An integrated nomogram combining clinical and radiomic features of hyperattenuated imaging markers to predict malignant cerebral edema following endovascular thrombectomy
Introduction
Endovascular thrombectomy (EVT) is a well-established treatment for anterior circulation stroke with large-vessel occlusion. Despite its success, a considerable portion of patients (approximately 45%) still experience poor functional outcomes following the procedure (1). One of the major complications is malignant cerebral edema (MCE), which occurs in up to 75% of cases (2) and is associated with an 80% mortality rate, which can offset the benefits of EVT (3). MCE typically manifests within 2–5 days postthrombectomy (4). Timely recognition of individuals susceptible to MCE is crucial for implementing appropriate treatments and interventions to mitigate complications, such as edema, brain herniation, and secondary injuries, and to improve patient outcomes (5). Several factors have been identified as potential predictors of MCE, including age, National Institutes of Health Stroke Scale (NIHSS) score, successful revascularization, cerebral blood supply, history of hypertension, area of cerebral ischemia, and etiology of ischemic stroke (2). However, current prediction models for MCE after EVT have not yet achieved satisfactory accuracy (6).
The hyperattenuated imaging marker (HIM) has been observed in 31.2% to 60.0% of patients in immediate post-EVT computed tomography (CT) images (7). HIM is characterized by hyperattenuation on head noncontrast CT (NCCT) immediately after the procedure, likely due to blood-brain barrier disruption (8). Studies have shown that HIM is associated with an increased risk of adverse functional outcomes at 90 days, suggesting its potential as a prognostic indicator following EVT (9). However, previous studies have not yielded satisfactory prediction results, specifically for MCE risk (10,11).
Radiomics analysis is an emerging technique in precision medicine that enables the automated extraction of radiomics features from clinical images. This approach can potentially overcome the limitations associated with visual image assessment and improve the prediction and evaluation of neurological diseases (12). Although prior studies have explored the application of radiomics analysis to predict factors such as hemorrhage and poor functional recovery in postthrombectomy stroke outcomes, there is a lack of research specifically focusing on the prediction or evaluation of MCE following thrombectomy based on HIM (13). Therefore, studies that explore the potential of radiomics analysis in this particular context are warranted.
This study thus aimed to examine the association between radiomics features extracted from immediate post-EVT CT images and the development of MCE in patients with acute anterior circulation cerebral infarction. Furthermore, we aimed to develop a predictive model for MCE and assess its performance. We present this article in accordance with the TRIPOD reporting checklist (available at https://qims.amegroups.com/article/view/10.21037/qims-24-99/rc).
Methods
Ethical approval of the study protocol
This study was conducted in accordance with the Declaration of Helsinki (as revised in 2013) and was approved by the Ethics Committee of the Fourth Affiliated Hospital of School of Medicine, and International School of Medicine, International Institutes of Medicine, Zhejiang University (approval No. Y2024029). The requirement for individual consent in this retrospective analysis was waived.
Data acquisition
Individuals who received EVT for large-vessel occlusive stroke in the anterior circulation at the Fourth Affiliated Hospital of School of Medicine, and International School of Medicine, International Institutes of Medicine, Zhejiang University, between September 2016 and November 2021, were included in this retrospective study. The inclusion and exclusion criteria for EVT and thrombolysis were based on the most recent guidelines (14,15). Data on general clinical features, laboratory examinations, clinical presentations, and imaging were analyzed.
Patients were included if they had head NCCT following mechanical thrombectomy, with the scan performed within 30 minutes of the procedure and showing HIM, which is characterized by increased attenuation in the brain parenchyma and/or subarachnoid space. The exclusion criteria were as follows: (I) a follow-up time of NCCT after mechanical thrombectomy not within the range of 2–5 days, (II) artifacts (e.g., metal artifacts or motion artifacts) affecting the judgment of HIM or MCE, and (III) patients with posterior circulation stroke. Figure 1 displays a flowchart of participant inclusion in this study.
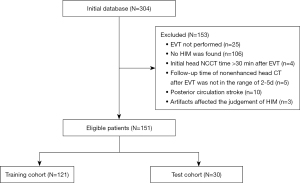
CT data acquisition
Images of the first postthrombectomy head nonenhanced CT were obtained within 30 minutes after thrombectomy from a 64-row spiral CT scanner (SOMATOM Definition AS, Siemens Healthineers, Erlangen, Germany) and a 62-row spiral scanner (Optima CT620, GE HealthCare, Chicago, IL, USA). The scanning parameters were as follows: axial mode, tube voltage 120 kV, tube current 250–300 mAs, scanning range from the skull base to the cranial roof, section thickness 5 mm, and reconstruction with the standard algorithm. Imaging data were evaluated randomly by two neuroradiologists with more than 5 years of working experience who were blinded to the clinical situation. For any discrepancies, the two evaluators discussed the images to reach a consensus.
Identification of HIM and MCE
HIM was evaluated on NCCT immediately after EVT. MCE was determined 2–5 days on follow-up NCCT following EVT therapy. In this study, two neuroradiology staff members (S.H. and Z.W., both with over 5 years of experience in neuroradiology) independently evaluated all the training and test datasets without knowledge of the patient outcome. Any discrepancy was resolved by consensus. We conducted a review of the NCCT images obtained after EVT. The development of MCE was defined as the occurrence of midline brain shift, specifically a displacement of the septum pellucidum of ≥5 mm, within the first 5 days after admission.
