The value of synthetic magnetic resonance imaging in the diagnosis and assessment of prostate cancer aggressiveness
Introduction
Prostate cancer (PCA) is currently the second most common malignant tumor in males worldwide (1), and the diagnosis and treatment of PCA has advanced over time. The Prostate Imaging Reporting and Data System (PI-RADS) is the core of multiparametric magnetic resonance imaging (mpMRI) interpretation for PCA. However, without objective quantitative parameters, there is inconsistency in lesion interpretation among radiologists, leading to potential overdiagnosis or misdiagnosis (2). The Gleason Score (GS) is the most widely used system for assessing PCA aggressiveness, and biopsy remains a routine method for obtaining GS before surgery and treatment. The 2014 International Society of Urological Pathology (ISUP) Consensus conference on Gleason Grading of PCA recommended a more meticulous system of classification. In this system, GS =7 PCAs are more specifically reported as being either GS =3+4 (with GS3 being more predominant than the GS4 pattern) or GS =4+3 (with GS4 being more predominant than GS3) (3). GS ≤3+4 PCA is thought to indicate a low-risk cancer as compared to GS ≥4+3 disease, which indicates intermediate-to-high risk PCA. Low-risk PCA grows slowly, involves a lower risk of metastasis, and can be treated with less aggressive methods (4), while intermediate-to-high risk PCA is associated with a higher likelihood of postoperative positive margins and biochemical recurrence; moreover, it has a 23% lower overall survival rate compared to its counterpart (5-7) and thus requires radical prostatectomy (RP) or radiotherapy (8). However, due to limitations in biopsy samples and the histological heterogeneity of PCA, there are discrepancies between biopsy and RP specimens, leading to the potential underestimation of patients’ conditions (9). Therefore, there is a need to develop a reliable preoperative examination method to better differentiate low-risk and intermediate-to-high risk PCA and help surgeons determine the appropriate procedures while minimizing surgical trauma and the occurrence of postoperative urinary incontinence or erectile dysfunction.
Due to the limitations of biopsy and the radiologists’ subjective interpretation of mpMRI, various quantitative MRI techniques have been used in PCA in recent years. Previous studies have suggested that apparent diffusion coefficient (ADC) value derived from diffusion-weighted imaging (DWI) may overlap across various grades of PCA (10-12). Some studies compared the value of DWI with derived diffusion imaging techniques such as diffusion kurtosis imaging, intravoxel incoherent motion data, and diffusion tensor imaging in assessing PCA aggressiveness (13-15); however, these studies included small sample sizes and reported inconsistent findings, with some suggesting that these techniques perform less ably than does ADC in diagnosing PCA and assessing aggressiveness. Furthermore, these techniques are time-consuming and have complex postprocessing and analysis methods, limiting their clinical applicability. In addition, some investigators have proposed a learning-based method, which is based on a convolutional neural network trained with synthetic data, to compute T1, T2, and proton density (PD) parametric maps from only a pair of T1WI and T2WI acquired in the clinical routine. However, this technique is currently limited to the brain, and the application of this method in prostate remains challenging due to the interference of motion artifacts (16,17).
Synthetic magnetic resonance imaging (SyMRI) was introduced in 1985. More recently, Warntjes et al. proposed a novel sequence involving the quantification of T1, T2, and PD with quantification of relaxation times and PD by multiecho acquisition of a saturation-recovery using turbo spin-echo readout (QRAPMASTER), which is now referred to as the multidynamic multiecho (MDME) sequence (18). Compared to traditional MRI, SyMRI is a relatively new technique for prostate disease. It is objective, standardized, and highly reproducible and can provide absolute quantitative values of T1, T2, and PD based on tissue characteristics with a single scan, indirectly reflecting tissue composition and pathophysiology (19).
Our study thus aimed to evaluate the feasibility of using SyMRI in the diagnosis of PCA and its value as a noninvasive prognostic biomarker for assessing PCA aggressiveness. We present this article in accordance with the STROBE reporting checklist (available at https://qims.amegroups.com/article/view/10.21037/qims-24-291/rc).
Methods
Ethical approval
This study was conducted in accordance with the Declaration of Helsinki (as revised in 2013). This study was approved by the institutional review board of The First Affiliated Hospital of Nanjing Medical University (No. 2023-SR-892). Given the nature and design of the study, the requirement for individual consent for this retrospective analysis was waived.
General information
A total of 1,040 patients suspected of PCA who underwent prostate mpMRI and SyMRI examinations between June 2022 and August 2023 were consecutively recruited in this study, among whom 395 had histopathological results obtained through transperineal prostate biopsy (TTPB) or RP. For patients with PCA who underwent RP after biopsy, RP results were considered as the final pathology (Figure 1). The inclusion criteria were as follows: (I) a prostate-specific antigen (PSA) level >4 ng/mL or positive findings on digital rectal examination; (II) performance of standardized mpMRI and SyMRI examinations with satisfactory image quality; and (III) pathological results obtained within 1 month after MRI examination. The exclusion criteria were as follows: (I) pathological results of abscess, granuloma, or neuroendocrine PCA; (II) TTPB performed within 6 weeks before MRI; (III) previous surgical resection for benign prostatic hyperplasia (BPH); (IV) maximum lesion diameter ≤5 mm; and (V) diffuse PCA lesions in which a region of interest (ROI) was difficult to delineate. Finally, a total of 309 patients with suspected PCA were included in this study (Table 1, Figure 1).
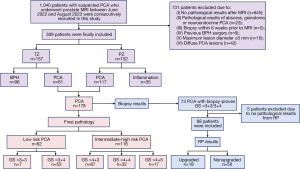
Table 1
Characteristic | Result |
---|---|
Age (years) | P=0.001 |
Non-PCA | 69.0±7.2 |
PCA | 66.2±7.2 |
PSA (ng/mL) | P<0.001 |
Non-PCA | 12.21 (7.40, 23.35) |
PCA | 9.52 (5.91, 14.36) |
Pathology | |
Non-PCA | 131 (42.4) |
TZ-BPH | 96 |
PZ-inflammation | 35 |
PCA | 178 (57.6) |
PCA-TZ | 61 |
PCA-PZ | 117 |
Final GS | |
Low-risk PCA | 62 (34.8) |
3+3 | 7 |
3+4 | 55 |
Intermediate-high PCA | 116 (65.2) |
4+3 | 67 |
4+4 | 32 |
4+5 | 17 |
GS ≤3+4 by TTPB | |
Upgraded | 18 (26.5) |
3+3 → 4+3 | 1 |
3+4 → 4+3 | 16 |
3+4 → 4+5 | 1 |
Non-upgraded | 50 (73.5) |
3+3 → 3+3 | 6 |
3+3 → 3+4 | 15 |
3+4 → 3+4 | 29 |
Data following a normal distribution are expressed as the mean ± standard deviation. Otherwise, data are expressed as the median (first quartile, third quartile) or n or n (%). PCA, prostate cancer; PSA, prostate-specific antigen; TZ, transformation zone; BPH, benign prostatic hyperplasia; PZ, peripheral zone; GS, Gleason Score; TTPB, transperineal prostate biopsy.
