Imaging diagnostics of pulmonary ground-glass nodules: a narrative review with current status and future directions
Introduction
The increase in individuals’ health consciousness, the widespread use of high-resolution computed tomography (CT), and the implementation of low-dose chest CT screening programs, particularly in the wake of the coronavirus disease 2019 (COVID-19) pandemic, have led to a surge in the use of chest CT scanning (1). Consequently, the detection rate of ground-glass nodules (GGNs) has improved significantly. GGNs are a social health problem that requires immediate attention. The early and precise diagnosis of GGNs represents a significant step in preventing excessive treatment and alleviating patients’ distress.
A GGN is a nodule with an increased density that does not obstruct lung tissue, bronchi, or blood vessels. GGNs are categorized as either pure GGNs (pGGNs) or mixed GGN (mGGNs), depending on the presence of solid components (2). In 2021, the World Health Organization reclassified lung cancers, designating atypical adenomatous hyperplasia (AAH) and adenocarcinoma in situ (AIS) as precursor glandular lesions (PGLs), and lung adenocarcinoma (LUAD) as primarily comprising micro-invasive adenocarcinoma (MIA) and invasive adenocarcinoma (IAC) (3). No matter how they are categorized, research has shown that GGNs may reflect the pathological development of lung tumors (4).
At present, the diagnosis of benign or malignant pulmonary GGNs primarily hinges on the CT characteristics and the experience of radiologists (5). Radiologists typically use chest CT morphologic and quantitative characteristics to evaluate the invasiveness of GGNs. The CT characteristics mainly include the location, size, margin, CT parameters, such as the mean CT value, consolidation tumor ratio (CTR), and volume doubling time (VDT), and all kinds of signs, such as the vacuole sign, air bronchus sign, vascular-related signs, and pleural depression sign. However, it is sometimes still difficult to distinguish between benign and malignant nodules (5).
For pulmonary nodules that are suspected to be malignant, CT-guided lung biopsy is a reliable procedure that has a 90% sensitivity for the diagnosis of lung cancer (6,7). The morphological dynamics of malignant GGN progression, along with its intricate growth characteristics and potential malignancy, pose challenges in its clinical management. Artificial intelligence (AI)-based diagnostic tools have the potential to increase early disease identification and expedite the interpretation of complicated images, all of which will benefit patients in the long term (8). Developments in AI technology have the potential to direct GGN screening and treatment strategies, and enhance image quality and the precision of GGN identification in clinical practice (9).
In this article, we examine the common signs of GGNs to elucidate the relationship between these signs and the identification of benign and malignant GGNs. We then describe the application of AI to image segmentation, automatic classification, and the invasiveness prediction of GGNs over the last three years, including its limitations and outlook. Finally, we discuss the necessity of biopsy of persistent pGGNs to better inform clinical diagnosis and treatment decision making. We present this article in accordance with the Narrative Review reporting checklist (available at https://qims.amegroups.com/article/view/10.21037/qims-24-674/rc).
Methods
We used PubMed, Elsevier ScienceDirect, Springer database, and Google Scholar to search for articles using specific keywords (Table 1). Once the search was completed, and duplicate articles were removed, we reviewed the abstracts and content of the remaining articles to ensure that they were relevant to this study. The research findings from the identified publications were then compiled, combined, and discussed in the text as necessary (Figure S1). We also gathered, examined, and interpreted pertinent imaging data from the Second Affiliated Hospital of Nanchang University’s Imaging Center. We used Adobe Illustrator 2020 to draw, resize, place, and resolve the figures.
Table 1
Items | Specification |
---|---|
Dates of searches | October 10, 2023, and March 15, 2024 |
Databases and other sources searched | PubMed; Elsevier ScienceDirect; Springer Database; Google Scholar |
Search terms used | Lung ground-glass nodule; Ground-glass nodule; Ground-glass opacity; Diagnostic imaging; Artificial intelligence; Lung biopsy; CT-guided biopsy |
Timeframe | From January 2000 to March 2024 |
Inclusion and exclusion criteria | Inclusion criteria: articles concerning patients with ground-glass nodules |
Exclusion criteria: articles not relevant to the purpose of this review | |
Selection process | All authors engaged in the selection process. A voting minority-majority strategy was used if uncertainties arose as to the relevance of any articles |
Identification of benign and malignant GGN imaging signs
Nodule location
An upper lobe distribution is linked to a higher risk of malignant tumors with an odds ratio of 1.9 (10), and the location of the GGN plays a role in determining the malignant risk (Figure 1). Lung cancer screening research has shown that the right upper lobe of the lung has the highest incidence of lung cancer, and the lesion is normally dispersed along the bronchovascular bundle (11). This might be because during breathing, the right upper lobe’s bronchus receives the greatest amount of airflow (11). LUADs in particular are frequently found in the periphery or sub-pleura of the lungs, and rarely appear in the central or central third of the lungs (11). This is not the case with squamous lung carcinomas, which can be found either peripherally or attached to the pleura and are also commonly found in the central or central third of the lungs (11).
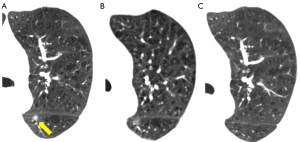
Nodule size
The size of the nodule is a crucial parameter in evaluating GGN features. The 2018 Chinese expert consensus defines tiny nodules as pulmonary nodules with a diameter of less than 5 mm, and small nodules as those with a diameter between 5 and 10 mm. According to the Fleischner guidelines (2), patient follow up is not required for a single pGGN or mGGN less than 6 mm in diameter; however, for a solitary pGGN more than 6 mm in diameter, a CT examination should be performed to confirm persistence in 6 to 12 months, and then every 2 years thereafter for 5 years (Figures 2,3), while for a solitary mGGN more than 6 mm in diameter, a CT examination should be performed to confirm persistence in 3 to 6 months, and then every year for 5 years if it is unaltered and the solid component is less than 6 mm (Figure 4). A persistent mGGN containing a solid component of more than 6mm is highly suspicious.
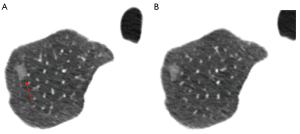
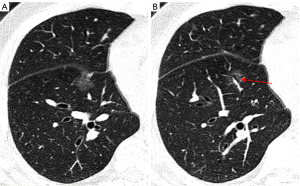
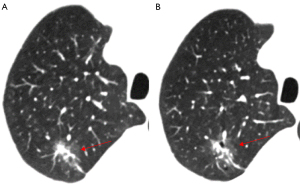
Lee et al. (12) conducted a retrospective analysis of 253 cases of pathologically confirmed GGNs using independent sample t-tests and a logistic regression analysis to examine the relationship between lesion size and invasiveness. Another study (13) made use of a Kaplan-Meier analysis, log-rank test, and Cox proportional hazards model to analyze the factors affecting GGN growth in 125 patients. Both studies reported that the optimal diameter cut-off value for distinguishing invasive GGNs was 10 mm. For a GGN more than 10 mm in diameter that persists for more than 3 months, the likelihood that malignant tumors will develop ranges from 10% to 50% (14). Generally, the diameter of an IAC is notably larger than that of a MIA, AIS, or AAH. As the diameter increases, the probability of a GGN being a MIA or IAC also increases (15). A thorough evaluation of the GGN size has the potential to enhance the detection of cancerous tumors. Nevertheless, reliance solely on size for identification is inadequate (Figures 5,6). A multivariate analysis showed that GGN size does not independently predict malignancy, and predictive models including size do not demonstrate optimal performance (16). Therefore, other CT features should be taken into account when making predictions about the benign or malignant nature of GGNs.
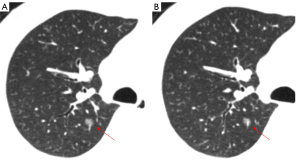
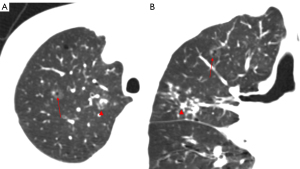
Mean CT value
The ranges of CT values for different neoplastic GGN subtypes frequently overlap, but research has shown that there is a statistically significant difference between the mean CT values of the subtypes (17). This difference is thought to be an independent predictor of the invasiveness of GGNs (17). In non-invasive lung cancer, the mean CT value was (−643.6±9.4) Hounsfield unit (HU) (Figures 2,3), but in invasive lung cancer, it was (−365.9±11.4) HU (P<0.0001) (18). Xiong et al. (19) conducted a study of 198 patients with a surgically proven diagnosis of MIA or IAC, who underwent multi-slice CT scans using scanners from various manufacturers (i.e., the Siemens SOMATOM Perspective or Emotion 16, General Electric Optima CT660, Discovery CT750 HD, Revolution CT or LightSpeed 16, and Philips Brilliance 16 P) with the following specific scanning parameters: tube voltage: 120 kV; tube current: 170–200 mA; slice thickness: 1.00–1.50 mm; matrix: 512×512; and rotation time: 0.5–0.6 s. The study revealed mean CT values for MIA and ICA of −701.16±61.68 and −612.72±72.48 HU, respectively, and maximum cross-sectional mean CT values for MIA and IAC of −687.60±66.17 and −598.59±83.32 HU, respectively (P<0.001). The results indicated that the magnitude of the maximum cross-sectional mean CT value might indicate the extent of tumor cell growth along the alveolar septum.
