Computed tomography (CT)-based skeletal muscle vertebral-related index to assess low muscle mass in patients with non-small cell lung cancer
Introduction
Skeletal muscles perform a critical function in numerous aspects of the human body, such as movement, posture maintenance, body support, regulation of temperature, and metabolic activities. Numerous studies have investigated the relationship between skeletal muscles and aspects of physical health, aging, the incidence of various diseases, and mortality rates (1,2). The European Working Group on Sarcopenia in Older People 2 (EWGSOP2) describes sarcopenia as a progressive and generalized skeletal muscle disorder associated with an increased likelihood of adverse outcomes including falls, fractures, physical disabilities, and mortality (3).
Recently, there has been considerable attention from numerous researchers on the relationship between sarcopenia and cancer. Several studies have investigated the relationship between lung cancer and sarcopenia, with consistent findings that sarcopenia is a poor prognostic factor for lung cancer (4,5). The presence of sarcopenia has been found to be a strong prognostic factor for a 2-fold increased risk of death in lung cancer patients, with some studies reporting a 3-fold increased risk in multivariate analysis (6). Sarcopenia is also associated with increased postoperative complications and decreased disease control rates with immune checkpoint inhibitors in patients with non-small cell lung cancer (NSCLC) (7). Overall, the evidence consistently suggests that sarcopenia is a significant factor in the prognosis and treatment of lung cancer, and further research is necessary to clarify the pathophysiology of sarcopenia and develop effective interventions for patients with lung cancer. Sarcopenia diagnosis is confirmed when low muscle strength and low muscle mass are present (3). Low muscle mass is considered an emerging biomarker for the prognosis of lung cancer patients (8), offering valuable prognostic information beyond traditional risk factors. With the growing recognition of muscle importance, various imaging methods have been introduced to assess muscle quantity.
Computed tomography (CT) is considered one of the gold standard non-invasive methods for assessing muscle mass (9,10). The measurement of skeletal muscle area (SMA) at the third lumbar vertebra (L3) level on a single axial CT image can provide an accurate estimation of the total skeletal muscle mass in patients (11). Muscle mass measurements based on CT imaging typically require standardization according to patient-specific body size parameters, such as height. This standardization is used, for instance, in calculations such as the skeletal muscle index (SMI) and the psoas muscle index (12,13). Typically, a patient’s height should be recorded in the medical electronic record. However, the assessment of sarcopenia has only begun to receive clinical attention in recent years. Affected by the differences in the electronic medical records systems and the standardization of medical document records in various countries and medical institutions, some SMI data has inevitably been missing in retrospective studies (14). This makes their muscle mass difficult to assess, even though CT image-based SMA measurements are available. In the field of forensic medicine, various attempts have been made to estimate height from available alternative measurements and imaging, such as long bones and the sacrum (15-17). Additionally, methods based on spinal parameters derived from CT images have been proposed for height estimation (18,19), and studies have also used bone as a standardized reference (20). Fortunately, patients undergoing CT scans can conveniently acquire both SMA and multiple 2-dimensional (2D) vertebral parameters at the L3 level. However, the feasibility of using standardized SMA based on vertebral 2D parameters (called the skeletal muscle vertebral related index, SMVI) remains unclear, and it is also unknown which vertebral parameter performs optimally in this regard.
Therefore, the aim of this retrospective study was to explore the feasibility of SMVI in the assessment of low muscle mass and to observe the relationship between SMVI and the overall survival (OS) in patients with NSCLC. We present this article in accordance with the STARD reporting checklist (available at https://qims.amegroups.com/article/view/10.21037/qims-24-120/rc).
Methods
Participants
We performed a retrospective study of consecutive NSCLC patients who underwent CT between January 2010 and December 2019 at the First Affiliated Hospital of Kunming Medical University. We collected data on sex, age, height, weight, body mass index (BMI), smoking status, histological subtypes, and tumor-node-metastasis (TNM) stage. All patients received surgical treatment and had histologically confirmed NSCLC. The exclusion criteria were as follows: (I) incomplete clinical information; (II) history of spinal instrumentation surgery; (III) second primary tumor; (IV) spinal infection; (V) lack of available CT images at the L3; and (VI) inadequate image quality or inability to measurement. A total of 433 patients were included in the final analysis (Figure 1). Height data were unavailable for 165 patients. The study was conducted following the Declaration of Helsinki (as revised in 2013). Approval for this study was obtained from the Ethics Committee of the First Affiliated Hospital of Kunming Medical University (No. 2022L305) and the requirement for individual consent for this retrospective analysis was waived.
