocessing, and feature extraction
CT images of the spleen in the noncontrast, arterial, and venous phases were automatically segmented using the uAI portal version 20230515 (Shanghai United Imaging Intelligence Co., Shanghai, China). We used a well-established deep learning model based on a visual basic net (VB-Net) to automatically segment the region of interest (ROI) (18). The algorithm was validated on 28,581 cases of data, 17% of which served as an independent test set. The results of the test set showed that the algorithm segmentation Dice value was 0.85±0.03, and it took less than 2 seconds to segment the ROI of a patient. Subsequently, the radiologist checked the segmented ROIs (Figure 2), and manual modifications were necessary in a limited number of cases. Preprocessing tasks, including discretization (bin width: 25), normalization (window level: 40; window width: 400), and image resampling (1×1×1 mm3; interpolator: B-spline), were performed using the abovementioned software before feature extraction. The purpose of preprocessing is to eliminate image differences caused by different scanning equipment. In this study, feature selection was performed only in the training set, and transformation was performed on both the training and test set. For all radiomics features, Z-score normalization was performed to eliminate the effect of feature dimensionality on the model. We obtained the mean and variance of the features based on the training set data and further performed Z-score normalization on the training set. Z-score normalization of features in the test set was performed using the mean and variance obtained from the training set. The most relevant features were identified in order using SelectKBest (k value: 15; method: F-value; P value: 0.05), maximum relevance and minimum redundancy (mRMR) (n selected features: 10), and least absolute shrinkage and selection operator (LASSO) algorithms (alpha value: 0.05). The selected features were used to construct radiomics models. To build an optimal classification model, we integrated the final selected features from CT images of different phases, and since there were more features after integration, we again performed feature selection using the LASSO algorithm.
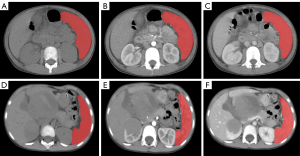
Model construction
All cases were completely randomized into training and test sets in a ratio of 7:3. Three classifiers, quadratic discriminant analysis (QDA) (regularization parameter =1.0; tolerance =0.0001), logistic regression (LR) (penalty = L2; tolerance =0.0001; C=1.0), and support vector machine (SVM) (gamma =0.01; Kernel = rbf; c=1.0), were chosen for modeling. The models were systematically constructed in the noncontrast CT images, arterial phases CT images, and venous phases CT images, respectively. As mentioned previously, we integrated the radiomics features of different phases for the construction of fusion models. Fusion models were created in the same way. We referred to the radiomics features used to construct fusion models as Feature 1 (noncontrast CT + arterial phase), Feature 2 (noncontrast CT + venous phase), Feature 3 (arterial phase+ venous phase), and Feature 4 (noncontrast CT + arterial phase + venous phase). Similarly, the constructed fusion models were referred to as Model 1, Model 2, Model 3, and Model 4. Finally, we validated the performance of the radiomics models in the training and test sets using evaluation metrics of sensitivity, specificity, accuracy, precision, and F1-score.
Statistical methods
Data analysis was conducted using SPSS 25.0 software (IBM Corp., Armonk, NY, USA). The chi-square test was used for categorical variables, while the t-test was used for continuous variables. Receiver operating characteristic (ROC) curves, decision curve analysis (DCA), and calibration curves were used to visualize the strengths and weaknesses of the models. Key metrics, including area under the curve (AUC), accuracy, sensitivity, specificity, precision, and F1-score were calculated to evaluate and compare the results of the models.
Results
Patients
We compared the patients with LBL and those with BL in terms of clinical characteristics, including gender, age, involvement stage, splenic involvement, and we found no statistically significant differences between these two groups of patients. The detailed results are shown in Table 1. In addition, patients were referred for positron emission tomography-CT (PET/CT) scans after diagnosis of lymphoma, and we could ascertain from the PET/CT report if the patient’s spleen was involved. If patients did not undergo PET/CT, splenic involvement was defined as a craniocaudal diameter >13 cm on CT.