In recent years, mean CT values have been used in research to evaluate the development of GGNs. He et al. (20) proposed that GGNs with a mean CT value ≥−507.8 HU were more likely to exhibit growth. The mean CT threshold for predicting GGN progression was determined by Eguchi et al. (21) and Tamura et al. (22), who reported values of −670 and −677 HU, respectively. For pGGNs specifically, the mean CT values were found to be (−659.5±58.7) HU during the growth phase, and (−711.2±54.3) HU (20) during the non-growth phase. The mean CT value is not yet accurate enough to distinguish between different forms of GGN; however, it is an effective instrument for diagnosing malignant GGNs and provides important diagnostic information for patients with lung cancer (17).
Halo sign and reverse halo sign
The term “halo sign” refers to the ground-glass attenuation surrounding pulmonary nodules, which can be observed in a range of lung conditions (Figure 7). The mGGN may present a slight resemblance to the halo sign, while the boundary of the halo sign is usually blurred. In addition, mGGN-like nodules with halo signs show changes in lesion size, shape, and density during short-term follow up. Persistent mGGNs show a clear boundary and no change in lesion size, shape, and density during short-term follow up. The halo sign, which is mainly associated with lesion hemorrhage, is thought to be a significant indicator of pulmonary aspergillosis in the early stages of CT imaging in patients with impaired or deficient immune systems (23). Moreover, non-hemorrhagic inflammatory lesions may also exhibit “halo sign”. For instance, patients suffering from visceral larva migrans caused by Ascaris suum may have tiny nodules with a halo of ground-glass attenuation and focal areas of ground-glass attenuation in the lung periphery on CT scans representing lung involvement (24). These abnormalities correspond pathologically to necrosis surrounded by infiltrating inflammatory cells and the infiltration of marked eosinophils into the alveolar septa (24,25). In individuals with normal immune function, AIS is the most frequent cause of the “halo sign”, but it is uncommon in cases of lung squamous cell carcinoma (26). One of the most important factors in differentiating between benign and malignant lesions is the transparency of the interface between the ground-glass opacity (GGO) and the solid components in the nodule or the adjacent lung fields.
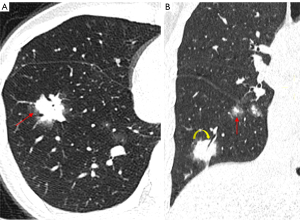
The reverse halo sign was initially identified in cases of cryptogenic organizing pneumonia (Figure 8). It appears as a core GGO surrounded by a periphery annular or crescent-shaped consolidation shadow on CT. It lacks specificity and can be present in both benign and malignant nodules. Additionally, it has been observed that the halo sign and the reverse halo sign can transition into each other, as evidenced by a gradual reversal process from the reverse halo sign to the halo sign in a follow-up case of LUAD (27).
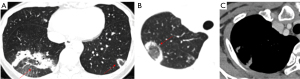
In tuberculosis patients, the reverse halo sign is typically observed in the right upper lobe. In contrast to non-organizing pneumonia, the presence of nodule walls or granuloma-like micronodules with the reverse halo sign strongly indicates active pulmonary tuberculosis (28). Mucormycosis, a vaso-invasive fungal infection, presents with non-specific clinical signs, making it challenging to differentiate from aspergillosis, which often leads to delayed diagnosis. The halo sign and reverse halo sign may assist in distinguishing between the two conditions (29,30). The reverse halo sign shows dynamic evolution in leukemia patients with pulmonary mucormycosis; it typically appears in 94% of chest CT scans within the first five days; however, this figure drops to 64% between days six and 14, and vanishes after the 14th day (31). Thus, the reverse halo sign represents a specific imaging feature and is thought to be a crucial signal of imaging presentations in hematological disorders complicated by pulmonary mucormycosis. Conversely, the halo sign strongly indicates invasive pulmonary aspergillosis. The presence of a reticular structure with an outer margin bigger than 1 cm with the reverse halo sign strongly suggests invasive mucormycosis in immunocompromised patients (32).
The reverse halo sign is also present in cases of pulmonary embolism. In patients with pulmonary thromboembolism, the reverse halo sign is often detected in the lower lobes of the lungs and is associated with acute thromboembolism in the corresponding segmental and subsegmental pulmonary arteries (33). A study of 62 patients with septic pulmonary embolism resulting from intravenous substance use disorder found that reverse halo signs were observed in 37 patients with no specific lobar preference (34). Further, episodes of septic pulmonary embolism may exhibit isolated consolidation and GGO with the reverse halo sign (34).
Following radiofrequency ablation for lung cancer, a reverse halo sign resembling a “bird’s nest” may be observed. This phenomenon is primarily attributed to the coagulative necrosis of cells resulting from the ablation process (35). Similarly, it may also be observed after radiotherapy for breast cancer, and is linked to the development of organizing pneumonia induced by radiotherapy. Radiation-induced coagulative necrosis disrupts the permeability of the basement membrane, triggering coagulation and fibrin accumulation in the alveoli (36).
CTR
The CTR is the ratio of the largest solid component’s diameter to the overall tumor’s largest diameter in the lung window of a high-resolution CT scan. Based on the number of solid components present, pulmonary GGNs can be divided into the following two categories using CTR values: pGGNs, which have a CTR value of 0 (Figure 5); and mGGNs, which have a CTR value between 0 and 1 (Figure 9). Previous studies have shown that the probability of unfavorable pathology types increases as the solid component of a nodule expands (37). A CTR value of 0.5 is considered the critical value for aggression in GGNs. This value is typically used to separate GGNs into GGO-dominant nodules (CTR ≤0.5) and solid-dominant nodules (0.5< CTR <1). According to a survival analysis, patients with GGO-dominant nodules have a considerably greater recurrence-free survival rate than those with solid-dominant GGNs (38). Additionally, a CTR value of 0.53 has been identified as the ideal cut-off value for calculating recurrence-free survival (38).
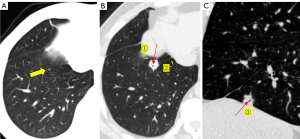
The CTR value also has significant diagnostic value in determining the presence of lymph node metastasis, as well as the necessity and extent of lymph node dissection in peripheral lung cancer (39). Individuals who have a high CTR value, especially one greater than 0.62, have a higher risk of lymph node metastasis (40). Interestingly, the CTR has a predictive potential comparable to positron emission tomography/CT in predicting regional lymph node metastasis before surgery [receiver operating characteristic (ROC) of maximum standardized uptake value: 0.816, ROC of CTR: 0.817] (40). Tsai et al. (41) conducted an analysis of 352 patients with LUAD who underwent standard lobectomy and lymph node dissection, and found that for patients with a maximum tumor diameter <1 cm, serum carcinoembryonic antigen (CEA) <5 ng/mL, and a CTR value <0.5, lymph node dissection may not be required. Several studies further indicated that lymph node dissection may be unnecessary when the CTR value is <0.5 (42,43). However, the clinical applicability of this conclusion requires validation through further prospective studies to enhance the rigor and standardization of lung cancer diagnosis and treatment.
Vacuole sign
Vacuoles are translucent regions in lesions of low-density gas that are less than 5 mm long (Figures 4-6). In cases in which LUAD presents as a GGN, the tumor cells obstruct the alveoli, leading to the formation of vacuoles and preventing the discharge of gas, ultimately resulting in alveolar rupture. As the tumor nodules are shrinking, the vacuoles can continue expanding. As the nodules progress, the vacuoles are filled with tumor cells and disappear. Vacuole growth position is a diagnostic marker that can be used to differentiate between benign and cancerous tumors. Malignant lesions are mainly dispersed throughout the lung’s periphery, while benign lesions are mainly found close to the heart side of the lung. The vacuole sign, which is rare in benign nodules and frequently used to determine the risk of GGN, is a significant characteristic of malignant pulmonary nodules. The presence of vacuoles also implies a faster pace of lesion growth, and research has shown that vacuoles are one of the variables that stimulate the growth of pulmonary nodules (44).
Air bronchus sign
The air bronchus sign is characterized by the presence of air-filled small bronchial shadows in a lesion, and it can be categorized into the following four types: type I, which resembles a green branch and is typically associated with pneumonia (Figures 3,7,10); type II, which is characterized by primarily dilated air-filled bronchial lumens (Figure 11); type III, which is distinguished by narrow and dilated bronchial lumens, irregular tube walls, and incomplete bronchial tree (Figure 9); and type IV, which is a mixed type that features both the bronchial mucus sign and air bronchus sign. However, these types lack distinguishing differences and are not precisely associated with specific diseases.
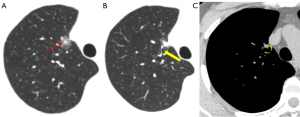
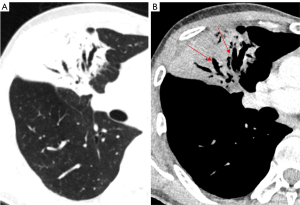
Benign lesions typically exhibit a normal or dilated bronchial lumen, while malignant bronchi are often narrow, truncated, occluded, or twisted. Further, the length of the bronchi affected by the air bronchus sign, the length of the diseased bronchi, and the ratio between these lengths are valuable in distinguishing between benign and malignant lesions (45). Marchiori et al. (46) proposed that the presence of an air bronchogram in conjunction with bronchiectasis can aid in the diagnosis of pulmonary lymphoma. The abnormal bronchial sign in pGGNs has been studied in the pathological categorization of LUAD and has been employed as a predictor of LUAD grade in certain studies (47). The air bronchogram sign, like the vacuole sign, is believed to be strongly correlated with the development of GGNs (44).