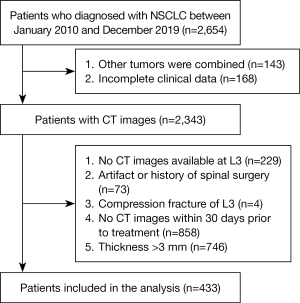
CT imaging and skeletal muscle measurement
All CT scans were obtained within 1 month before treatment (Somatom Definition AS; Siemens, Erlangen, Germany) in this study (21). The scanning parameters were as follows: slice thickness, 1 or 3 mm; section spacing, 1 or 3 mm; tube voltage, 100 kVp; tube current, 500 mAs. For each participant, a single axial CT image at the L3 level was analyzed to measure the area of skeletal muscle and vertebral body. The CT images were analyzed using a commercially available body composition analysis software platform (Slice-O-Matic V5.0; TomoVision, Magog, Canada). CT values ranging from −29 to +150 Hounsfield units (HU) were used to identify muscle and −29 to 1,000 HU for vertebral body (22). To account for variations in SM due to differences in patient size, including the SMI, which was obtained by dividing the SMA by the square of height in meters, as per the following calculation formula: SMI = SMA/h2. The SMI threshold for diagnosing low muscle mass in sarcopenia varies across gender. Male patients with an SMI <40.3 cm2/m2 and female patients with an SMI <30.8 cm2/m2 were diagnosed with low muscle mass (23).
Assessment of SMVI
CT images were transferred to a picture archiving and communication system (PACS; Neusoft, Shenyang, China) and were measured in this workstation. The transverse vertebral diameter (TVD), longitudinal vertebral diameter (LVD), and area of vertebral body (VBA) at the center cross-section were measured at the L3 level. Besides, the vertebral height (VH) of L3 was measured at the central sagittal level of lumbar vertebrae. Additionally, standardization of SMA was performed using 2D vertebral parameters (SMVI), calculated by the following formulas: skeletal muscle vertebral ratio (SMVR) = SMA/VBA; skeletal muscle transverse vertebral diameter index (SMTVDI) = SMA/TVD2; skeletal muscle longitudinal vertebral diameter index (SMLVDI) = SMA/LVD2; and skeletal muscle vertebral height index (SMVHI) = SMA/VH2. All measurements were performed by a trained radiology researcher (FY) and reviewed by a musculoskeletal radiologist (ZGZ) with more than 13 years of experience, who were blinded to the patients’ clinical data, SMI, and outcomes. The CT image segmentation process of SMA, VBA, TVD, LVD, and VH is shown in Figure 2.
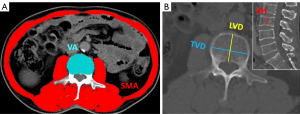
Patient outcomes
The primary outcome was OS, defined as the time from the date of first surgery to the date of death. The date of death was determined through telephone follow-up and electronic medical record system searches.
Statistical analysis
Continuous variables were reported as mean ± standard deviation (SD), and categorical variables were presented as frequencies with percentages. Normality of continuous variables was assessed by the Shapiro-Wilk test. A sample size was calculated using GPower 3.1. The estimated sample size was 48 per group with total sample size of 96. Continuous variables with normal distribution were analyzed usingindependent t-test, continuous variables with non-normal distribution subjected to Mann-Whitney U test. Categorical variables were compared using the chi-square test. Correlation between SMI and SMVI were analyzed by Pearson’s coefficient. Diagnostic performance of SMVI was measured by receiver operating characteristic (ROC) curve and area under ROC curve (AUC). Kaplan-Meier curves and log rank test were used for survival analysis. Statistical analyzes were calculated using R software (version 4.2.2; R Foundation for Statistical Computing, Vienna, Austria). A P value of less than 0.05 (P<0.05) was considered significant.
