Deep learning models for rapid discrimination of high-grade gliomas from solitary brain metastases using multi-plane T1-weighted contrast-enhanced (T1CE) images
Introduction
High-grade gliomas (HGG) and solitary brain metastases (SBM) are two of the most common types of brain tumors in middle-aged and elderly patients. HGG originate from glial cells, accounting for approximately 70% of all primary brain tumors (1). In contrast, SBM result from cancer cells spreading from other parts of the body to the brain (2). Given their distinct origins and mechanisms of occurrence, their therapeutic principles significantly differ (3,4). The primary treatment for HGG often involves surgery, supplemented by postoperative radiotherapy and chemotherapy. Meanwhile, for SBM, it is crucial first to ascertain the original location of the tumor, with treatment primarily targeting the original lesion.
Brain magnetic resonance imaging (MRI) is a routine examination for intracranial tumor patients. However, HGG and SBM display a high degree of similarity on MRI images, manifesting as irregular enhancement in the core region accompanied by extensive edema in the surrounding regions. Consequently, differential diagnosis using preoperative MRI remains challenging. In current clinical practice, for patients with unclear diagnoses, invasive procedures like biopsy or open surgery are often adopted to obtain tumor tissue for pathological diagnosis (5). For many patients, these methods induce additional physical and psychological stress, escalating surgery risks and potential complications. If a non-invasive method could be employed for accurate preoperative differential diagnosis, it would positively impact patient treatment and recovery. In recent years, with the introduction of magnetic resonance spectroscopy (MRS), perfusion magnetic resonance (MR), and positron emission tomography (PET)/MR, the ability to differentiate between HGG and SBM has improved (6). However, these multimodal MR technologies is time-consuming and expensive, investigating on ways to use conventional T1-weighted contrast-enhanced (T1CE) images to improve diagnostic accuracy is of great value.
In the past few years, machine learning and deep learning have shown promising applications in disease diagnosis, especially in tumor automatic identification and segmentation. Some studies leveraging traditional machine learning models for differentiating HGG and SBM rely heavily on various radiomic features, with support vector machine (SVM) classifiers demonstrating commendable performance (7-9). Nonetheless, extracting radiomics features demands rigorous image preprocessing. Moreover, these features require manual extraction and selection, making clinical applications cumbersome (10). Deep learning, a subset of machine learning, with its models based on convolutional neural network (CNN), has been applied in medical image processing (11). It consistently delivers exceptional performance in clinical classification and diagnostic tasks. The advantage of deep learning over traditional machine learning is that it can extract features directly from images without manual intervention, often surpassing the discrimination performance of traditional machine learning models (12). Recent researches have capitalized on deep learning for classifying and grading predictions between HGG and SBM (10,13,14). Nevertheless, current studies tend to focus on the tumor core region and often overlook the peritumoral edema region. Whether the edema region contributes to tumor classification remains under-researched. Comparing machine learning classifiers is crucial as it helps in identifying the most effective model for accurately distinguishing between HGG and SBM, particularly when considering different regions of the tumor and surrounding areas. By evaluating various models, we can determine the strengths and weaknesses of each approach, ensuring that the chosen method provides the highest diagnostic accuracy. This is especially timely given the advancements in imaging techniques and the growing importance of non-invasive diagnostic methods in clinical practice.
This study employed current mainstream deep learning models to conduct a comparative analysis of the core regions, peritumoral edema regions, and overall characteristics between HGG and SBM using multi-plane T1CE images. We present this article in accordance with the TRIPOD reporting checklist (available at https://qims.amegroups.com/article/view/10.21037/qims-24-380/rc).