Data preprocessing
In this study, 151 patients were enrolled, with 121 cases randomly assigned to the training cohort and 30 patients to the test cohort. Patient charts, procedure notes, and follow-up notes were reviewed to extract baseline and preprocedural factors including age, sex, history of drinking or smoking, hypertension, hyperlipidemia, diabetes mellitus, atrial fibrillation, coronary artery disease, and baseline NIHSS score. MCE imaging markers, such as midline shift (MLS), were obtained from the patient’s medical records. Two experienced neuroradiologists, each with over 5 years of experience, determined the Baseline Alberta Stroke Program Early Computed Tomography Score (ASPECTS) in both the anterior and posterior circulation, as well as the maximum Hounsfield unit (HUmax), through discussions while being unaware of the patient’s clinical information. Furthermore, we collected modified thrombolysis in cerebral infarction (mTICI) scores and various preinterventional parameters, including intravenous thrombolysis therapy. Additionally, interventional parameters were recorded, such as the percentage of stent implantation, stent pass number, and the type of stent.
Radiomics feature extraction
The NCCT images were reconstructed with a voxel size of 1×1×1 mm3 and gray-scale discretization to standardize the images acquired by different CT scanners. Two radiologists independently segmented HIM using a double-blind approach, and their measurements were compared to calculate the intraclass correlation coefficient (ICC). An ICC value of ≥0.75 was considered robust. To extract radiomic features from the HIM in the NCCT images, we use Pyradiomic’s internal feature analysis software (http://pyradiomics.readthedocs.io). A total of 1834 radiomics features were extracted from the NCCT images. These features were derived using various texture feature extraction methods, including first-order statistics, shape-based features, gray-level co-occurrence matrix (GLCM), gray-level run length matrix (GLRLM), neighboring gray-tone difference matrix (NGTDM), gray-level size zone matrix (GLSZM), and gray-level dependence matrix (GLDM).
Development of the radiomics signature
The feature dimensions were reduced, as outlined below, to minimize radiomics bias. Characteristics demonstrating strong agreement within and between observers, as indicated by an ICC exceeding 0.75, were chosen. Following this, a Mann-Whitney test was conducted, and characteristics with a P value less than 0.05 were retained. Furthermore, the Spearman rank correlation coefficient was computed to evaluate the correlation between features that exhibited strong repeatability. If the correlation coefficient between any two features exceeded 0.9, we retained one of the features.
In addition, a methodical recursive elimination approach was employed for feature selection, in which the feature with the highest overlap in the existing set was removed in each step. Following this, the least absolute shrinkage and selection operator (LASSO) regression model was used on the discovery dataset to create a signature. LASSO shrinks regression coefficients toward zero and sets coefficients of irrelevant features to zero based on the regularization weight λ. The optimal λ value was determined using 10-fold cross-validation with the minimum criteria, with the value that yielded the lowest cross-validation error being selected. To build the radiomics signatures, regression models were fitted through an analysis of the features that had nonzero coefficients. The radiomics score (Rad-score) for every patient was calculated as a linear combination of the selected features weighted by their respective LASSO coefficients.
Various radiomics models were created and evaluated with seven machine learning classification algorithms, including logistic regression (LR), support vector machine (SVM), k-nearest neighbor (KNN), random forest (RF), extremely randomized trees (Extra-Trees), extreme gradient boosting (XGBoost), and light gradient boosting machine (LightGBM). Among these models, the XGBoost machine learning model demonstrated a balanced average area under the curve (AUC) on the test set for both the radiomics model and clinical model. The chosen characteristics were then entered into the XGBoost algorithm to create the risk prediction model. The final radiomics signature was obtained through a fivefold cross-validation process. Figure 2 illustrates the detailed process of model building.
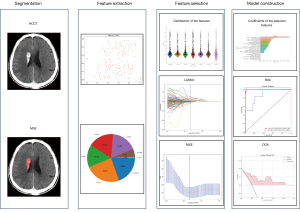
Development of the clinical signature
Univariable LR analysis was conducted to investigate the clinical factors associated with predicting MCE in the training cohort. Significant variables identified from the univariable analysis were then included in a multivariable LR analysis using a backward stepwise selection procedure, with a P value threshold of <0.05 as the retention criteria. Subsequently, the chosen clinical characteristics were entered into the XGBoost machine learning model that was previously employed for the radiomics signature in order to develop the risk model, and fivefold cross-validation was conducted to obtain the final clinical markers.
Construction of the clinical radiomics nomogram
The clinical radiomics nomogram was created using LR analysis. The AUC was used to evaluate the diagnostic accuracy of the clinical model, radiomics model, and clinical radiomics nomogram in both the training and test cohorts.
