Assessment of body compositions in the prediction of metabolic syndrome in adults
Introduction
Metabolic syndrome (MS) consists of several metabolic abnormalities, including elevated blood pressure, insulin resistance, a hypercoagulable state, dyslipidemia, and a proinflammatory state (1). Patients with MS have an increased risk of atherosclerotic cardiovascular disease and type 2 diabetes (2). The musculoskeletal system disorders may occur under MS conditions, such as insulin resistance and sarcopenia (3-5). Evidence suggests that body compositions play an important role in the pathophysiology of MS and are involved in fat oxidation in muscle and adipose tissue lipolysis (6). Research has also shown that inflammatory cytokines exert a significant influence on cardiovascular diseases in metabolic disorders (7).
Adipose tissue and skeletal muscle have been used as biomarkers of chronic metabolism diseases in research and clinical practice. In metabolism-related diseases, the assessment of regional fat distribution is more important than the body mass index (BMI) (8), or the total fat content (9). In MS, ectopic fat accumulates in the skeletal muscle and visceral organs, including the liver, heart, and visceral adipose tissue (VAT) (10). Muscle mass in the thigh has been shown to be an indicator of cardiovascular health–related metabolic disorders (11). Increased VAT is associated with a higher risk of cardiovascular disease (12). The BMI can reflect the obesity of the whole body but cannot be used to evaluate metabolic risks. Extramyocellular lipid (EMCL) between muscle bundles is thought to be an indicator of muscle metabolism (13) and muscle quality. Further, EMCL and muscle mass have been shown to be related to amyotrophy (14,15).
The waist circumference (WC) is related to the BMI and is thought to be a symbol of central obesity (16,17). However, even patients with a normal WC and BMI may suffer from metabolic disorders (8,18). There are many modalities for assessing body compositions, such as magnetic resonance imaging, computed tomography (CT) and dual-energy X-ray absorptiometry. CT has been used as the standard reference in body composition analyses according to different attenuation characteristics of the tissues and has been shown to have high resolution and efficiency (19,20).
In this study, we aimed to explore the association between metabolic disorders and body compositions in Asians, and determine whether body compositions can be used to detect metabolic disorders with different WCs in CT images. We present this article in accordance with the STROBE reporting checklist (available at https://qims.amegroups.com/article/view/10.21037/qims-23-1724/rc).
Methods
This single-center, retrospective study was conducted in accordance with the Declaration of Helsinki (as revised in 2013). The study was approved by the Institutional Review Board of the Tongji Hospital (TJ-IRB20210602), and the requirement of informed consent for this retrospective analysis was waived.
Subjects
Patients at Tongji Hospital who underwent CT examinations of lower extremities between June 2020 and July 2022 with a complete medical history and high-quality images were included in the study. To be eligible for inclusion in this study, the patients had to meet the following inclusion criterion: have careful medical recordings and have undergone complete systematic assessments [e.g., laboratory and anthropometric examinations, and blood pressure, plasma triglycerides, and high-density lipoprotein cholesterol (HDL-C) tests]. Patients were excluded from the study if they met any of the following exclusion criteria: had experienced a trauma or had undergone surgery in the abdomen or thigh (e.g., amputation and arthroplasty); had congenital muscular atrophy; had a chronic disease affecting muscle mass, such as tuberculosis, cancer, stroke; and/or had uncomplete medical records or poor-quality images. The study flowchart is displayed in Figure 1.
The subjects included in this study were mostly from the Internal Medicine Department of Tongji Hospital, and were suspected to have metabolic disorders, such as diabetes or dyslipidemia; however, some had attended the hospital to undergo health examinations. The patients included in the study were divided into the following four groups according to their WC and the presence of an MS: Group 1, which comprised healthy control patients with normal WCs and no MS risk factors; Group 2, which comprised patients with normal WCs and metabolic disorders (one or two metabolic risk factors: either diabetes, hypertension, or hyperlipidemia); Group 3, which comprised patients with normal WCs and MS; and Group 4, which comprised patients with larger WCs and MS.
MS diagnosis criteria
MS was diagnosed based on the International Diabetes Federation (IDF) criteria (21,22) as follows: an ethnicity specific WC (see below) or a BMI >30 kg/m2, plus ≥2 other of the following criteria: fasting plasma glucose ≥5.6 mmol/L (100 mg/dL) or a previous diagnosis of type 2 diabetes mellitus (an oral glucose tolerance test is also strongly recommended but not necessary); blood pressure ≥130/80 mm Hg, or treatment of previously diagnosed hypertension; plasma triglycerides ≥1.7 mmol/L (150 mg/dL), or specific treatment for hypertriglyceridemia; HDL-C of <1.03 mmol/L (40 mg/dL) for males or <1.29 mmol/L (50 mg/dL) for females, or specific treatment for low HDL-C. In relation to the ethnicity specific WC, the criteria were as follows: a WC ≥94 cm for male or ≥80 cm for female Europids; a WC ≥90 cm for male or ≥80 cm for female South Asians; a WC ≥90 cm for male or ≥80 cm for female Chinese; or a WC ≥85 cm for male or ≥90 cm for female Japanese.
