Nomogram of magnetic resonance imaging (MRI) histogram analysis to predict telomerase reverse transcriptase promoter mutation status in glioblastoma
Introduction
The 2021 update of the fifth edition of the World Health Organization Classification of Tumors of the Central Nervous System (WHO CNS 5) (1) combines molecular typing with histological classification and simplifies the classification of adult diffuse gliomas. It highlights the importance of molecular typing in glioma classification, treatment, and prognosis. Glioblastoma (GBM), isocitrate dehydrogenase wildtype (IDH-wildtype) is the most common and aggressive primary malignant brain tumor in adults (2). The standard treatment is surgical resection, followed by radiotherapy and temozolomide chemotherapy (3). However, the 5-year recurrence rate after standardized treatment is as high as 90%. The median survival time is 15 months (4,5). The highly heterogeneous molecular expression of GBM leads to major differences in the treatment and prognosis of patients.
Telomerase reverse transcriptase promoter (pTERT) mutations play a crucial role in the two stages of tumorigenesis in patients with glioma: they promote cell immortalization and genomic instability. pTERT status is a strong biomarker for predicting the prognosis of glioma, and pTERT mutations account for approximately 70–80% of GBM (6-8). WHO CNS 5 incorporates IDH-wildtype diffuse astrocytic tumors with pTERT mutation or EGFR gene amplification or +7/−10 chromosome copy number changes as criteria for a diagnosis of WHO 4 grade GBM (1,9). pTERT mutation status is currently integrated into GBM diagnostic procedures. It also strongly predicts disease progression in patients with GBM (10). Therefore, preoperative prediction of pTERT mutation status in GBM is useful for diagnosis and treatment decisions.
Based on the imaging criteria for Visually AcceSIble Rembrandt Images, no valid magnetic resonance imaging (MRI) biomarkers have been found to predict the pTERT mutation status in GBM (11). MRI histogram analysis compiles each voxel within the region of interest (ROI) into a histogram that can better elucidate tumor heterogeneity. Compared with the traditional ROI analysis method, it is more comprehensive, objective, and repeatable (12,13). MRI histogram analysis has been used to analyze glioma heterogeneity (14-17). However, the relationship between histogram parameters and pTERT mutation status in patients with GBM is currently unclear, and there are few relevant studies. This study aimed to investigate the predictive value of preoperative MRI histogram analysis for evaluating pTERT mutation status in GBM as well as establishing a logistic regression model nomogram, so as to provide objective guidance basis for individual treatment of patients. We present this article in accordance with the TRIPOD reporting checklist (available at https://qims.amegroups.com/article/view/10.21037/qims-24-71/rc).
Methods
This study was performed in line with the principles of the Declaration of Helsinki (as revised in 2013) and approved by the Clinical Ethics Committee of Lanzhou University Second Hospital (No. 2020A-070). Individual consent for this retrospective analysis was waived.
Patients
According to the classification of 2021 WHO CNS 5, the clinical and imaging data for IDH-wildtype GBM patients in Lanzhou University Second Hospital between November 2018 and April 2023 were retrospectively collected. Inclusion criteria included: (I) no biopsy or chemoradiotherapy was performed before MRI scan; (II) GBM was confirmed by histopathology and immunohistochemistry; (III) IDH-wildtype was confirmed by molecular detection. Exclusion criteria included: (I) The molecular states of IDH and telomerase reverse transcriptase (TERT) were not detected; (II) the MRI sequence was incomplete or the image quality was poor 1 week before surgery; (III) age <18 years. Finally, 181 patients were recruited in this study. The age ranged from 19 to 79 years, with an average age of 53.38±11.68 years, including 91 males and 90 females. The inclusion and exclusion processes are shown in Figure 1.
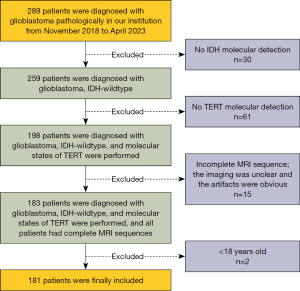
Image acquisition
Patients underwent a head MR plain scan and enhanced scan using Siemens Verio 3.0 T MR scanner (Siemens AG, Henkestrasse 127, D-91052, Erlangen, Germany) in a supine position. The scanning parameters for T1-weighted imaging (T1WI) (gradient echo sequence) were as follows: repetition time (TR), 550 ms; echo time (TE), 11 ms; layer thickness, 5 mm; layer spacing, 1.5 mm; field of view (FOV), 260 mm × 260 mm; and matrix 256×256; and T2-weighted imaging (T2WI) (turbo spin-echo sequence): TR, 2,200 ms; TE, 96 ms; layer thickness, 5 mm; layer spacing, 1.5 mm; FOV 260 mm × 260 mm; and matrix 256×256. T1W1 enhanced scan was performed in the axial, sagittal, and coronal views. The Gd-DTPA (Bayer Schering Pharma AG, Berlin, Germany) (/kg) was used as the contrast agent. Intravenous bolus injection rate was 0.1 mmol/kg and flow rate was 3.0 mL/s; the corresponding scanning parameters were the same of those of a plain scan.