Table 1
Characteristic | LBL (n=48) | BL (n=61) | P value |
---|---|---|---|
Gender (%) | 0.108 | ||
Female | 15 (31.3) | 11 (18.0) | |
Male | 33 (68.7) | 50 (82.0) | |
Splenic involvement (%) | 0.923 | ||
Yes | 9 (18.8) | 11 (18.0) | |
No | 39 (81.2) | 50 (82.0) | |
Involvement stage (%) | 0.724 | ||
I | 0 (0.0) | 0 (0.0) | |
II | 4 (8.3) | 4 (6.6) | |
III | 23 (47.9) | 44 (72.1) | |
IV | 21 (43.8) | 13 (21.3) | |
Age (years), median (Q1–Q3) | 8.14 (5–11) | 6.57 (4–10) | 0.417 |
LBL, lymphoblastic lymphoma; BL, Burkitt lymphoma.
Feature selection
A total of 2,264 features were automatically extracted. First, 15 features were selected by SelectKBest, mRMR reduced these to 10 features, and finally, the selected features were derived via the LASSO algorithm. The selected features of each single and fused models are available in the supplementary material (Table S1). Figure 3 shows the features of fusion Model 4 and its corresponding coefficients, including 4 first-order features, 2 gray-level co-occurrence matrix (GLCM) features, 2 gray-level size zone matrix (GLSZM) features, and 2 gray-level run-length matrix (GLRLM) features.
Single model construction and evaluation
Table 2 lists the AUCs and other results for the training and test sets of each model. More detailed results are available in the supplementary material (Table S2). Among the three different classifiers—QDA, LR, and SVM—the highest AUC values were generated by the nonenhanced CT models. The AUCs in the training and tests sets, respectively, for QDA CT were 0.85 (95% CI: 0.76–0.94) and 0.719 (95% CI: 0.541–0.896), those for LR CT were 0.868 (95% CI: 0.785–0.95) and 0.726 (95% CI: 0.55–0.902), and those for SVM CT were 0.872 (95% CI: 0.793–0.952) and 0.737 (95% CI: 0.565–0.909). According to the Delong test (Tables S3-S5), the AUC of QDA CT was significantly higher than that of QDA venous phase, and the AUC of logistic CT was significantly higher than that of the logistic arterial phase in the training set.
Table 2
Model name | Training group | Testing group | |||||||
---|---|---|---|---|---|---|---|---|---|
AUC (95% CI) | Sensitivity | Specificity | F1-score | AUC (95% CI) | Sensitivity | Specificity | F1-score | ||
QDA_CT | 0.85 (0.76–0.94) | 0.698 | 0.818 | 0.759 | 0.719 (0.541–0.896) | 0.667 | 0.6 | 0.667 | |
Logistic_CT | 0.868 (0.785–0.95) | 0.767 | 0.818 | 0.805 | 0.726 (0.55–0.902) | 0.611 | 0.6 | 0.629 | |
SVM_CT | 0.872 (0.793–0.952) | 0.86 | 0.667 | 0.813 | 0.737 (0.565–0.909) | 0.722 | 0.6 | 0.703 | |
QDA_arterial | 0.753 (0.645–0.862) | 0.721 | 0.636 | 0.721 | 0.73 (0.551–0.909) | 0.722 | 0.6 | 0.703 | |
Logistic_arterial | 0.757 (0.649–0.865) | 0.698 | 0.636 | 0.706 | 0.726 (0.545–0.907) | 0.667 | 0.6 | 0.667 | |
SVM_arterial | 0.791 (0.691–0.892) | 0.86 | 0.636 | 0.804 | 0.674 (0.483–0.866) | 0.611 | 0.4 | 0.579 | |
QDA_venous | 0.776 (0.667–0.884) | 0.721 | 0.636 | 0.721 | 0.707 (0.519–0.896) | 0.667 | 0.8 | 0.727 | |
Logistic_venous | 0.787 (0.678–0.896) | 0.721 | 0.697 | 0.738 | 0.693 (0.503–0.882) | 0.667 | 0.667 | 0.686 | |
SVM_venous | 0.796 (0.691–0.901) | 0.744 | 0.667 | 0.744 | 0.704 (0.52–0.888) | 0.667 | 0.667 | 0.686 |
AUC, area under the curve; CI, confidence interval; QDA, quadratic discriminant analysis; CT, computed tomography; SVM, support vector machine.