Results
Demographic and clinical characteristics of the study sample
A total of 433 patients (191 male and 242 female) with available CT images were enrolled in the study, with a mean age of 59.74±11.04 years and a mean BMI of 22.76±3.15 kg/m2. The comparison of the baseline characteristics between different muscle mass groups is shown in Table 1. There was a significant difference between the high and low muscle mass groups for the age, male proportion, weight, BMI, OS, and histological subtypes (all P<0.05). SMI, SMVR, SMTVDI, SMLDVI, and SMVHI in the low muscle mass group were significantly lower than those in the high muscle mass group (all P<0.001). As shown in Table 2, significant differences in the SMI, SMVR, SMTVDI, SMLDVI, and SMVHI across the high and low muscle mass groups were detected in a subgroup analysis based on gender. Regardless of the high or low muscle mass groups, the SMI, SMVR, SMTVDI, SMLDVI, and SMVHI were higher in male patients than in female patients (all P<0.001).
Table 1
Characteristic | Total (n=433) | High muscle mass (n=302) | Low muscle mass (n=131) | P value |
---|---|---|---|---|
Age, years | 59.74±11.04 | 58.57±10.31 | 62.44±12.18 | 0.002* |
Height, m | 1.62±0.07 | 1.62±0.07 | 1.63±0.07 | 0.301 |
Weight, kg | 60.10±10.46 | 61.61±10.52 | 56.60±9.46 | <0.001* |
BMI, kg/m2 | 22.76±3.15 | 23.47±3.09 | 21.12±2.63 | <0.001* |
SMI, cm2/m2 | 38.65±8.38 | 41.75±7.47 | 31.51±5.53 | <0.001* |
SMVR, cm2/cm2 | 9.16±1.91 | 9.84±1.69 | 7.60±1.40 | <0.001* |
SMTVDI, cm2/cm2 | 5.61±1.18 | 6.03±1.06 | 4.64±0.82 | <0.001* |
SMLVDI, cm2/cm2 | 10.64±2.45 | 11.44±2.26 | 8.80±1.77 | <0.001* |
SMVHI, cm2/cm2 | 21.17±7.16 | 22.94±7.24 | 17.10±4.98 | <0.001* |
Overall survival, days | 1,270.98±840.24 | 1,357.44±870.92 | 1,071.66±729.72 | 0.001* |
Sex, n (%) | 0.031* | |||
Male | 191 (44.11) | 123 (40.73) | 68 (51.91) | |
Female | 242 (55.89) | 179 (59.27) | 63 (48.09) | |
Smoking status, n (%) | 0.169 | |||
No | 357 (82.45) | 254 (84.11) | 103 (78.63) | |
Yes | 76 (17.55) | 48 (15.89) | 28 (21.37) | |
Histological subtypes, n (%) | <0.001* | |||
Adenocarcinoma | 355 (81.99) | 253 (83.77) | 102 (77.86) | |
Squamous cell carcinoma | 18 (4.16) | 5 (1.66) | 13 (9.92) | |
NSCLC | 60 (13.86) | 44 (14.57) | 16 (12.21) | |
TNM stage, n (%) | 0.883 | |||
I | 325 (75.06) | 228 (75.50) | 97 (74.05) | |
II | 53 (12.24) | 35 (11.59) | 18 (13.74) | |
III | 28 (6.47) | 19 (6.29) | 9 (6.87) | |
IV | 27 (6.24) | 20 (6.62) | 7 (5.34) |
Continuous variables are presented as mean ± standard deviation. Categorical parameters are presented as number (frequency). *, P<0.05. BMI, body mass index; SMI, skeletal muscle index; SMVR, skeletal muscle vertebral ratio; SMTVDI, skeletal muscle transverse vertebral diameter index; SMLVDI, skeletal muscle longitudinal vertebral diameter index; SMVHI, skeletal muscle vertebral height index; NSCLC, non-small cell lung cancer; TNM, tumor-node-metastases.