Methods
Patients
We retrospectively reviewed data from The First Medical Center of the Chinese PLA General Hospital (101 patients with HGG and 82 patients with SBM) and The Second People’s Hospital of Yibin (26 patients with SBM), covering patients who underwent surgery for intracranial gliomas and metastatic tumors from January 2016 to December 2023. The study was conducted in accordance with the Declaration of Helsinki (as revised in 2013). The study was approved by ethics board of The First Medical Center of the Chinese PLA General Hospital and The Second People’s Hospital of Yibin (No. 2022DZKY-072-01 and No. 2023-161-01), and informed consent was taken from all individual participants. The diagnosis of all patients was confirmed by pathological results. After screening, a total of 209 patients were included, and preoperative MRI image data were collected. Among them, 101 cases were HGG and 108 were SBM (Figure 1), including 60 males and 41 females for HGG, and 58 males and 50 females for SBM. The average age of patients with SBM was 58.21±9.00 years, while the average age for HGG patients was 50.5±12.22 years. It is important to note that gliomas were graded according to the World Health Organization (WHO) classification system. This system categorizes gliomas into four grades based on histological features, cellular atypia, and mitotic activity: Grade I (pilocytic astrocytoma, most benign), Grade II (low-grade gliomas), Grade III (anaplastic gliomas), and Grade IV (glioblastoma, most malignant). HGG in this study refer to WHO Grade III and IV gliomas, which are more aggressive and have a poorer prognosis compared to lower grades.
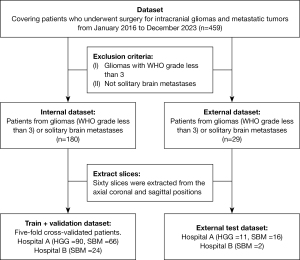
Segmentation
IWS software (version 1.0, MEDINSIGHT TECHNOLOGY CO. LTD., Shanghai, China) was used to view and classify MRI DICOM images. MRIConvert software (Version 2.1, University of Oregon, Eugene, USA) was used to convert DICOM images into NIFTI images. A senior radiologist (Q.L.) manually segmented tumors core and edema on T1CE images using ITK-Snap software (Version 3.8, University of Pennsylvania, Philadelphia, USA). Segmentation labels of tumor and edema were saved as masks.
Image preprocessing
Sixty multi-plane slices were extracted and collected for each patient, 20 slices from each axial, coronal, and sagittal view. Tumor core and edema as regions of interest (ROI) were extracted according to segmentation masks from the multi-plane T1CE slices. Considering that multi-plane slices have different dimensions, we padded the sides to 256×256 uniformly.
Deep learning models
We analyzed four mainstream CNN architectures: U-shaped network (U-Net), visual geometry group network (VGG), ResNet, and GoogLeNet. U-Net is the most popular neuro network used for medical images, characterized by its symmetric contraction and expansion paths, which helps capture more refined contextual information within images (15). The VGG network is renowned for its simplicity and efficiency, constructing deep networks through the repetitive use of small convolutional kernels (16). ResNet addresses the issue of vanishing gradients in deep networks by introducing residual connections. This allows the network to extract more complex features through deep learning (17). Because the Inception module effectively extracts features through parallel 1×1, 3×3, and 5×5 convolutional kernels (Figure 2), GoogLeNet can capture image features at various scales, which is particularly crucial for extracting key features from complex brain scan images (18).
Dataset split
To increase the number of images available for network training, we treated all multi-plane slices from each patient as independent images. This means that even if the slices come from the same patient, they are input into the network separately. This enhances data diversity and training effectiveness. The data split for 5-fold cross-validation was based on patients, not slices, in order to ensure that MRI slices from the same patient were grouped together. One hundred and eighty patients (10,800 slices) were used for training and validation, while 29 patients (1,740 slices) were used for testing. The model training of the core area and edema area also follows this data split.
Training parameters for models
In the training phase, MRI images were randomly enhanced to improve sharpness and contrast a probability of 0.5, aiming at enhancing the recognizability of details in the images and thus optimizing the quality of input data for model training (19). We set the batch size for training at 32 and used the stochastic gradient descent (SGD) optimizer to train for 100 epochs (20). We used an initial learning rate of 1e−5, chosen based on recommendations from prior literature and results from experimental tuning (18). Additionally, we adopted an adaptive learning rate adjustment strategy to optimize model convergence speed and accuracy. Cross-entropy was chosen as the loss function for training. All the models were trained on two NVIDIA Quadro RTX 6000 GPUs each with 24 GB of memory.