Evaluation of the fusion models
Figure 4 depicts each fusion model’s ROC curves, and Table 3 lists the AUC values of each fusion model’s training and test sets, along with other evaluation criteria. More detailed results can be found in the supplementary material (Table S6) We can see that the SVM_4 model [AUC =0.967, 95% confidence interval (CI): 0.935–0.998; AUC =0.754, 95% CI: 0.584–0.924] had the highest AUC value in the training set, while the models with the highest AUC value in the test set were SVM_1 (AUC =0.888, 95% CI: 0.812–0.964; AUC =0.763, 95% CI: 0.594–0.932), QDA_4 (AUC =0.825, 95% CI: 0.729–0.92; AUC =0.763, 95% CI: 0.59–0.935), and Logistic_4 (AUC =0.844, 95% CI: 0.754–0.933; AUC = 0.763, 95% CI: 0.59–0.936). We performed the Delong test (Tables S7-S9) to determine whether the differences between the fusion models were statistically significant or not. We found that in training set, the P values of SVM4 in comparison with the other three models were all less than 0.05. Therefore, we concluded that the AUC of SVM_4 was significantly superior to that of fusion Model 1, 2, and 3 in the training set; meanwhile, for the QDA and LR classifiers, the differences between models were not significant. To perform a comprehensive comparison of the advantages and disadvantages of fusion models, we plotted calibration curves (Figure 5) and decision curves (Figure 6), which indicated that SVM_4 significantly outperformed the other fusion models in the training set. Comprehensive analysis of the above data suggested that among the four fusion models constructed by the SVM classifier, Model 4 had the highest efficacy in distinguishing between LBL and BL.
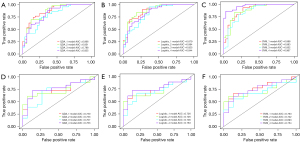
Table 3
Model name | Training group | Testing group | |||||||
---|---|---|---|---|---|---|---|---|---|
AUC (95% CI) | Sensitivity | Specificity | F1-score | AUC (95% CI) | Sensitivity | Specificity | F1-score | ||
QDA_1 | 0.866 (0.784–0.948) | 0.744 | 0.848 | 0.8 | 0.73 (0.55–0.909) | 0.556 | 0.933 | 0.69 | |
Logistic_1 | 0.879 (0.801–0.956) | 0.907 | 0.727 | 0.857 | 0.726 (0.547–0.905) | 0.556 | 0.933 | 0.69 | |
SVM_1 | 0.888 (0.812–0.964) | 0.93 | 0.727 | 0.869 | 0.763 (0.594–0.932) | 0.667 | 0.867 | 0.75 | |
QDA_2 | 0.851 (0.761–0.94) | 0.698 | 0.879 | 0.779 | 0.733 (0.554–0.913) | 0.611 | 0.933 | 0.733 | |
Logistic_2 | 0.884 (0.808–0.961) | 0.837 | 0.818 | 0.847 | 0.726 (0.545–0.907) | 0.611 | 0.933 | 0.733 | |
SVM_2 | 0.883 (0.806–0.96) | 0.837 | 0.848 | 0.857 | 0.722 (0.539–0.905) | 0.611 | 0.933 | 0.733 | |
QDA_3 | 0.788 (0.685–0.891) | 0.721 | 0.758 | 0.756 | 0.707 (0.523–0.892) | 0.722 | 0.733 | 0.743 | |
Logistic_3 | 0.825 (0.73–0.921) | 0.953 | 0.636 | 0.854 | 0.7 (0.515–0.885) | 0.667 | 0.8 | 0.727 | |
SVM_3 | 0.852 (0.761–0.943) | 0.884 | 0.697 | 0.835 | 0.693 (0.506–0.879) | 0.556 | 0.867 | 0.667 | |
QDA_4 | 0.825 (0.729–0.92) | 0.793 | 0.606 | 0.833 | 0.763 (0.59–0.935) | 0.722 | 0.867 | 0.788 | |
Logistic_4 | 0.844 (0.754–0.933) | 0.907 | 0.636 | 0.83 | 0.763 (0.59–0.936) | 0.722 | 0.867 | 0.788 | |
SVM_4 | 0.967 (0.935–0.998) | 0.86 | 0.97 | 0.913 | 0.754 (0.584–0.924) | 0.611 | 0.867 | 0.71 |
AUC, area under the curve; CI, confidence interval; QDA, quadratic discriminant analysis; SVM, support vector machine.