Image analysis
The CT images were obtained using CT scanners manufactured by Toshiba (Toshiba Medical Systems, Otawara, Japan). The following parameters were used for the CT scans: tube voltage: 80 kvp; slice thickness: 1 mm; tube current: 145 mmA; and in-plane pixel range: 0.656×0.656–0.832×0.832 mm2.
Subcutaneous adipose tissue (SAT) was separated, fascia, bone, and vessels were excluded semi-automatically with manual corrections on the CT images. Adipose tissue distributed between the muscle bundles was defined as EMCL (23). Visceral fat was outlined by the abdominal muscular and viscera organs in the abdomen. The cross-sectional areas of the adipose (SAT, VAT, and EMCL) and skeletal muscles (abdomen: the sum of the abdominal oblique, the rectus abdominis, psoas muscles, and erector spinae; thigh: mainly the sum of the hamstrings and quadriceps femoris) were measured. The measurement of body compositions was based on the Hounsfield unit (Hu) (muscle: 50 to 80 Hu, SAT: −80 to −150 Hu, and EMCL: −60 to −150 Hu) at the mid-thigh and L4 vertebra level. In the abdomen, the cross-sectional areas of the muscle and adipose tissue were calculated in continuous slices at the L4 vertebrate level, and the sum of the measurements were divided by the slice number. The measurement of the mid-thigh was similar to that of the abdomen with the additional average of the left and right thighs. The cross-sectional area was divided by the height square to reduce the bias. The following ratios between the adipose and muscle were calculated: the SAT/muscle ratio (SMR), the VAT/muscle ratio (VMR), and the EMCL/muscle ratio (EMR). The HU values of the muscle in the thigh and abdomen in the CT images were also calculated.
The muscle, SAT, and EMCL were separated based on HU thresholds and anatomy. All the measurements were performed using ImageJ (version 1.53, National Institutes of Health, Bethesda, MD, USA) and FireVoxel software (Department of Radiology, New York University, USA). The tissue separation illustration is displayed in Figure 2.
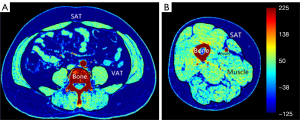
Statistical analysis
The statistical analysis was performed using SPSS (IBM, Chicago, USA). The chi-squared test and Kruskal-Wallis test were used to assess differences in the anthropological results, laboratory examinations, and body compositions among the groups. Post-hoc multiple comparisons were performed using the Kruskal-Wallis test and chi-squared test with Bonferroni correction.
A logistic regression analysis with MS and metabolic disorders as the dependent variables after the adjustment of clinical parameters (e.g., age and weight) was performed to examine the relationship between metabolic disorders and body compositions. The area under the curve (AUC) obtained from the receiver operating characteristic (ROC) curve analysis was calculated as a discriminative performance characteristic. The DeLong test was used to compare the AUCs among the different models. The two-sided (double-tailed) P value for significance was set at 0.05.
Results
Demographic and clinical characteristics
A total of 116 Asian patients (of whom 79 were male) were included in this study. The patients were allocated to the different groups according to WC and MS. Age (P=0.023), plasma triglycerides (P<0.001), hypertension (P<0.001), and diabetes history (P<0.001) differed significantly among the groups. However, no significant differences were observed in terms of sex, height, HDL-C, and smoking history among the different groups. The statistical results of the anthropological and laboratory parameters are set out in Table 1.