Evaluation metrics for model performance
In this study, we evaluated our models using a patient-based prediction method. Since both the training and prediction of the models were based on multi-plane slices, each slice during the training process was treated as an independent entity. Consequently, the models we trained can only provide slice-based prediction results. To generate predictions for individual patients, we used slice-voting method. We predicted each of the 60 slices for a single patient separately. If more than half of the slices were accurate, the patient was deemed accurately.
In order to evaluate the performance of models, we used accuracy (ACC) and area under the curve (AUC). Additionally, we selected sensitivity (SE) and specificity (SP) as supplementary evaluation metrics. Through the use of these comprehensive assessment methods, we were able to gain a more comprehensive understanding of the models’ performance in distinguishing HGG and SBM.
where ACC is the accuracy, TP is the true positive class, TN is the true negative class, FN is the false negative class and FP is the false positive class.
Results
Patient information
Utilizing independent sample t-tests (Table 1), we found significant differences in age between the SBM and HGG groups, suggesting clinically meaningful disparities in age distribution between the two groups. For patients with SBM, the lungs were the most common primary site (72 cases), followed by the breast and urinary system (both with 9 cases each), intestines (5 cases), and other sites collectively accounting for 6 cases. Among HGG patients, 22 had World Health Organization (WHO) Grade III tumors, while 86 had WHO Grade IV tumors. Additionally, we conducted independent sample t-tests for age among males and females in both groups, revealing significant age differences for both genders. This further underscores the potential role of gender in the age distribution of patients, which may influence treatment choices and prognoses for the two types of diseases.
Table 1
Parameter | Metastases | Gliomas | t-tests | P value |
---|---|---|---|---|
Age (years) (mean ± SD) | 58.21±9.00 | 50.50±12.22 | 4.917 | <0.001 |
Gender | ||||
Male | 58 | 60 | 4.582 | <0.001 |
Female | 50 | 41 | 2.341 | 0.02 |
Mean volume (mm3) | 38,009.03 | 35,891.56 | −0.482 | 0.63 |
SD, standard deviation.
Performance comparison of models
Model performance of four CNN models was compared using the same dataset (tumor core and edema) and training process (Table 2). For the U-Net network, the average cross-validation accuracy was 76.11%, with a sensitivity of 72.22% and a specificity of 80.00%. For the VGG network, the average cross-validation accuracy was 82.68%, with a sensitivity of 80.54% and a specificity of 84.44%. For the ResNet network, the average cross-validation accuracy was 83.78%, with a sensitivity of 86.24% and a specificity of 81.11%. However, GoogLeNet exhibited the highest performance with an average accuracy of 92.78% in cross-validation, a sensitivity of 95.55%, and a specificity of 90.00%. Additionally, we further tested GoogLeNet’s performance on axial images alone, where the average accuracy in cross-validation was 73.78%, sensitivity 62.22%, and specificity 85.38%, showing a decrease of 19.35% in accuracy compared to using multi-plane data.
Table 2
Models | 1-flod | 2-flod | 3-flod | 4-flod | 5-flod | Average |
---|---|---|---|---|---|---|
U-Net | ||||||
Accuracy, % | 83.33 | 77.78 | 75.00 | 63.89 | 80.56 | 76.11 |
Sensitivity, % | 88.89 | 77.78 | 66.67 | 55.56 | 72.22 | 72.22 |
Specificity, % | 77.78 | 77.78 | 83.33 | 72.22 | 88.89 | 80.00 |
VGG-16 | ||||||
Accuracy, % | 89.47 | 86.84 | 73.68 | 73.68 | 89.74 | 82.68 |
Sensitivity, % | 85.00 | 85.00 | 75.00 | 70.00 | 90.48 | 80.54 |
Specificity, % | 94.44 | 88.89 | 72.22 | 77.78 | 88.89 | 84.44 |
ResNet-50 | ||||||
Accuracy, % | 86.84 | 81.58 | 78.95 | 89.47 | 82.05 | 83.78 |
Sensitivity, % | 95.00 | 95.00 | 80.00 | 85.00 | 76.19 | 86.24 |
Specificity, % | 77.78 | 66.67 | 77.78 | 94.44 | 88.89 | 81.11 |
GoogLeNet Axial | ||||||
Accuracy, % | 77.14 | 74.29 | 71.43 | 74.29 | 71.79 | 73.78 |
Sensitivity, % | 66.67 | 77.78 | 50.00 | 61.11 | 55.56 | 62.22 |
Specificity, % | 88.24 | 70.59 | 94.12 | 88.24 | 85.71 | 85.38 |
GoogLeNet | ||||||
Accuracy, % | 86.11 | 94.44 | 97.22 | 94.44 | 91.67 | 92.78 |
Sensitivity, % | 88.89 | 99.99 | 99.99 | 99.99 | 88.89 | 95.55 |
Specificity, % | 83.33 | 88.89 | 94.44 | 88.89 | 94.44 | 90.00 |
VGG, visual geometry group network.