Molecular analysis
Genomic deoxyribonucleic acid (DNA) was extracted from the sections containing many tumor cells that were scraped from paraffin-embedded tissue slides. DNA concentration and purity were measured by a ND8000 spectrophotometer (NanoDrop Technologies, Wilmington, DE, USA). The molecular states IDH and TERT were detected by direct sequencing. Sequences covering the mutational hotspots in the TERT core promoter [nucleotide numbers 1,295,228 (C228T) and 1,295,250 (C250T) from the human reference sequence (GRCh37 February 2009; http://genome.ucsc.edu/), were amplified by nested Polymerase Chain Reaction. Accordingly, we used molecular sequencing results to classify the datasets into pTERT mutation (C228T and C250T) and pTERT-wildtype groups.
Image analysis
All patients were independently analyzed in a blinded fashion by two experienced neuroradiologists (X.K. and Q.Z. with 10 and 5 years of brain MRI experience, respectively). Tumor maximal diameter, peritumoral edema maximal length, percentage of peritumoral edema, across the midline, multifocal lesion, margin (clear/fuzzy) and percentage of unenhanced tumor area were recorded.
Two neuroradiologists imported images in the Dicom format into the freely available software package FireVoxel (current version: 416C, NYU School of Medicine, NY, https://firevoxel.org/). Then select the largest layer of the tumor. Based on the T1WI and T2WI images, two neuroradiologists manually mapped all 181 GBM of axial T1-weighted contrast-enhanced (T1C) tumor areas as the regions of interest (ROI), excluding peritumoral oedema. Considering the effect of partial volume effects, the ROI outlined area was slightly smaller than the visible tumor boundary. The ROI encompassed all tumor information at the largest lesion slice, including necrosis and cystic differentiation. Then the software automatically generated a grayscale histogram of the ROI. Examples of the histogram ROI and parameters were shown in Figures 2-4, the ROI was filled with red. Histogram analysis was performed using the largest slices to obtain the following histogram parameters: maximum, minimum, mean, standard deviation (SD), variance, coefficient of variation (CV), skewness, kurtosis, entropy, and 1st–99th percentiles.
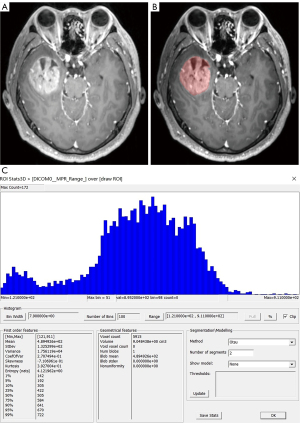
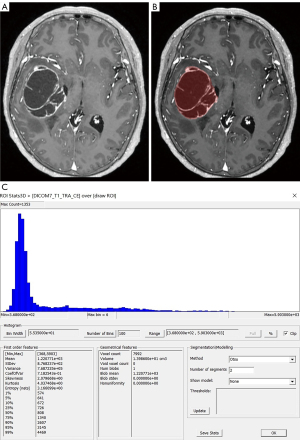
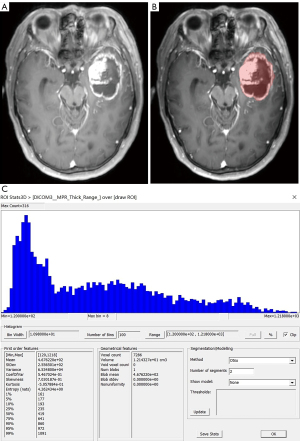
Statistical analysis
Statistical analysis was performed in SPSS 25.0 (IBM Corporation, Armonk, NY, USA) and R Software (version 4.2.3; http://www.rproject.org). The concordance of histogram parameters measured by two radiologists was examined by intra-class correlation coefficient (ICC) analysis. An ICC value >0.75 indicated good agreement. The χ2 test was used to analyze categorical variables. The Kolmogorov-Smirnov test was used to check whether the variables conformed to normal distribution. Two independent sample t-test was used for comparison between groups in case of normal data distribution; otherwise, the Mann-Whitney U test was used. A P value <0.05 was considered statistically significant. The predictive models for pTERT mutation status were constructed using logistic regression, and a nomogram was generated. Calibration and decision curves were constructed to evaluate the consistency and clinical availability of the predictive model in relation to the actual probability. A receiver operating characteristic (ROC) analysis was used to evaluate the differential diagnosis ability of nomogram in the area under the curve (AUC).
Results
Patient grouping
pTERT mutations were observed in 122/181 (67.4 %) of all GBM analysed. Of these, 98 cases (80.3%) had a C228T mutation, and 24 cases (19.7%) had a C250T mutation.
The average age of pTERT mutation group was 57±9 years, and the average age of pTERT-wildtype group was 46±14 years. It indicated that the age were significantly older in pTERT mutation group than in pTERT-wildtype group (P<0.001). The average percentage of unenhanced tumor area of pTERT mutation group was 0.37±0.18, and the average percentage of unenhanced tumor area of pTERT-wildtype group was 0.19±0.19. It indicated that the percentage of unenhanced tumor area were significantly greater in pTERT mutation group than in pTERT-wildtype group (P<0.001). The clinicopathologic characteristics and conventional MRI features of patients in different subtypes are shown in Table 1.