Table 1
Parameters | Group 1 | Group 2 | Group 3 | Group 4 | P |
---|---|---|---|---|---|
Sex (F, M) | 5, 17 | 12, 27 | 11, 25 | 9, 10 | 0.392 |
Age (years) | 50.76 (17.23) | 57.32 (15.48) | 63.2 (9.8) | 62.74 (14.39) | 0.023* |
Height (meters) | 1.65 (0.07) | 1.66 (0.10) | 1.65 (0.08) | 1.67 (0.08) | 0.619 |
Weight (kg) | 59.24 (14.72) | 64.53 (13.79) | 66.48 (12.22) | 75.19 (13.90) | 0.016* |
Systolic pressure (mmHg) | 122.77 (13.90) | 126.67 (20.77) | 139.8 (19.54) | 138.84 (27.06) | <0.001* |
Diastolic pressure (mmHg) | 80.23 (11.12) | 79.44 (13.56) | 80.89 (8.37) | 84.47 (11.98) | 0.001* |
Waist circumference (cm) | 79.53 (11.81) | 82.49 (9.22) | 87.69 (6.87) | 105.94 (6.68) | <0.001* |
HDL-C (mmol/L) | 1.10 (0.42) | 1.08 (0.33) | 0.95 (0.18) | 0.91 (0.27) | 0.157 |
Plasma triglycerides (mmol/L) | 1.25 (1.03) | 1.17 (0.80) | 2.16 (1.05) | 2.30 (1.22) | <0.001* |
Diabetes history | 0 (0%) | 35 (89.7%) | 27 (75%) | 14 (73.68%) | <0.001* |
Smoke history | 5 (22.7%) | 12 (30.8%) | 16 (44.4%) | 4 (21.1%) | 0.211 |
Hypertension history | 0 (0%) | 13 (33.3%) | 25 (69.4%) | 11 (57.9%) | <0.001* |
Data are presented as the n (%), or the median (interquartile range). *, there was a significant difference between groups, P<0.05. Group 1: healthy control subjects with a normal WC and non-MS risk factors. Group 2: subjects with a normal WC and metabolic disorders. Group 3: subjects with a normal WC and MS. Group 4: subjects with a larger WC and MS. F, female; M, male; HDL-C, high-density lipoprotein cholesterol; WC, waist circumference; MS, metabolic syndrome.
Evaluation of body compositions
There were differences among groups in the following body composition parameters: SAT (P=0.001), EMCL (P=0.04), and the SMR (P=0.004) in the thigh. SAT (P<0.001) and VAT (P=0.001), the SMR (P<0.001), and the VMR (P<0.001) were found statistically different between groups in the abdomen. The SMR (P<0.001) and VMR (P<0.001) were significantly different between groups. The HU of muscle in the thigh and abdomen were compared, and the mean (P=0.006 and 0.006) and median value (P=0.009 and 0.008) of the thigh and abdomen were significantly different between groups, and there was a significant difference in the standard deviation (SD) assessment between the groups (P=0.003 and 0.009). For further details, see Table 2 and Figures 3,4.
Table 2
Parameters (adjusted) | Group 1 | Group 2 | Group 3 | Group 4 | P |
---|---|---|---|---|---|
Thigh (cm2) | |||||
Total area | 5,715.59±1,272.61 | 5,526.90±1,269.66 | 5,890.79±986.87 | 6,816.67±1,076.04 | 0.005* |
Muscle | 3,572.79±848.31 | 3,672.61±935.83 | 3,830.87±865.28 | 3,864.34±694.97 | 0.612 |
SAT | 1,620.96±810.65 | 1,326.81±711.61 | 1,478.88±641.48 | 2,344.63±937.53 | 0.001* |
EMCL | 305.08±174.522 | 284.63±135.33 | 441.21±475.06 | 378.60±167.83 | 0.040* |
SMR | 0.465±0.23 | 0.379±0.22 | 0.420±0.27 | 0.630±0.31 | 0.004* |
EMR | 0.097±0.09 | 0.082±0.043 | 0.140±0.25 | 0.100±0.05 | 0.289 |
Mean | 62.36±7.92 | 57.15±8.64 | 57.83±8.02 | 43.11±16.77 | 0.006* |
Median | 65.56±7.45 | 58.67±8.32 | 59.9±8.05 | 50.27±9.41 | 0.009* |
SD | 21.70±5.49 | 23.24±4.14 | 23.26±3.87 | 27.59±5.94 | 0.003* |
Abdomen (cm2) | |||||
VAT | 2,775.72±2,173.01 | 3,055.03±2,501.85 | 4,022.32±1,792.23 | 5,525.74±2,703.07 | 0.001* |
SAT | 4,653.02±1,933.62 | 4,751.03±2,311.97 | 5,736.08±2,178.60 | 9,256.25±3,680.43 | <0.001* |
Muscle | 4,295.60±1,025.30 | 4,097.69±1,100.65 | 4,211.12±775.47 | 4,121.23±928.57 | 0.772 |
EMCL | 107.43±45.90 | 109.76±54.15 | 112.21±66.22 | 151.74±99.14 | 0.502 |
SVR | 2.75±1.84 | 2.62±2.06 | 2.02±2.71 | 2.52±2.39 | 0.137 |
SMR | 1.18±0.53 | 1.18±0.56 | 1.39±0.56 | 2.39±1.21 | <0.001* |
VMR | 1.13±0.65 | 1.51±1.24 | 1.92±0.92 | 2.74±1.54 | <0.001* |
EMR | 0.03±0.01 | 0.03±0.02 | 0.03±0.02 | 0.04±0.03 | 0.639 |
Mean | 58.85±10.87 | 54.10±9.26 | 51.10±9.00 | 36.98±16.08 | 0.006* |
Median | 59.88±11.00 | 55.6±8.61 | 52.80±8.75 | 40.09±14.64 | 0.008* |
SD | 19.42±3.97 | 17.98±4.53 | 19.81±3.21 | 25.58±7.42 | 0.009* |
Data are presented as the mean ± SD. *, there was a significant difference between groups, P<0.05. Group 1: healthy control subjects with a normal WC and non-MS risk factors. Group 2: subjects with a normal WC and metabolic disorders. Group 3: subjects with a normal WC and MS. Group 4: subjects with a larger WC and MS. SAT, subcutaneous adipose tissue; EMCL, extramyocellular lipid; SMR, SAT/muscle ratio; EMR, EMCL/muscle ratio; SD, standard deviation; VAT, visceral adipose tissue; SVR, SAT/VAT ratio; VMR, VAT/muscle ratio; WC, waist circumference; MS, metabolic syndrome.