Statistics analysis
Statistical analysis was conducted using SPSS version 26.0 (IBM Corp., Armonk, NY, USA). The normality of the evaluated variables was assessed using the Kolmogorov-Smirnov and Shapiro-Wilk tests. Continuous variables are expressed as means with standard deviations, and categorical variables are expressed as frequency counts and percentages. Categorical variables were compared using chi-square or Fisher exact tests, while continuous variables were compared using the Mann-Whitney tests or independent t-test. Python 3.11.1 (Python Software Foundation, Wilmington, DE, USA) was used for extracting features, screening, and constructing models. The “rms” (regression modeling strategies) package in R (The R Foundation for Statistical Computing) was employed to develop the nomogram. The three nomograms were assessed based on metrics including AUC with the 95% CI, accuracy, sensitivity, and specificity. For all tests, a P value less than 0.05 was deemed to be statistically significant.
Results
Patient characteristics
This study included 151 patients, comprising 121 patients in the training cohort and 30 patients in the test cohort. The baseline demographic, clinical, and imaging characteristics of the patients are summarized in Table 1. Among the 151 patients, 28 developed MCE. There were no significant differences in clinical characteristics between the training and test cohorts.
Table 1
Feature name | Training | Test | P value |
---|---|---|---|
Age (years), mean ± SD | 67.60±14.01 | 65.67±16.22 | 0.514 |
Males, n (%) | 75 (61.98) | 20 (66.67) | 0.637 |
Atrial fibrillation, n (%) | 45 (37.19) | 4 (13.33) | 0.778 |
Hypertension, n (%) | 69 (57.02) | 16 (53.33) | 0.717 |
Hyperlipidemia, n (%) | 2 (1.65) | 2 (6.67) | 0.128 |
Diabetes mellitus, n (%) | 13 (10.74) | 3 (10.00) | 0.690 |
Coronary artery disease, n (%) | 14 (11.57) | 6 (20.00) | 0.225 |
Drinking, n (%) | 24 (19.83) | 6 (20.00) | 0.984 |
Smoking, n (%) | 25 (20.66) | 10 (33.33) | 0.143 |
Thrombolysis, n (%) | 50 (41.32) | 13 (43.33) | 0.843 |
Baseline NIHSS, median [Q1, Q3] | 15 [13, 18] | 15 [11, 20] | 0.106 |
ASPECTS, median [Q1, Q3] | 9 [8, 10] | 8 [6, 9] | 0.753 |
HUmax ≥90, n (%) | 39 (32.23) | 9 (30.00) | 0.816 |
mTICI beyond 2b, n (%) | 108 (89.26) | 27 (90.00) | 0.906 |
Stent type, n (%) | 0.482 | ||
Solitaire | 72 (59.50) | 15 (50.00) | |
Trevo | 21 (17.36) | 6 (20.00) | |
Solitaire + Trevo | 11 (9.09) | 6 (20.00) | |
Others | 17 (14.05) | 3 (10.00) | |
Pass number, median [Q1, Q3] | 2 [1, 3] | 2 [1, 4] | 0.541 |
Stent implantation, n (%) | 26 (21.49) | 5 (16.67) | 0.561 |
SD, standard deviation; NIHSS, National Institutes of Health Stroke Scale; Q1, first quartile; Q3, third quartile; ASPECTS, Alberta Stroke Program Early Computed Tomography Score; HUmax, maximum Hounsfield unit; mTICI, modified thrombolysis in cerebral infarction.
Evaluation and development of the clinical signatures
The results of the univariate analysis for clinical risk factors associated with MCE in the training cohort are presented in Table 2. After multivariate LR, two clinical features were selected: HUmax ≥90 and ASPECTS. These two features were then used to establish the clinical signature for predicting the occurrence of MCE.
Table 2
Feature name | Non-MCE | MCE | P value |
---|---|---|---|
Age (years), mean ± SD | 67.86±13.29 | 67.07±14.74 | 0.794 |
Males, n (%) | 74 (61.16) | 21 (75.00) | 0.144 |
Atrial fibrillation, n (%) | 48 (39.02) | 9 (32.14) | 0.501 |
Hypertension, n (%) | 70 (56.91) | 16 (57.14) | 0.920 |
Hyperlipidemia, n (%) | 2 (1.63) | 2 (7.14) | 0.102 |
Diabetes mellitus, n (%) | 13 (10.57) | 4 (14.29) | 0.577 |
Coronary artery disease, n (%) | 15 (12.20) | 5 (17.86) | 0.428 |
Drinking, n (%) | 22 (17.89) | 8 (28.57) | 0.203 |
Smoking, n (%) | 27 (21.95) | 8 (28.57) | 0.457 |
Thrombolysis, n (%) | 53 (43.09) | 10 (35.71) | 0.478 |
Baseline NIHSS, median [Q1, Q3] | 15 [13, 18] | 15 [11, 20] | 0.942 |
ASPECTS, median [Q1, Q3] | 9 [8, 10] | 8 [6, 9] | 0.003* |
HUmax ≥90, n (%) | 30 (24.39) | 18 (64.29) | 0.000* |
mTICI beyond 2b, n (%) | 111 (90.24) | 24 (85.71) | 0.485 |
Stent type, n (%) | 0.217 | ||
Solitaire | 67 (54.47) | 20 (71.43) | |
Trevo | 25 (20.33) | 2 (7.14) | |
Solitaire + Trevo | 13 (10.57) | 4 (14.29) | |
Others | 18 (14.63) | 2 (7.14) | |
Pass number, median [Q1, Q3] | 2 [1, 3] | 2 [1, 4] | 0.015* |
Stent implantation, n (%) | 27 (21.95) | 4 (14.29) | 0.368 |
*, P<0.05. MCE, malignant cerebral edema; SD, standard deviation; NIHSS, National Institutes of Health Stroke Scale; Q1, first quartile; Q3, third quartile; ASPECTS, Alberta Stroke Program Early Computed Tomography Score; HUmax, maximum Hounsfield unit; mTICI, modified thrombolysis in cerebral infarction.