Based on the final pathological results, the 309 patients were divided into a PCA group (178 cases) and non-PCA group (131 cases). According to the location of the lesion, PCA lesions were classified as peripheral zone (PZ) PCA (PCA-PZ) and transitional zone (TZ) PCA (PCA-TZ). Among the 152 cases of PZ lesions, 117 were PCA-PZ, and 35 were PZ inflammation. Among the 157 cases of TZ lesions, 61 were PCA-TZ, and 96 were BPH. According to the 2014 ISUP grade system for PCA aggressiveness (3), the 178 patients with PCA were further divided into low-risk group (GS ≤3+4; 62 cases) and intermediate-to-high risk group (GS ≥4+3; 116 cases).
To further determine whether the SyMRI parameters could predict the upgrade of biopsy-confirmed PCA in the low-risk group, among the 178 patients with PCA with TTPB as the initial pathology, we retrospectively collected 73 patients with PCA whose initial pathology was GS =3+3/3+4. The exclusion criteria for this group were as follows: (I) no RP pathological results or an interval of more than 1 month between biopsy and RP and (II) prior endocrine and/or radiotherapy before RP. Among the eligible patients, 5 were ultimately excluded based on these criteria while 68 patients were included. These patients were further divided into a GS-upgraded group (18 cases) and nonupgraded group (50 cases) based on whether the RP results were upgraded to GS ≥4+3. The above process is specified in Figure 1 and Table 1.
MRI parameters and image processing
All MRI examinations were performed using a 3.0-T MRI scanner (SIGNA PET/MR; GE HealthCare, Chicago, IL, USA) with a pelvic phased-array surface coil without an endorectal coil. The following sequences were acquired for all patients: axial, coronal, and sagittal T2-weighted imaging (T2WI), axial diffusion-weighted imaging (DWI), T1WI and SyMRI. The DWI sequence was acquired with b-values of 50, 800, 1,400, and 2,000 s/mm2, and ADC maps were generated using postprocessing software (READYView). The SyMRI technique involved an MDME sequence with two echo times (22/95 msec) and four saturation delay times (170, 670, 1,840, and 3,840 msec). The detailed imaging sequence parameters are provided in Table S1. Quantitative parameter maps (T1, T2, and PD maps) were generated within 10 seconds using an offline postprocessing software (SyMRI 8.0; SyntheticMR, Linköping, Sweden) called “Magic”, based on the MDME raw data.
ROI delineation
Two experienced radiologists first selected the maximum lesion diameter image on the ADC map and manually delineated the ROI, covering the lesion contour as much as possible. Subsequently, according to the ADC map, the corresponding ROI was manually delineated on the SyMRI T2 parameter map to simultaneously obtain the T1, T2, and PD values of the lesion. Because SyMRI T2 demonstrated the best lesion contrast compared to SyMRI T1 and SyMRI PD, which was consistent with the ADC map. Each radiologist performed two consecutive measurements, with the average value being recorded. The specific examples are shown in Figure 2.
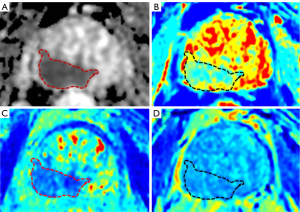
Statistical analysis
Statistical analysis was performed using SPSS software version 27.0 (IBM Corp., Armonk, NY, USA) and MedCalc software version 15 (MedCalc Software, Ostend, Belgium). Measurement data are presented as mean ± standard deviation () or as the median with quartiles (P25, P75), and categorical variables are expressed as counts with percentages. The sensitivity, specificity, positive likelihood ratio, negative likelihood ratio, positive predictive value (PPV), and negative predictive value (NPV) were calculated using Youden index as the cutoff point. Independent samples t-tests or Mann-Whitney tests were used to assess the differences in parameters between the PCA and non-PCA groups. The diagnostic performance of each parameter was evaluated using receiver operative characteristic (ROC) curve analysis, and the area under the curve (AUC) was calculated for quantification (20). The differences in ADC, T1, T2, and PD values among the different GS grades of PCA were assessed using the Kruskal-Wallis test. Spearman rank correlation coefficient was used to evaluate the correlation between each parameter and GS grades. Mann-Whitney tests were employed to compare the differences of various parameter between the intermediate-to-high risk and low-risk PCA groups and those between the upgraded and nonupgraded PCA groups. The risk factors associated with GS grades were identified via univariate analysis, and then the effects of T1 values were excluded as confounding factors via multivariate logistic regression analysis, and the independent predictive factors, including ADC, T2 and PD values, were then calculated to establish the combined model—ADC+Sy(T2+PD) model, for predicting PCA risk grades or whether PCA was upgraded. The diagnostic performance of each parameter and the ADC+Sy(T2+PD) combined model was analyzed, the AUC was used for discrimination, the calibration curve was calculated with internal validation via the bootstrapping method (200 bootstrap resamples), and calibration was evaluated with the Hosmer-Lemeshow goodness-of-fit test. Calibration was performed using R language version 4.3.1 (The Foundation of Statistical Computing), specifically with the “pROC” and “rms” packages. The interreader reproducibility of the measured parameters was evaluated via intraclass correlation coefficients (ICCs) and 95% confidence intervals (CIs). The ICC values ranged from 0 to 1.00, with values closer to 1.00 representing better reproducibility. Differences with a two-tailed P value <0.05 were considered statistically significant.
Results
Comparison of MRI quantitative parameters between PCA and non-PCA
The clinical characteristics of the participants are shown in Table 1. There were significant differences in age and PSA levels between those with PCA and those without PCA (P≤0.001).
The T1, T2, PD, and ADC values of prostate lesions with different pathological types are provided in Table 2 and Figure 3, and the results showed that the PCA groups had significantly lower T1, T2, PD, and ADC values compared with the TZ BPH (Figure 3A-3D) and PZ inflammation groups (Figure 3E-3H) (P≤0.001).