Vascular-related signs
Tumors and the circulatory system are closely related to malignant growths that frequently display altered blood flow. Based on imaging features, there are four types of associations between GGNs and blood vessels (Figure 12): type I: the blood vessels are adjacent to the lesion but there is no vascular supply inside the lesion (Figure 2); type II: the blood vessels pass through the lesion without undergoing significant morphological changes (Figures 3,5,6); type III: the blood vessels are bent or rigid without proliferation; and type IV: the blood vessels in the lesion exhibit a variety of shapes and intricate relationships (Figures 4,10,13), such as irregular dilation and the traction of blood vessels (48). On contrast-enhanced CT scans of the lungs, angiographic signals are described by magnified blood vessel pictures in lesions. These affected blood vessels tend to occur in malignant lesions, such as malignant lymphoma and diffuse lung carcinoma, where they frequently demonstrate signs of deformation, truncation, and constriction. The encapsulation of pulmonary veins by nodules indicates an elevated likelihood of lung cancer. As the nourishing small blood vessels penetrate the GGNs, they present as various CT features, including confluence, penetration, truncation, rigidity, and traction, which are collectively known as microvascular signs or tumor microvascular imaging signs. These signs are considered common indicators of early-stage lung cancer, particularly in peripheral lung cancer.
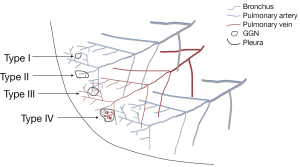
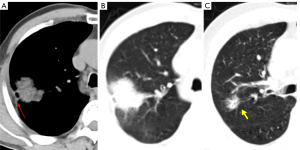
It is widely contended that in cross-sectional thin-section scanning, it might occasionally be difficult to identify the presence of microvessels surrounding the lesion. Novel modes of CT image post-processing, such as maximum density projection, multi-plane image reconstruction, and volume rendering, allow for multi-plane and multi-angle imaging observation, thereby enhancing the accuracy of the imaging diagnosis of early-stage lung cancer. In addition, the vascular bundle sign, which is primarily observed in peripheral lung cancer, is a feature in which nearby blood vessels congregate around the nodule without directly contacting or abutting the nodule’s edge.
Margin features
The uneven lobular contour at the periphery of the nodule is known as the lobulation sign, which is a regularly detected hallmark of peripheral lung cancer (Figure 9). Lobules can be shallow, moderate, or deep in degree, and their presence in the nodule is linked to differences in growth rate or obstruction by neighboring structures (Figures 2-4). Lobulation is a common mark of invasiveness; peripheral lung cancer with deep lobulation shows a higher level of malignant biological behavior than those lacking this trait (49). A unique type of lobulated modification, akin to a notch, is commonly observed in cancerous nodules (50). A radiological signal that falls between lobulation and spiculation is the spinous protuberant sign (Figure 4). It appears as a thick, blunt, club-like structure denoting the lesion’s infiltrative expansion toward the edge. A feature known as the pseudopod sign occurs when the spinous protrusions vary in thickness and length, resembling crab legs. This signal can assist in discriminating between benign and malignant nodules, as it is much more common in the latter. The spicule sign, observed on CT scans, presents as small spinous protrusions at the margin of a nodule, typically displaying consistent thickness (Figure 9). Cancerous nodule spicules are generally indicative of infiltrative growth, exudation, or proliferative stromal reaction in the lesion, often appearing as short and fine spicules. Conversely, benign nodule spicules, which are typically longer and softer in form, are often made of proliferating fibrous connective tissue and can be seen in inflammatory pseudotumors and tuberculosis.
Tumorous GGNs are recognized by their distinctive development patterns, which result in unique margins on imaging. These GGNs often have non-smooth margins and are more likely to have irregular, lobulated, serrated, or spiculated features. Conversely, benign non-inflammatory GGNs frequently feature sharper, smoother angles and crisper margins and may have fibrous strands. Moreover, inflammatory lesion boundaries often appear indistinct (51).
Pleural depression sign
A linear or cone-shaped shadow that is situated between the lesion and the pleura is indicative of pleural depression. Usually, this occurrence is explained by the reactive growth of peritumoral fibrous tissue, which results in the pleura contracting and creating a fluid-filled space between the parietal and visceral pleura. A depressed pleura without any visible thickening is frequently seen on the lung window in LUAD. Conversely, benign lesions, such as sclerosing hemangioma, lung abscess, inflammatory pseudotumor, and tuberculosis, might show markedly thicker pleura of increased density that may be visible in the lung and mediastinal windows. Pleural depression can be classified into the following five categories based on the relationship between the GGNs and the pleura (Figure 14): type a: the tumor’s edge attaches to the pleura without causing any deformation (Figures 1B,9); type b: a strip or line extends from the distal end of the pleura to the pleural surface with pleural folding (Figures 4,9); type c: the tumor displays pleural vegetation without folding (Figure 9); type d: the tumor wraps around the pleura causing retraction (Figures 3,10); and type e: the pleura is invaginated toward the tumor, contributing to retraction (Figure 13A) (52). Pleural traction depression is more likely to appear in IACs than PGLs, and of the different types, pleural deformation is the most typical (53).
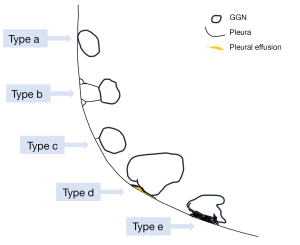
VDT and MDT
The VDT of nodules is a more useful metric to evaluate the pace of growth of persistent GGNs than the diameter (Figure 9). In making treatment decisions, this characteristic is essential for discriminating between benign and cancerous GGNs (54). The accuracy, methodology, and reproducibility of the VDT for lung nodules are susceptible to several factors. Generally, the following formula is used to calculate the VDT (55):
where V0 is the volume of the nodule measured at the first visit (mm3), Vt is the volume of the nodule measured at the last visit (mm3), and t is the interval time (d).
The volume of the above formula is usually measured using the following equation:
where a is the maximum diameter, and b is the vertical diameter.
It can also be calculated by the following formula:
where d0 is the diameter of the nodule measured at the first visit (mm), dt is the diameter of the nodule measured at the last visit (mm), and t is the interval time (d).
A VDT of more than 800 days often denotes benign lesions, while a VDT of 400 to 600 days is linked to AIS, AAH, and MIA, and a VDT of less than 400 days is linked to IAC (Figure 10). Conversely, a VDT of less than 20 days might suggest the presence of infection or inflammatory diseases. Hasegawa et al. (56) carried out a widespread high-resolution CT (GE) screening of lung cancer using the following scanning parameters: matrix: 512×512; tube voltage: 120 kV; tube current: 200 mA; and collimation: 1 or 3 mm. The study found that the average VDTs were 813 and 457 days for pGGNs and mGGNs, respectively. Li et al. (57) retrospectively analyzed CT scans from 167 individuals over three months. They manually segmented the GGN pictures in three dimensions, compared the volume growth rate, and calculated the VDT. Their data demonstrated that the VDT was 848 days for pGGNs and 598 days for mGGNs.
The growth rate of GGN is also influenced by the CTR. The higher the CTR value, the shorter the VDT. It is clear that in the case of malignant GGNs, a shorter VDT signifies a more aggressive histology tumor (58). Neoplastic GGNs develop slowly and inertly even throughout the growth phase, usually contributing to a prolonged VDT that might remain unchanged for many years. If a GGN does not diminish or vanish after three months, long-term follow up should be explored as per the 2019 Fleischner Pulmonary Disease Guidelines for managing GGNs.
However, in recent years, researchers have focused on employing mass double time (MDT) in quantitative analyses of specific small and slow-growing nodules, which monitors nodule growth sooner than VDT, making measurements of observed values more sensitive and realistic (59). MDT is expressed as follows:
where V is the volume of the lesion (mm3), and Amean is the mean density change (HU).
AI in GGN
GGN segmentation
The key component of a medical image analysis is image segmentation, which plays a crucial role in the precise calculation of the GGN volume and clinical management (60,61). Studies (62,63) have shown that deep-learning image reconstruction algorithms outperform conventional techniques in terms of accuracy when it comes to assessing GGN volume in low-dose CT. Moreover, because manual segmentation takes a great deal of time and is hampered by ambiguous borders and inter- or intra-observer variances, the use of AI to produce reliable automatic segmentation has become popular in clinical practice.
Kido et al. (64) created a novel loss function and proposed a nested three-dimensional (3D) fully connected convolutional network (CCN) with residual cell structure. The Dice coefficient and Intersection over Union (IoU) values of the CCN were 0.845±0.008 and 0.738±0.011, respectively (Table 2). Luo et al. (65) invented the 3D dual-attention shadowing network, DAS-Net, which improves segmentation accuracy and nodal surface information perception. In comparison to the baseline model, the model that included the adaptive dual-attention module had a Dice coefficient, sensitivity, and Hausdorff distance of 0.9205±0.0308, 0.9081±0.0635, and 0.93±0.0187, respectively.