Extraction, selection, and construction of the radiomics signatures
Initially, a total of 1,834 radiomics characteristics were extracted from the reconstructed images of NCCT in this study. An assessment of intraobserver and interobserver reproducibility was conducted to ensure the reliability of these features. Among the extracted features, 1,681 (ICC >0.75) demonstrated satisfactory reproducibility and were retained for further analysis. Pairs with high correlations were omitted to reduce redundancy and multicollinearity among the features. This step resulted in a final set of 219 features per patient for subsequent selection. After LASSO analysis, a total of 22 significant features were identified and used to construct the radiomics signature. The optimal parameter, which controls the degree of feature selection, was found to be 0.0193 (Figure 3A,3B). Figure 3C illustrates the 22 selected features along with their corresponding weights.
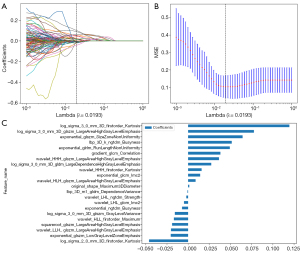
Establishment of the clinical radiomics nomogram
The combined clinical radiomics nomogram used to calculate the risk MCE occurrence is depicted in Figure 4. To calculate the risk of MCE, each influencing factor is assigned a score based on its contribution to the overall risk. The sum of these scores is used to determine the overall value. From the total score, a line is drawn to the risk axis on the nomogram, allowing for the determination of the corresponding MCE risk. A greater MCE risk is correlated with a higher total score.
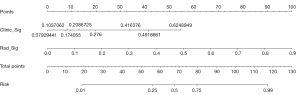
Performance of the clinical, radiomics, and combined nomograms
The diagnostic accuracy of the clinical, radiomics, and combined nomograms is outlined in Table 3. The AUC values in the training group were 0.999 for the model that combined both radiomics and clinical data, 0.999 for the radiomics-only model, and 0.790 for the clinical data-only model. Following validation, the AUC scores were 0.938 for the integrated model, 0.868 for the clinical model, and 0.875 for the radiomics model, as shown in Table 3 and Figure 5.
Table 3
Model | Training cohort (n=121) | Test cohort (n=30) | |||||
---|---|---|---|---|---|---|---|
AUC (95% CI) | Sensitivity* | Specificity* | AUC (95% CI) | Sensitivity* | Specificity* | ||
Clinical model | 0.790 (0.683–0.898) | 0.455 | 0.909 | 0.868 (0.738–0.998) | 0.833 | 0.792 | |
Radiomics model | 0.999 (0.996–1.000) | 0.955 | 0.980 | 0.875 (0.730–1.000) | 0.667 | 0.792 | |
Radiomics + clinical model | 0.999 (0.996–1.000) | 0.955 | 0.970 | 0.938 (0.834–1.000) | 0.667 | 0.917 |
*, balanced sensitivity and specificity at the cutoff yielding the largest Youden index value. AUC, area under the curve; CI, confidence interval.
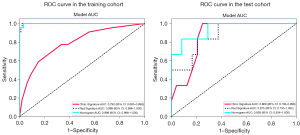
Discussion
In this study, a nomogram was developed and validated to predict the occurrence of MCE after EVT. The nomogram combines clinical features highly correlated with MCE and five robust radiomics features extracted from HIM images on CT immediately after EVT. Our findings demonstrate the nomogram’s relatively high predictive power, with AUC values of 0.999 and 0.938 for the training and test groups, respectively. Importantly, this study is the first to use a combination of clinical variables and HIM-based radiomics to identify patients at risk of developing MCE.
MCE following EVT occurs due to the altered permeability of the blood-brain barrier, disrupting tight junctions between endothelial cells and forming ionic or vasogenic edema (16). The severity of blood-brain barrier disruption is directly associated with the likelihood of MCE. In this study, the clinical model incorporated two clinical features that exhibited the highest correlation with MCE: ASPECTS score and HUmax ≥90. The test set of the clinical model demonstrated a strong correlation between these two features and the occurrence of MCE, with an AUC of 0.868. Previous studies have also indicated that lower ASPECTS scores are linked to a higher risk of MCE (17). The ASPECTS score reflects the extent of early ischemic changes on CT and can indicate the severity of the stroke. A lower ASPECTS score suggests a larger area of ischemia and may indicate a higher risk of developing MCE. The HUmax of a patient’s HIM reflects the degree of blood-brain barrier damage, with a higher HUmax value indicating more severe blood-brain barrier disruption. When the HUmax exceeds a 90-HU threshold, this suggests significant blood-brain barrier damage and a higher risk of developing MCE (18). Incorporating these clinical features into the model made it possible to predict the risk of MCE after EVT more accurately. This information can help guide clinical decision-making and potentially improve patient outcomes through the identification of patients at a higher risk of developing MCE.