Table 2
Pathology | TZ | PZ | PCA risk grade | TTPB GS ≤3+4 | |||||||||||
---|---|---|---|---|---|---|---|---|---|---|---|---|---|---|---|
PCA (n=61) | BPH (n=96) | P | PCA (n=117) | Inflammation (n=117) | P | GS ≥4+3 (n=116) | GS ≤3+4 (n=62) | P | Upgraded (n=18) |
Nonupgraded (n=50) |
P | ||||
T1 (msec) | 1,203.83±121.03 | 1,339.37±169.41 | <0.001 | 1,270.27±126.12 | 1,402.80±210.84 | <0.001 | 1,261.52±130.66 | 1,207.00 (1,137.75, 1,275.25) |
0.03 | 1,192.00 (1,112.00, 1,263.75) |
1,287.50 (1,217.25, 1,454.25) |
0.007 | |||
T2 (msec) | 74.15±8.10 | 80.00 (76.00, 85.00) |
<0.001 | 78.00 (73.00, 82.00) |
88.57±13.60 | <0.001 | 77.00 (73.00, 82.00) |
73.31±7.49 | 0.001 | 73.00 (67.00, 79.00) |
78.00 (75.75, 81.25) |
0.003 | |||
PD (pu) | 79.22±3.86 | 81.00 (80.00, 84.00) |
<0.001 | 81.00 (78.00, 83.00) |
83.00 (81.00, 85.00) |
<0.001 | 81.00 (78.50, 84.00) |
79.00 (77.00, 81.00) |
0.003 | 79.50 (77.00, 82.00) |
83.50 (78.75, 84.25) |
0.009 | |||
ADC (×10−6 mm2/s) |
599.22±87.23 | 837.00 (785.50, 907.50) |
<0.001 | 605.74±100.80 | 888.97±160.24 | <0.001 | 580.94±90.10 | 634.50 (604.00, 695.75) |
<0.001 | 636.50 (602.75, 695.00) |
574.00 (528.75, 629.25) |
0.002 |
Data following a normal distribution are expressed as the mean ± standard deviation. Otherwise, data are expressed as the median (first quartile, third quartile). PD, proton density; ADC, apparent diffusion coefficient; PCA, prostate cancer; GS, Gleason Score; TZ, transformation zone; PZ, peripheral zone; TTPB, transperineal prostate biopsy; BPH, benign prostatic hyperplasia.
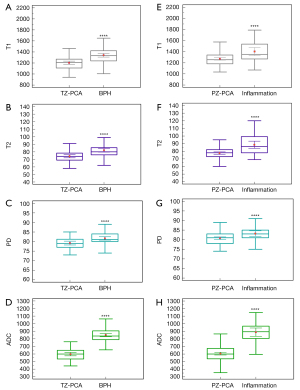
Table 3 and Figure 4 are a summary of each quantitative parameter (T1, T2, PD, and ADC) in regard to its ability to identify the benign and malignant nature of prostate lesions and the aggressiveness of PCA. The second and third columns of Table 3 present the AUC value, cutoff value, sensitivity, and specificity of each parameter for distinguishing PCA from non-PCA. The AUC of ADC was significantly higher than that of T1, T2, and PD regardless of whether the lesions were in the PZ or TZ (P<0.001), and the ROC is shown in Figure 4A,4B. The results of the univariate analysis showed that the T1, T2, PD, and ADC values were associated with the benign or malignant nature of the lesions, whether in the TZ or PZ (Table 4). All of these variables were selected to establish a multivariate logistic regression model. The enter method was used in the multivariate logistic regression analysis. After the effects of confounding variables were removed, only ADC value remained as an independent risk factor for predicting benign or malignant prostate lesions (Table 4). Moreover, the interobserver agreement was excellent in our study (Table S2).
Table 3
Parameter | TZ PCA vs. BPH | PZ PCA vs. inflammation | Intermediate-to-high risk vs. low-risk PCA | GS upgraded vs. nonupgraded PCA |
---|---|---|---|---|
T1 (msec) | ||||
AUC | 0.760 (0.685–0.824) | 0.698 (0.618–0.769) | 0.601 (0.525–0.674) | 0.717 (0.595–0.820) |
Sen (%) | 77.1 (64.5–86.8) | 55.6 (46. –64.7) | 53.5 (44.0–62.8) | 83.3 (58.6–96.4) |
Spe (%) | 64.6 (54.2–74.1) | 77.1 (59.9–89.6) | 54.8 (41.7–67.5) | 56.0 (41.3–70.0) |
Cutoff value | ≤1,269 | ≤1,267 | ≥1,227 | >1,203 |
T2 (msec) | ||||
AUC | 0.742 (0.666–0.808) | 0.744 (0.667–0.812) | 0.646 (0.571–0.717) | 0.735 (0.614–0.835) |
Sen (%) | 62.3 (49.0–74.4) | 89.0 (81.7–93.9) | 84.5 (76.6–90.5) | 94.4 (72.7–99.9) |
Spe (%) | 79.2 (69.7–86.8) | 54.3 (36.6–71.2) | 43.6 (31.0–56.7) | 48.0 (33.7–62.6) |
Cutoff value | ≤75 | ≤85 | ≥71 | >71 |
PD (pu) | ||||
AUC | 0.699 (0.620–0.769) | 0.682 (0.601–0.755) | 0.636 (0.561–0.707) | 0.707 (0.584–0.811) |
Sen (%) | 50.8 (37.7–63.9) | 67.5 (58.2–75.9) | 27.6 (19.7–36.7) | 50.0 (26.0–74.0) |
Spe (%) | 80.2 (70.8–87.6) | 68.6 (50.7–83.1) | 95.2 (86.5–99.0) | 78.0 (64.0–88.5) |
Cutoff value | ≤79 | ≤82 | ≥83 | >83 |
ADC (×10−6 mm2/s) | ||||
AUC | 0.974 (0.935–0.993) | 0.935 (0.884–0.969) | 0.708 (0.635–0.774) | 0.743 (0.622–0.841) |
Sen (%) | 91.8 (81.9–97.3) | 89.7 (82.8–94.6) | 66.4 (57.0–74.9) | 66.7 (41.0–86.7) |
Spe (%) | 94.8 (88.3–98.3) | 91.4 (76.9–98.2) | 77.4 (65.0–87.1) | 78.0 (64.0–88.5) |
Cutoff value | ≤700 | ≤711 | ≤600 | ≤597 |
PLR | 17.63 (7.48–41.52) | 10.47 (3.54–30.95) | 2.94 (1.82–447) | 3.03 (1.64–5.61) |
NLR | 0.086 (0.037–0.20) | 0.11 (0.065–0.19) | 0.43 (0.33–0.58) | 0.43 (0.22–0.83) |
PPV (%) | 91.8 (82.6–96.3) | 97.2 (92.2–99.0) | 84.6 (77.3–89.9) | 52.2 (37.1–66.9) |
NPV (%) | 94.8 (88.7–97.7) | 72.7 (60.7–82.1) | 55.2 (48.0–62.2) | 86.7 (76.9–92.7) |
ADC+Sy(T2+PD) | ||||
AUC | – | – | 0.818 (0.754–0.872) | 0.947 (0.864–0.987) |
Sen (%) | – | – | 66.38 (57.0–74.9) | 94.44 (72.7–99.9) |
Spe (%) | – | – | 90.32 (80.1–96.4) | 92.00 (80.8–97.8) |
Cutoff value | – | – | ≥0.72349 | ≥0.26734 |
PLR | – | – | 6.86 (3.17–14.84) | 11.81 (4.58–30.42) |
NLR | – | – | 0.37 (0.28–0.49) | 0.06 (0.009–0.41) |
PPV (%) | – | – | 92.8 (85.6–96.5) | 81.0 (62.3–91.6) |
NPV (%) | – | – | 58.9 (52.3–65.3) | 97.9 (87.2–99.7) |
Values in parentheses are the 95% CI. ROC, receiver operating characteristic; PD, proton density; ADC, apparent diffusion coefficient; PCA, prostate cancer; BPH, benign prostatic hyperplasia; GS, Gleason Score; TZ, transformation zone; PZ, peripheral zone; AUC, area under the curve; Sen, sensitivity; Spe, specificity; PLR, positive likelihood ratio; NLR, negative likelihood ratio; PPV, positive predictive value; NPV, negative predictive value; CI, confidence interval.