Table 1
Parameter | pTERT mutation (n=122) | pTERT-wildtype (n=59) | P value |
---|---|---|---|
Age (years) | 56 [51, 63] | 49 [33, 55] | <0.001* |
Gender | 0.169 | ||
Male | 57 | 34 | |
Female | 65 | 25 | |
Maximal diameter (mm)a | 46.07±14.21 | 45.05±14.28 | 0.653 |
Peritumoral edema maximal length (mm) | 20.96 [14.88, 29.35] | 18.48 [11.34, 31.00] | 0.607 |
Percentage of peritumoral edema (%) | 44.83 [28.43, 72.82] | 37.14 [22.03, 67.07] | 0.960 |
Across the midline | 0.223 | ||
Yes | 18 | 13 | |
No | 104 | 46 | |
Multifocal lesion | 0.386 | ||
Yes | 32 | 12 | |
No | 90 | 47 | |
Margin | 0.211 | ||
Clear | 48 | 29 | |
Fuzzy | 74 | 30 | |
Percentage of unenhanced tumor area (%) | 36.63 [23.97, 51.28] | 12.83 [5.13, 24.66] | <0.001* |
Ki67 (%) | 40.00 [30.00, 51.25] | 50.00 [30.00, 60.00] | 0.446 |
p53 | 0.173 | ||
Wildtype | 53 | 32 | |
Mutation | 69 | 27 |
a, data conformed to normal distribution, data were presented as mean ± standard deviation, other data were presented as median [interquartile range] or number. *, indicates P<0.05. pTERT, telomerase reverse transcriptase promoter; MRI, magnetic resonance imaging.
Histogram analysis for differentiating pTERT status in GBM
In this study, ICCs for T1C histogram parameters calculated by the two observers ranged from 0.84 to 0.92.
Typical cases are shown in Figures 2-4. Table 2 shows the results of T1C histogram parameter analysis in both groups. There were significant between-group differences in the maximum, SD, variance, CV, skewness, 5th, 10th, 25th, 95th and 99th percentiles (P=0.000–0.040); however, there were no significant between-group differences in the minimum, mean, kurtosis, entropy, as well as the 1st, 50th, 75th and 90th (P=0.115–0.856).
Table 2
Parameter | pTERT mutation (n=122) | pTERT-wildtype (n=59) | P value |
---|---|---|---|
Maximum | 955.00 (792.00, 1,160.50) | 830.00 (602.00, 1,008.00) | 0.005* |
Minimum | 137.50 (78.75, 172.50) | 146.00 (93.00, 179.00) | 0.470 |
Mean | 433.71 (320.66, 514.92) | 438.89 (355.49, 512.47) | 0.832 |
SD | 166.37 (125.42, 202.13) | 127.29 (91.30, 159.85) | <0.001* |
Variance | 2.77 (1.57, 4.08) | 1.62 (0.83, 2.55) | <0.001* |
CVa | 4.21±1.35 | 2.97±1.14 | <0.001* |
Skewnessa | 4.66±6.14 | 2.23±5.87 | 0.014* |
Kurtosis | −3.70 (−7.83, 2.43) | −1.69 (−6.30, 3.60) | 0.150 |
Entropy | 4.21 (4.02, 4.30) | 4.21 (4.06, 4.31) | 0.856 |
Perc.01 | 169.00 (115.75, 213.25) | 195.00 (140.00, 254.00) | 0.132 |
Perc.05 | 195.50 (141.50, 254.25) | 239.00 (172.00, 304.00) | 0.021* |
Perc.10 | 217.00 (154.50, 292.25) | 273.00 (191.00, 348.00) | 0.019* |
Perc.25 | 272.00 (206.75, 384.75) | 357.00 (252.00, 413.00) | 0.040* |
Perc.50 | 414.00 (282.50, 517.25) | 451.00 (305.00, 508.00) | 0.610 |
Perc.75 | 530.50 (425.00, 665.50) | 539.00 (447.00, 620.00) | 0.447 |
Perc.90 | 630.00 (512.00, 783.75) | 609.00 (482.00, 702.00) | 0.115 |
Perc.95 | 697.50 (571.50, 850.50) | 654.00 (521.00, 762.00) | 0.037* |
Perc.99 | 847.00 (686.25, 964.50) | 738.00 (565.00, 860.00) | 0.005* |
a, data conformed to normal distribution, data were presented as mean ± standard deviation, other data were presented as median (interquartile range). *, indicates P<0.05. T1C, T1-weighted contrast-enhanced; pTERT, telomerase reverse transcriptase promoter; SD, standard deviation; CV, coefficient of variation.
Multivariate logistic regression and nomogram
Multivariate logistic regression analysis of age, percentage of unenhanced tumor area and T1C histogram parameters showed that age, percentage of unenhanced tumor area, SD and CV were independent risk factors for predicting pTERT mutation status in GBM patients (Table 3). Based on these results, we generated an individualized nomogram to predict pTERT mutation status in patients with GBM using four independent risk factors (Figure 5). The logistic regression model based on four features, age, percentage of unenhanced tumor area, SD and CV, showed a better sample predictive performance. The calibration curve exhibited favorable agreement between the model’s predicted probability and actual probability (Figure 6). The decision curve revealed that the prediction model exhibited favorable clinical availability (Figure 7). The AUC, accuracy, sensitivity, specificity were 0.842, 0.796, 0.820, and 0.729, respectively (Table 4, Figure 8).