Previous research has established that the extent and severity of HIM are associated with cerebral hemorrhage and poor clinical outcomes in patients who have undergone the procedure (19). Additionally, the presence of HIM has been identified as a reliable predictor of MCE (9). A retrospective analysis of 39 patients (11) found that an HIM-to-hemispheric area ratio greater than 0.2 on immediate post-EVT head CT is linked to an increased risk of developing MCE. However, the authors acknowledged that the threshold of 0.2 may vary among patients and may not be universally applicable. Wang et al. (10) investigated the spatial distribution of HIM on post-EVT CT scans within 24 hours, using ASPECTS as a reference. They developed a scoring system that measures the extent of hyperattenuation. This scoring system demonstrated high specificity (0.87), but its sensitivity (0.73) was insufficient for predicting MCE. We developed a predictive tool for MCE that exhibited good performance according to our findings. This tool can be valuable for identifying patients at risk for MCE after EVT.
Previous research has primarily relied on visual assessment of conventional imaging methods, such as CT scans, to extract relevant information. However, these methods may not be optimal for the identifying features of HIM. On the other hand, radiomics analysis allows for a noninvasive assessment of disease heterogeneity by capturing parameters that are not visible to the naked eye, such as entropy, kurtosis, and pixel distribution. These parameters provide insights into angiogenesis, cell density, and necrosis, enhancing our understanding of the disease process (20). CT-based radiomics analysis has shown promising results in various aspects in the management of acute ischemic stroke (AIS). It has been shown to successfully identify AIS lesions, assess the extent of ischemic lesions, and predict symptom onset related to basal ganglia infarction (21,22).
Moreover, radiomics analysis has demonstrated superiority over previous methods in predicting the occurrence of MCE after EVT in those with AIS. Wen et al. (23) developed a predictive model using radiomic features extracted from preoperative NCCT images before EVT. This model outperformed previous models and achieved AUC values of 0.870 and 0.837 for the training and test sets, respectively. However, it is essential to note that the predictive accuracy of preoperative NCCT for postoperative outcomes is limited, which might have resulted in suboptimal effectiveness in this study.
Moreover, we focused on CT images acquired immediately after EVT to construct a radiomics model for predicting the occurrence of MCE. By selecting post-EVT images, we aimed to eliminate the potential impact of preoperative waiting time and unforeseen events during surgery, thus improving the model’s accuracy. From a pool of 1,834 candidate radiomics features, we rigorously selected 22 features that showed a high correlation with MCE. The radiomic features identified, particularly firstorder_Kurtosis, glszm_large area high gray level emphasis (LAHGLE) and exponential_glszm_Size zone non-uniformity, are derived from kurtosis and GLSZM transformations. Kurtosis features describe the sharpness or flatness of the intensity distribution across relevant image regions, while GLSZM features quantify the occurrence of specific gray levels across varying regional sizes within an image. The LAHGLE feature, associated with GLSZM, signifies the presence of larger bright areas in the image and is correlated with a broader range of high-density regions on CT scans. These variations in radiomic features align with the pathophysiological disruption of the blood-brain barrier and cellular damage. These features reflect the heterogeneity of the tissue under investigation and correlate with the degree of blood-brain barrier damage. Betrouni et al. (24) reported there to be a correlation between radiomic features and damaged brain parenchyma in rats, particularly emphasizing the relationship between kurtosis and alterations in neural structures. This suggests the presence of a link between radiomics features and the structural changes resulting from blood-brain barrier damage. In essence, the changes in brain tissue caused by blood-brain barrier disruption can be monitored through radiomic features, which play a crucial role in the pathogenesis of MCE. Previous research has suggested that HIMs of greater intensity and extent are associated with an increased risk of MCE (10,11) and are potentially linked to ionic or vasogenic edema formation following blood-brain barrier damage. Machine learning algorithms have proven to be highly effective in identifying associations and patterns in complex data, surpassing traditional statistical methods such as LR. With increased computing power and storage capacity, machine learning algorithms can quickly analyze complex data and generate accurate outputs. In this study, a machine learning classifier was employed to extract features from the HIM observed on NCCT scans after EVT to predict MCE occurrence. Among the seven machine learning classifiers used, XGBoost demonstrated the highest predictive performance. XGBoost is a boosting algorithm that combines multiple weak classifiers to create a robust classifier. Its built-in regularization feature minimizes output variability and prevents overfitting. Consistent with previous research (25) on low-dimensional structured data analysis, XGBoost exhibited enhanced processing speed and increased accuracy. The radiomics model, which included the XGBoost classifier, performed better than did the clinical model in both the training and test sets. However, the nomogram model achieved the best performance, which combined clinical and radiomics features.