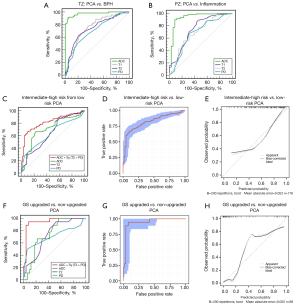
Table 4
Parameters | Univariate analysis | Multivariate logistic regression analysis | |||
---|---|---|---|---|---|
OR (95% CI) | P | OR (95% CI) | P | ||
TZ | |||||
T1 (msec) | 1.007 (1.004–1.010) | <0.001 | 1.003 (0.997–1.010) | 0.303 | |
T2 (msec) | 1.101 (1.052–1.153) | <0.001 | 1.035 (0.953–1.124) | 0.414 | |
PD (pu) | 1.160 (1.061–1.270) | <0.001 | 1.061 (0.852–1.323) | 0.595 | |
ADC (×10−6 mm2/s) | 1.032 (1.021–1.044) | <0.001 | 1.031 (1.019–1.043) | <0.001* | |
PZ | |||||
T1 (msec) | 1.005 (1.003–1.008) | <0.001 | 0.999 (0.994–1.004) | 0.615 | |
T2 (msec) | 1.097 (1.053–1.143) | <0.001 | 1.046 (0.975–1.123) | 0.213 | |
PD (pu) | 1.180 (1.059–1.314) | 0.003 | 1.170 (0.978–1.398) | 0.086 | |
ADC (×10−6 mm2/s) | 1.017 (1.011–1.023) | <0.001 | 1.016 (1.010–1.022) | <0.001* |
*, a statistically significant value. PCA, prostate cancer; OR, odds ratio; CI, confidence interval; TZ, transformation zone; PD, proton density; ADC, apparent diffusion coefficient; PZ, peripheral zone.
Assessment of PCA aggressiveness with T1, T2, PD, and ADC values
Kruskal-Wallis tests revealed significant differences in the T1, T2, PD, and ADC values among the different GS grades of PCA (Table 5). T2 (r=0.226; P=0.002) and PD values (r=0.215; P=0.004) showed a positive correlation with GS grades, while ADC value showed a negative correlation (r=−0.372; P<0.001), and T1 value (r=0.133, P=0.08) increased with the increase in GS grade but showed no significant correlation with GS grade (Table 6). The T1 values of seven patients with GS =3+3 was higher (1,354±101.66) than those with other PCA grades. Furthermore, the T1, T2, and PD values were significantly higher in the intermediate-to-high risk group compared with the low-risk group (P<0.05), while the ADC value was significantly lower in the intermediate-to-high risk group (P<0.001) (Table 2, Figure 5A-5D).
Table 5
Parameter | GS =3+3 | GS =3+4 | GS =4+3 | GS =4+4 | GS =4+5 | P |
---|---|---|---|---|---|---|
T1 (msec) | 1,354±101.66 | 1,193 (1,118, 1,255) | 1,259.88±146.19 | 1,261.91±105.00 | 1,267.24±114.61 | 0.005 |
T2 (msec) | 71.57±7.48 | 73.53±7.53 | 77.40±9.27 | 79.00±9.56 | 77.05±8.34 | 0.025 |
PD (pu) | 80.71±4.15 | 78.9 5±3.09 | 80.73±4.01 | 80.81±4.97 | 81.12±3.52 | 0.03 |
ADC (×10−6 mm2/s) | 671±101.80 | 633 (604, 693) | 594.07±94.60 | 581 (528, 652) | 567.94±76.20 | <0.001 |
Data following a normal distribution are expressed as the mean ± standard deviation. Otherwise, data are expressed as the median (first quartile, third quartile). PD, proton density; ADC, apparent diffusion coefficient; PCA, prostate cancer; GS, Gleason Score.
Table 6
Parameter | T1 (msec) | T2 (msec) | PD (pu) | ADC (×10−6 mm2/s) |
---|---|---|---|---|
P | 0.08 | 0.002 | 0.004 | <0.001 |
r | 0.133 | 0.226 | 0.215 | −0.372 |
GS, Gleason Score; MRI, magnetic resonance imaging; PD, proton density; ADC, apparent diffusion coefficient.