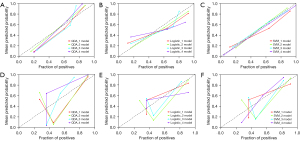
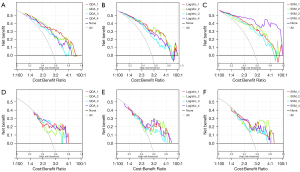
Discussion
Lymphomas are one of the most prevalent malignant tumors in children, most of which are NHL, with the most common subtypes being LBL and BL. Accurate diagnosis of subtypes is important in selecting the appropriate treatment plan. The most important approach for determining the pathological subtype of lymphoma is excisional biopsy; it is the precursor to appropriate diagnosis, but this test is not only invasive for pediatric patients but also difficult to achieve in cases of lymphoma located in the mediastinum (19), puncture biopsy can also identify tumor subtypes, but the accuracy of puncture biopsy is not high due to sampling errors. In clinical practice, the examination of imaging remains critical for patients with lymphoma. The most frequently used imaging modality is CT, and the most accurate imaging modality to determine clinical staging is PET/CT or PET-magnetic resonance imaging (20). However, regardless of the method, radiologists are unable to use images to identify the pathological subtype of lymphoma in patients. Radiomics is rapidly developing and has been used in a variety of tumor diagnostic and prognostic studies, demonstrating excellent predictive capabilities (21,22). Similarly, radiomics has a wide range of applications in lymphoma. Xu et al. (23) extracted the textural features of lymphoma on enhanced CT, using 5 feature selection methods and 9 classifiers to construct a total of 45 models to differentiate intrahepatic cholangiocarcinoma from hepatic lymphoma. Eertink et al. (24) reported that combining radiomic features in baseline PET/CT with MYC gene status could predict progression over the following two years in patients with aggressive B-cell lymphoma.
Previous studies have focused on enlarged lymph nodes, but in clinical practice, not all patients with lymphoma present with enlarged lymph nodes (11,25,26), and most enlarged lymph nodes are found incidentally, as the majority of patients are admitted for various other medical reasons. The spleen is the major lymphatic organ, and splenic involvement or primary lymphoma of the spleen may occur in patients with lymphoma (27). Choosing the spleen over enlarged lymph nodes as the region of interest has several advantages: first, the technology of automatic splenic segmentation is highly mature (28,29), is significantly more efficient than is manual delineation, and allows for the method of study to be more easily applied in the clinic. VB-Net was developed on the basis of the V-Net architecture, which is an encoder-decoder network with skip and residual connections (30). Second, patients with lymphoma often do not undergo lymphadenectomy, so when radiologists select multiple lymph nodes for segmentation they are often unable to confirm that the selected lymph nodes are enlarged because of lymphoma invasion (31). Moreover, on CT images, enlarged lymph nodes often appear as fused masses, making it difficult to determine the boundaries of a single lymph node, which undoubtedly makes it more challenging for radiologists to manually segment them. With the spleen, there is no such problem with segmentation.