Nomograms function as visual aids in clinical decision-making, simplifying the interpretation of prognostic models. This enables healthcare professionals to intuitively evaluate disease risks and patient outcomes (26). They are particularly instrumental in managing AIS, for which they can predict the efficacy of intravenous thrombolysis (27), estimate the likelihood of recurrence, and assess hemorrhage risk postthrombectomy (28). This can guide the formulation of personalized treatment strategies. MCE, a severe postendovascular thrombectomy complication, can increase mortality risk and neurological deterioration. Our nomogram, which combines radiomics features with clinical data, enhances prediction accuracy and facilitates the early identification of MCE risk factors and the implementation of preventive measures. These measures encompass intracranial pressure monitoring, elevated head position, osmotic therapy with agents such as mannitol or hypertonic saline, therapeutic hypothermia, hyperventilation, and decompressive craniectomy. Nomograms aid in rapidly communicating the need for surgical interventions to medical teams and families, thereby facilitating timely therapeutic decisions and potentially reducing the severity and associated risks of MCE.
This study has several limitations which should be addressed. First, the use of varied CT equipment from our institution for image acquisition might have introduced bias stemming from differences in technology and imaging protocols. Although CT remains the standard for emergency stroke assessment, we acknowledge that integrating additional imaging methods, including MRI, could bolster the robustness of our findings. Moving forward, we intend to incorporate a range of imaging modalities in our future research to enhance the model’s broad applicability and predictive precision. Second, while evidence from the literature supports the stability of the selected radiomic features across various imaging conditions (24,29), we still took measures to ensure the uniformity of image processing, including adopting a standardized slice thickness and window width and level, alongside resampling and normalizing the features. We also use LASSO to minimize the influence of irrelevant and redundant features and employed cross-validation to bolster the model’s reproducibility. However, we recognize that the limitations posed by the small sample size and the imbalanced distribution within groups may reduce the model’s robustness. Future research will focus on increasing the sample size and pursuing multicenter collaborations to address these concerns. Although our study suggests potential correlations between radiomics features and the development of MCE, we acknowledge that these hypotheses need to be validated in further experimental and pathological evaluations. Moreover, we intend to conduct external validation to assess the applicability and accuracy of these models in a variety of clinical scenarios. Third, we acknowledge the inefficiency, subjectivity, and absence of standardized protocols in manual delineation during emergencies, which can affect reproducibility. Therefore, we intend to explore automated or semiautomated methods of segmentation and analytical techniques in the future to enhance efficiency and consistency. Fourth, our study used advanced statistical methods but did not incorporate the latest technologies, such as deep learning. Therefore, we intend to integrate deep learning algorithms into future research to improve the model’s performance and predictive accuracy and to enhance the clinical interpretability of our findings for greater applicability. Fifth, we recognize that our study did not include comprehensive clinical markers, such as serum C-reactive protein, to enhance model precision, nor did we employ outcome metrics such as the 90-day modified Rankin Scale for assessment. We believe that the integration of clinical and outcome indicators will contribute to the development of a more precise and clinically relevant predictive model. Consequently, we plan to incorporate these factors in our future research to provide more valuable information for the clinical management of stroke survivors. Lastly, the clinical application of nomograms presents challenges, including their complexity, which may require additional training for effective utilization, and the risk of overfitting, which could compromise generalizability. Furthermore, biases in variable selection and the constraints of single-center data impede widespread applicability (30). To address these issues, we aim to enhance the model’s practicality and clinical utility by standardizing data, fostering multicenter collaborations, and integrating models within clinical practice, including the development of more user-friendly dynamic nomograms. Despite these limitations, this study successfully developed a predictive model for MCE after EVT by combining radiomics features and clinical factors. This model has the potential to serve as a foundation for early and accurate clinical decision-making and for predicting the risk of MCE in patients with acute anterior circulation infarction following EVT.
Conclusions
We used both clinical characteristics and radiomics modeling of HIM post-EVT to create a nomogram that can predict MCE in patients with acute anterior circulation infarction who have received EVT. The nomogram may serve as a valuable tool for the accurate and timely prediction of MCE risk, assisting clinicians in making informed clinical decisions.
Acknowledgments
Some of our experiments were carried out on the Onekey AI platform. We would like to thank Onekey AI and its developers for helping in this scientific study.
Funding: This work was supported by
Footnote
Reporting Checklist: The authors have completed the TRIPOD reporting checklist. Available at https://qims.amegroups.com/article/view/10.21037/qims-24-99/rc
Conflicts of Interest: All authors have completed the ICMJE uniform disclosure form (available at https://qims.amegroups.com/article/view/10.21037/qims-24-99/coif). The authors have no conflicts of interest to declare.
Ethical Statement: The authors are accountable for all aspects of the work in ensuring that questions related to the accuracy or integrity of any part of the work are appropriately investigated and resolved. This study was conducted in accordance with the Declaration of Helsinki (as revised in 2013) and was approved by the Ethics Committee of the Fourth Affiliated Hospital of School of Medicine, and International School of Medicine, International Institutes of Medicine, Zhejiang University (approval No. Y2024029). The requirement for individual consent in this retrospective analysis was waived.
Open Access Statement: This is an Open Access article distributed in accordance with the Creative Commons Attribution-NonCommercial-NoDerivs 4.0 International License (CC BY-NC-ND 4.0), which permits the non-commercial replication and distribution of the article with the strict proviso that no changes or edits are made and the original work is properly cited (including links to both the formal publication through the relevant DOI and the license). See: https://creativecommons.org/licenses/by-nc-nd/4.0/.