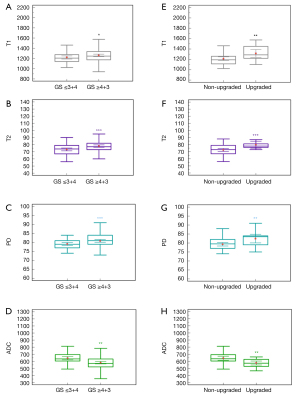
As shown in the data in the fourth column of Table 3 and the ROC in Figure 4C, the individual T1, T2, and PD quantitative parameters still had slightly lower AUC values compared with ADC for distinguishing low-risk and intermediate-to-high risk PCA, but their differences were not statistically significant (P=0.07, P=0.37, and P=0.25, respectively). The results of the univariate analysis showed that the higher T1, T2, PD, and the lower ADC values were associated with a more invasive risk grade of PCA (GS ≥4+3). All of these variables were used to establish a multivariate logistic regression model. The enter method was used in conducting multivariate logistic regression analysis. After effects of confounding variables were removed, three variables (T2, PD, and ADC) were retained in the final logistic regression model (Table 7). Finally, as shown in Table 3, the combined model ADC+(T2+PD) performed significantly better in differentiating low-risk PCA from intermediate-to-high risk PCA. In comparison with ADC alone, the combined model increased specificity by 12.9%, and provided significantly higher AUC improvement of 0.110 (P=0.003).
Table 7
Parameters | Univariate analysis | Multivariate logistic regression analysis | |||
---|---|---|---|---|---|
OR (95% CI) | P | OR (95% CI) | P | ||
T1 (msec) | 1.003 (1.000–1.005) | 0.044 | 0.999 (0.995–1.002) | 0.419 | |
T2 (msec) | 1.070 (1.026–1.116) | 0.002 | 1.131 (1.066–1.200) | <0.001* | |
PD (pu) | 1.118 (1.027–1.217) | 0.010 | 1.227 (1.095–1.375) | <0.001* | |
ADC (×10−6 mm2/s) | 1.139 (1.041–1.247) | 0.005 | 0.989 (0.984–0.993) | <0.001* |
*, a statistically significant value. GS, Gleason Score; PCA, prostate cancer; OR, odds ratio; CI, confidence interval; PD, proton density; ADC, apparent diffusion coefficient.
Moreover, the combined model performed well in terms of calibration (Hosmer-Lemeshow goodness-of-fit test P=0.203), and good calibration was observed in the internal validation cohort (Figure 4D,4E).
Assessment of the T1, T2, PD, and ADC values as predictors of GS upgrade in low-risk PCA
Among the 68 patients with low-risk PCA on the basis of TTPB, 18 (26.5%) patients had their GS upgraded to intermediate-to-high risk after RP (Table 1). Compared with the nonupgraded group, the upgraded group showed significantly higher T1, T2, and PD values (P<0.01), while their ADC values were significantly lower (P=0.002) (Table 2, Figure 5E-5H). Moreover, the individual quantitative parameters of T1, T2, and PD showed similar AUC values to that of ADC (P=0.074, P=0.37, P=0.25, respectively).
The results of the univariate analysis showed that T1, T2, PD, and ADC values were associated with the upgrade of the lesions. The effects of confounding factors were excluded by multivariate logistic regression analysis, and the new combined predictive factors, ADC+Sy(T2+PD), were calculated (Table 8). The combined model, ADC+Sy(T2+PD), exhibited improved sensitivity, specificity, PPV, and NPV (increases of 27.7%, 14.0%, 28.8.%, and 11.2%, respectively), with a 0.207 increase in AUC (P=0.001). The results are illustrated in the last column of Table 3 and Figure 4F.
Table 8
Parameters | Univariate analysis | Multivariate logistic regression analysis | |||
---|---|---|---|---|---|
OR (95% CI) | P | OR (95% CI) | P | ||
T1 (msec) | 1.005 (1.001–1.009) | 0.013 | 0.997 (0.989–1.005) | 0.426 | |
T2 (msec) | 1.151 (1.037–1.277) | 0.008 | 1.462 (1.160–1.842) | 0.001* | |
PD (pu) | 1.250 (1.058–1.478) | 0.01 | 1.535 (1.112–2.120) | 0.009* | |
ADC (×10−6 mm2/s) | 0.991 (0.983–0.998) | 0.013 | 0.978 (0.964–0.992) | 0.002* |
*, a statistically significant value. GS, Gleason Score; PCA, prostate cancer; OR, odds ratio; CI, confidence interval; PD, proton density; ADC, apparent diffusion coefficient.
Moreover, the combined model performed well in terms of calibration (Hosmer-Lemeshow goodness-of-fit test P=0.101), and good calibration was observed in the internal validation cohort (Figure 4G,4H). These findings suggested that the combined model provided better prediction of postoperative GS upgrade in patients with biopsy-confirmed low-risk PCA.
Discussion
The signal intensity of conventional T1WI, T2WI, and PDWI depends on different acquisition parameters and MR scanners, and these images mainly show the variable contrast of different tissues. Therefore, this signal intensity was all weighted, so it is not comparable. SyMRI is a quantitative sequence based on QRAPMASTER (18). This technique facilitates the absolute quantification of T1, T2, and PD values, which is objective and can reflect the characteristics of lesions. We hypothesized that objective quantitative parameter values of T1, T2, and PD obtained with SyMRI could help to distinguish PCA from non-PCA and that combining mpMRI and SyMRI may be a viable method for assessing PCA aggressiveness and predicting the postoperative upgrade of low-risk PCA. We used the MDME sequence to obtain SyMRI T1, T2, and PD parametric maps, which required only 5 minutes, could ensure the same in-plane resolution as that of T1WI and T2WI on mpMRI, and had a lower motion susceptibility compared with traditional quantitative techniques such as T2 mapping, whose scanning time is over 10 minutes (21,22). Furthermore, the MDME sequence calculates the radiofrequency field value for each voxel during the imaging process, enabling radiofrequency field correction and thus further enhancing the accuracy of quantitative parameters measurement (23). Additionally, by using uniform scanning parameters, the MDME sequence maintains high accuracy in the measurement of T1, T2 and PD values (coefficient of variation <5%) across various MRI scanner vendors (24) and thus should be generalizable to other centers. However, SyMRI is not a conventional weighted image that is widely used in the clinical routine, meaning that this method is not available for everyone and is not conducive to promotion for its proprietary.