Table 2
Authors | Year | Methods | Data sources | GGN applications | Results | Merits | Limitations |
---|---|---|---|---|---|---|---|
Kido S, et al. (64) | 2022 | 3D-CCN | In-house dataset (332 cases) | Segmentation | IoU: 0.738±0.011; DSC: 0.845±0.008 | Segments lung nodules attached to the chest wall or with GGOs | Sample number and type |
Luo S, et al. (65) | 2022 | DAS-Net | LIDC-IDRI | Segmentation | DSC: 0.920±0.030; Sn: 0.9081±0.0635; HD: 0.93±0.0187 | Enhances surface detailed information, shrinks parameters and model computation | – |
Akila Agnes S, et al. (66) | 2024 | Wavelet U-Net++ | LIDC-IDRI | Segmentation | IoU: 0.878±0.09; DSC: 0.937±0.14; Acc: 0.989±0.08 | Improves the segmentation of small and irregular nodules | – |
Wang S, et al. (67) | 2022 | CAP-Trans; CNN | LIDC-IDRI | Segmentation | DSC: 0.8986±0.0859; Sn: 0.9050±0.0946 | Improves and refines boundary segmentation | – |
Li X, et al. (68) | 2022 | Enhanced random wandering algorithm | LIDC-IDRI | Segmentation | Acc: 0.9434; OS: 0.86±0.08; Fscore: 0.8951 | Improves the segmentation of GGO nodules | – |
Miao J, et al. (69) | 2023 | 3D-CNN transformer | Luna16 dataset | Identification | Acc: 0.9589; Fscore: 0.9594 | Enhances 3D image automatic feature extraction | Single encoding method, sample number, and type |
Meng Q, et al. (70) | 2022 | 3D-DCNN | In-house dataset (736 cases) | Classification | Acc: 0.9177; Fscore: 0.9558; MCC: 0.3715; AUC (CI): 0.734 (0.585–0.884) | Improves clinical risk stratification management | Single-center, retrospective study, sample type |
Ma H, et al. (71) | 2022 | 3D U-Net; R-CNN | VESSEL12; LTRC; LCTSC; In-house dataset (456 cases) | Identification and classification | mAP: 0.518 (0.50–0.531) | Reduces false-positive rate, and improves detection accuracy | The ratio of benign to malignant lesions |
Wang C, et al. (72) | 2024 | 3D nnU-Net; AAG; SAM | LIDC-IDRI; in-house dataset (807 cases) | Identification and location | Acc: 0.955; Fscore: 0.876; Sp: 0.947; AUC (CI): 0.992 (0.984–0.998) | Increases reliability, interpretability, and accuracy | LIDC dataset classification standard |
Ding Y, et al. (73) | 2022 | 3D DenseSharp | In-house dataset (424 cases) | Diagnosis | Sn: 0.8229; Sp: 0.9048; PPV: 0.9724; AUC (CI): 0.899 (0.851–0.936) | Shows good diagnostic performance for nodules less than 2 cm |
Single-center study, sample number, and type |
AI, artificial intelligence; GGN, ground-glass nodule; 3D, three-dimensional; CCN, connected convolutional network; IoU, Intersection over Union; DSC, Dice similarity coefficient; GGO, ground-glass opacity; LIDC-IDRI, Lung Image Database Consortium and Image Database Resource Initiative; Sn, sensitivity; HD, Hausdroff distance; –, none reported; Acc, accuracy; CAP-Trans, Cascade-Axial-Prune Transformer; CNN, convolutional neural network; OS, overlap score; DCNN, deep convolutional neural network; MCC, Matthews correlation coefficient; AUC, area under the curve; CI, confidence interval; VESSEL12, VESsel SEgmentation in the Lung 2012; LTRC, Lung Tissue Research Consortium; LCTSC, Lung CT Segmentation Challenge 2017; mAP, mean average precision; AAG, anatomical attention gate; SAM, soft activation map; Sp, specificity; PPV, positive predictive value.
The implementation of AI has significantly enhanced the segmentation performance of image edges for some complex GGNs with fuzzy boundaries, irregular shapes, and low contrast with the surroundings. Akila et al. (66) introduced a new Wavelet U-Net++ method that combines the U-Net++ structure with wavelet pooling to capture high- and low-frequency information in the image, thus enhancing segmentation accuracy, especially small, irregular nodules. Wang et al. (67) developed the dual-path lung nodule segmentation model, DPBET, which uses a hybrid architecture made up of a convolutional neural network (CNN) and Cascade-Axial-Prune Transformer (CAP-Trans) to produce a global representation of the target lesion. Additionally, an edge detection operator is incorporated into the edge path to effectively perceive local characteristics at various scales and ranges. The test results on the public dataset Lung Image Database Consortium and Image Database Resource Initiative (LIDC-IDRI) had an average Dice coefficient of 89.86% and an average sensitivity of 90.50%. To improve the acquisition of the images’ edge information, the enhanced random wandering algorithm also uses a local search technique to generate more reliable seeds, thus enhancing the accuracy of GGN segmentation (68).
Automatic identification and classification of GGN
Recently, it has been recognized that AI shows promise in improving the sensitivity and accuracy of CT imaging. Due to the similarities in imaging, the accurate identification and categorization of GGNs is challenging. Currently, there is no reliable method for distinguishing among GGNs, with physicians relying on their empirical opinions. Deep-learning techniques, such as CNNs, have demonstrated potential in extracting comprehensive features from complex datasets, allowing for the screening of a large number of CT images and the quick labeling of suspicious lesions (74). Thus, it is a simple, convenient, non-invasive, and reproducible method that can aid imaging physicians in the identification and classification of GGNs. From the perspective of global 3D asymmetric feature representation, Miao et al. (69) proposed a transformer-based 3D lung GGN recognition model. The model used a 3D-CNN as its foundation, allowing it to learn richer global 3D asymmetric features and improving its feature extraction ability.
Meng et al. (70) integrated a multi-stage 3D-deep CNN algorithm (Figure 15) with the cLung Imaging Reporting and Data System 1.1 (cLung-RADS 1.1) to predict GGNs on the CT images of 506 patients. In the validation test, the accuracy, Matthews correlation coefficient (MCC), and area under the curve (AUC) were reported as 91.77%, 31.75%, and 0.734 [95% confidence interval (CI): 0.585–0.884], respectively. Thus, their approach demonstrated excellent performance in distinguishing between non-tumorigenic and tumorigenic GGNs. Another study proposed a two-stage 3D-GGN detection and classification framework, which used a two-stage image processing approach with a 3D U-Net and region CNN (R-CNN) on the CT images of 456 patients (71). Subsequently, the 3D model was used to detect and classify the GGNs. The detection accuracy was further improved through feature-weighted clustering.
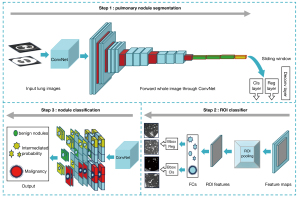
Wang et al. (72) developed a multi-task interpretable deep-learning model called ExPN-Net. This model not only detects and pinpoints important nodule features, such as subtlety, calcification, texture, sphericity, and edge features, but also diagnoses GGNs using the 3D nnU-Net, the anatomical attention gate (AAG) mechanism, and the soft activation map (SAM) module. The AUC values on the LIDC public and internal datasets were impressive at 0.992 and 0.923, respectively.
There has been an increasing number of studies in the field of multimodal collaborative AI modeling in recent years. Ding et al. (73) built a new lung nodule detection model by combining seven autoantibodies (p53, GAGE7, PGP9.5, CAGE, MAGEA1, SOX2, and GBU4-5), CEA, and a 3D DenseSharp network. The diagnostic model performed well in differentiating between benign and malignant lung nodules, especially those under 2 cm. The sensitivity, specificity, AUC, and positive predictive value of the model were 82.29%, 90.48%, 97.24%, and 0.899 (95% CI: 0.851–0.936), respectively. A joint AI model that integrated 3D-CNN, radiomics, and clinical data was shown to improve the benign/malignant classification, histologic type classification, and Lung-RADS classification of GGNs (75).
Assessment of GGN invasiveness
Radiologists typically use chest CT morphologic and quantitative data to evaluate the invasiveness of GGNs. Nonetheless, subjectivity and inter-observer variation in morphology are intrinsic and rely on the radiologists’ experience. Compared to morphological features, quantitative features are more reproducible when used in AI-assisted diagnosis (76).
A few studies (77,78) have shown that the use of AI-based nomograms might provide some important radiolytic parameters that could not be recognized by the naked eye, such as skewness, kurtosis, and entropy, which appear to have a good clinical application in assessing GGN invasiveness. Thus, an increasing number of scholars have combined nomograms with AI during the research process to explore their potential.
To screen out independent predictors of GGN invasiveness and establish an AI diagnostic model of CT histogram quantitative parameters, Deng et al. (79) retrospectively analyzed 102 chest high-resolution CT images of GGNs with a pathologically confirmed diameter ≤10 mm. The results showed that the entropy and mean CT value had a high diagnostic efficacy. Similarly, some researchers (80) assessed the efficacy of CT histogram metrics analyzed by AI in predicting GGN invasiveness in both plain and enhanced CT. These findings revealed that the mean CT value was an independent predictor of tumor invasiveness, and the presence of a contrast agent did not appear to affect its predictive performance.