References
- Shao Y, Chen X, Wang H, Shang Y, Xu J, Zhang J, Wang P, Geng Y. Large mismatch profile predicts rapidly progressing brain edema in acute anterior circulation large vessel occlusion patients undergoing endovascular thrombectomy. Front Neurol 2022;13:982911. [Crossref] [PubMed]
- Liu L, He CY, Yang JX, Zheng ST, Zhou J, Kong Y, Chen WB, Xie Y. Prediction models for post-thrombectomy brain edema in patients with acute ischemic stroke: a systematic review and meta-analysis. Front Neurol 2023;14:1254090. [Crossref] [PubMed]
- Kimberly WT, Dutra BG, Boers AMM, Alves HCBR, Berkhemer OA, van den Berg L, Sheth KN, Roos YBWEM, van der Lugt A, Beenen LFM, Dippel DWJ, van Zwam WH, van Oostenbrugge RJ, Lingsma HF, Marquering H, Majoie CBLM. MR CLEAN Investigators. Association of Reperfusion With Brain Edema in Patients With Acute Ischemic Stroke: A Secondary Analysis of the MR CLEAN Trial. JAMA Neurol 2018;75:453-61. [Crossref] [PubMed]
- Lin J, Frontera JA. Decompressive Hemicraniectomy for Large Hemispheric Strokes. Stroke 2021;52:1500-10. [Crossref] [PubMed]
- Jiang L, Zhang C, Wang S, Ai Z, Shen T, Zhang H, Duan S, Yin X, Chen YC. MRI Radiomics Features From Infarction and Cerebrospinal Fluid for Prediction of Cerebral Edema After Acute Ischemic Stroke. Front Aging Neurosci 2022;14:782036. [Crossref] [PubMed]
- Ng FC, Churilov L, Yassi N, Kleinig TJ, Thijs V, Wu TY, Shah D, Dewey HM, Sharma G, Desmond PM, Yan B, Parsons MW, Donnan GA, Davis SM, Mitchell PJ, Campbell BC. Association between pre-treatment perfusion profile and cerebral edema after reperfusion therapies in ischemic stroke. J Cereb Blood Flow Metab 2021;41:2887-96. [Crossref] [PubMed]
- Xu T, Wang Y, Yuan J, Chen Y, Luo H. Contrast extravasation and outcome of endovascular therapy in acute ischaemic stroke: a systematic review and meta-analysis. BMJ Open 2021;11:e044917. [Crossref] [PubMed]
- Portela de Oliveira E, Chakraborty S, Patel M, Finitsis S, Iancu D. Value of high-density sign on CT images after mechanical thrombectomy for large vessel occlusion in predicting hemorrhage and unfavorable outcome. Neuroradiol J 2021;34:120-7. [Crossref] [PubMed]
- Jiang Q, Hou J, Ge J, Huang Z, Wang H, Guo Z, Cao Y, You S, Xiao G. Clinical Significance of Hyperdense Area after Endovascular Therapy in Patients with Acute Ischemic Stroke: A Systematic Review and Meta-Analysis. Cerebrovasc Dis 2021;50:500-9. [Crossref] [PubMed]
- Wang C, Zhu Q, Cui T, Wang L, Yang T, Hao Z, Wu S, Zheng H, Hu F, Wu B. Early Prediction of Malignant Edema After Successful Recanalization in Patients with Acute Ischemic Stroke. Neurocrit Care 2022;36:822-30. [Crossref] [PubMed]
- Song SY, Ahn SY, Rhee JJ, Lee JW, Hur JW, Lee HK. Extent of Contrast Enhancement on Non-Enhanced Computed Tomography after Intra-Arterial Thrombectomy for Acute Infarction on Anterior Circulation: As a Predictive Value for Malignant Brain Edema. J Korean Neurosurg Soc 2015;58:321-7. [Crossref] [PubMed]
- Chen Q, Xia T, Zhang M, Xia N, Liu J, Yang Y. Radiomics in Stroke Neuroimaging: Techniques, Applications, and Challenges. Aging Dis 2021;12:143-54. [Crossref] [PubMed]
- Porto-Álvarez J, Martínez AM, Martínez Fernández J, Sanmartín López M, Blanco Ulla M, Vázquez Herrero F, Pumar JM, Rodríguez-Yáñez M, Minguillón Pereiro AM, Villaverde AB, Rey RI, Souto-Bayarri M. How Can Radiomics Help the Clinical Management of Patients with Acute Ischemic Stroke? Appl Sci 2023;13:10061.