Table 2
Characteristic | Male | Female | |||||||
---|---|---|---|---|---|---|---|---|---|
Total (n=191) | High muscle mass (n=123) | Low muscle mass (n=68) | P | Total (n=242) | High muscle mass (n=179) | Low muscle mass (n=63) | P | ||
SMI, cm2/m2 | 43.65±8.02 | 48.20±5.61 | 35.44±4.34 | <0.001* | 34.70±6.31 | 37.32±4.94 | 27.27±2.94 | <0.001* | |
SMVR, cm2/cm2 | 9.89±2.02 | 10.87±1.60 | 8.12±1.38 | <0.001* | 8.59±1.62 | 9.14±1.37 | 7.03±1.19 | <0.001* | |
SMTVDI, cm2/cm2 | 5.98±1.29 | 6.59±1.06 | 4.87±0.86 | <0.001* | 5.32±1.01 | 5.65±0.89 | 4.40±0.72 | <0.001* | |
SMLVDI, cm2/cm2 | 11.45±2.68 | 12.66±2.25 | 9.25±1.86 | <0.001* | 10.01±2.04 | 10.61±1.86 | 8.31±1.54 | <0.001* | |
SMVHI, cm2/cm2 | 23.73±8.29 | 26.57±8.19 | 18.59±5.56 | <0.001* | 19.15±5.33 | 20.44±5.22 | 15.49±3.69 | <0.001* |
Continuous variables are presented as mean ± standard deviation. *, P<0.05. SMI, skeletal muscle index; SMVR, skeletal muscle vertebral ratio; SMTVDI, skeletal muscle transverse vertebral diameter index; SMLVDI, skeletal muscle longitudinal vertebral diameter index; SMVHI, skeletal muscle vertebral height index.
Correlation between SMI and SMVI
The heatmap shows the correlation analysis between SMI, SMVR, SMTVDI, SMLDVI, and SMVHI (Figure 3). The SMVR was highly positively correlated with the SMI, and the Pearson correlation coefficient was 0.83 (P<0.001; Figure 3A). Besides, SMTVDI, SMLDVI, and SMVHI had moderately positive correlation with the SMI (r=0.76, 0.75, and 0.67, respectively; all P<0.001) (Figure 3B-3D). In addition, highly and moderately positive correlations were observed in different SMVI parameters (P<0.001).
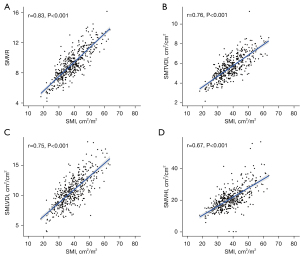
SMVI and low muscle mass
Under the diagnostic criteria by SMI, in the male and female patients, the AUC of the SMVR for the diagnosis of low muscle mass was 0.902 and 0.892, the cutoff value was 8.86 and 8.10 cm2/cm2, the specificity was 89.4% and 77.7%, and the sensitivity was 75.0% and 87.3%, respectively. A similar diagnostic performance was observed for the SMTVDI and SMLDVI in both males and females. The DeLong test indicated an insignificant difference between AUCs of SMVR, SMTVDI, and SMLDVI (P>0.05); the SMVHI had the lowest AUC in the male and female patients, and the differences with other SMVIs were statistically significant (P<0.05; Table 3, Figure 4).
Table 3
Characteristic | Cut-off value | Specificity | Sensitivity | AUC (95% CI) |
---|---|---|---|---|
Male | ||||
SMVR, cm2/cm2 | 8.86 | 0.894 | 0.750 | 0.902 (0.856–0.947) |
SMTVDI, cm2/cm2 | 5.78 | 0.756 | 0.882 | 0.905 (0.864–0.946) |
SMLVDI, cm2/cm2 | 11.22 | 0.724 | 0.882 | 0.881 (0.834–0.928) |
SMVHI, cm2/cm2 | 23.12 | 0.691 | 0.841 | 0.823* (0.763–0.88) |
Female | ||||
SMVR, cm2/cm2 | 8.10 | 0.777 | 0.873 | 0.892 (0.848–0.937) |
SMTVDI, cm2/cm2 | 4.99 | 0.771 | 0.825 | 0.866 (0.816–0.916) |
SMLVDI, cm2/cm2 | 9.00 | 0.816 | 0.730 | 0.837 (0.781–0.893) |
SMVHI, cm2/cm2 | 18.51 | 0.670 | 0.841 | 0.803* (0.742–0.864) |
*, P<0.05. AUC, area under the curve; CI, confidence interval; SMVR, skeletal muscle vertebral ratio; SMTVDI, skeletal muscle transverse vertebral diameter index; SMLVDI, skeletal muscle longitudinal vertebral diameter index; SMVHI, skeletal muscle vertebral height index.
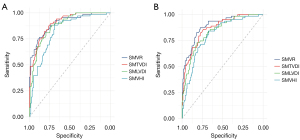
SMVI and OS
We used the cutoff value of SMVR, SMTVDI, SMLDVI, and SMVHI to diagnose low muscle mass and observe differences in survival probability based on SMVIs classification. There was a significant difference in OS for patients with low SMI compared to high SMI (P=0.0082; Figure 5A). Patients in the low SMVR, SMTVDI, or SMLDVI groups had a shorter OS (P=0.0024, 0.003, 0.0018, respectively; Figure 5B-5D). There was no significant difference in OS between patients with low and high SMVHI using the cutoff value of SMVHI <23.12 cm2/cm2 in the male and SMVHI <18.51 cm2/cm2 in the female (P=0.28; Figure 5E).