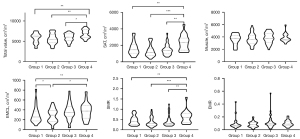
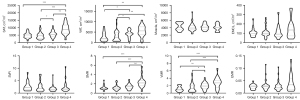
Regression analysis of body compositions and metabolic disorders
Using metabolic disorders as the independent variables, EMCL (AUC =0.790, sensitivity: 64.13%, specificity: 77.27%) and mean attenuation value of muscle (AUC =0.791, sensitivity: 86.54%, specificity: 77.78%) performed better than other body composition parameters in the thigh. The VMR (AUC =0.752, sensitivity: 73.02%, specificity: 75.00%) and SMR (AUC =0.746, sensitivity:75.28%, specificity: 75.00%) model was better than other parameters in the determination of metabolic disorders in the abdomen.
In terms of performance, the AUCs of the body composition model to diagnose MS in the thigh ranged from 0.681 to 0.768; the SAT and EMCL models performed better (0.768, 0.760) than others with sensitivity rates of 92.73% and 80%, and specificity rates of 57.63% of 69.49%, respectively. There was no difference in the performance of the body composition models in the thigh (P>0.05). The AUCs of the models ranged from 0.702 (SAT: sensitivity: 79.63%, specificity: 70.91%) to 0.788 (VAT: sensitivity: 64.81%, specificity: 80.00%) in the abdomen. The ability of the adipose and muscle parameters to diagnose MS in the abdomen were similar (P>0.05). The ability of the body composition models to diagnose MS after the adjustment of the clinical parameters are displayed in Tables 3,4 and Figure 5.
Table 3
Model and descriptors | AUC (95% CI) | Youden index | P | Sensitivity | Specificity | P |
---|---|---|---|---|---|---|
Thigh (cm2) | >0.05 | |||||
Total area | 0.742 (0.651–0.819) | 0.5267 | 0.0001* | 66.30% | 86.36% | |
SAT | 0.668 (0.574–0.754) | 0.3666 | 0.0126* | 68.48% | 68.18% | |
EMCL | 0.790 (0.597–0.873) | 0.4140 | 0.0041* | 64.13% | 77.27% | |
SMR | 0.666 (0.664–0.845) | 0.3478 | 0.0158* | 84.78% | 53.20% | |
Mean | 0.791 (0.667–0.884) | 0.6432 | 0.0014* | 86.54% | 77.78% | |
Median | 0.675 (0.650–0.872) | 0.5278 | 0.003* | 75.00% | 77.78% | |
SD | 0.637 (0.504–0.757) | 0.3667 | 0.2106 | 66.67% | 80.00% | |
Abdomen (cm2) | >0.05 | |||||
VAT | 0.732 (0.639–0.812) | 0.4416 | 0.0001* | 74.16% | 70.00% | |
SAT | 0.741 (0.648–0.820) | 0.4803 | 0.0001* | 73.03% | 75.00% | |
SMR | 0.746 (0.654–0.825) | 0.5000 | 0.0001* | 75.28% | 75.00% | |
VMR | 0.752 (0.660–0.829) | 0.4303 | 0.0001* | 73.03% | 70.00% | |
Mean | 0.703 (0.570–0.815) | 0.4755 | 0.0455* | 72.55% | 75.00% | |
Median | 0.733 (0.602–0.840) | 0.4559 | 0.0291* | 70.59% | 75.00% | |
SD | 0.717 (0.583–0.849) | 0.2157 | 0.0255* | 70.57% | 77.25% |
*, there was a significant difference between groups, P<0.05. Group 1: healthy control subjects with a normal WC and non-MS risk factors. Group 2: subjects with a normal WC and metabolic disorders. Group 3: subjects with a normal WC and MS. Group 4: subjects with a larger WC and MS. AUC, area under the curve; CI, confidence interval; SAT, subcutaneous adipose tissue; EMCL, extramyocellular lipid; SMR, SAT/muscle ratio; SD, standard deviation; VAT, visceral adipose tissue; VMR, VAT/muscle ratio; WC, waist circumference; MS, metabolic syndrome.