Table 3
Parameter | z value | P value | OR (95% CI) |
---|---|---|---|
Age | 3.723 | <0.001 | 3.000 (1.784–5.045) |
Percentage of unenhanced tumor area | 2.973 | 0.003 | 1.764 (1.428–2.180) |
Maximum | 0.492 | 0.623 | 1.001 (0.996–1.007) |
SD | −1.693 | 0.002 | 1.109 (1.039–1.183) |
Variance | 0.820 | 0.412 | 1.025 (1.003–1.047) |
CV | 1.607 | 0.012 | 2.319 (1.680–3.202) |
Skewness | −1.225 | 0.167 | 0.772 (0.709–1.287) |
Perc.05 | −1.176 | 0.239 | 0.984 (0.957–1.011) |
Perc.10 | −0.074 | 0.941 | 0.999 (0.966–1.033) |
Perc.25 | −0.770 | 0.442 | 0.991 (0.969–1.014) |
Perc.95 | 0.707 | 0.480 | 1.013 (0.978–1.048) |
Perc.99 | 1.484 | 0.138 | 1.014 (0.996–1.032) |
pTERT, telomerase reverse transcriptase promoter; OR, odds ratio; CI, confidence interval; SD, standard deviation; CV, coefficient of variation.
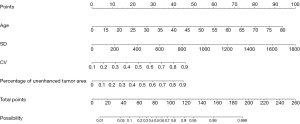
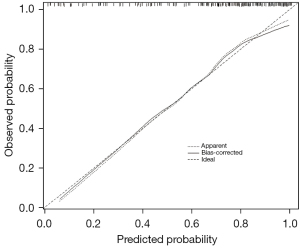
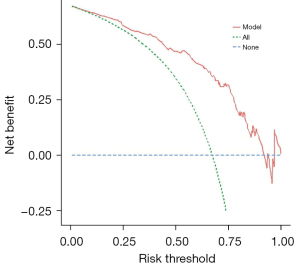
Table 4
Risk factors | AUC (95% CI) | Accuracy | Sensitivity | Specificity |
---|---|---|---|---|
Age | 0.734 (0.651–0.817) | 0.696 | 0.697 | 0.695 |
Percentage of unenhanced tumor area | 0.780 (0.702–0.858) | 0.773 | 0.803 | 0.712 |
SD | 0.706 (0.629–0.784) | 0.613 | 0.508 | 0.831 |
CV | 0.775 (0.702–0.848) | 0.779 | 0.803 | 0.729 |
Nomogram | 0.842 (0.767–0.917) | 0.796 | 0.820 | 0.729 |
pTERT, telomerase reverse transcriptase promoter; AUC, area under the curve; CI, confidence interval; SD, standard deviation; CV, coefficient of variation.
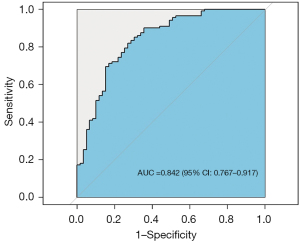
Histogram analysis for differentiating C228T and C250T
There were no statistically significant differences in T1C histogram parameters between C228T and C250T groups (P=0.055–0.854) (Table 5).
Table 5
Parameter | C228T (n=98) | C250T (n=24) | P value |
---|---|---|---|
Maximum | 944.50 (794.25, 1,160.50) | 993.00 (762.50, 1,189.25) | 0.854 |
Minimum | 137.50 (77.50, 170.25) | 131.00 (104.00, 203.25) | 0.473 |
Mean | 425.34 (318.26, 511.58) | 449.89 (334.35, 598.24) | 0.374 |
SD | 161.62 (125.42, 200.69) | 169.25 (123.41, 207.95) | 0.553 |
Variance | 2.61 (1.57, 4.03) | 2.86 (1.52, 4.32) | 0.553 |
CV | 4.08 (3.37, 5.17) | 4.03 (3.47, 5.14) | 0.802 |
Skewness | 5.16 (0.43, 9.30) | 2.73 (0.50, 7.21) | 0.310 |
Kurtosis | −3.10 (−7.40, 3.58) | −7.50 (−9.35, 0.45) | 0.055 |
Entropy | 4.20 (4.00, 4.30) | 4.27 (4.15, 4.32) | 0.162 |
Perc.01 | 169.00 (114.75, 206.75) | 172.00 (132.50, 262.25) | 0.465 |
Perc.05 | 193.00 (139.50, 247.00) | 199.00 (146.00, 306.75) | 0.577 |
Perc.10 | 213.50 (154.50, 289.75) | 226.50 (154.25, 330.75) | 0.652 |
Perc.25 | 267.50 (206.75, 381.75) | 327.00 (211.50, 402.00) | 0.369 |
Perc.50 | 410.50 (263.75, 516.25) | 457.00 (337.25, 588.25) | 0.216 |
Perc.75 | 530.50 (424.25, 649.25) | 548.00 (434.25, 755.75) | 0.434 |
Perc.90 | 625.00 (511.50, 780.00) | 655.50 (542.25, 854.75) | 0.606 |
Perc.95 | 687.00 (567.00, 847.00) | 710.00 (593.00, 922.25) | 0.591 |
Perc.99 | 847.00 (690.50, 964.50) | 827.50 (670.50, 1038.00) | 0.730 |
Data are presented as median (interquartile range). T1C, T1-weighted contrast-enhanced; SD, standard deviation; CV, coefficient of variation.