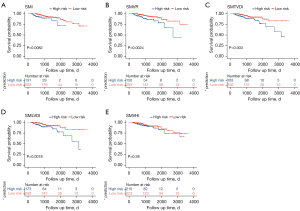
Discussion
To address the potential absence of SMI in clinical practice and retrospective studies, this study standardized SMA using 2D vertebral measurements from CT images at the L3 level for the first time. We explored the feasibility of SMVIs as a reasonable substitute for SMI in NSCLC patients. Besides, we evaluated the diagnostic performance of the SMVIs in patients with low muscle mass and their relationship with patient prognosis. We found there was a positive correlation between SMI and SMVRs at the L3 level. The SMVIs effectively distinguished low muscle mass and were associated with the OS of NSCLC patients.
When SMI data derived from CT or magnetic resonance imaging (MRI) is unavailable, researchers may be able to refer to existing assessment of dual-energy X-ray absorptiometry and bioelectrical impedance analysis. This strategy still might not address all the issues when the patient has only had a CT scan. This study was not aimed at highlighting the limitations of SMI and seeking new alternative indicators in the routine clinical practice. However, how to generate from existing CT images a new indicator that can assess low muscle mass is meaningful, especially in retrospective studies. Firstly, by adopting previously reported SMI cutoff values indicative of low muscle mass in Asian populations (23), all 4 SMVI parameters showed a significant decrease in patients classified within the low muscle mass group, mirroring the outcomes observed with SMI. Furthermore, this result remained consistent regardless of gender influence. In forensic studies, skeletal dimensions are closely associated with height, such as long bones and vertebrae (18,24). A study conducted on a Spanish population sample found that the femur was the most accurate predictor of stature in males (R=0.851), whereas the tibia was the best predictor in females (R=0.876) (24). In our study, we compared the correlation between SMVIs and SMI, revealing a moderate-to-strong positive correlation between SMVIs and SMI. The ROC curve analysis demonstrated that SMVIs exhibit good diagnostic performance for identifying low muscle mass, with all 4 parameters having an AUC greater than 0.75. In the assessment of survival risk, our findings indicated that NSCLC patients with low SMI have a shorter OS, which aligns with previous research outcomes (25). Furthermore, by employing diagnostic thresholds for SMVR, SMTVDI, SMLVDI, and SMVHI to categorize patients into high- and low-risk groups for diagnosing low muscle mass, Kaplan-Meier curves demonstrated a noticeable disparity in OS among NSCLC patients based on differing SMVR, SMTVDI, and SMLVDI levels. Previous studies have suggested that sarcopenia can significantly impact survivability in NSCLC patients through multiple interconnected mechanisms, including reduced treatment tolerance, impaired immune function, metabolic dysregulation, functional decline, and increased risk of complications (26-28). Therefore, identifying and addressing sarcopenia early in the course of NSCLC management may improve treatment outcomes and OS.
It is worth noting that not all SMVIs exhibit consistent performance. Considering results across both male and female populations for identifying low muscle mass, SMVR demonstrated the optimal diagnostic efficacy, achieving the highest combined values for AUC, sensitivity, and specificity. Conversely, SMVHI exhibited the lowest correlation with SMI (r=0.67) and demonstrated the poorest performance in diagnosing low muscle mass (AUC in males =0.763, AUC in females =0.742). It provided insignificant assistance in predicting the OS for NSCLC patients with low muscle mass. This is attributed to the significant role of age in relation to height (29). Studies indicate that VH decreases with age, particularly in later stages of life, which is associated with age-related bone loss (30,31). Additionally, VH ratios are subject to age-related changes, often decreasing with advancing age, especially among postmenopausal women (32). Therefore, when not adjusted for age, SMVHI’s ability to identify low muscle mass is less effective compared to other SMVIs.