A heat map visualization technique was adopted in the model training process of GoogLeNet. When it made predictions, the model successfully focused on tumor core and peritumoral edema in all axial, coronal, and sagittal slices (Figure 3). The heatmaps in these slices used brighter colors to highlight these critical areas, provided a visual representation of the model’s focus during diagnostic assessments. This not only aided in understanding the model’s decision-making process but also offered an intuitive means to verify the accuracy and reliability of its predictions.
GoogLeNet performance on tumor core and peritumoral edema
Based on GoogleNet’s exceptional discriminative ability, we used the same training method to differentiate HGG and SBM using tumoral core and peritumoral edema regions (Figure 4), separately. On the peritumoral edema region, the average cross-validation accuracy was 90.00%, with a sensitivity of 93.33% and a specificity of 86.66%. On the tumoral core region, the average cross-validation accuracy was 91.11%, with a sensitivity of 87.78% and a specificity of 95.55%. Additionally, an external dataset was used to test GoogLeNet’s discriminative performance in regions of the tumoral core, edema, and overall. On the peritumoral edema region, accuracy was 79.31%, with an AUC of 0.826 [95% confidential interval (CI): 0.656–0.971), a sensitivity of 81.82%, and a specificity of 77.78%. On the tumoral core region, accuracy was 86.76%, with an AUC of 0.866 (95% CI: 0.722–0.991), a sensitivity of 81.82%, and a specificity of 83.33%. The model still performed best in the overall region, with an accuracy of 89.66%, an AUC of 0.939 (95% CI: 0.842–1.000), a sensitivity of 90.91%, and a specificity of 83.33%. Furthermore, the normalized confusion matrix for the overall region showed a prediction accuracy of 0.91 for HGG and 0.83 for SBM (Figure 5).
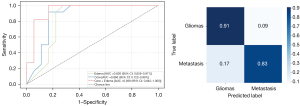
Discussion
In clinical settings, MRI images are indispensable, particularly for neurological examinations. Their rich lesion information, coupled with the power of CNN, offer an optimal solution for medical image classification and predictive tasks (21). In this study, we tested U-Net, VGG, ResNet, and GoogLeNet using T1CE images. All models could achieve differential diagnosis of HGG and SBM. With the same training images and training process, the performance of the GoogLeNet outperformed the other three models (Table 2). It showed that GoogLeNet could extract more information on T1CE images when making diagnostic predictions between HGG and SBM. Additionally, using single-modal rather than multimodal imaging reduces costs and simplifies clinical procedures, making this method more suitable for rapid clinical decision-making.