Discussion
This study showed that the model based on these four features: age, percentage of unenhanced tumor area, and T1C histogram parameters, including the SD and CV, have good predictive performance for pTERT mutation status in patients with GBM. A logistic regression model nomogram based on conventional MRI features and T1C histogram parameters may be a useful tool for non-invasive identification of the pTERT status in patients with GBM.
In human cells, telomerase repression or shorter telomeres prevent uncontrolled cellular proliferation (18). The repression of telomerase is mainly achieved by transcriptional inhibition of its catalytic component, TERT gene. pTERT mutations may contribute to multi-cancer hallmarks via telomere lengthening (8). The Consortium to Inform Molecular and Practical Approaches to CNS Tumor Taxonomy (cIMPACT-NOW) Update 3 recommended that there is no difference in overall survival between GBM and WHO grade 2 and 3 IDH-wildtype diffuse astrocytic glioma containing a pTERT mutation. Lower grade gliomas with pTERT mutations have the molecular features of WHO 4-grade tumors (19-21). This is also the premise behind telomerase-targeted therapies effectively treating this fatal malignancy (22). Therefore, GBM with pTERT mutations has an influence on classification and prognosis.
Among the patients in our study, about 70% had pTERT mutations, basically consistent with the overall incidence of pTERT mutations. Meanwhile, the C228T mutation was the most prevalent cancer-associated pTERT variant in our study, consistent with reported findings (23,24). In addition, previous studies have shown that the frequency of pTERT mutations increases with age in IDH-wildtype diffuse gliomas, and glioma prognosis is related to age at diagnosis (7,25-27). Therefore, age could have some influence on TERT mutation frequency. Our results showed that patients with pTERT mutations were significantly older than those with pTERT-wildtype. Our results are consistent with those reported in the literature and indicate that our data have strong clinical applicability.
Cells with the pTERT mutation have longer telomeres, which inhibit replicative aging caused by telomere erosion in normal cells. These cells have the potential to become immortal and continuously proliferate (28). The growth mode of pTERT mutation tumors is mainly reflected in their malignant proliferative potential. It is often accompanied by necrosis, high cell density, hemorrhage, angiogenesis, and other tumor heterogeneity characteristics (29). Histogram features can reflect tumor heterogeneity by the distribution of gray-level frequency in a given ROI (30). It can provide multiple histogram feature parameters for quantitative analysis and is related to the malignant degree of tumor and the survival of patients (31,32). Compared with traditional ROI methods, analysis of the pTERT mutation status of GBM using histogram feature parameters can reflect the internal heterogeneity of the tumor more comprehensively, and the reproducibility is strong. In addition, T1C is a routine MRI sequence that is widely used for the diagnosis of brain tumors. The T1C sequence can clarify the contour of the lesion and provide more information regarding the internal heterogeneity of the tumor following enhanced contrast agent injection (33). Xue et al. (14) thoroughly evaluated the level of tumor-infiltrating CD8+ T cells in GBM using T1C histogram analysis and concluded that the parameters of the T1C histogram could accurately reflect tumor heterogeneity in GBM. In our study, the maximum level of the tumor was used as the delineated area of ROI, which not only contained as much comprehensive tumor information as possible, but was more convenient and easier to apply in daily clinical work than 3D whole tumor histogram analysis. Therefore, it is feasible to analyze the pTERT mutation status of GBM by using the T1C histogram parameters.
In our study, the histogram feature parameters SD and CV better distinguished the pTERT mutation group from the pTERT-wildtype group. Research found that the irregularity of contrast enhancing areas could predict survival in GBM patients (34). Therefore, we compared the percentage of unenhanced tumor area between the pTERT mutation group and the wildtype group on T1C images. We found that the proportion of unenhanced tumor area at the largest tumor level in the mutation group was significantly higher than that in the wildtype group. The unenhanced area of the tumor was usually necrosis/cystic changes, indirectly reflecting that the tumor area of pTERT mutation group was more prone to necrosis/cystic changes, this conclusion is consistent with that of Yamashita et al. (26). The necrosis/cystic changes in the GBM on T1C usually manifest as an unenhanced area, and its gray level is significantly different from that of the surrounding enhanced area. In addition, the histogram feature can reflect the gray level information of each pixel, and the SD and CV in the histogram feature parameter usually represents the fluctuation size of the histogram. The greater the SD and CV, the more mixed and uneven the MRI signal (35,36). Previous studies have also found that SD should be evaluated as a potential biomarker of GBM aggressiveness (37). This may explain why the SD and CV in the pTERT mutation group was significantly higher than that in the wildtype group. Moreover, necrosis might be more common in patients with pTERT mutations, possibly related to the fact that the pTERT mutation promotes the malignant proliferation of cells more vigorously, resulting in insufficient nutrient supply and cell necrosis at the center of the lesion.