The aim of this study was to demonstrate that splenic radiomic features can be used to identify common pathologic subtypes in pediatric patients. Although Enke et al. (15) demonstrated that radiomics features of the spleen are valuable in the diagnosis and subtype classification of lymphomas, the related research has only been conducted in adult patients; however, the distribution of subtypes differs between adult and pediatric patients, and research on pediatric patients is lacking. Therefore, in our study, three classifiers were used to model the features of different phases separately. A total of 21 models were built, and the AUC values, specificity, sensitivity, and F1-score of single models and fusion models were compared. This revealed that fusion models perform better, and the analysis of fusion models indicated no significant difference between the results of fusion models constructed by QDA and LR in the training set and test set. The training set of SVM_4 showed significantly better results compared with models, but the test set did not show a significant difference, which we believe was due to the small sample size of the test set.
Among the 10 features used to construct Model 4, there are 4 first-order statistics, 2 GLCM features, 2 GLSZM features, and 2 GLRLM features. First-order statistics quantify the intensity distribution of individual voxels through histogram analysis, such as mean, median, percentile, skewness, and entropy. However, the first-order features cannot describe the relative positional relationships between voxels, and the texture features are derived by calculating the relative positional relationships between voxels, which can better reflect the structural texture differences of the tissue in the tumor (32). The GLCM is the joint probability distribution of the simultaneous occurrence of two gray-scale pixels at a certain distance from each other (17); GLSZM reflects the number of times elements j and i are adjacent to each other in the image, and is a measure of the degree of gray scale inhomogeneity in the tumor (33); and GLRLM represents the information about the runs of pixels with the same gray value in a certain direction. First-order statistics and GLCM play roles in differentiating Borrmann type IV gastric cancer from primary gastric lymphoma (34), and GLCM and GLRLM can also help differentiate DLBCL from follicular lymphoma (35). Previous studies have indicated that radiomic features differ between lymphomas and other tumors or between different subtypes of lymphoma. Our study confirmed that such features from the spleen differed between patients with LBL and those with BL. This may be due to the fact that in normal spleens, the red pulp consists of a complex network of blood vessels while the white pulp consists of lymphoid tissue, whereas in the spleens of patients with lymphoma, the white pulp structures are infiltrated and compress the red pulp, causing changes in voxel values that are imperceptible to the naked eye on CT images (14,36). Such changes are different depending on the pathologic subtypes. Moreover, the majority of features selected for this model are wavelet-transformed features. Wavelet is a higher-order statistical method that places linear or radial wave matrix on images, helping to reveal valuable information in the lesion that is not visible to the naked eye.
There are some limitations to this study that should be noted. (I) We employed a retrospective design, which has problems of selection bias and the inability to strictly control the quality of images. (II) Lymphoma is a malignant disease which has a complex array subtypes, but in this study, the two subtypes most common in child patients were selected. (III) We used a limited sample size (IV) and a single-center design that lacked external validation. In the future, multicenter, prospective, and multimodal studies can be conducted to increase the sample size and number of features to build more reliable and convincing models. (V) In this study, spleen invasion was determined based on PET/CT reports, which might have led to differences in interpretation. Imaging protocols for patients were not available for this study, and it is possible that the type of software, doses of fluorodeoxyglucose, and image acquisition time could have varied between institutions.
Conclusions
In conclusion, the radiomic features of the spleen on enhanced CT can be used to predict pathologic subtypes in pediatric lymphoma patients. However, this study’s findings are not sufficient to support clinical application at this time due to the limitations mentioned above. In the future, we will increase the sample size, add different pathological subtypes, and conduct multicenter studies to continuously improve these models. It is hoped that spleen radiomic features can be used predict the subtypes more accurately in the future and be productivity employed role for the early clinical diagnosis and customization of treatment plans. Through this noninvasive and rapid method, more information is obtained from the images. We will continue to conduct research on the value of splenic radiomic features in staging prediction and the prognostic prediction in patients with pediatric lymphoma.
Acknowledgments
Funding: This study was supported by
Footnote
Reporting Checklist: The authors have completed the TRIPOD reporting checklist. Available at https://qims.amegroups.com/article/view/10.21037/qims-24-122/rc
Conflicts of Interest: All authors have completed the ICMJE uniform disclosure form (available at https://qims.amegroups.com/article/view/10.21037/qims-24-122/coif). F.W. is an employee of Shanghai United Imaging Intelligence Co., Ltd. The other authors have no conflicts of interest to declare.