Studies published for distinguishing between HGG and SBM using traditional machine-learning approaches often involve extracting numerous radiomic features and testing various classifiers (12,22). The prediction accuracy ranges from 64.00% to 83.00 (8,9). On the deep learning frontier, Shin et al. (10) utilized T1-weighted imaging (T1WI) and T2-weighted imaging (T2WI) images as input and employed the ResNet50 to distinguish between gliomas and metastatic tumors, achieving an internal test set accuracy of 89.00% and an external test set accuracy of 85.90%. Tariciotti et al. (13) leveraged the ResNet-101 to discern among gliomas, metastatic tumors, and lymphomas based on T1Gd images, recording accuracy rates of 80.37% for gliomas and metastatic tumors, respectively. Yan et al. (14) employed ResNest-18 as a classification model and, when combined with diffusion-weighted imaging on T1WI and T2WI MRI images, exhibited the best differentiation results between HGG and SBM, with a validation set accuracy of 88.50% and an external test set accuracy of 80.70%. Bathla et al. (23) used 3D CNNs to distinguish between glioblastoma and brain metastases by combining images from four different sequences, achieving an average accuracy of 87.50% on internal data and 85.58% on an external test set. When compared to previous studies, our GoogLeNet model exhibits stellar performance. It demonstrated an average accuracy of 92.78% on cross-validation and an average accuracy of 89.66% on the test set (Table 3). The reasons we speculated are as follows: (I) compared to traditional machine learning models, GoogLeNet can extract a more extensive array of feature information automatically. (II) Compared to deep learning models using multimodal imaging, GoogLeNet using axial T1CE images is at a disadvantage (25). However, using single-modal multi-plane T1CE images allows GoogLeNet to surpass multimodal models’ performance. It indicates that multi-plane images can serve as multi-modal images and be more cost-effectiveness. (III) Due to the Inception module, GoogLeNet can flexibly capture multi-level features, and extract rich tumor imaging information from deeper network (26,27). Despite the superior performance demonstrated by GoogLeNet in our internal dataset, significant discrepancies were observed when transitioning to external datasets, highlighting potential issues with the model’s robustness across different imaging conditions and patient demographics. Variations in image quality, the diversity of the external dataset, and differences in tumor characteristics could contribute to these performance gaps.
Table 3
Author | Number of patients | Method | Used radiomics features | Used multimodal images | Internal ACC (%) | External ACC (%) |
---|---|---|---|---|---|---|
Dong et al. (7) | 120 | Combine five classifiers | Y | Y | 64.00 | – |
Artzi et al. (8) | 439 | SVM | Y | Y | 85.00 | – |
Qian et al. (9) | 412 | SVM + LASSO | Y | Y | 83.00 | – |
Bae et al. (24) | 248 | LDA + LASSO | Y | Y | 81.70 | – |
Shin et al. (10) | 741 | ResNet-50 | N | Y | 89.00 | 85.90 |
Tariciotti et al. (13) | 126 | ResNet-101 | N | N | 80.37 | – |
Yan et al. (14) | 234 | ResNet-18 | N | Y | 88.50 | 80.70 |
Bathla et al. (23) | 366 | 3D CNN | N | Y | 87.50 | 85.58 |
Ours | 209 | GoogLeNet | N | N | 92.78 | 89.66 |
ACC, accuracy; SVM, support vector machine; LASSO, least absolute shrinkage and selection operator; LDA, latent dirichlet allocations; CNN, convolutional neural network; Y, yes; N, no.
Thin-slice T1CE images were collected and used for model training in this study. The reasons are as follows: (I) thin-slice T1CE images can be reconstructed into multi-plane slices (coronal and sagittal slices), which provides more structural and texture tumor information (28). (II) With contrast injection, the tumor core is enhanced significantly, which helps distinguish the tumor core and edema clearly. (III) A model trained with multi-plane slices has better compatibility for axial, coronal, and sagittal T1CE images.
Both HGG and SBM have enhanced tumor core and significant peritumoral edema on T1CE images. The core region is a primary concentration of tumor cells and necrosis, featuring high cell density and compact cell arrangement, emphasizing the morphological characteristics of the tumor (29). Conversely, the edema region mainly reflects tissue edema levels and inflammatory responses, with low cell density and a relatively loose arrangement (30). For HGG, the edema region contains infiltrating tumor cells; for metastatic tumors, the edema region typically lacks tumor cells. Histologically, edematous areas also aid in the differential diagnosis of tumors, although this distinction is not detectable to the human eye in MRI images (24,31). To investigate if deep learning models could capture histological features of tumor core and edema areas in MRI images for differential diagnosis, we trained the GoogLeNet model using tumor core and edema region separately. The GoogLeNet model achieved over 90% prediction accuracy using either tumor core or edema areas. These findings suggest that a deep learning model can extract features at the histological level from MRI images to aid in the differential diagnosis of tumors. This approach might opens up new avenues for predicting tumor pathological type using deep learning methods.