In this study, we recommend using SMVIs as an alternative for assessing muscle mass when SMI data is unavailable. Cutoff values are provided for diagnosing low muscle mass using SMVIs. Recent research involving deep learning methods for fully automated muscle segmentation and the associated 2D vertebral parameters utilized may provide a relatively straightforward approach for quickly obtaining muscle mass assessments in clinical settings (33). However, if SMI data is available, we advocate for the use of this more widely accepted and recognized metric.
There are several limitations to this current study. Firstly, routinely obtaining CT images at the L3 level is not standard practice in thoracic examinations for lung cancer. In this study, in order to effectively evaluate the feasibility of SMVI, we chose the SMI measured at the L3 level recommended in the guideline as a reference. Whether the L3 level is the optimal choice remains unclear and requires further investigation in future studies. Secondly, in this study, we did not focus on the potential impact of SMVR on treatment. Thirdly, in this single-center retrospective study, the participants were limited to NSCLC patients. The generalization of the study findings to other populations remains to be investigated but can serve as a reference.
Conclusions
SMVI is derived from the standardization of SMA using 2D vertebral parameters, namely SMVR, SMTVDI, SMLDVI, and SMVHI. It exhibits a significant positive correlation with SMI at the L3 level. SMVI effectively identifies low muscle mass as defined by SMI. NSCLC patients with low SMVR, SMTVDI, or SMLDVI exhibit a notably reduced OS. For patients with missing SMI data, SMVI serves as a feasible alternative method for assessing skeletal muscle mass.
Acknowledgments
Funding: This work was supported by
Footnote
Reporting Checklist: The authors have completed the STARD reporting checklist. Available at https://qims.amegroups.com/article/view/10.21037/qims-24-120/rc
Conflicts of Interest: All authors have completed the ICMJE uniform disclosure form (available at https://qims.amegroups.com/article/view/10.21037/qims-24-120/coif). The authors have no conflicts of interest to declare.
Ethical Statement: The authors are accountable for all aspects of the work in ensuring that questions related to the accuracy or integrity of any part of the work are appropriately investigated and resolved. The study was conducted following the Declaration of Helsinki (as revised in 2013). Approval for this study was obtained from the Ethics Committee of the First Affiliated Hospital of Kunming Medical University (No. 2022L305) and the requirement for individual consent for this retrospective analysis was waived.
Open Access Statement: This is an Open Access article distributed in accordance with the Creative Commons Attribution-NonCommercial-NoDerivs 4.0 International License (CC BY-NC-ND 4.0), which permits the non-commercial replication and distribution of the article with the strict proviso that no changes or edits are made and the original work is properly cited (including links to both the formal publication through the relevant DOI and the license). See: https://creativecommons.org/licenses/by-nc-nd/4.0/.
References
- Cartee GD, Hepple RT, Bamman MM, Zierath JR. Exercise Promotes Healthy Aging of Skeletal Muscle. Cell Metab 2016;23:1034-47. [Crossref] [PubMed]
- Wilkinson DJ, Piasecki M, Atherton PJ. The age-related loss of skeletal muscle mass and function: Measurement and physiology of muscle fibre atrophy and muscle fibre loss in humans. Ageing Res Rev 2018;47:123-32. [Crossref] [PubMed]
- Cruz-Jentoft AJ, Bahat G, Bauer J, Boirie Y, Bruyère O, Cederholm T, Cooper C, Landi F, Rolland Y, Sayer AA, Schneider SM, Sieber CC, Topinkova E, Vandewoude M, Visser M, Zamboni M. Writing Group for the European Working Group on Sarcopenia in Older People 2 (EWGSOP2), and the Extended Group for EWGSOP2. Sarcopenia: revised European consensus on definition and diagnosis. Age Ageing 2019;48:16-31. [Crossref] [PubMed]