T1, T2, PD, and ADC values in differentiating PCA from benign lesions
Our study demonstrated that ADC values provide the best diagnostic performance in distinguishing PCA from benign lesions, which may be attributed to the higher proportion of intermediate-to-high risk patients with PCA recruited in our study (65.2%, 116/178). Compared with that in previous studies, our DWI sequence used a higher b-value (2,000 s/mm2) for imaging (16,25), thereby reducing the influence of microvascular perfusion and enhancing the visibility of the cancerous lesions. This has been shown to improve the detection rate of PCA (26,27). The high b-value might therefore have contributed to the better performance of ADC. Furthermore, contrary to previous research findings (16), our study suggested that PD value, in addition to T1 and T2 values, is a useful parameter in differentiating PCA from benign lesions, which may be attributed to the larger sample size in our study. Additionally, the decrease in T1 and T2 values of PCA lesions was consistent with the typical mpMRI characteristics of PCA, which included a high signal in T1WI and a low signal in T2WI (28). This can be explained by the normal prostate glands undergoing carcinomatous transformation having densely arranged tumor cells replace loosely arranged acinar tissue, resulting in a decrease of mucin and fluid content, leading to a decrease of T1 and T2 values on the corresponding quantitative parameters maps (29,30). The contrast of PDWI is determined by the PD within the tissues and reflects the amount of water molecules (31,32). We found that compared with normal prostate glandular tissue, inadequately formed glandular architecture in PCA lesions leads to an increase in epithelial cytoplasm and a decrease in luminal space (33), resulting in a decrease in PD. Although SyMRI quantitative parameters did contribute to the differentiation of PCA from benign lesions, the overall diagnostic efficacy did not surpass that of the ADC value. However, the DWI sequence with high b-value has a relatively low signal-to-noise ratio and is highly susceptible to magnetic field inhomogeneities that produce artifacts or anatomical distortion (34); therefore, SyMRI can partially compensate for these limitations and increases radiologist confidence in diagnosis.
Assessment of T1, T2, PD, and ADC values as predictors of aggressiveness in PCA
We further found that the T1, T2, and PD values in the intermediate-to-high-risk PCA group were significantly higher than those in the low-risk group, while their diagnostic performance was similar to that of the ADC value. In the lesions of the intermediate-to-high-risk PCA group, there were more loosely structured glands, such as the cribriform and glomeruloid glands, which have abundant water content and high molecular mobility, similar to the normal tissue in the PZ (35). Therefore, we suggest that these special glandular components contribute to the higher T1, T2, and PD values in intermediate-to-high-risk PCA as compared to low-risk PCA. Furthermore, the hydrogen proton number increased with increasing cell density, leading to the significantly higher PD value in the intermediate-to-high risk PCA group. However, the T1 values (1,354±101.66) of seven patients with GS =3+3 were higher than those with other grades of PCA. Our analysis suggests that this is due to the better differentiation of GS =3+3 PCA, whose cancer tissue accounted for 15–20% of the total, while atrophic glands, interstitial inflammatory cells, and normal prostate tissue accounted for about 80%. Therefore, the T1 values of GS =3+3 are similar to those of BPH and inflammation.
Another study also indicated that the unique structure of cribriform glands in PCA results in less obvious diffusion restriction on DWI and higher signal intensity on T2WI (36). Therefore, the PCA detection rate of the cribriform glandular structure under mpMRI is relatively low (37). As SyMRI is able to achieve different contrasts, when combined with quantitative parameter maps, it displays clearer lesion margins and more regions of suspicious signal, as shown in Figure 2, with a potential to probe the anatomical details of the prostate lesions. T1, T2, and PD values, as inherent tissue parameters, indirectly reflect tissue composition and pathophysiological information. For distinguishing low-risk from intermediate-to-high risk PCA, the ADC+Sy(T2+PD) combined model yielded an increase in AUC of 0.112 compared to that of ADC alone, thus demonstrating the potential to serve as a noninvasive prognostic biomarker for the assessment of PCA.
SyMRI parameter maps combined with ADC in supporting the prediction of postoperative GS upgrade in patients with low-risk PCA
Due to the histological heterogeneity of PCA, biopsy results may not reflect the complete tumor profile, leading to a potential for missed diagnoses of highly aggressive PCA lesions, with an estimated probability of 25% (38). However, the treatment for different aggressiveness of PCA varies. Those with low-risk PCA can experience good prognosis, and active surveillance is recommended to avoid overtreatment; meanwhile, intermediate-to-high risk PCA requires lymph node dissection during RP because of a higher rate of lymph node metastasis (39).
Our study found that the T1, T2, and PD values obtained from SyMRI exhibited similar diagnostic performance to that of ADC in predicting the postoperative upgrade of low-risk PCA, and the ADC+Sy(T2+PD) combined model outperformed ADC alone. Based on the inherent pathological and physiological characteristics of prostate lesions, SyMRI quantitative parameters maps possess the potential to accurately demonstrate tumor heterogeneity and better identify target regions for biopsy, improving the detection of highly aggressive lesions. Considering the relatively low time cost and high reproducibility of SyMRI technology, the combination of ADC values and SyMRI quantitative parameters may be a better approach to predicting GS upgrade and optimizing PCA diagnosis and treatment, ultimately benefiting patients. In the era of precision medicine, SyMRI quantitative technology is also expected to provide more objective and accurate information for cutting-edge technologies such as big data analysis, medical artificial intelligence, and radiomics, broadening the prospects for development.
Limitations
This study had the following limitations: (I) the sample size of the upgraded PCA group and nonupgraded group was small. (II) As a single-center design was employed, we expect further multicenter studies with larger sample sizes to further validate the results. (III) There might have been a partial mismatch between the ROI and the pathological region, but this is an inherent systematic error that cannot be completely avoided in any biopsy method. (IV) There were four patients with low-risk PCA who only underwent biopsy in this study, which carries a risk of GS upgrade. However, these patients only represented 2.2% (4/178) of the sample and had a minimal impact on the research results. (V) Only 3.4% (6/178) of our patient sample underwent risk downgrade after RP, and thus the predictive value of the SyMRI technique for the presence of possible downgrade was not explored.
Conclusions
The quantitative MRI parameters (T1, T2 and PD) obtained from SyMRI make negligible contributions to differentiating PCA from non-PCA beyond that of ADC alone. However, the performance of T1, T2, and PD in distinguishing low-risk and intermediate-to-high-risk PCA is similar to that of ADC, but their combination with ADC yields a significant improvement. Considering the low time requirement and high reproducibility of SyMRI, the combination of mpMRI and SyMRI may be a feasible approach to assessing the aggressiveness of PCA and predicting postoperative GS upgrade in patients with low-risk PCA.
Acknowledgments
Funding: This work was supported by
Footnote
Reporting Checklist: The authors have completed the STROBE reporting checklist. Available at https://qims.amegroups.com/article/view/10.21037/qims-24-291/rc
Conflicts of Interest: All authors have completed the ICMJE uniform disclosure form (available at https://qims.amegroups.com/article/view/10.21037/qims-24-291/coif). The authors have no conflicts of interest to declare.