Table 4
Model and descriptors | AUC (95% CI) | Cut-off value | P | Sensitivity | Specificity | P |
---|---|---|---|---|---|---|
Thigh | >0.05 | |||||
Total area | 0.758 (0.673–0.837) | 0.3908 | 0.001* | 76.36% | 62.71% | |
SAT | 0.768 (0.680–0.842) | 0.5035 | 0.001* | 92.73% | 57.63% | |
EMCL | 0.760 (0.671–0.835) | 0.4949 | 0.001* | 80.00% | 69.49% | |
SMR | 0.754 (0.665–0.830) | 0.4598 | 0.001* | 78.18% | 67.80% | |
Mean | 0.709 (0.575–0.831) | 0.2198 | 0.138 | 87.50% | 34.48% | |
Median | 0.681 (0.534–0.862) | 0.1821 | 0.375 | 90.62% | 27.69% | |
SD | 0.696 (0.665–0.907) | 0.4680 | 0.005* | 81.25% | 65.52% | |
Abdomen | >0.05 | |||||
VAT | 0.775 (0.686–0.850) | 0.4340 | 0.0001* | 87.04% | 56.36% | |
SAT | 0.704 (0.617–0.874) | 0.4869 | 0.0001* | 77.78% | 70.91% | |
SMR | 0.702 (0.615–0.872) | 0.5050 | 0.0001* | 79.63% | 70.91% | |
VMR | 0.788 (0.686–0.850) | 0.4481 | 0.0001* | 64.81% | 80.00% | |
Mean | 0.763 (0.628–0.881) | 0.3113 | 0.0219* | 71.87% | 59.26% | |
Median | 0.756 (0.621–0.875) | 0.3223 | 0.0296* | 71.87% | 59.26% | |
SD | 0.766 (0.631–0.883) | 0.3783 | 0.0232* | 78.12% | 59.35% |
*, there was a significant difference between groups, P<0.05. Group 1: healthy control subjects with a normal WC and non-MS risk factors. Group 2: subjects with a normal WC and metabolic disorders. Group 3: subjects with a normal WC and MS. Group 4: subjects with a larger WC and MS. MS, metabolic syndrome; AUC, area under the curve; CI, confidence interval; SAT, subcutaneous adipose tissue; EMCL, extramyocellular lipid; SMR, SAT/muscle ratio; SD, standard deviation; VAT, visceral adipose tissue; VMR, VAT/muscle ratio; WC, waist circumference.
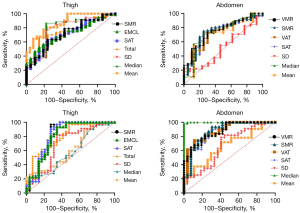
Discussion
Musculoskeletal system pathological changes (e.g., ectopic fat accumulation, amyotrophy) are associated with the metabolic risk factors (24). This study evaluated body compositions in the thigh and abdomen of CT images. Compared with the control group, more adipose tissue (SAT, VAT, and EMCL) was distributed in the patients with metabolic disorders, who also had a higher adipose tissue and muscle ratio. The accumulation of adipose tissue in the abdomen (SAT and VAT) is often reflected by a larger WC. The results of this study suggest that patients with more adipose tissue but a normal WC have higher metabolic risks. Thus, in addition to obesity, body compositions should also be considered in metabolic condition assessments. Body compositions play an important role in the pathophysiology of MS (e.g., oxidation stress, inflammatory cytokines, and adipokines). In the determination of metabolic disorders, the performance of the body composition model was similar in the thigh and the abdomen.
The findings of this study are consistent with those of previous studies, which have shown than MS subjects have more fat accumulation and muscle loss (1,6,11,25-28). In this study, the data suggested that in the abdomen, the SMR and VMR showed sensitivity in detecting metabolic disorders and MS. Conversely, in the thigh, EMCL and mean attenuation were able to detect metabolic disorders with higher sensitivity, while SAT and EMCL were able to detect to MS with higher sensitivity. EMCL accompanied by lower muscle attenuation reflected the degree of fat infiltration and lower muscle quality in the thigh, and had higher AUCs in MS and metabolic disorders. There were no differences between the body compositions of the thigh and abdomen in the diagnosis of metabolic disorders.