- Yang M, Shen Y, Tan L, Li W. Prognostic Value of Sarcopenia in Lung Cancer: A Systematic Review and Meta-analysis. Chest 2019;156:101-11. [Crossref] [PubMed]
- Kim EY, Kim YS, Park I, Ahn HK, Cho EK, Jeong YM. Prognostic Significance of CT-Determined Sarcopenia in Patients with Small-Cell Lung Cancer. J Thorac Oncol 2015;10:1795-9. [Crossref] [PubMed]
- Buentzel J, Heinz J, Bleckmann A, Bauer C, Röver C, Bohnenberger H, Saha S, Hinterthaner M, Baraki H, Kutschka I, Emmert A. Sarcopenia as Prognostic Factor in Lung Cancer Patients: A Systematic Review and Meta-analysis. Anticancer Res 2019;39:4603-12. [Crossref] [PubMed]
- Wang J, Cao L, Xu S. Sarcopenia affects clinical efficacy of immune checkpoint inhibitors in non-small cell lung cancer patients: A systematic review and meta-analysis. Int Immunopharmacol 2020;88:106907. [Crossref] [PubMed]
- Bye A, Sjøblom B, Wentzel-Larsen T, Grønberg BH, Baracos VE, Hjermstad MJ, Aass N, Bremnes RM, Fløtten Ø, Jordhøy M. Muscle mass and association to quality of life in non-small cell lung cancer patients. J Cachexia Sarcopenia Muscle 2017;8:759-67. [Crossref] [PubMed]
- Chargi N, Bril SI, Smid EJ, de Jong PA, de Bree R. Cut-off values for low skeletal muscle mass at the level of the third cervical vertebra (C3) in patients with head and neck cancer. Quant Imaging Med Surg 2022;12:3024-33. [Crossref] [PubMed]
- Zaffina C, Wyttenbach R, Pagnamenta A, Grasso RF, Biroli M, Del Grande F, Rizzo S. Body composition assessment: comparison of quantitative values between magnetic resonance imaging and computed tomography. Quant Imaging Med Surg 2022;12:1450-66. [Crossref] [PubMed]
- Tagliafico AS, Bignotti B, Torri L, Rossi F. Sarcopenia: how to measure, when and why. Radiol Med 2022;127:228-37. [Crossref] [PubMed]
- Janssen I, Baumgartner RN, Ross R, Rosenberg IH, Roubenoff R. Skeletal muscle cutpoints associated with elevated physical disability risk in older men and women. Am J Epidemiol 2004;159:413-21. [Crossref] [PubMed]
- Bahat G, Turkmen BO, Aliyev S, Catikkas NM, Bakir B, Karan MA. Cut-off values of skeletal muscle index and psoas muscle index at L3 vertebra level by computerized tomography to assess low muscle mass. Clin Nutr 2021;40:4360-5. [Crossref] [PubMed]
- Schiaffino S, Albano D, Cozzi A, Messina C, Arioli R, Bnà C, et al. CT-derived Chest Muscle Metrics for Outcome Prediction in Patients with COVID-19. Radiology 2021;300:E328-36. [Crossref] [PubMed]
- TROTTER M. GLESER GC. Estimation of stature from long bones of American Whites and Negroes. Am J Phys Anthropol 1952;10:463-514. [Crossref] [PubMed]
- Simon S, Fischer B, Rinner A, Hummer A, Frank BJH, Mitterer JA, Huber S, Aichmair A, Schwarz GM, Hofstaetter JG. Body height estimation from automated length measurements on standing long leg radiographs using artificial intelligence. Sci Rep 2023;13:8504. [Crossref] [PubMed]
- Torimitsu S, Makino Y, Saitoh H, Ishii N, Hayakawa M, Yajima D, Inokuchi G, Motomura A, Chiba F, Iwase H. Stature estimation in Japanese cadavers using the sacral and coccygeal length measured with multidetector computed tomography. Leg Med (Tokyo) 2014;16:14-9. [Crossref] [PubMed]
- Waduud MA, Sucharitkul PPJ, Drozd M, Gupta A, Hammond C, Ashbridge Scott DJ. Validation of two-dimensional vertebral body parameters in estimating patient height in elderly patients. Br J Radiol 2019;92:20190342. [Crossref] [PubMed]
- Zhang K, Chang YF, Fan F, Deng ZH. Estimation of stature from radiologic anthropometry of the lumbar vertebral dimensions in Chinese. Leg Med (Tokyo) 2015;17:483-8. [Crossref] [PubMed]