The use of CT-guided percutaneous biopsy in the diagnosis of persistent lung GGNs
CT-guided transthoracic lung biopsy is a well-established and commonly practiced procedure in thoracic interventional radiology for the histologic diagnosis of lung lesions (81). Indications for imaging-guided chest biopsy include a new or enlarging solitary nodule or mass, multiple nodules in a patient without known neoplastic disease or in prolonged remission, focal parenchymal infiltrates in which an infectious organism cannot be isolated, a diagnosis of hilar masses following negative bronchoscopy, an undiagnosed mediastinal mass, and biopsy or re-biopsy of malignancy for targeted therapy (81).
Obtaining lesion tissue by percutaneous lung lesion biopsy under CT guidance is a minimally invasive, safe, and repeatable approach. It is crucial for diagnosing lung nodules and establishing the pathological diagnosis of lung lesions. LUNG-RADS version 1.1 recommends biopsy for four categories of nodules to determine the pathological diagnosis. A single-center retrospective analysis (82) of 114 patients with 117 lung nodules, of which 40 were GGNs and 77 were solitary pulmonary nodule (SPNs), sought to determine the accuracy of biopsy for nodules smaller than 8 mm. The sensitivity for malignant nodules, specificity for benign nodules, and the total diagnostic accuracy of lung nodule biopsy were 95.8% (91/95), 95.5% (21/22), and 95.7% (112/117), respectively. The positive and negative predictive values were 98.9% (91/92) and 87.5% (21/24), respectively, and the incidence of pneumothorax requiring chest tube placement was 6.8% (8/117). According to another meta-analysis (83), the sensitivity, specificity, diagnostic odds ratio, and AUC of the CT-guided percutaneous lung nodule biopsy were 0.91, 0.99, 138.72, and 0.97, respectively.
Nevertheless, false-negative results from lung nodule biopsies, particularly for mGGNs, can sometimes arise for a variety of reasons, including off-target sampling or failing to capture the invasiveness of the GGN (Figure 13). Meanwhile, bleeding (84.6%), pneumothorax (41.0%), and air embolism (0.9%) are among the complications of lung biopsy that increase medical expenses and put patients at risk (82).
It is necessary to discuss the advantages and disadvantages of lung GGN biopsy. As current research shows, the vast majority of lung GGNs, which are continuously present, correspond to various development stages of LUAD. CT plays an important role in the diagnosis of pulmonary GGNs, due to its high diagnostic accuracy and specificity, and radiomics is also excellent at predicting the invasiveness of GGNs (75). The integrity of the alveolar basement membrane and the vessel may be disrupted by biopsy for pGGNs. A single-center study showed Grade 1 pulmonary hemorrhages occurred in 10 of 92 (10.9%) procedures immediately after CT-guided fine needle aspiration biopsy (84). Pulmonary hemorrhage raises concerns about lesion basement membrane integrity. A key factor in determining the prognosis of LUAD is whether tumor cells invade through the basement membrane. Thus, CT-guided transthoracic lung biopsy might stimulate the lesion, speed up its progression, or even spread cancer cells (85). As a result, for patients with persistent GGNs, biopsy should be performed cautiously (Figure 10). Patients with GGNs who suffer from excessive anxiety may choose to engage in a form of mind-body exercise (e.g., Baduanjin) to help distract themselves, reduce their anxiety, and improve their lung function (86), or may ultimately choose to undergo thoracoscopic surgical resection to confirm the diagnosis of the lesion.
Discussion and outlook
According to the Fleischner guidelines, 6 mm was used as a cut-off value to define whether solitary GGNs require follow up to monitor the possibility of malignant changes (2). Mean CT values were used not only to predict GGN invasiveness but also to evaluate GGN growth (17). Previous studies have shown that the probability of unfavorable pathology types increases with an expansion in the solid component of a nodule (37). Further, the CTR value holds significant diagnostic value in determining the presence of lymph node metastasis, as well as the necessity and extent of lymph node dissection in peripheral lung cancer (39). VDT is used to estimate the growth rate of persistent GGNs, and a shorter VDT in malignant GGNs compared to benign lesions indicates a more histologically aggressive tumor (58). Malignant GGNs frequently exhibit invasive manifestations, such as vacuoles, air bronchus signs, tumor vascular signs, spicules, lobulations, and pleural depression (78,87,88). Among these, benign lesions typically exhibit a normal or dilated bronchial lumen, while malignant bronchi are often narrow, truncated, occluded, or twisted.
Near the hilum of the lung and superior lung lobe, especially the right lung, GGNs are more likely to be missed, and on CT images, it can be challenging for doctors to recognize GGNs that are overlaid with surrounding structures, such as the ribs, lung vessels, heart, and mediastinum (89), which complicates image interpretation and reduces diagnostic accuracy. To quantify the association between blood vessels and GGNs, some researchers (90) created a Residual U-Network (ResUNet) structure for the fully automated detection and quantification of vascular structures in GGNs. In cases in which lung nodules are too small, doctors may overlook them. Physician sensitivity is quite low when small lung nodules are present. The development of multi-slice CT technology and computer-aided design tools has improved the ability of radiologists to identify very small pulmonary lesions (89).
A nodule’s edge characteristics can aid radiology doctors to better define a nodule’s nature and make a differential diagnosis; however, indistinct edges can also contribute to the identification of pulmonary nodules being ignored. Benzakoun et al. (91) proposed that GGO makes it more difficult for software to distinguish attenuation variations from the surrounding thin-walled tissue, which could complicate diagnosis. Saha et al. (92) conducted a study focusing on the segmentation, morphology, and distribution patterns of GGOs, and developed an end-to-end unsupervised deep-learning method, PointNet++, to detect and quantify GGOs in the CT scans of COVID-19 patients, achieving GGO detection with an accuracy of up to 98%.
At the moment, the most used technique for evaluating GGN features is thin-layer CT imaging. Morphologic features can be used to distinguish between benign and malignant GGNs. A single indicator is not particularly helpful in determining invasiveness because of its low sensitivity and specificity. A more promising method for predicting pathologic invasiveness would be to use combined prediction models that incorporate numerous variables. The prediction of GGN invasiveness has greatly improved with the use of AI, and column-line drawings based on AI have the potential to enhance diagnostic efficacy and support clinical GGN management (77).
Diagnostic models based on deep learning, which have made significant strides in image processing and diagnosis, can quickly identify suspicious lesions in a large number of CT images. This allows physicians to make more informed decisions, reduces workloads, and enhances the precision of differential diagnosis. Greffier et al. (93) discovered that applying AI deep-learning reconstruction methods enhances the contrast-to-noise ratio and lowers image noise, which enhances lesion identification and boosts diagnostic confidence. Similarly, a related study discovered that using higher degrees of iterative reconstruction intensity led to decreased picture noise and enhanced contrast-to-noise ratios (94), further optimizing the effectiveness of automated nodule recognition. Despite their benefits, to reach a high accuracy, models require a large amount of high-quality training data, most of the published studies lack generalization, internal work is opaque, and there is little information available as to how these tools affect radiologists’ decision making and patient prognosis. Further, one major problem with AI pulmonary nodule identification is the false-positive rate (95).
Given the high detection rate of pulmonary GGNs, it is encouraging that more and more significant guidelines and new technologies are being developed to provide better diagnostic and prognostic indicators. It is essential that the questions of how to optimize and rationally combine existing diagnostic tools and techniques to improve diagnostic sensitivity and specificity, and how to choose and implement therapy methods are explored and addressed. In the future, we anticipate that further research and investigations will be conducted on GGN diagnostic imaging (e.g., on the use and improvement of magnetic resonance imaging in diagnosing pulmonary GGNs) and molecular imaging (e.g., on the effects of contrast agent-carrying nanoparticle material on diagnosing the aggressiveness of GGNs).
Acknowledgments
Funding: This study was supported by
Footnote
Reporting Checklist: The authors have completed the Narrative Review reporting checklist. Available at https://qims.amegroups.com/article/view/10.21037/qims-24-674/rc
Conflicts of Interest: All authors have completed the ICMJE uniform disclosure form (available at https://qims.amegroups.com/article/view/10.21037/qims-24-674/coif). The authors have no conflicts of interest to declare.
Ethical Statement: The authors are accountable for all aspects of the work in ensuring that questions related to the accuracy or integrity of any part of the work are appropriately investigated and resolved.
Open Access Statement: This is an Open Access article distributed in accordance with the Creative Commons Attribution-NonCommercial-NoDerivs 4.0 International License (CC BY-NC-ND 4.0), which permits the non-commercial replication and distribution of the article with the strict proviso that no changes or edits are made and the original work is properly cited (including links to both the formal publication through the relevant DOI and the license). See: https://creativecommons.org/licenses/by-nc-nd/4.0/.