While our deep learning models demonstrate remarkable performance, there are certain limitations inherent to this study. The model was initially constructed based on three distinct MRI scanners used in two centers, which might affect its reliability across diverse MRI scanners and patient populations from multiple centers. Although GoogLeNet and other classic models excel in processing single-modal images, they are less efficient at handling multimodal MRI images and clinical features, potentially restricting their applicability in clinical practice. Considering these limitations, future research involving models equipped with attention mechanisms or the latest deep learning frameworks promises to address these issues. Such advancements would not only mitigate the limitations posed by the use of single-modal image datasets but also enhance performance and applicability in complex clinical settings.
Conclusions
We investigated the capability of T1CE images in deep learning models to differentiate HGG from SBM. According to this study, the GoogLeNet model demonstrated a higher level of diagnostic accuracy compared to other CNN models and previously reported methods. It achieved an average patient accuracy of 92.78% in a five-fold cross-validation. Moreover, on an external test set, it reached an accuracy of 89.66%. It also achieved a high prediction accuracy solely using tumor core or edema region for differentiation between HGG and SBM. This approach provides patients with robust preoperative and non-invasive diagnostic support for fast and initial screening for HGG and SBM.
Acknowledgments
We thank Yiyang Zhao and Guodong Yang for technical support in this research.
Funding: This work was supported by
Footnote
Reporting Checklist: The authors have completed the TRIPOD reporting checklist. Available at https://qims.amegroups.com/article/view/10.21037/qims-24-380/rc
Conflicts of Interest: All authors have completed the ICMJE uniform disclosure form (available at https://qims.amegroups.com/article/view/10.21037/qims-24-380/coif). Z.X. and S.C. both disclosed the funding from the National Natural Science Foundation of China (No. 62102133), the High-level and Urgently Needed Overseas Talent Programs of Jiangxi Province (No. 20232BCJ25026), the Natural Science Foundation of Henan Province (No. 242300421402), the Kaifeng Major Science and Technology Project (No. 21ZD011), the Ji’an Finance and Science Foundation (Nos. 20211-085454, 20222-151746, 20222-151704), Ji’an Key Core Common Technology “Reveal The List” Project (No. 2022-1). Z.X. also reports the funding from the Henan University Graduate Student Excellence Program (No. SYLYC2023135). X.S. discloses the funding from the Hospital Management Project (No. 2023LCYYQH025). The other authors have no conflicts of interest to declare.
Ethical Statement: The authors are accountable for all aspects of the work in ensuring that questions related to the accuracy or integrity of any part of the work are appropriately investigated and resolved. The study was conducted in accordance with the Declaration of Helsinki (as revised in 2013). The study was approved by ethics board of The First Medical Center of the Chinese PLA General Hospital and The Second People’s Hospital of Yibin (No. 2022DZKY-072-01 and No. 2023-161-01), and informed consent was taken from all individual participants.
Open Access Statement: This is an Open Access article distributed in accordance with the Creative Commons Attribution-NonCommercial-NoDerivs 4.0 International License (CC BY-NC-ND 4.0), which permits the non-commercial replication and distribution of the article with the strict proviso that no changes or edits are made and the original work is properly cited (including links to both the formal publication through the relevant DOI and the license). See: https://creativecommons.org/licenses/by-nc-nd/4.0/.