- Keyl J, Hosch R, Berger A, Ester O, Greiner T, Bogner S, Treckmann J, Ting S, Schumacher B, Albers D, Markus P, Wiesweg M, Forsting M, Nensa F, Schuler M, Kasper S, Kleesiek J. Deep learning-based assessment of body composition and liver tumour burden for survival modelling in advanced colorectal cancer. J Cachexia Sarcopenia Muscle 2023;14:545-52. [Crossref] [PubMed]
- Choi H, Park YS, Na KJ, Park S, Park IK, Kang CH, Kim YT, Goo JM, Yoon SH. Association of Adipopenia at Preoperative PET/CT with Mortality in Stage I Non-Small Cell Lung Cancer. Radiology 2021;301:645-53. [Crossref] [PubMed]
- Prado CM, Lieffers JR, McCargar LJ, Reiman T, Sawyer MB, Martin L, Baracos VE. Prevalence and clinical implications of sarcopenic obesity in patients with solid tumours of the respiratory and gastrointestinal tracts: a population-based study. Lancet Oncol 2008;9:629-35. [Crossref] [PubMed]
- Hamaguchi Y, Kaido T, Okumura S, Kobayashi A, Shirai H, Yao S, Yagi S, Kamo N, Okajima H, Uemoto S. Proposal for new selection criteria considering pre-transplant muscularity and visceral adiposity in living donor liver transplantation. J Cachexia Sarcopenia Muscle 2018;9:246-54. [Crossref] [PubMed]
- Muñoz JI, Liñares-Iglesias M, Suárez-Peñaranda JM, Mayo M, Miguéns X, Rodríguez-Calvo MS, Concheiro L. Stature estimation from radiographically determined long bone length in a Spanish population sample. J Forensic Sci 2001;46:363-6. [Crossref] [PubMed]
- Xiao J, Caan BJ, Cespedes Feliciano EM, Meyerhardt JA, Peng PD, Baracos VE, Lee VS, Ely S, Gologorsky RC, Weltzien E, Kroenke CH, Kwan ML, Alexeeff SE, Castillo AL, Prado CM. Association of Low Muscle Mass and Low Muscle Radiodensity With Morbidity and Mortality for Colon Cancer Surgery. JAMA Surg 2020;155:942-9. [Crossref] [PubMed]
- Best TD, Mercaldo SF, Bryan DS, Marquardt JP, Wrobel MM, Bridge CP, Troschel FM, Javidan C, Chung JH, Muniappan A, Bhalla S, Meyers BF, Ferguson MK, Gaissert HA, Fintelmann FJ. Multilevel Body Composition Analysis on Chest Computed Tomography Predicts Hospital Length of Stay and Complications After Lobectomy for Lung Cancer: A Multicenter Study. Ann Surg 2022;275:e708-15. [Crossref] [PubMed]
- de Jong C, Chargi N, Herder GJM, van Haarlem SWA, van der Meer F, van Lindert ASR, Ten Heuvel A, Brouwer J, de Jong PA, Devriese LA, Huitema ADR, Egberts TCG, de Bree R, Deneer VHM. The association between skeletal muscle measures and chemotherapy-induced toxicity in non-small cell lung cancer patients. J Cachexia Sarcopenia Muscle 2022;13:1554-64. [Crossref] [PubMed]
- de Leeuw SP, Pruis MA, Sikkema BJ, Mohseni M, Veerman GDM, Paats MS, Dumoulin DW, Smit EF, Schols AMWJ, Mathijssen RHJ, van Rossum EFC, Dingemans AC. Analysis of Serious Weight Gain in Patients Using Alectinib for ALK-Positive Lung Cancer. J Thorac Oncol 2023;18:1017-30. [Crossref] [PubMed]
- Krishan K, Kanchan T, Menezes RG, Ghosh A. Forensic anthropology casework-essential methodological considerations in stature estimation. J Forensic Nurs 2012;8:45-50. [Crossref] [PubMed]
- Shao Z, Rompe G, Schiltenwolf M. Radiographic changes in the lumbar intervertebral discs and lumbar vertebrae with age. Spine (Phila Pa 1976) 2002;27:263-8. [Crossref] [PubMed]
- O'Neill TW, Varlow J, Felsenberg D, Johnell O, Weber K, Marchant F, Delmas PD, Cooper C, Kanis J, Silman AJ. Variation in vertebral height ratios in population studies. European Vertebral Osteoporosis Study Group. J Bone Miner Res 1994;9:1895-907. [Crossref] [PubMed]
- Sone T, Tomomitsu T, Miyake M, Takeda N, Fukunaga M. Age-related changes in vertebral height ratios and vertebral fracture. Osteoporos Int 1997;7:113-8. [Crossref] [PubMed]
- Engelke K, Chaudry O, Gast L, Eldib MA, Wang L, Laredo JD, Schett G, Nagel AM. Magnetic resonance imaging techniques for the quantitative analysis of skeletal muscle: State of the art. J Orthop Translat 2023;42:57-72. [Crossref] [PubMed]