Ethical Statement: The authors are accountable for all aspects of the work in ensuring that questions related to the accuracy or integrity of any part of the work are appropriately investigated and resolved. This study was conducted in accordance with the Declaration of Helsinki (as revised in 2013) and was approved by the institutional review board of the First Affiliated Hospital of Nanjing Medical University (No. 2023-SR-892). The requirement of individual consent for this retrospective analysis was waived.
Open Access Statement: This is an Open Access article distributed in accordance with the Creative Commons Attribution-NonCommercial-NoDerivs 4.0 International License (CC BY-NC-ND 4.0), which permits the non-commercial replication and distribution of the article with the strict proviso that no changes or edits are made and the original work is properly cited (including links to both the formal publication through the relevant DOI and the license). See: https://creativecommons.org/licenses/by-nc-nd/4.0/.
References
- Sung H, Ferlay J, Siegel RL, Laversanne M, Soerjomataram I, Jemal A, Bray F. Global Cancer Statistics 2020: GLOBOCAN Estimates of Incidence and Mortality Worldwide for 36 Cancers in 185 Countries. CA Cancer J Clin 2021;71:209-49. [Crossref] [PubMed]
- Turkbey B, Purysko AS. PI-RADS: Where Next? Radiology 2023;307:e223128. [Crossref] [PubMed]
- Epstein JI, Egevad L, Amin MB, Delahunt B, Srigley JR, Humphrey PA. Grading Committee. The 2014 International Society of Urological Pathology (ISUP) Consensus Conference on Gleason Grading of Prostatic Carcinoma: Definition of Grading Patterns and Proposal for a New Grading System. Am J Surg Pathol 2016;40:244-52. [Crossref] [PubMed]
- Morash C, Tey R, Agbassi C, Klotz L, McGowan T, Srigley J, Evans A. Active surveillance for the management of localized prostate cancer: Guideline recommendations. Can Urol Assoc J 2015;9:171-8. [Crossref] [PubMed]
- Alenda O, Ploussard G, Mouracade P, Xylinas E, de la Taille A, Allory Y, Vordos D, Hoznek A, Abbou CC, Salomon L. Impact of the primary Gleason pattern on biochemical recurrence-free survival after radical prostatectomy: a single-center cohort of 1,248 patients with Gleason 7 tumors. World J Urol 2011;29:671-6. [Crossref] [PubMed]
- Amin A, Partin A, Epstein JI. Gleason score 7 prostate cancer on needle biopsy: relation of primary pattern 3 or 4 to pathological stage and progression after radical prostatectomy. J Urol 2011;186:1286-90. [Crossref] [PubMed]
- Cole AI, Morgan TM, Spratt DE, Palapattu GS, He C, Tomlins SA, Weizer AZ, Feng FY, Wu A, Siddiqui J, Chinnaiyan AM, Montgomery JS, Kunju LP, Miller DC, Hollenbeck BK, Wei JT, Mehra R. Prognostic Value of Percent Gleason Grade 4 at Prostate Biopsy in Predicting Prostatectomy Pathology and Recurrence. J Urol 2016;196:405-11. [Crossref] [PubMed]
- Kamel MH, Khalil MI, Alobuia WM, Su J, Davis R. Incidence of metastasis and prostate-specific antigen levels at diagnosis in Gleason 3+4 versus 4+3 prostate cancer. Urol Ann 2018;10:203-8. [Crossref] [PubMed]
- Sarici H, Telli O, Yigitbasi O, Ekici M, Ozgur BC, Yuceturk CN, Eroglu M. Predictors of Gleason score upgrading in patients with prostate biopsy Gleason score ≤6. Can Urol Assoc J 2014;8:E342-6. [Crossref] [PubMed]
- Hambrock T, Somford DM, Huisman HJ, van Oort IM, Witjes JA, Hulsbergen-van de Kaa CA, Scheenen T, Barentsz JO. Relationship between apparent diffusion coefficients at 3.0-T MR imaging and Gleason grade in peripheral zone prostate cancer. Radiology 2011;259:453-61. [Crossref] [PubMed]
- Vargas HA, Akin O, Franiel T, Mazaheri Y, Zheng J, Moskowitz C, Udo K, Eastham J, Hricak H. Diffusion-weighted endorectal MR imaging at 3 T for prostate cancer: tumor detection and assessment of aggressiveness. Radiology 2011;259:775-84. [Crossref] [PubMed]
- Verma S, Rajesh A, Morales H, Lemen L, Bills G, Delworth M, Gaitonde K, Ying J, Samartunga R, Lamba M. Assessment of aggressiveness of prostate cancer: correlation of apparent diffusion coefficient with histologic grade after radical prostatectomy. AJR Am J Roentgenol 2011;196:374-81. [Crossref] [PubMed]
- Nezzo M, Di Trani MG, Caporale A, Miano R, Mauriello A, Bove P, Capuani S, Manenti G. Mean diffusivity discriminates between prostate cancer with grade group 1&2 and grade groups equal to or greater than 3. Eur J Radiol 2016;85:1794-801. [Crossref] [PubMed]
- Pang Y, Turkbey B, Bernardo M, Kruecker J, Kadoury S, Merino MJ, Wood BJ, Pinto PA, Choyke PL. Intravoxel incoherent motion MR imaging for prostate cancer: an evaluation of perfusion fraction and diffusion coefficient derived from different b-value combinations. Magn Reson Med 2013;69:553-62. [Crossref] [PubMed]
- Tamura C, Shinmoto H, Soga S, Okamura T, Sato H, Okuaki T, Pang Y, Kosuda S, Kaji T. Diffusion kurtosis imaging study of prostate cancer: preliminary findings. J Magn Reson Imaging 2014;40:723-9. [Crossref] [PubMed]
- Cui Y, Han S, Liu M, Wu PY, Zhang W, Zhang J, Li C, Chen M. Diagnosis and Grading of Prostate Cancer by Relaxation Maps From Synthetic MRI. J Magn Reson Imaging 2020;52:552-64. [Crossref] [PubMed]
- Moya-Sáez E, Peña-Nogales Ó, Luis-García R, Alberola-López C. A deep learning approach for synthetic MRI based on two routine sequences and training with synthetic data. Comput Methods Programs Biomed 2021;210:106371. [Crossref] [PubMed]