References
- Yao M, Li S, Wu X, Diao S, Zhang G, He H, Bian L, Lu Y. Cellular origin of glioblastoma and its implication in precision therapy. Cell Mol Immunol 2018;15:737-9. [Crossref] [PubMed]
- Guan X. Cancer metastases: challenges and opportunities. Acta Pharm Sin B 2015;5:402-18. [Crossref] [PubMed]
- Weller M, van den Bent M, Tonn JC, Stupp R, Preusser M, Cohen-Jonathan-Moyal E, et al. European Association for Neuro-Oncology (EANO) guideline on the diagnosis and treatment of adult astrocytic and oligodendroglial gliomas. Lancet Oncol 2017;18:e315-29. [Crossref] [PubMed]
- Suh JH, Kotecha R, Chao ST, Ahluwalia MS, Sahgal A, Chang EL. Current approaches to the management of brain metastases. Nat Rev Clin Oncol 2020;17:279-99. [Crossref] [PubMed]
- Jiang B, Chaichana K, Veeravagu A, Chang SD, Black KL, Patil CG. Biopsy versus resection for the management of low-grade gliomas. Cochrane Database Syst Rev 2017;4:CD009319. [Crossref] [PubMed]
- Caulo M, Panara V, Tortora D, Mattei PA, Briganti C, Pravatà E, Salice S, Cotroneo AR, Tartaro A. Data-driven grading of brain gliomas: a multiparametric MR imaging study. Radiology 2014;272:494-503. [Crossref] [PubMed]
- Dong F, Li Q, Jiang B, Zhu X, Zeng Q, Huang P, Chen S, Zhang M. Differentiation of supratentorial single brain metastasis and glioblastoma by using peri-enhancing oedema region-derived radiomic features and multiple classifiers. Eur Radiol 2020;30:3015-22. [Crossref] [PubMed]
- Artzi M, Bressler I, Ben Bashat D. Differentiation between glioblastoma, brain metastasis and subtypes using radiomics analysis. J Magn Reson Imaging 2019;50:519-28. [Crossref] [PubMed]
- Qian Z, Li Y, Wang Y, Li L, Li R, Wang K, Li S, Tang K, Zhang C, Fan X, Chen B, Li W. Differentiation of glioblastoma from solitary brain metastases using radiomic machine-learning classifiers. Cancer Lett 2019;451:128-35. [Crossref] [PubMed]
- Shin I, Kim H, Ahn SS, Sohn B, Bae S, Park JE, Kim HS, Lee SK. Development and Validation of a Deep Learning-Based Model to Distinguish Glioblastoma from Solitary Brain Metastasis Using Conventional MR Images. AJNR Am J Neuroradiol 2021;42:838-44. [Crossref] [PubMed]
- Talo M, Yildirim O, Baloglu UB, Aydin G, Acharya UR. Convolutional neural networks for multi-class brain disease detection using MRI images. Comput Med Imaging Graph 2019;78:101673. [Crossref] [PubMed]
- Afshar P, Mohammadi A, Plataniotis KN, Oikonomou A, Benali H. From handcrafted to deep-learning-based cancer radiomics: challenges and opportunities. EEE Signal Processing Magazine 2019;36:132-60. [Crossref] [PubMed]
- Tariciotti L, Caccavella VM, Fiore G, Schisano L, Carrabba G, Borsa S, Giordano M, Palmisciano P, Remoli G, Remore LG, Pluderi M, Caroli M, Conte G, Triulzi F, Locatelli M, Bertani G. A Deep Learning Model for Preoperative Differentiation of Glioblastoma, Brain Metastasis and Primary Central Nervous System Lymphoma: A Pilot Study. Front Oncol 2022;12:816638. [Crossref] [PubMed]
- Yan Q, Li F, Cui Y, Wang Y, Wang X, Jia W, Liu X, Li Y, Chang H, Shi F, Xia Y, Zhou Q, Zeng Q. Discrimination Between Glioblastoma and Solitary Brain Metastasis Using Conventional MRI and Diffusion-Weighted Imaging Based on a Deep Learning Algorithm. J Digit Imaging 2023;36:1480-8. [Crossref] [PubMed]
- Szegedy C, Liu W, Jia Y, Sermanet P, Reed S, Anguelov D, Erhan D, Vanhoucke V, Rabinovich A. Going deeper with convolutions. 2015 IEEE Conference on Computer Vision and Pattern Recognition (CVPR), Boston, MA, USA, 2015:1-9.