Ethical Statement: The authors are accountable for all aspects of the work in ensuring that questions related to the accuracy or integrity of any part of the work are appropriately investigated and resolved. This study was conducted in accordance with the Declaration of Helsinki (as revised in 2013) and was approved by the Ethics Committee of the Children’s Hospital of Chongqing Medical University (issue No. 386[2023]; date: 2023.8.20). The requirement for individual consent in this retrospective analysis was waived.
Open Access Statement: This is an Open Access article distributed in accordance with the Creative Commons Attribution-NonCommercial-NoDerivs 4.0 International License (CC BY-NC-ND 4.0), which permits the non-commercial replication and distribution of the article with the strict proviso that no changes or edits are made and the original work is properly cited (including links to both the formal publication through the relevant DOI and the license). See: https://creativecommons.org/licenses/by-nc-nd/4.0/.
References
- Sandlund JT, Downing JR, Crist WM. Non-Hodgkin's lymphoma in childhood. N Engl J Med 1996;334:1238-48. [Crossref] [PubMed]
- Miller KD, Fidler-Benaoudia M, Keegan TH, Hipp HS, Jemal A, Siegel RL. Cancer statistics for adolescents and young adults, 2020. CA Cancer J Clin 2020;70:443-59. [Crossref] [PubMed]
- Belsky JA, Hochberg J, Giulino-Roth L. Diagnosis and management of Hodgkin lymphoma in children, adolescents, and young adults. Best Pract Res Clin Haematol 2023;36:101445. [Crossref] [PubMed]
- Li S, Young KH, Medeiros LJ. Diffuse large B-cell lymphoma. Pathology 2018;50:74-87. [Crossref] [PubMed]
- Sandlund JT, Martin MG. Non-Hodgkin lymphoma across the pediatric and adolescent and young adult age spectrum. Hematology Am Soc Hematol Educ Program 2016;2016:589-97. [Crossref] [PubMed]
- Temple WC, Mueller S, Hermiston ML, Burkhardt B. Diagnosis and management of lymphoblastic lymphoma in children, adolescents and young adults. Best Pract Res Clin Haematol 2023;36:101449. [Crossref] [PubMed]
- Barth M, Xavier AC, Armenian S, Audino AN, Blazin L, Bloom D, et al. Pediatric Aggressive Mature B-Cell Lymphomas, Version 3.2022, NCCN Clinical Practice Guidelines in Oncology. J Natl Compr Canc Netw 2022;20:1267-75. [Crossref] [PubMed]
- McCarten KM, Nadel HR, Shulkin BL, Cho SY. Imaging for diagnosis, staging and response assessment of Hodgkin lymphoma and non-Hodgkin lymphoma. Pediatr Radiol 2019;49:1545-64. [Crossref] [PubMed]
- Hamrick-Turner JE, Saif MF, Powers CI, Blumenthal BI, Royal SA, Iyer RV. Imaging of childhood non-Hodgkin lymphoma: assessment by histologic subtype. Radiographics 1994;14:11-28. [Crossref] [PubMed]
- Abramson SJ, Price AP. Imaging of pediatric lymphomas. Radiol Clin North Am 2008;46:313-38. ix. [Crossref] [PubMed]
- Rao L, Wang X, Zong Z, Chen Z, Shi X, Yi C, Zhang X, Yang Z. PET-CT for Evaluation of Spleen and Liver 18F-FDG Diffuse Uptake Without Lymph Node Enlargement in Lymphoma. Medicine (Baltimore) 2016;95:e3750. [Crossref] [PubMed]