The present study was first limited by its retrospective and single-center design. Further data will be collected in the future to validate the nomogram, thereby increasing the generalizability of our study results. Second, the data for the pTERT-wildtype and C250T mutations were smaller than those for the C228T mutation; this may have caused data bias. However, this dataset is consistent with the incidence of GBM pTERT mutations and their subtypes and is more in line with real-world scenarios. Third, we will attempt to use multimodal MRI indicators, including diffusion tensor imaging, diffusion kurtosis imaging, and perfusion-weighted imaging, in the future.
Conclusions
In conclusion, the present study confirmed the predictive value of preoperative T1C histogram analysis for evaluating pTERT mutations status in GBM. A nomogram based on conventional MRI features and T1C histogram parameters is a reliable tool for the pTERT mutation status, allowing for non-invasive radiological prediction before surgery.
Acknowledgments
Funding: This work was supported by
Footnote
Reporting Checklist: The authors have completed the TRIPOD reporting checklist. Available at https://qims.amegroups.com/article/view/10.21037/qims-24-71/rc
Conflicts of Interest: All authors have completed the ICMJE uniform disclosure form (available at https://qims.amegroups.com/article/view/10.21037/qims-24-71/coif). All authors report that this work was supported by the National Natural Science Foundation of China (Nos. 82071872 and 82371914), the Science and Technology Program of Gansu Province (Nos. 21YF5FA123 and 21JR11RA105), and the China International Medical Foundation (No. Z-2014-07-2101). The authors have no other conflicts of interest to declare.
Ethical Statement: The authors are accountable for all aspects of the work in ensuring that questions related to the accuracy or integrity of any part of the work are appropriately investigated and resolved. This study was performed in line with the principles of the Declaration of Helsinki (as revised in 2013) and approved by the Clinical Ethics Committee of Lanzhou University Second Hospital (No. 2020A-070). Individual consent for this retrospective analysis was waived.
Open Access Statement: This is an Open Access article distributed in accordance with the Creative Commons Attribution-NonCommercial-NoDerivs 4.0 International License (CC BY-NC-ND 4.0), which permits the non-commercial replication and distribution of the article with the strict proviso that no changes or edits are made and the original work is properly cited (including links to both the formal publication through the relevant DOI and the license). See: https://creativecommons.org/licenses/by-nc-nd/4.0/.
References
- Louis DN, Perry A, Wesseling P, Brat DJ, Cree IA, Figarella-Branger D, Hawkins C, Ng HK, Pfister SM, Reifenberger G, Soffietti R, von Deimling A, Ellison DW. The 2021 WHO Classification of Tumors of the Central Nervous System: a summary. Neuro Oncol 2021;23:1231-51. [Crossref] [PubMed]
- Bosio A, Cerretti G, Padovan M, Caccese M, Denaro L, Chioffi F, Della Puppa A, Aldegheri V, Guarneri V, Zagonel V, Lombardi G. Metronomic Temozolomide in Heavily Pretreated Patients With Recurrent Isocitrate Dehydrogenase Wild-type Glioblastoma: A Large Real-Life Mono-Institutional Study. Clin Oncol (R Coll Radiol) 2023;35:e319-27. [Crossref] [PubMed]
- Stupp R, Mason WP, van den Bent MJ, Weller M, Fisher B, Taphoorn MJ, et al. Radiotherapy plus concomitant and adjuvant temozolomide for glioblastoma. N Engl J Med 2005;352:987-96. [Crossref] [PubMed]
- Ostrom QT, Gittleman H, Fulop J, Liu M, Blanda R, Kromer C, Wolinsky Y, Kruchko C, Barnholtz-Sloan JS. CBTRUS Statistical Report: Primary Brain and Central Nervous System Tumors Diagnosed in the United States in 2008-2012. Neuro Oncol 2015;17:iv1-iv62. [Crossref] [PubMed]
- Uetani H, Azuma M, Khant ZA, Watanabe Y, Kudo K, Kadota Y, Yokogami K, Takeshima H, Kuroda JI, Shinojima N, Hamasaki T, Mukasa A, Hirai T. Importance of Age and Noncontrast-Enhancing Tumor as Biomarkers for Isocitrate Dehydrogenase-Mutant Glioblastoma: A Multicenter Study. J Comput Assist Tomogr 2023;47:659-65. [Crossref] [PubMed]