- Ronneberger O, Fischer P, Brox T. U-Net: Convolutional Networks for Biomedical Image Segmentation. In: Navab N, Hornegger J, Wells W, Frangi A. editors. Medical Image Computing and Computer-Assisted Intervention – MICCAI 2015. Lecture Notes in Computer Science, Springer, 2015;9351:234-41.
- He K, Zhang X, Ren S, Sun J. Deep residual learning for image recognition. 2016 IEEE Conference on Computer Vision and Pattern Recognition (CVPR), Las Vegas, NV, USA, 2016:770-8.
- Simonyan K, Zisserman A. Very deep convolutional networks for large-scale image recognition. arXiv preprint arXiv: 1409.1556, 2014.
- Goceri E. Medical image data augmentation: techniques, comparisons and interpretations. Artif Intell Rev 2023; Epub ahead of print. [Crossref]
- Robbins H, Monro S. A stochastic approximation method. Annals of Mathematical Statistics 1951;22:400-7.
- Jiang Y, Yang M, Wang S, Li X, Sun Y. Emerging role of deep learning-based artificial intelligence in tumor pathology. Cancer Commun (Lond) 2020;40:154-66. [Crossref] [PubMed]
- Lotan E, Jain R, Razavian N, Fatterpekar GM, Lui YW. State of the Art: Machine Learning Applications in Glioma Imaging. AJR Am J Roentgenol 2019;212:26-37. [Crossref] [PubMed]
- Bathla G, Dhruba DD, Liu Y, Le NH, Soni N, Zhang H, Mohan S, Roberts-Wolfe D, Rathore S, Sonka M, Priya S, Agarwal A. Differentiation Between Glioblastoma and Metastatic Disease on Conventional MRI Imaging Using 3D-Convolutional Neural Networks: Model Development and Validation. Acad Radiol 2024;31:2041-9. [Crossref] [PubMed]
- Bae S, An C, Ahn SS, Kim H, Han K, Kim SW, Park JE, Kim HS, Lee SK. Robust performance of deep learning for distinguishing glioblastoma from single brain metastasis using radiomic features: model development and validation. Sci Rep 2020;10:12110. [Crossref] [PubMed]
- Razzak M I, Naz S, Zaib A. Deep Learning for Medical Image Processing: Overview, Challenges and the Future. In: Dey N, Ashour A, Borra S. editors. Classification in BioApps. Lecture Notes in Computational Vision and Biomechanics, Springer, 2018;26:323-50.
- Yang X, Yeo SY, Hong JM, Wong ST, Tang WT, Wu ZZ, Lee G, Chen S, Ding V, Pang B, Choo A, Su Y. A deep learning approach for tumor tissue image classification. IASTED Biomedical Engineering 2016. doi:
10.2316/P.2016.832-025 . - Yamashita R, Nishio M, Do RKG, Togashi K. Convolutional neural networks: an overview and application in radiology. Insights Imaging 2018;9:611-29. [Crossref] [PubMed]
- Candemir S, Nguyen XV, Folio LR, Prevedello LM. Training Strategies for Radiology Deep Learning Models in Data-limited Scenarios. Radiol Artif Intell 2021;3:e210014. [Crossref] [PubMed]
- Bastola S, Pavlyukov MS, Yamashita D, Ghosh S, Cho H, Kagaya N, et al. Glioma-initiating cells at tumor edge gain signals from tumor core cells to promote their malignancy. Nat Commun 2020;11:4660. [Crossref] [PubMed]
- Baris MM, Celik AO, Gezer NS, Ada E. Role of mass effect, tumor volume and peritumoral edema volume in the differential diagnosis of primary brain tumor and metastasis. Clin Neurol Neurosurg 2016;148:67-71. [Crossref] [PubMed]
- Tateishi M, Nakaura T, Kitajima M, Uetani H, Nakagawa M, Inoue T, Kuroda JI, Mukasa A, Yamashita Y. An initial experience of machine learning based on multi-sequence texture parameters in magnetic resonance imaging to differentiate glioblastoma from brain metastases. J Neurol Sci 2020;410:116514. [Crossref] [PubMed]