BMI reflects general obesity, while body compositions offer more metabolism information for subjects with a normal BMI, providing information about ectopic fat accumulation (SAT, VAT, and EMCL), and muscle atrophy. CT examinations have been used as a reliable and non-invasive tool in the diagnosis of musculoskeletal diseases, and allow for the detailed body composition assessment of adipose tissue and muscle (6). EMCL measurements based on CT can be used to assess increased cardiometabolic risks in chronic obstructive pulmonary disease patients (29). Measurements of myocardial, pericardial and perivascular adipose tissue by CT images can assist in the diagnosis of coronary artery diseases (30-32).
The growing prevalence of MS in the population has attracted attention worldwide. MS is associated with several risk factors, such as smoking, sex hormone deficiency, low-grade inflammation, and increased oxidative stress (2). A number of studies have emphasized the importance of VAT, pericardial and perirenal adipose tissue in metabolic disorders; however, few studies have focused on adipose tissue changes in muscle, especially EMCL, in metabolic disorders. In the musculoskeletal system, proinflammatory cytokines increase the rate of lipolysis of skeletal muscle in chronic metabolism conditions (6). Adipose and muscle dysfunction can aid in the diagnosis and prognosis of metabolic disorders in clinical practice (33). An inverse association has been reported between thigh muscle mass and MS (34), and the ratio between adipose tissue and muscle changes could be an indicator of the MS (35). Visceral fat has been shown to be correlated with early atherosclerotic changes in diabetes and MS (36).
This study had several limitations. The sample size of the study was small, which lowers the generalizability of the findings. Several factors, such as lifestyle and diet, may affect body composition metabolism, but these factors were not considered in this study. The subjects in the control group were from the same hospital, thus this study might be affected by selection bias. Further investigations with a large population and more comprehensive assessments are needed in the future.
Conclusions
The body compositions parameters of EMCL, VMR, VAT, and muscle quality could offer information and assist in detecting patients with an increased risk of MS. The ability of the body composition models to detect metabolic disorders was similar in the thigh and the abdomen. The results need to be confirmed in a larger population.
Acknowledgments
Funding: This study was supported by
Footnote
Reporting Checklist: The authors have completed the STROBE reporting checklist. Available at https://qims.amegroups.com/article/view/10.21037/qims-23-1724/rc
Conflicts of Interest: All authors have completed the ICMJE uniform disclosure form (available at https://qims.amegroups.com/article/view/10.21037/qims-23-1724/coif). The authors have no conflicts of interest to declare.
Ethical Statement: The authors are accountable for all aspects of the work, including ensuring that any questions related to the accuracy or integrity of any part of the work have been appropriately investigated and resolved. The study was conducted in accordance with the Declaration of Helsinki (as revised in 2013). The study was approved by the Institutional Review Board of the Tongji Hospital (TJ-IRB20210602), and the requirement of informed consent for this retrospective analysis was waived.
Open Access Statement: This is an Open Access article distributed in accordance with the Creative Commons Attribution-NonCommercial-NoDerivs 4.0 International License (CC BY-NC-ND 4.0), which permits the non-commercial replication and distribution of the article with the strict proviso that no changes or edits are made and the original work is properly cited (including links to both the formal publication through the relevant DOI and the license). See: https://creativecommons.org/licenses/by-nc-nd/4.0/.
References
- Dubé MC, Lemieux S, Piché ME, Corneau L, Bergeron J, Riou ME, Weisnagel SJ. Relationship of mid-thigh adiposity to the metabolic syndrome in postmenopausal women. Metab Syndr Relat Disord 2010;8:365-72. [Crossref] [PubMed]
- Huang PL. A comprehensive definition for metabolic syndrome. Dis Model Mech 2009;2:231-7. [Crossref] [PubMed]
- DeFronzo RA, Tripathy D. Skeletal muscle insulin resistance is the primary defect in type 2 diabetes. Diabetes Care 2009;32:S157-63. [Crossref] [PubMed]
- Hart DA, Scott A. Getting the dose right when prescribing exercise for connective tissue conditions: the Yin [corrected] and the Yang of tissue homeostasis. Br J Sports Med 2012;46:696-8. Erratum in: Br J Sports Med 2012;46:953.