- Littooij AS, Kwee TC, Barber I, Granata C, de Keizer B, Beek FJ, Hobbelink MG, Fijnheer R, Stoker J, Nievelstein RA. Accuracy of whole-body MRI in the assessment of splenic involvement in lymphoma. Acta Radiol 2016;57:142-51. [Crossref] [PubMed]
- de Jong PA, van Ufford HM, Baarslag HJ, de Haas MJ, Wittebol SH, Quekel LG, de Klerk JM CT. 18F-FDG PET for noninvasive detection of splenic involvement in patients with malignant lymphoma. AJR Am J Roentgenol 2009;192:745-53. [Crossref] [PubMed]
- Reinert CP, Hinterleitner C, Fritz J, Nikolaou K, Horger M. Diagnosis of diffuse spleen involvement in haematological malignancies using a spleen-to-liver attenuation ratio on contrast-enhanced CT images. Eur Radiol 2019;29:450-7. [Crossref] [PubMed]
- Enke JS, Moltz JH, D'Anastasi M, Kunz WG, Schmidt C, Maurus S, Mühlberg A, Katzmann A, Sühling M, Hahn H, Nörenberg D, Huber T. Radiomics Features of the Spleen as Surrogates for CT-Based Lymphoma Diagnosis and Subtype Differentiation. Cancers (Basel) 2022;14:713. [Crossref] [PubMed]
- Wang H, Chen X, He L. A narrative review of radiomics and deep learning advances in neuroblastoma: updates and challenges. Pediatr Radiol 2023;53:2742-55. [Crossref] [PubMed]
- Gillies RJ, Kinahan PE, Hricak H. Radiomics: Images Are More than Pictures, They Are Data. Radiology 2016;278:563-77. [Crossref] [PubMed]
- Wu J, Xia Y, Wang X, Wei Y, Liu A, Innanje A, Zheng M, Chen L, Shi J, Wang L, Zhan Y, Zhou XS, Xue Z, Shi F, Shen D. uRP: An integrated research platform for one-stop analysis of medical images. Front Radiol 2023;3:1153784. [Crossref] [PubMed]
- Bhatt VR, Mourya R, Shrestha R, Armitage JO. Primary mediastinal large B-cell lymphoma. Cancer Treat Rev 2015;41:476-85. [Crossref] [PubMed]
- Barrington SF, Mikhaeel NG, Kostakoglu L, Meignan M, Hutchings M, Müeller SP, Schwartz LH, Zucca E, Fisher RI, Trotman J, Hoekstra OS, Hicks RJ, O'Doherty MJ, Hustinx R, Biggi A, Cheson BD. Role of imaging in the staging and response assessment of lymphoma: consensus of the International Conference on Malignant Lymphomas Imaging Working Group. J Clin Oncol 2014;32:3048-58. Erratum in: J Clin Oncol 2016;34:2562. [Crossref] [PubMed]
- Yuan H, Xu X, Tu S, Chen B, Wei Y, Ma Y. The CT-based intratumoral and peritumoral machine learning radiomics analysis in predicting lymph node metastasis in rectal carcinoma. BMC Gastroenterol 2022;22:463. [Crossref] [PubMed]
- Chen X, Wang H, Huang K, Liu H, Ding H, Zhang L, Zhang T, Yu W, He L. CT-Based Radiomics Signature With Machine Learning Predicts MYCN Amplification in Pediatric Abdominal Neuroblastoma. Front Oncol 2021;11:687884. [Crossref] [PubMed]
- Xu H, Zou X, Zhao Y, Zhang T, Tang Y, Zheng A, Zhou X, Ma X. Differentiation of Intrahepatic Cholangiocarcinoma and Hepatic Lymphoma Based on Radiomics and Machine Learning in Contrast-Enhanced Computer Tomography. Technol Cancer Res Treat 2021;20:15330338211039125. [Crossref] [PubMed]
- Eertink JJ, Zwezerijnen GJC, Wiegers SE, Pieplenbosch S, Chamuleau MED, Lugtenburg PJ, de Jong D, Ylstra B, Mendeville M, Dührsen U, Hanoun C, Hüttmann A, Richter J, Klapper W, Jauw YWS, Hoekstra OS, de Vet HCW, Boellaard R, Zijlstra JM. Baseline radiomics features and MYC rearrangement status predict progression in aggressive B-cell lymphoma. Blood Adv 2023;7:214-23. [Crossref] [PubMed]