References
- Walter JE, Heuvelmans MA, de Bock GH, Yousaf-Khan U, Groen HJM, van der Aalst CM, Nackaerts K, van Ooijen PMA, de Koning HJ, Vliegenthart R, Oudkerk M. Relationship between the number of new nodules and lung cancer probability in incidence screening rounds of CT lung cancer screening: The NELSON study. Lung Cancer 2018;125:103-8. [Crossref] [PubMed]
- MacMahon H, Naidich DP, Goo JM, Lee KS, Leung ANC, Mayo JR, Mehta AC, Ohno Y, Powell CA, Prokop M, Rubin GD, Schaefer-Prokop CM, Travis WD, Van Schil PE, Bankier AA. Guidelines for Management of Incidental Pulmonary Nodules Detected on CT Images: From the Fleischner Society 2017. Radiology 2017;284:228-43. [Crossref] [PubMed]
- Nicholson AG, Tsao MS, Beasley MB, Borczuk AC, Brambilla E, Cooper WA, Dacic S, Jain D, Kerr KM, Lantuejoul S, Noguchi M, Papotti M, Rekhtman N, Scagliotti G, van Schil P, Sholl L, Yatabe Y, Yoshida A, Travis WD. The 2021 WHO Classification of Lung Tumors: Impact of Advances Since 2015. J Thorac Oncol 2022;17:362-87. [Crossref] [PubMed]
- Cai Y, Chen T, Zhang S, Tan M, Wang J. Correlation exploration among CT imaging, pathology and genotype of pulmonary ground-glass opacity. J Cell Mol Med 2023;27:2021-31. [Crossref] [PubMed]
- Henschke CI, Yankelevitz DF, Mirtcheva R, McGuinness G, McCauley D, Miettinen OS. ELCAP Group. CT screening for lung cancer: frequency and significance of part-solid and nonsolid nodules. AJR Am J Roentgenol 2002;178:1053-7. [Crossref] [PubMed]
- de Margerie-Mellon C, de Bazelaire C, de Kerviler E. Image-guided biopsy in primary lung cancer: Why, when and how. Diagn Interv Imaging 2016;97:965-72. [Crossref] [PubMed]
- Kops SEP, Heus P, Korevaar DA, Damen JAA, Idema DL, Verhoeven RLJ, Annema JT, Hooft L, van der Heijden EHFM. Diagnostic yield and safety of navigation bronchoscopy: A systematic review and meta-analysis. Lung Cancer 2023;180:107196. [Crossref] [PubMed]
- Pinto-Coelho L. How Artificial Intelligence Is Shaping Medical Imaging Technology: A Survey of Innovations and Applications. Bioengineering (Basel) 2023;10:1435. [Crossref] [PubMed]
- de Margerie-Mellon C, Chassagnon G. Artificial intelligence: A critical review of applications for lung nodule and lung cancer. Diagn Interv Imaging 2023;104:11-7. [Crossref] [PubMed]
- McWilliams A, Tammemagi MC, Mayo JR, Roberts H, Liu G, Soghrati K, et al. Probability of cancer in pulmonary nodules detected on first screening CT. N Engl J Med 2013;369:910-9. [Crossref] [PubMed]
- Horeweg N, van der Aalst CM, Thunnissen E, Nackaerts K, Weenink C, Groen HJ, Lammers JW, Aerts JG, Scholten ET, van Rosmalen J, Mali W, Oudkerk M, de Koning HJ. Characteristics of lung cancers detected by computer tomography screening in the randomized NELSON trial. Am J Respir Crit Care Med 2013;187:848-54. [Crossref] [PubMed]
- Lee SM, Park CM, Goo JM, Lee HJ, Wi JY, Kang CH. Invasive pulmonary adenocarcinomas versus preinvasive lesions appearing as ground-glass nodules: differentiation by using CT features. Radiology 2013;268:265-73. [Crossref] [PubMed]
- Hiramatsu M, Inagaki T, Inagaki T, Matsui Y, Satoh Y, Okumura S, Ishikawa Y, Miyaoka E, Nakagawa K. Pulmonary ground-glass opacity (GGO) lesions-large size and a history of lung cancer are risk factors for growth. J Thorac Oncol 2008;3:1245-50. [Crossref] [PubMed]
- Truong MT, Ko JP, Rossi SE, Rossi I, Viswanathan C, Bruzzi JF, Marom EM, Erasmus JJ. Update in the evaluation of the solitary pulmonary nodule. Radiographics 2014;34:1658-79. [Crossref] [PubMed]
- He W, Guo G, Du X, Guo S, Zhuang X. CT imaging indications correlate with the degree of lung adenocarcinoma infiltration. Front Oncol 2023;13:1108758. [Crossref] [PubMed]
- Sun Y, Li C, Jin L, Gao P, Zhao W, Ma W, Tan M, Wu W, Duan S, Shan Y, Li M. Radiomics for lung adenocarcinoma manifesting as pure ground-glass nodules: invasive prediction. Eur Radiol 2020;30:3650-9. [Crossref] [PubMed]
- He S, Chen C, Wang Z, Yu X, Liu S, Huang Z, Chen C, Liang Z, Chen C. The use of the mean computed-tomography value to predict the invasiveness of ground-glass nodules: A meta-analysis. Asian J Surg 2023;46:677-82. [Crossref] [PubMed]
- Tamura M, Matsumoto I, Saito D, Yoshida S, Kakegawa S, Takemura H. Mean Computed Tomography Value to Predict the Tumor Invasiveness in Clinical Stage IA Lung Cancer. Ann Thorac Surg 2017;104:261-6. [Crossref] [PubMed]
- Xiong Z, Jiang Y, Che S, Zhao W, Guo Y, Li G, Liu A, Li Z. Use of CT radiomics to differentiate minimally invasive adenocarcinomas and invasive adenocarcinomas presenting as pure ground-glass nodules larger than 10 mm. Eur J Radiol 2021;141:109772. [Crossref] [PubMed]
- He Y, Xiong Z, Zhang J, Xie J, Zhu W, Zhao M, Li Z. Growth assessment of pure ground-glass nodules on CT: comparison of density and size measurement methods. J Cancer Res Clin Oncol 2023;149:9937-46. [Crossref] [PubMed]
- Eguchi T, Kondo R, Kawakami S, Matsushita M, Yoshizawa A, Hara D, Matsuoka S, Takeda T, Miura K, Agatsuma H, Sakaizawa T, Tominaga Y, Saito G, Toishi M, Hamanaka K, Hashizume M, Shiina T, Amano J, Koizumi T, Yoshida K. Computed tomography attenuation predicts the growth of pure ground-glass nodules. Lung Cancer 2014;84:242-7. [Crossref] [PubMed]
- Tamura M, Shimizu Y, Yamamoto T, Yoshikawa J, Hashizume Y. Predictive value of one-dimensional mean computed tomography value of ground-glass opacity on high-resolution images for the possibility of future change. J Thorac Oncol 2014;9:469-72. [Crossref] [PubMed]
- Chabi ML, Goracci A, Roche N, Paugam A, Lupo A, Revel MP. Pulmonary aspergillosis. Diagn Interv Imaging 2015;96:435-42. [Crossref] [PubMed]
- Okada F, Ono A, Ando Y, Yotsumoto S, Yotsumoto S, Tanoue S, Matsumoto S, Wakisaka M, Mori H. Pulmonary computed tomography findings of visceral larva migrans caused by Ascaris suum. J Comput Assist Tomogr 2007;31:402-8. [Crossref] [PubMed]
- Park S, Kim YS, Kim YJ, Kyung SY, Park JW, Jeong SH, Lee SP. Toxocariasis masquerading as liver and lung metastatic nodules in patents with gastrointestinal cancer: clinicopathologic study of five cases. Dig Dis Sci 2012;57:155-60. [Crossref] [PubMed]
- Kaneria SS, Tarkin J, Williams G, Bain G, Quigley M. Case report: the CT halo sign: a rare manifestation of squamous cell carcinoma of the lung. Clin Radiol 2012;67:613-5. [Crossref] [PubMed]
- Rampinelli C, Vecchi V, Bellomi M. From reversed halo sign to halo sign: unusual centripetal growing of a lung adenocarcinoma. J Thorac Oncol 2014;9:1230. [Crossref] [PubMed]
- Wetscherek MTA, Sadler TJ, Lee JYJ, Karia S, Babar JL. Active pulmonary tuberculosis: something old, something new, something borrowed, something blue. Insights Imaging 2022;13:3. [Crossref] [PubMed]
- Ma X, Li A, Cao W, Li H, Zhang S, Li L, Xing H, Tian W, Jiao P, Chen J, Zhang Q, Xu A, Xing L. Characteristics of Mucormycosis in Hematological Patients and a Death Prediction Model. Front Microbiol 2021;12:784974. [Crossref] [PubMed]
- Alexander BD, Lamoth F, Heussel CP, Prokop CS, Desai SR, Morrissey CO, Baddley JW. Guidance on Imaging for Invasive Pulmonary Aspergillosis and Mucormycosis: From the Imaging Working Group for the Revision and Update of the Consensus Definitions of Fungal Disease from the EORTC/MSGERC. Clin Infect Dis 2021;72:S79-88. [Crossref] [PubMed]
- Legouge C, Caillot D, Chrétien ML, Lafon I, Ferrant E, Audia S, Pagès PB, Roques M, Estivalet L, Martin L, Maitre T, Bastie JN, Dalle F. The reversed halo sign: pathognomonic pattern of pulmonary mucormycosis in leukemic patients with neutropenia? Clin Infect Dis 2014;58:672-8. [Crossref] [PubMed]