- Warntjes JB, Leinhard OD, West J, Lundberg P. Rapid magnetic resonance quantification on the brain: Optimization for clinical usage. Magn Reson Med 2008;60:320-9. [Crossref] [PubMed]
- Ji S, Yang D, Lee J, Choi SH, Kim H, Kang KM. Synthetic MRI: Technologies and Applications in Neuroradiology. J Magn Reson Imaging 2022;55:1013-25. [Crossref] [PubMed]
- DeLong ER, DeLong DM, Clarke-Pearson DL. Comparing the areas under two or more correlated receiver operating characteristic curves: a nonparametric approach. Biometrics 1988;44:837-45. [Crossref] [PubMed]
- Bojorquez JZ, Bricq S, Brunotte F, Walker PM, Lalande A. A novel alternative to classify tissues from T 1 and T 2 relaxation times for prostate MRI. MAGMA 2016;29:777-88. [Crossref] [PubMed]
- Zaitsev M, Maclaren J, Herbst M. Motion artifacts in MRI: A complex problem with many partial solutions. J Magn Reson Imaging 2015;42:887-901. [Crossref] [PubMed]
- Callaghan MF, Mohammadi S, Weiskopf N. Synthetic quantitative MRI through relaxometry modelling. NMR Biomed 2016;29:1729-38. [Crossref] [PubMed]
- Hagiwara A, Hori M, Cohen-Adad J, Nakazawa M, Suzuki Y, Kasahara A, Horita M, Haruyama T, Andica C, Maekawa T, Kamagata K, Kumamaru KK, Abe O, Aoki S. Linearity, Bias, Intrascanner Repeatability, and Interscanner Reproducibility of Quantitative Multidynamic Multiecho Sequence for Rapid Simultaneous Relaxometry at 3 T: A Validation Study With a Standardized Phantom and Healthy Controls. Invest Radiol 2019;54:39-47. [Crossref] [PubMed]
- Mai J, Abubrig M, Lehmann T, Hilbert T, Weiland E, Grimm MO, Teichgräber U, Franiel T. T2 Mapping in Prostate Cancer. Invest Radiol 2019;54:146-52. [Crossref] [PubMed]
- Kitajima K, Takahashi S, Ueno Y, Yoshikawa T, Ohno Y, Obara M, Miyake H, Fujisawa M, Sugimura K. Clinical utility of apparent diffusion coefficient values obtained using high b-value when diagnosing prostate cancer using 3 tesla MRI: comparison between ultra-high b-value (2000 s/mm2) and standard high b-value (1000 s/mm2). J Magn Reson Imaging 2012;36:198-205. [Crossref] [PubMed]
- Rosenkrantz AB, Hindman N, Lim RP, Das K, Babb JS, Mussi TC, Taneja SS. Diffusion-weighted imaging of the prostate: Comparison of b1000 and b2000 image sets for index lesion detection. J Magn Reson Imaging 2013;38:694-700. [Crossref] [PubMed]
- O'Shea A, Harisinghani M. PI-RADS: multiparametric MRI in prostate cancer. MAGMA 2022;35:523-32. [Crossref] [PubMed]
- Mitchell DG, Burk DL Jr, Vinitski S, Rifkin MD. The biophysical basis of tissue contrast in extracranial MR imaging. AJR Am J Roentgenol 1987;149:831-7. [Crossref] [PubMed]
- Langer DL, van der Kwast TH, Evans AJ, Sun L, Yaffe MJ, Trachtenberg J, Haider MA. Intermixed normal tissue within prostate cancer: effect on MR imaging measurements of apparent diffusion coefficient and T2--sparse versus dense cancers. Radiology 2008;249:900-8. [Crossref] [PubMed]
- Herskovits EH, Itoh R, Melhem ER. Accuracy for detection of simulated lesions: comparison of fluid-attenuated inversion-recovery, proton density--weighted, and T2-weighted synthetic brain MR imaging. AJR Am J Roentgenol 2001;176:1313-8. [Crossref] [PubMed]
- Gracien RM, Reitz SC, Hof SM, Fleischer V, Zimmermann H, Droby A, Steinmetz H, Zipp F, Deichmann R, Klein JC. Changes and variability of proton density and T1 relaxation times in early multiple sclerosis: MRI markers of neuronal damage in the cerebral cortex. Eur Radiol 2016;26:2578-86. [Crossref] [PubMed]
- Zhao M, Myint E, Watson G, Bourne R. Comparison of conventional histology and diffusion weighted microimaging for estimation of epithelial, stromal, and acinar volumes in prostate tissue. In: Proceedings of the Twenty-First Meeting of the International Society for Magnetic Resonance in Medicine, Berkeley, Calif: International Society for Magnetic Resonance in Medicine, 2013:abstr 3090.
- Tamada T, Ueda Y, Ueno Y, Kojima Y, Kido A, Yamamoto A. Diffusion-weighted imaging in prostate cancer. MAGMA 2022;35:533-47. [Crossref] [PubMed]
- Kazan O, Gunduz N, Kir G, Iplikci A, Dogan MB, Cecikoglu GE, Culpan M, Yildirim A. The cribriform morphology impairs Gleason 7 prostate cancer lesion detection on multiparametric magnetic resonance imaging. Prostate 2023;83:331-9. [Crossref] [PubMed]
- Downes MR, Gibson E, Sykes J, Haider M, van der Kwast TH, Ward A. Determination of the Association Between T2-weighted MRI and Gleason Sub-pattern: A Proof of Principle Study. Acad Radiol 2016;23:1412-21. [Crossref] [PubMed]
- Bernardino R, Fleshner N. Re: Sensitivity of Multiparametric MRI and Targeted Biopsy for Detection of Adverse Pathologies (Cribriform Gleason Pattern 4 and Intraductal Carcinoma): Correlation of Detected and Missed Prostate Cancer Foci with Whole Mount Histopathology. Eur Urol 2023;83:583-4. [Crossref] [PubMed]
- Epstein JI, Feng Z, Trock BJ, Pierorazio PM. Upgrading and downgrading of prostate cancer from biopsy to radical prostatectomy: incidence and predictive factors using the modified Gleason grading system and factoring in tertiary grades. Eur Urol 2012;61:1019-24. [Crossref] [PubMed]
- Eifler JB, Feng Z, Lin BM, Partin MT, Humphreys EB, Han M, Epstein JI, Walsh PC, Trock BJ, Partin AW. An updated prostate cancer staging nomogram (Partin tables) based on cases from 2006 to 2011. BJU Int 2013;111:22-9. [Crossref] [PubMed]