- Killela PJ, Reitman ZJ, Jiao Y, Bettegowda C, Agrawal N, Diaz LA Jr, et al. TERT promoter mutations occur frequently in gliomas and a subset of tumors derived from cells with low rates of self-renewal. Proc Natl Acad Sci U S A 2013;110:6021-6. [Crossref] [PubMed]
- Vinagre J, Almeida A, Pópulo H, Batista R, Lyra J, Pinto V, et al. Frequency of TERT promoter mutations in human cancers. Nat Commun 2013;4:2185. [Crossref] [PubMed]
- Yuan X, Larsson C, Xu D. Mechanisms underlying the activation of TERT transcription and telomerase activity in human cancer: old actors and new players. Oncogene 2019;38:6172-83. [Crossref] [PubMed]
- Stichel D, Ebrahimi A, Reuss D, Schrimpf D, Ono T, Shirahata M, Reifenberger G, Weller M, Hänggi D, Wick W, Herold-Mende C, Westphal M, Brandner S, Pfister SM, Capper D, Sahm F, von Deimling A. Distribution of EGFR amplification, combined chromosome 7 gain and chromosome 10 loss, and TERT promoter mutation in brain tumors and their potential for the reclassification of IDHwt astrocytoma to glioblastoma. Acta Neuropathol 2018;136:793-803. [Crossref] [PubMed]
- Spiegl-Kreinecker S, Lötsch D, Ghanim B, Pirker C, Mohr T, Laaber M, Weis S, Olschowski A, Webersinke G, Pichler J, Berger W. Prognostic quality of activating TERT promoter mutations in glioblastoma: interaction with the rs2853669 polymorphism and patient age at diagnosis. Neuro Oncol 2015;17:1231-40. [Crossref] [PubMed]
- Ersoy TF, Keil VC, Hadizadeh DR, Gielen GH, Fimmers R, Waha A, Heidenreich B, Kumar R, Schild HH, Simon M. New prognostic factor telomerase reverse transcriptase promotor mutation presents without MR imaging biomarkers in primary glioblastoma. Neuroradiology 2017;59:1223-31. [Crossref] [PubMed]
- Zhu Q, Zou J, Ye J, Zhu W, Wu J, Chen W. Comparative study of conventional ROI-based and volumetric histogram analysis derived from CT enhancement in differentiating malignant and benign renal tumors. Br J Radiol 2022;95:20210801. [Crossref] [PubMed]
- Yang F, Li X, Li Y, Lei H, Du Q, Yu X, Li L, Zhao Y, Xie L, Lin M. Histogram analysis of quantitative parameters from synthetic MRI: correlations with prognostic factors in nasopharyngeal carcinoma. Eur Radiol 2023;33:5344-54. [Crossref] [PubMed]
- Xue C, Zhou Q, Zhang P, Zhang B, Sun Q, Li S, Deng J, Liu X, Zhou J. MRI histogram analysis of tumor-infiltrating CD8+ T cell levels in patients with glioblastoma. Neuroimage Clin 2023;37:103353. [Crossref] [PubMed]
- Gao A, Zhang H, Yan X, Wang S, Chen Q, Gao E, Qi J, Bai J, Zhang Y, Cheng J. Whole-Tumor Histogram Analysis of Multiple Diffusion Metrics for Glioma Genotyping. Radiology 2022;302:652-61. [Crossref] [PubMed]
- Sun Y, Yang Z, Deng K, Geng Y, Hu X, Song Y, Jiang R. Histogram analysis of quantitative susceptibility mapping and apparent diffusion coefficient for identifying isocitrate dehydrogenase genotypes and tumor subtypes of adult-type diffuse gliomas. Quant Imaging Med Surg 2023;13:8681-93. [Crossref] [PubMed]
- Ge X, Gan T, Yang Z, Liu G, Hu W, Ma W, Bai Y, Xiong Y, Li M, Zhao J, Zhou L, Li J, Li D, Wang X, Zhang J. Whole-tumor histogram analysis of synthetic magnetic resonance imaging predicts isocitrate dehydrogenase mutation status in gliomas. Quant Imaging Med Surg 2024;14:2225-39. [Crossref] [PubMed]
- Seluanov A, Gladyshev VN, Vijg J, Gorbunova V. Mechanisms of cancer resistance in long-lived mammals. Nat Rev Cancer 2018;18:433-41. [Crossref] [PubMed]
- Arita H, Yamasaki K, Matsushita Y, Nakamura T, Shimokawa A, Takami H, et al. A combination of TERT promoter mutation and MGMT methylation status predicts clinically relevant subgroups of newly diagnosed glioblastomas. Acta Neuropathol Commun 2016;4:79. [Crossref] [PubMed]
- Brat DJ, Aldape K, Colman H, Holland EC, Louis DN, Jenkins RB, Kleinschmidt-DeMasters BK, Perry A, Reifenberger G, Stupp R, von Deimling A, Weller M. cIMPACT-NOW update 3: recommended diagnostic criteria for "Diffuse astrocytic glioma, IDH-wildtype, with molecular features of glioblastoma, WHO grade IV". Acta Neuropathol 2018;136:805-10. [Crossref] [PubMed]
- Ramos-Fresnedo A, Pullen MW, Perez-Vega C, Domingo RA, Akinduro OO, Almeida JP, Suarez-Meade P, Marenco-Hillembrand L, Jentoft ME, Bendok BR, Trifiletti DM, Chaichana KL, Porter AB, Quiñones-Hinojosa A, Burns TC, Kizilbash SH, Middlebrooks EH, Sherman WJ. The survival outcomes of molecular glioblastoma IDH-wildtype: a multicenter study. J Neurooncol 2022;157:177-85. [Crossref] [PubMed]