- Marchiori E, Marom EM, Zanetti G, Hochhegger B, Irion KL, Godoy MCB. Reversed halo sign in invasive fungal infections: criteria for differentiation from organizing pneumonia. Chest 2012;142:1469-73. [Crossref] [PubMed]
- Casullo J, Semionov A. Reversed halo sign in acute pulmonary embolism and infarction. Acta Radiol 2013;54:505-10. [Crossref] [PubMed]
- Almeida RR, Marchiori E, Flores EJ. Frequency and Reliability of the Reversed Halo Sign in Patients With Septic Pulmonary Embolism Due to IV Substance Use Disorder. AJR Am J Roentgenol 2020;214:59-67. [Crossref] [PubMed]
- Mango VL, Naidich DP, Godoy MC. Reversed halo sign after radiofrequency ablation of a lung nodule. J Thorac Imaging 2011;26:W150-2. [Crossref] [PubMed]
- Tzilas V, Poletti V, Bouros D. Reversed halo sign in radiation induced organizing pneumonia: natural course of the underlying pathophysiology. Pulmonology 2021;27:460-4. [Crossref] [PubMed]
- Suzuki K, Kusumoto M, Watanabe S, Tsuchiya R, Asamura H. Radiologic classification of small adenocarcinoma of the lung: radiologic-pathologic correlation and its prognostic impact. Ann Thorac Surg 2006;81:413-9. [Crossref] [PubMed]
- Xi J, Yin J, Liang J, Zhan C, Jiang W, Lin Z, Xu S, Wang Q. Prognostic Impact of Radiological Consolidation Tumor Ratio in Clinical Stage IA Pulmonary Ground Glass Opacities. Front Oncol 2021;11:616149. [Crossref] [PubMed]
- Huang H, Ye B. Research Progress in the Effect of Consolidation Tumor Ratio on the Diagnosis and Treatment of Early-stage Peripheral Lung Cancer. Zhongguo Fei Ai Za Zhi 2022;25:764-70. [Crossref] [PubMed]
- Chen YC, Lin YH, Chien HC, Hsu PK, Hung JJ, Huang CS, Hsieh CC, Hsu WH, Hsu HS. Preoperative consolidation-to-tumor ratio is effective in the prediction of lymph node metastasis in patients with pulmonary ground-glass component nodules. Thorac Cancer 2021;12:1203-9. [Crossref] [PubMed]
- Tsai TM, Liu CY, Lin MW, Hsu HH, Chen JS. Factors Associated with Nodal Upstaging in Clinical T1a-bN0M0 Non-Small Cell Lung Cancers. Cancers (Basel) 2022;14:1277. [Crossref] [PubMed]
- Zhang Y, Fu F, Wen Z, Deng L, Wang S, Li Y, Chen H. Segment Location and Ground Glass Opacity Ratio Reliably Predict Node-Negative Status in Lung Cancer. Ann Thorac Surg 2020;109:1061-8. [Crossref] [PubMed]
- Suzuki S, Sakurai H, Yotsukura M, Masai K, Asakura K, Nakagawa K, Motoi N, Watanabe SI. Clinical Features of Ground Glass Opacity-Dominant Lung Cancer Exceeding 3.0 cm in the Whole Tumor Size. Ann Thorac Surg 2018;105:1499-506. [Crossref] [PubMed]
- Zhou Y, Zhang Y, Zhang S, Zhang C, Chen Z. Growth Regularity of Pulmonary Ground Glass Nodules Based on 3D Reconstruction Technology. Zhongguo Fei Ai Za Zhi 2023;26:265-73. [Crossref] [PubMed]
- Qu H, Zhang W, Yang J, Jia S, Wang G. The value of the air bronchogram sign on CT image in the identification of different solitary pulmonary consolidation lesions. Medicine (Baltimore) 2018;97:e11985. [Crossref] [PubMed]
- Marchiori E, Hochhegger B, Zanetti G. Dilated Air Bronchogram Inside Areas of Consolidation: A Tomographic Finding Suggestive of Pulmonary Lymphoma. Arch Bronconeumol (Engl Ed) 2019;55:383-4. [Crossref] [PubMed]
- Yang Y, Gao J, Jin M, Luo C. WU C, Zhao S. Abnormal air bronchogram within pure ground glass opacity lung adenocarcinoma: value for predicting histopathologic subtypes. Chin J Radiol 2017;489-92.
- Gao F, Li M, Ge X, Zheng X, Ren Q, Chen Y, Lv F, Hua Y. Multi-detector spiral CT study of the relationships between pulmonary ground-glass nodules and blood vessels. Eur Radiol 2013;23:3271-7. [Crossref] [PubMed]
- Chu ZG, Li WJ, Fu BJ, Lv FJ. CT Characteristics for Predicting Invasiveness in Pulmonary Pure Ground-Glass Nodules. AJR Am J Roentgenol 2020;215:351-8. [Crossref] [PubMed]
- Snoeckx A, Reyntiens P, Desbuquoit D, Spinhoven MJ, Van Schil PE, van Meerbeeck JP, Parizel PM. Evaluation of the solitary pulmonary nodule: size matters, but do not ignore the power of morphology. Insights Imaging 2018;9:73-86. [Crossref] [PubMed]
- Cruickshank A, Stieler G, Ameer F. Evaluation of the solitary pulmonary nodule. Intern Med J 2019;49:306-15. [Crossref] [PubMed]
- Zhao Q, Wang JW, Yang L, Xue LY, Lu WW. CT diagnosis of pleural and stromal invasion in malignant subpleural pure ground-glass nodules: an exploratory study. Eur Radiol 2019;29:279-86. [Crossref] [PubMed]
- Jiang Y, Xiong Z, Zhao W, Tian D, Zhang Q, Li Z. Pathological components and CT imaging analysis of the area adjacent pleura within the pure ground-glass nodules with pleural deformation in invasive lung adenocarcinoma. BMC Cancer 2022;22:958. [Crossref] [PubMed]
- Oudkerk M, Liu S, Heuvelmans MA, Walter JE, Field JK. Lung cancer LDCT screening and mortality reduction - evidence, pitfalls and future perspectives. Nat Rev Clin Oncol 2021;18:135-51. [Crossref] [PubMed]
- Oxnard GR, Zhao B, Sima CS, Ginsberg MS, James LP, Lefkowitz RA, Guo P, Kris MG, Schwartz LH, Riely GJ. Variability of lung tumor measurements on repeat computed tomography scans taken within 15 minutes. J Clin Oncol 2011;29:3114-9. [Crossref] [PubMed]
- Hasegawa M, Sone S, Takashima S, Li F, Yang ZG, Maruyama Y, Watanabe T. Growth rate of small lung cancers detected on mass CT screening. Br J Radiol 2000;73:1252-9. [Crossref] [PubMed]
- Li J, Xia T, Yang X, Dong X, Liang J, Zhong N, Guan Y. Malignant solitary pulmonary nodules: assessment of mass growth rate and doubling time at follow-up CT. J Thorac Dis 2018;10:S797-806. [Crossref] [PubMed]
- Oda S, Awai K, Murao K, Ozawa A, Utsunomiya D, Yanaga Y, Kawanaka K, Yamashita Y. Volume-doubling time of pulmonary nodules with ground glass opacity at multidetector CT: Assessment with computer-aided three-dimensional volumetry. Acad Radiol 2011;18:63-9. [Crossref] [PubMed]
- Song YS, Park CM, Park SJ, Lee SM, Jeon YK, Goo JM. Volume and mass doubling times of persistent pulmonary subsolid nodules detected in patients without known malignancy. Radiology 2014;273:276-84. [Crossref] [PubMed]
- Takahashi S, Tanaka N, Okimoto T, Tanaka T, Ueda K, Matsumoto T, Ashizawa K, Kunihiro Y, Kido S, Matsunaga N. Long term follow-up for small pure ground-glass nodules: implications of determining an optimum follow-up period and high-resolution CT findings to predict the growth of nodules. Jpn J Radiol 2012;30:206-17. [Crossref] [PubMed]
- Ma J, He Y, Li F, Han L, You C, Wang B. Segment anything in medical images. Nat Commun 2024;15:654. [Crossref] [PubMed]
- Kim C, Kwack T, Kim W, Cha J, Yang Z, Yong HS. Accuracy of two deep learning-based reconstruction methods compared with an adaptive statistical iterative reconstruction method for solid and ground-glass nodule volumetry on low-dose and ultra-low-dose chest computed tomography: A phantom study. PLoS One 2022;17:e0270122. [Crossref] [PubMed]
- Mikayama R, Shirasaka T, Kojima T, Sakai Y, Yabuuchi H, Kondo M, Kato T. Deep-learning reconstruction for ultra-low-dose lung CT: Volumetric measurement accuracy and reproducibility of artificial ground-glass nodules in a phantom study. Br J Radiol 2022;95:20210915. [Crossref] [PubMed]
- Kido S, Kidera S, Hirano Y, Mabu S, Kamiya T, Tanaka N, Suzuki Y, Yanagawa M, Tomiyama N. Segmentation of Lung Nodules on CT Images Using a Nested Three-Dimensional Fully Connected Convolutional Network. Front Artif Intell 2022;5:782225. [Crossref] [PubMed]
- Luo S, Zhang J, Xiao N, Qiang Y, Li K, Zhao J, Meng L, Song P. DAS-Net: A lung nodule segmentation method based on adaptive dual-branch attention and shadow mapping. Appl Intell 2022;52:15617-31.