- Weiss R, Bremer AA, Lustig RH. What is metabolic syndrome, and why are children getting it? Ann N Y Acad Sci 2013;1281:123-40. [Crossref] [PubMed]
- Lee TY, Jeon YJ, Kim CR, Kang BJ, Park GM. Abdominal Muscles and Metabolic Syndrome According to Patient Sex: A Retrospective Cross-Sectional Study. Healthcare (Basel) 2021;9:1197. [Crossref] [PubMed]
- Yun AJ, Lee PY. Maldaptation of the link between inflammation and bone turnover may be a key determinant of osteoporosis. Med Hypotheses 2004;63:532-7. [Crossref] [PubMed]
- Sureka B, George T, Garg MK, Banerjee M, Deora S, Sukhla R, Goel A, Garg PK, Yadav T, Khera PS. Cutoff values of body fat composition to predict metabolic risk factors with normal waist circumference in Asian Indian population. Eur Radiol 2023;33:711-9. [Crossref] [PubMed]
- Lamb HJ. Total body fat distribution as part of multiorgan MR imaging: new tool for risk assessment in the metabolic syndrome? Radiology 2010;257:307-8. [Crossref] [PubMed]
- Schumann T, König J, von Loeffelholz C, Vatner DF, Zhang D, Perry RJ, Bernier M, Chami J, Henke C, Kurzbach A, El-Agroudy NN, Willmes DM, Pesta D, de Cabo R. O Sullivan JF, Simon E, Shulman GI, Hamilton BS, Birkenfeld AL. Deletion of the diabetes candidate gene Slc16a13 in mice attenuates diet-induced ectopic lipid accumulation and insulin resistance. Commun Biol 2021;4:826. [Crossref] [PubMed]
- Londoño FJ, Calderón JC, Gallo J. Association between thigh muscle development and the metabolic syndrome in adults. Ann Nutr Metab 2012;61:41-6. [Crossref] [PubMed]
- Fox CS, Massaro JM, Hoffmann U, Pou KM, Maurovich-Horvat P, Liu CY, Vasan RS, Murabito JM, Meigs JB, Cupples LA, D'Agostino RB Sr, O'Donnell CJ. Abdominal visceral and subcutaneous adipose tissue compartments: association with metabolic risk factors in the Framingham Heart Study. Circulation 2007;116:39-48. [Crossref] [PubMed]
- Kim M, Oh JH, Won CW. Sex-Specific Differences in Lower Body Fat Distribution and Association with Physical Performance among Healthy Community-Dwelling Older Adults: A Pilot Study. Int J Environ Res Public Health 2022;19:4201. [Crossref] [PubMed]
- Yoshiko A, Maeda H, Takahashi H, Koike T, Tanaka N, Akima H. Importance of skeletal muscle lipid levels for muscle function and physical function in older individuals. Appl Physiol Nutr Metab 2022;47:649-58. [Crossref] [PubMed]
- Yu J, Wang M, Shen H. Intermuscular Fat, But Not Subcutaneous Fat, Correlated With Major Complications After Primary Total Hip Arthroplasty. Acad Radiol 2023;30:1367-73. [Crossref] [PubMed]
- Grundy SM, Neeland IJ, Turer AT, Vega GL. Waist circumference as measure of abdominal fat compartments. J Obes 2013;2013:454285. [Crossref] [PubMed]
- Pasanta D, Htun KT, Pan J, Tungjai M, Kaewjaeng S, Chancharunee S, Tima S, Kim HJ, Kæwkhao J, Kothan S. Waist Circumference and BMI Are Strongly Correlated with MRI-Derived Fat Compartments in Young Adults. Life (Basel) 2021;11:643. [Crossref] [PubMed]
- Goday A, Calvo E, Vázquez LA, Caveda E, Margallo T, Catalina-Romero C, Reviriego J. Prevalence and clinical characteristics of metabolically healthy obese individuals and other obese/non-obese metabolic phenotypes in a working population: results from the Icaria study. BMC Public Health 2016;16:248. [Crossref] [PubMed]
- Seeram E. Computed Tomography: A Technical Review. Radiol Technol 2018;89:279CT-302CT.