- Aquilanti E, Kageler L, Watson J, Baird DM, Jones RE, Hodges M, Szegletes ZM, Doench JG, Strathdee CA, Figueroa JRMF, Ligon KL, Beck M, Wen PY, Meyerson M. Telomerase inhibition is an effective therapeutic strategy in TERT promoter-mutant glioblastoma models with low tumor volume. Neuro Oncol 2023;25:1275-85. [Crossref] [PubMed]
- Johanns TM, Fu Y, Kobayashi DK, Mei Y, Dunn IF, Mao DD, Kim AH, Dunn GP. High incidence of TERT mutation in brain tumor cell lines. Brain Tumor Pathol 2016;33:222-7. [Crossref] [PubMed]
- Palsgrove DN, Taheri D, Springer SU, Cowan M, Guner G, Mendoza Rodriguez MA, Rodriguez Pena MDC, Wang Y, Kinde I, Ricardo BFP, Cunha I, Fujita K, Ertoy D, Kinzler KW, Bivalacqua TJ, Papadopoulos N, Vogelstein B, Netto GJ. Targeted sequencing of plasmacytoid urothelial carcinoma reveals frequent TERT promoter mutations. Hum Pathol 2019;85:1-9. [Crossref] [PubMed]
- Fujimoto K, Arita H, Satomi K, Yamasaki K, Matsushita Y, Nakamura T, et al. TERT promoter mutation status is necessary and sufficient to diagnose IDH-wildtype diffuse astrocytic glioma with molecular features of glioblastoma. Acta Neuropathol 2021;142:323-38. [Crossref] [PubMed]
- Yamashita K, Hatae R, Hiwatashi A, Togao O, Kikuchi K, Momosaka D, Yamashita Y, Kuga D, Hata N, Yoshimoto K, Suzuki SO, Iwaki T, Iihara K, Honda H. Predicting TERT promoter mutation using MR images in patients with wild-type IDH1 glioblastoma. Diagn Interv Imaging 2019;100:411-9. [Crossref] [PubMed]
- Akyerli CB, Yüksel Ş, Can Ö, Erson-Omay EZ, Oktay Y, Coşgun E, Ülgen E, Erdemgil Y, Sav A, von Deimling A, Günel M, Yakıcıer MC, Pamir MN, Özduman K. Use of telomerase promoter mutations to mark specific molecular subsets with reciprocal clinical behavior in IDH mutant and IDH wild-type diffuse gliomas. J Neurosurg 2018;128:1102-14. [Crossref] [PubMed]
- Chiba K, Johnson JZ, Vogan JM, Wagner T, Boyle JM, Hockemeyer D. Cancer-associated TERT promoter mutations abrogate telomerase silencing. Elife 2015;4:e07918. [Crossref] [PubMed]
- Nelson DA, Tan TT, Rabson AB, Anderson D, Degenhardt K, White E. Hypoxia and defective apoptosis drive genomic instability and tumorigenesis. Genes Dev 2004;18:2095-107. [Crossref] [PubMed]
- Lohmann P, Galldiks N, Kocher M, Heinzel A, Filss CP, Stegmayr C, Mottaghy FM, Fink GR, Jon Shah N, Langen KJ. Radiomics in neuro-oncology: Basics, workflow, and applications. Methods 2021;188:112-21. [Crossref] [PubMed]
- O'Connor JP, Rose CJ, Waterton JC, Carano RA, Parker GJ, Jackson A. Imaging intratumor heterogeneity: role in therapy response, resistance, and clinical outcome. Clin Cancer Res 2015;21:249-57. [Crossref] [PubMed]
- Li C, Wang S, Serra A, Torheim T, Yan JL, Boonzaier NR, Huang Y, Matys T, McLean MA, Markowetz F, Price SJ. Multi-parametric and multi-regional histogram analysis of MRI: modality integration reveals imaging phenotypes of glioblastoma. Eur Radiol 2019;29:4718-29. [Crossref] [PubMed]
- Di Ieva A, Russo C, Liu S, Jian A, Bai MY, Qian Y, Magnussen JS. Application of deep learning for automatic segmentation of brain tumors on magnetic resonance imaging: a heuristic approach in the clinical scenario. Neuroradiology 2021;63:1253-62. [Crossref] [PubMed]
- Pérez-Beteta J, Martínez-González A, Molina D, Amo-Salas M, Luque B, Arregui E, et al. Glioblastoma: does the pre-treatment geometry matter? A postcontrast T1 MRI-based study. Eur Radiol 2017;27:1096-104. [Crossref] [PubMed]
- Just N. Improving tumour heterogeneity MRI assessment with histograms. Br J Cancer 2014;111:2205-13. [Crossref] [PubMed]
- Liang HY, Huang YQ, Yang ZX. Ying-Ding, Zeng MS, Rao SX. Potential of MR histogram analyses for prediction of response to chemotherapy in patients with colorectal hepatic metastases. Eur Radiol 2016;26:2009-18. [Crossref] [PubMed]
- Sarkar S, Rojas R, Lespinasse E, Zhang XF, Zeron R. Standard deviations of MR signal intensities show a consistent trend during imaging follow-ups for glioblastoma patients when corrected for non-biological heterogeneity due to hardware and software variation. Clin Neurol Neurosurg 2023;224:107553. [Crossref] [PubMed]