- Akila Agnes S, Arun Solomon A, Karthick K. Wavelet U-Net++ for accurate lung nodule segmentation in CT scans: Improving early detection and diagnosis of lung cancer. Biomed Signal Process Control 2024;87:105509.
- Wang S, Jiang A, Li X, Qiu Y, Li M, Li F. DPBET: A dual-path lung nodules segmentation model based on boundary enhancement and hybrid transformer. Comput Biol Med 2022;151:106330. [Crossref] [PubMed]
- Li X, Li B, Yin H, Xu B. An Automatic Random Walker Algorithm for Segmentation of Ground Glass Opacity Pulmonary Nodules. J Healthc Eng 2022;2022:6727957. [Crossref] [PubMed]
- Miao J, Zhang M, Chang Y, Qiao Y. Transformer-Based Recognition Model for Ground-Glass Nodules from the View of Global 3D Asymmetry Feature Representation. Symmetry 2023;15:2192.
- Meng Q, Li B, Gao P, Liu W, Zhou P, Ding J, Zhang J, Ge H. Development and Validation of a Risk Stratification Model of Pulmonary Ground-Glass Nodules Based on Complementary Lung-RADS 1.1 and Deep Learning Scores. Front Public Health 2022;10:891306. [Crossref] [PubMed]
- Ma H, Guo H, Zhao M, Qi S, Li H, Tian Y, Li Z, Zhang G, Yao Y, Qian W. Automatic pulmonary ground‐glass opacity nodules detection and classification based on 3D neural network. Medical Physics 2022;49:2555-69. [Crossref] [PubMed]
- Wang C, Liu Y, Wang F, Zhang C, Wang Y, Yuan M, Yang G. Towards reliable and explainable AI model for pulmonary nodule diagnosis. Biomed Signal Process Control 2024;88:105646.
- Ding Y, Zhang J, Zhuang W, Gao Z, Kuang K, Tian D, Deng C, Wu H, Chen R, Lu G, Chen G, Mendogni P, Migliore M, Kang MW, Kanzaki R, Tang Y, Yang J, Shi Q, Qiao G. Improving the efficiency of identifying malignant pulmonary nodules before surgery via a combination of artificial intelligence CT image recognition and serum autoantibodies. Eur Radiol 2023;33:3092-102. [Crossref] [PubMed]
- Yadav SS, Jadhav SM. Deep convolutional neural network based medical image classification f or disease diagnosis. J Big Data 2019;6:113.
- Lin CY, Guo SM, Lien JJ, Lin WT, Liu YS, Lai CH, Hsu IL, Chang CC, Tseng YL. Combined model integrating deep learning, radiomics, and clinical data to classify lung nodules at chest CT. Radiol Med 2024;129:56-69. [Crossref] [PubMed]
- Liu J, Yang X, Li Y, Xu H, He C, Zhou P, Qing H. Predicting the Invasiveness of Pulmonary Adenocarcinomas in Pure Ground-Glass Nodules Using the Nodule Diameter: A Systematic Review, Meta-Analysis, and Validation in an Independent Cohort. Diagnostics (Basel) 2024;14:147. [Crossref] [PubMed]
- Gao J, Qi Q, Li H, Wang Z, Sun Z, Cheng S, Yu J, Zeng Y, Hong N, Wang D, Wang H, Yang F, Li X, Li Y. Artificial-intelligence-based computed tomography histogram analysis predicting tumor invasiveness of lung adenocarcinomas manifesting as radiological part-solid nodules. Front Oncol 2023;13:1096453. [Crossref] [PubMed]
- Zhu M, Yang Z, Wang M, Zhao W, Zhu Q, Shi W, Yu H, Liang Z, Chen L. A computerized tomography-based radiomic model for assessing the invasiveness of lung adenocarcinoma manifesting as ground-glass opacity nodules. Respir Res 2022;23:96. [Crossref] [PubMed]
- Deng Q, Pan A, Xu Z. The predictive value of CT histogram parameter model based on artificial intelligence technology for the invasiveness of microscopic ground-glass nodular lung adenocarcinoma. Radiologic Practice 2022;37:977-81.
- Zhang H, Wang D, Li W, Tian Z, Ma L, Guo J, Wang Y, Sun X, Ma X, Ma L, Zhu L. Artificial intelligence system-based histogram analysis of computed tomography features to predict tumor invasiveness of ground-glass nodules. Quant Imaging Med Surg 2023;13:5783-95. [Crossref] [PubMed]
- Anzidei M, Porfiri A, Andrani F, Di Martino M, Saba L, Catalano C, Bezzi M. Imaging-guided chest biopsies: techniques and clinical results. Insights Imaging 2017;8:419-28. [Crossref] [PubMed]
- Zhao Y, Matsui Y, Hiraki T, Iguchi T, Tomita K, Uka M, Gobara H, Toyooka S, Kanazawa S. Computed tomography fluoroscopy-guided cutting needle biopsy of pulmonary nodules ≤8 mm: A retrospective study including 117 nodules. Eur J Radiol 2020;125:108903. [Crossref] [PubMed]
- Wang B, Zhong F, An W, Liao M. The diagnostic value of CT-guided percutaneous puncture biopsy of pulmonary ground-glass nodules: a meta-analysis. Acta Radiol 2023;64:1431-8. [Crossref] [PubMed]
- Peng JZ, Bie ZX, Li YM, Li B, Guo RQ, Wang CE, Xu S, Li XG. Diagnostic performance and safety of percutaneous fine-needle aspiration immediately before microwave ablation for pulmonary ground-glass nodules. Quant Imaging Med Surg 2023;13:3852-61. [Crossref] [PubMed]
- Wu CC, Maher MM, Shepard JA. Complications of CT-guided percutaneous needle biopsy of the chest: prevention and management. AJR Am J Roentgenol 2011;196:W678-82. [Crossref] [PubMed]
- Lu Y, Li J, Ni W, Li J, Song J, Jiang J, Zhao X. Effectiveness of mind-body exercise via Baduanjin on physical and psychological outcomes in patients with pulmonary ground-glass nodules: A non-randomized controlled pilot study. Complement Ther Clin Pract 2023;50:101679. [Crossref] [PubMed]
- Liang ZR, Ye M, Lv FJ, Fu BJ, Lin RY, Li WJ, Chu ZG. Differential diagnosis of benign and malignant patchy ground-glass opacity by thin-section computed tomography. BMC Cancer 2022;22:1206. [Crossref] [PubMed]
- Chen X, Yao B, Li J, Liang C, Qi R, Yu J. Feasibility of Using High-Resolution Computed Tomography Features for Invasiveness Differentiation of Malignant Nodules Manifesting as Ground-Glass Nodules. Can Respir J 2022;2022:2671772. [Crossref] [PubMed]
- Del Ciello A, Franchi P, Contegiacomo A, Cicchetti G, Bonomo L, Larici AR. Missed lung cancer: when, where, and why? Diagn Interv Radiol 2017;23:118-26. [Crossref] [PubMed]
- Zhao B, Wang X, Sun K, Kang H, Zhang K, Yin H, Liu K, Xiao Y, Liu S. Correlation Between Intranodular Vessels and Tumor Invasiveness of Lung Adenocarcinoma Presenting as Ground-glass Nodules: A Deep Learning 3-Dimensional Reconstruction Algorithm-based Quantitative Analysis on Noncontrast Computed Tomography Images. J Thorac Imaging 2023;38:297-303. [Crossref] [PubMed]
- Benzakoun J, Bommart S, Coste J, Chassagnon G, Lederlin M, Boussouar S, Revel MP. Computer-aided diagnosis (CAD) of subsolid nodules: Evaluation of a commercial CAD system. Eur J Radiol 2016;85:1728-34. [Crossref] [PubMed]
- Saha M, Amin SB, Sharma A, Kumar TKS, Kalia RK. AI-driven quantification of ground glass opacities in lungs of COVID-19 patients using 3D computed tomography imaging. PLoS One 2022;17:e0263916. [Crossref] [PubMed]
- Greffier J, Si-Mohamed S, Frandon J, Loisy M, de Oliveira F, Beregi JP, Dabli D. Impact of an artificial intelligence deep-learning reconstruction algorithm for CT on image quality and potential dose reduction: A phantom study. Med Phys 2022;49:5052-63. [Crossref] [PubMed]
- Schwyzer M, Messerli M, Eberhard M, Skawran S, Martini K, Frauenfelder T. Impact of dose reduction and iterative reconstruction algorithm on the detectability of pulmonary nodules by artificial intelligence. Diagn Interv Imaging 2022;103:273-80. [Crossref] [PubMed]
- Lan CC, Hsieh MS, Hsiao JK, Wu CW, Yang HH, Chen Y, Hsieh PC, Tzeng IS, Wu YK. Deep Learning-based Artificial Intelligence Improves Accuracy of Error-prone Lung Nodules. Int J Med Sci 2022;19:490-8. [Crossref] [PubMed]