- Sugiyama Y, Ishizu Y, Ando Y, Yokoyama S, Yamamoto K, Ito T, Imai N, Nakamura M, Honda T, Kawashima H, Ishikawa T, Ishigami M. An improved method to assess skeletal muscle mass in patients with liver cirrhosis based on computed tomography images. Hepatol Res 2022;52:937-46. [Crossref] [PubMed]
- Alberti KG, Zimmet P, Shaw J. Metabolic syndrome--a new world-wide definition. A Consensus Statement from the International Diabetes Federation. Diabet Med 2006;23:469-80. [Crossref] [PubMed]
- Kazamel M, Stino AM, Smith AG. Metabolic syndrome and peripheral neuropathy. Muscle Nerve 2021;63:285-93. [Crossref] [PubMed]
- Mühlberg A, Museyko O, Bousson V, Pottecher P, Laredo JD, Engelke K. Three-dimensional Distribution of Muscle and Adipose Tissue of the Thigh at CT: Association with Acute Hip Fracture. Radiology 2019;290:426-34. [Crossref] [PubMed]
- Alabdali LAS, Jaeken J, Dinant GJ, van den Akker M, Winkens B, Ottenheijm RPG. Prevalence of Upper Extremity Musculoskeletal Disorders in Patients with Type 2 Diabetes in General Practice. Medicines (Basel) 2021;8:8. [Crossref] [PubMed]
- Collins KH, Herzog W, MacDonald GZ, Reimer RA, Rios JL, Smith IC, Zernicke RF, Hart DA. Obesity, Metabolic Syndrome, and Musculoskeletal Disease: Common Inflammatory Pathways Suggest a Central Role for Loss of Muscle Integrity. Front Physiol 2018;9:112. [Crossref] [PubMed]
- Tanaka M, Okada H, Hashimoto Y, Kumagai M, Nishimura H, Fukui M. Trunk muscle quality and quantity predict the development of metabolic syndrome and the increase in the number of its components in individuals without metabolic syndrome. Nutr Metab Cardiovasc Dis 2020;30:1161-8. [Crossref] [PubMed]
- Tanaka M, Okada H, Hashimoto Y, Kumagai M, Nishimura H, Oda Y, Fukui M. Relationship between metabolic syndrome and trunk muscle quality as well as quantity evaluated by computed tomography. Clin Nutr 2020;39:1818-25. [Crossref] [PubMed]
- Liu D, Zhong J, Ruan Y, Zhang Z, Sun J, Chen H. The association between fat-to-muscle ratio and metabolic disorders in type 2 diabetes. Diabetol Metab Syndr 2021;13:129. [Crossref] [PubMed]
- Vivodtzev I, Moncharmont L, Tamisier R, Borel JC, Arbib F, Wuyam B, Lévy P, Maltais F, Ferretti G, Pépin JL. Quadriceps muscle fat infiltration is associated with cardiometabolic risk in COPD. Clin Physiol Funct Imaging 2018;38:788-97. [Crossref] [PubMed]
- Ichikawa Y, Kitagawa K, Chino S, Ishida M, Matsuoka K, Tanigawa T, Nakamura T, Hirano T, Takeda K, Sakuma H. Adipose tissue detected by multislice computed tomography in patients after myocardial infarction. JACC Cardiovasc Imaging 2009;2:548-55. [Crossref] [PubMed]
- McClain J, Hsu F, Brown E, Burke G, Carr J, Harris T, Kritchevsky S, Szklo M, Tracy R, Ding J. Pericardial adipose tissue and coronary artery calcification in the Multi-ethnic Study of Atherosclerosis (MESA). Obesity (Silver Spring) 2013;21:1056-63. [Crossref] [PubMed]
- Antonopoulos AS, Sanna F, Sabharwal N, Thomas S, Oikonomou EK, Herdman L, et al. Detecting human coronary inflammation by imaging perivascular fat. Sci Transl Med 2017;9:eaal2658. [Crossref] [PubMed]
- Linge J, Petersson M, Forsgren MF, Sanyal AJ, Dahlqvist Leinhard O. Adverse muscle composition predicts all-cause mortality in the UK Biobank imaging study. J Cachexia Sarcopenia Muscle 2021;12:1513-26. [Crossref] [PubMed]
- Eastwood SV, Tillin T, Wright A, Mayet J, Godsland I, Forouhi NG, Whincup P, Hughes AD, Chaturvedi N. Thigh fat and muscle each contribute to excess cardiometabolic risk in South Asians, independent of visceral adipose tissue. Obesity (Silver Spring) 2014;22:2071-9. [Crossref] [PubMed]
- Wang Q, Zheng D, Liu J, Fang L, Li Q. Skeletal muscle mass to visceral fat area ratio is an important determinant associated with type 2 diabetes and metabolic syndrome. Diabetes Metab Syndr Obes 2019;12:1399-407. [Crossref] [PubMed]
- Reijrink M, de Boer SA, Spoor DS, Lefrandt JD, Lambers Heerspink HJ, Boellaard R, Greuter MJ, Borra RJH, Hillebrands JL, Slart RHJA, Mulder DJ. Visceral adipose tissue volume is associated with premature atherosclerosis in early type 2 diabetes mellitus independent of traditional risk factors. Atherosclerosis 2019;290:87-93. [Crossref] [PubMed]