- Powers WJ, Derdeyn CP, Biller J, Coffey CS, Hoh BL, Jauch EC, Johnston KC, Johnston SC, Khalessi AA, Kidwell CS, Meschia JF, Ovbiagele B, Yavagal DRAmerican Heart Association Stroke Council. 2015 American Heart Association/American Stroke Association Focused Update of the 2013 Guidelines for the Early Management of Patients With Acute Ischemic Stroke Regarding Endovascular Treatment: A Guideline for Healthcare Professionals From the American Heart Association/American Stroke Association. Stroke 2015;46:3020-35. [Crossref] [PubMed]
- Powers WJ, Rabinstein AA, Ackerson T, Adeoye OM, Bambakidis NC, Becker K, Biller J, Brown M, Demaerschalk BM, Hoh B, Jauch EC, Kidwell CS, Leslie-Mazwi TM, Ovbiagele B, Scott PA, Sheth KN, Southerland AM, Summers DV, Tirschwell DLAmerican Heart Association Stroke Council. 2018 Guidelines for the Early Management of Patients With Acute Ischemic Stroke: A Guideline for Healthcare Professionals From the American Heart Association/American Stroke Association. Stroke 2018;49:e46-e110. [Crossref] [PubMed]
- Frisullo G, Bellavia S, Scala I, Rizzo PA, Broccolini A, Brunetti V, Pepe M, Pilato F, Morosetti R, Marca GD, Calabresi P. Cerebral edema in acute stroke: Effect of thrombolytic treatment. J Neurol Sci 2022;436:120206. [Crossref] [PubMed]
- Guo W, Xu J, Zhao W, Zhang M, Ma J, Chen J, Duan J, Ma Q, Song H, Li S, Ji X. A nomogram for predicting malignant cerebral artery infarction in the modern thrombectomy era. Front Neurol 2022;13:934051. [Crossref] [PubMed]
- Cai J, Zhou Y, Zhao Y, Xu C, Yan S, Ding X, Lou M. Comparison of various reconstructions derived from dual-energy CT immediately after endovascular treatment of acute ischemic stroke in predicting hemorrhage. Eur Radiol 2021;31:4419-27. [Crossref] [PubMed]
- Byrne D, Walsh JP, Schmiedeskamp H, Settecase F, Heran MKS, Niu B, Salmeen AK, Rohr B, Field TS, Murray N, Rohr A. Prediction of Hemorrhage after Successful Recanalization in Patients with Acute Ischemic Stroke: Improved Risk Stratification Using Dual-Energy CT Parenchymal Iodine Concentration Ratio Relative to the Superior Sagittal Sinus. AJNR Am J Neuroradiol 2020;41:64-70. [Crossref] [PubMed]
- Wen X, Li Y, He X, Xu Y, Shu Z, Hu X, Chen J, Jiang H, Gong X. Prediction of Malignant Acute Middle Cerebral Artery Infarction via Computed Tomography Radiomics. Front Neurosci 2020;14:708. [Crossref] [PubMed]
- Peter R, Korfiatis P, Blezek D, Oscar Beitia A, Stepan-Buksakowska I, Horinek D, Flemming KD, Erickson BJ. A quantitative symmetry-based analysis of hyperacute ischemic stroke lesions in noncontrast computed tomography. Med Phys 2017;44:192-9. [Crossref] [PubMed]
- Yao X, Mao L, Lv S, Ren Z, Li W, Ren K. CT radiomics features as a diagnostic tool for classifying basal ganglia infarction onset time. J Neurol Sci 2020;412:116730. [Crossref] [PubMed]
- Wen X, Hu X, Xiao Y, Chen J. Radiomics analysis for predicting malignant cerebral edema in patients undergoing endovascular treatment for acute ischemic stroke. Diagn Interv Radiol 2023;29:402-9. [Crossref] [PubMed]
- Betrouni N, Yasmina M, Bombois S, Pétrault M, Dondaine T, Lachaud C, Laloux C, Mendyk AM, Henon H, Bordet R. Texture Features of Magnetic Resonance Images: an Early Marker of Post-stroke Cognitive Impairment. Transl Stroke Res 2020;11:643-52. [Crossref] [PubMed]
- Wang K, Gu L, Liu W, Xu C, Yin C, Liu H, Rong L, Li W, Wei X. The predictors of death within 1 year in acute ischemic stroke patients based on machine learning. Front Neurol 2023;14:1092534. [Crossref] [PubMed]
- Xie W, Ma X, Xu G, Wang Y, Huang W, Liu M, Sheng S, Yuan J, Wang J. Development and validation of a nomogram for the risk prediction of malignant cerebral edema after acute large hemispheric infarction involving the anterior circulation. Front Neurol 2023;14:1221879. [Crossref] [PubMed]
- Cappellari M, Turcato G, Forlivesi S, Zivelonghi C, Bovi P, Bonetti B, Toni D. STARTING-SICH Nomogram to Predict Symptomatic Intracerebral Hemorrhage After Intravenous Thrombolysis for Stroke. Stroke 2018;49:397-404. [Crossref] [PubMed]
- Cappellari M, Mangiafico S, Saia V, Pracucci G, Nappini S, Nencini P, et al. IER-SICH Nomogram to Predict Symptomatic Intracerebral Hemorrhage After Thrombectomy for Stroke. Stroke 2019;50:909-16. [Crossref] [PubMed]
- Zhang L, Wu J, Yu R, Xu R, Yang J, Fan Q, Wang D, Zhang W. Non-contrast CT radiomics and machine learning for outcomes prediction of patients with acute ischemic stroke receiving conventional treatment. Eur J Radiol 2023;165:110959. [Crossref] [PubMed]
- Bonnett LJ, Snell KIE, Collins GS, Riley RD. Guide to presenting clinical prediction models for use in clinical settings. BMJ 2019;365:l737. [Crossref] [PubMed]