- Liu Y. Clinical significance of diffusely increased splenic uptake on FDG-PET. Nucl Med Commun 2009;30:763-9. [Crossref] [PubMed]
- Herrinton LJ, Liu L, Abramson O, Jaffe ES. The incidence of hepatosplenic T-cell lymphoma in a large managed care organization, with reference to anti-tumor necrosis factor therapy, Northern California, 2000-2006. Pharmacoepidemiol Drug Saf 2012;21:49-52. [Crossref] [PubMed]
- Brox A, Shustik C. Non-Hodgkin's lymphoma of the spleen. Leuk Lymphoma 1993;11:165-71. [Crossref] [PubMed]
- Xu Z, Gertz AL, Burke RP, Bansal N, Kang H, Landman BA, Abramson RG. Improving Spleen Volume Estimation Via Computer-assisted Segmentation on Clinically Acquired CT Scans. Acad Radiol 2016;23:1214-20. [Crossref] [PubMed]
- Humpire-Mamani GE, Bukala J, Scholten ET, Prokop M, van Ginneken B, Jacobs C. Fully Automatic Volume Measurement of the Spleen at CT Using Deep Learning. Radiol Artif Intell 2020;2:e190102. [Crossref] [PubMed]
- Shi F, Hu W, Wu J, Han M, Wang J, Zhang W, Zhou Q, Zhou J, Wei Y, Shao Y, Chen Y, Yu Y, Cao X, Zhan Y, Zhou XS, Gao Y, Shen D. Deep learning empowered volume delineation of whole-body organs-at-risk for accelerated radiotherapy. Nat Commun 2022;13:6566. [Crossref] [PubMed]
- Seidler M, Forghani B, Reinhold C, Pérez-Lara A, Romero-Sanchez G, Muthukrishnan N, Wichmann JL, Melki G, Yu E, Forghani R, Dual-Energy CT. Texture Analysis With Machine Learning for the Evaluation and Characterization of Cervical Lymphadenopathy. Comput Struct Biotechnol J 2019;17:1009-15. [Crossref] [PubMed]
- Rogers W, Thulasi Seetha S, Refaee TAG, Lieverse RIY, Granzier RWY, Ibrahim A, Keek SA, Sanduleanu S, Primakov SP, Beuque MPL, Marcus D, van der Wiel AMA, Zerka F, Oberije CJG, van Timmeren JE, Woodruff HC, Lambin P. Radiomics: from qualitative to quantitative imaging. Br J Radiol 2020;93:20190948. [Crossref] [PubMed]
- Lee HS, Oh JS, Park YS, Jang SJ, Choi IS, Ryu JS. Differentiating the grades of thymic epithelial tumor malignancy using textural features of intratumoral heterogeneity via (18)F-FDG PET/CT. Ann Nucl Med 2016;30:309-19. [Crossref] [PubMed]
- Ma Z, Fang M, Huang Y, He L, Chen X, Liang C, Huang X, Cheng Z, Dong D, Liang C, Xie J, Tian J, Liu Z. CT-based radiomics signature for differentiating Borrmann type IV gastric cancer from primary gastric lymphoma. Eur J Radiol 2017;91:142-7. [Crossref] [PubMed]
- Wu X, Sikiö M, Pertovaara H, Järvenpää R, Eskola H, Dastidar P, Kellokumpu-Lehtinen PL. Differentiation of Diffuse Large B-cell Lymphoma From Follicular Lymphoma Using Texture Analysis on Conventional MR Images at 3.0 Tesla. Acad Radiol 2016;23:696-703. [Crossref] [PubMed]
- Reinert CP, Kloth C, Fritz J, Nikolaou K, Horger M. Discriminatory CT-textural features in splenic infiltration of lymphoma versus splenomegaly in liver cirrhosis versus normal spleens in controls and evaluation of their role for longitudinal lymphoma monitoring. Eur J Radiol 2018;104:129-35. [Crossref] [PubMed]