Comparison of dual-energy computed tomography (DECT) polychromatic and monochromatic images with and without iterative metal artifact reduction algorithm in patients with dental implants
Introduction
Computed tomography (CT) is widely employed to diagnose head and neck diseases, such as tumors, inflammation, vascular diseases, and injuries. However, artifacts arising from metal dental implants often considerably affect the examination outcomes. Dental implants often contain high atomic number materials, which can lead to artifacts in CT images, including photon starvation, beam hardening, and scatter artifacts (1-3). In addition, older patients often have more than one dental implant, which makes artifact correction harder.
There are numerous strategies for reducing metal artifacts in CT images. The traditional artifact removal method involves increasing the tube voltage and current; however, this method also increases the radiation dose (4). In terms of hardware, dual-energy CT (DECT) imaging effectively improves the quality of CT images of patients with metal implants (5). This technique uses two tube voltage (kV)-triggered X-rays to simultaneously scan an object and create two image sets, subsequently obtaining polychromatic images (i.e., linear blending images) or monochromatic images (i.e., virtual monoenergetic images) alongside postprocessing to reduce metal artifacts. The polychromatic images utilize different composition ratios to linearly blend high and low kV images. With an increase in composition ratio, the rendered image is more characteristic of a low kV image. Therefore, polychromatic images with a low composition ratio can simulate high kV images to reduce the influence of metal artifacts (6,7). DECT detects low and high energy photons separately, allowing for reconstructions of the monochromatic images that correspond to images resulting from true monoenergetic X-ray examinations. Monochromatic images at higher keV values are more resistant against beam hardening and thereby reduce metal artifacts (8-11). In addition to DECT techniques, there are many reconstruction techniques that can reduce metal artifacts, such as dedicated metal artifact reduction (MAR) algorithms. These algorithms identify the projected data of damage due to the presence of metal and then replace them with approximate or interpolated data. Metal artifacts reduce the effects of beam hardening and photon hunger. Currently, some vendors offer their own versions of MAR, such as iterative MAR (iMAR, Siemens Healthineers, Forchheim, Germany), MAR for orthopedic implants (O-MAR, Philips Healthcare, Best, Netherlands), single-energy MAR (SEMAR, Canon Medical Systems, Otawara, Japan), and smart MAR (SmartMAR, GE Healthcare, Waukesha, USA) (12-14).
Recent studies have investigated the significance of using DECT in combination with the MAR algorithm. For example, the combined application of DECT monochromatic images and iMAR to reduce the effect of metal artifacts in imaging patients with spinal fixation nails, total knee arthroplasty, and total shoulder prostheses was analyzed. Most results revealed that 90–170 keV images can achieve good MAR effects while maintaining acceptable image quality (15-19). The latest Photon-Counting detector CT study explored the effects of different reconstruction kernels and DECT monochromatic images combined with iMAR technology (20). In these studies, the exploration of DECT polychromatic images with different composition ratios was only seen in knee arthroplasty study (21).
Therefore, this study aimed to compare the MAR effect of polychromatic images with different composition ratios and monochromatic images with multiple keV with or without using iMAR in patients with dental implants. We present this article in accordance with the STROBE reporting checklist (available at https://qims.amegroups.com/article/view/10.21037/qims-24-19/rc).
Methods
Patients
This retrospective study was approved by the local institutional review board of Liuzhou Municipal Liutie Central Hospital (reference No. KY2023-055-01) and was conducted in accordance with the Declaration of Helsinki (as revised in 2013). The requirement for informed consent was waived. Between April and May 2022, 132 patients who had undergone head and neck DECT examinations were retrospectively identified. Among them, 55 patients had dental implants, including 30 patients with unremovable metal dental implants who were ultimately enrolled, including 16 men and 14 women (mean age, 69.2±10.1 years; range, 50–89 years). Of the 30 patients enrolled, 5 had one or two implants, 11 had three or four, and 14 had more than four. Patients were divided into two groups according to the number of implants for subgroup analysis. More than 4 implants were considered as large implants (n=14) and the others as small implants (n=16). The implants placed on side by side. Individual implant sizes range from 7.6 to 13 mm. Scanning was performed for clinical reasons only; no scans were conducted explicitly for this study.
Image acquisition
All examinations were performed via dual-source CT (SOMATOM Drive; Siemens Healthineers, Forchheim, Germany). Standard dual-energy (DE) protocol of the manufacturer was applied with the following parameters: A tube voltage, 100 kV; B tube voltage, 140 kV (tin plate filtered); the average effective tube current was 91 mAs and automatic tube current modulation technology (CARE Dose 4D) was used for automatic dose control; detector collimation was 64×0.6 mm; pitch was 0.9; rotation time was 0.28 s; and the matrix was 512×512. An adaptive model-based iterative reconstruction technology based on the Q30f algorithm was adopted, and the thickness of the reconstructed CT images was 1.0 mm.
DECT images with and without using iMAR were reconstructed, including polychromatic and monochromatic images (Figure 1). The polychromatic images were linearly blended from 100 kV and tin-filtered (Sn) 140 kV images, which could be reconstructed using the scanning platform (FAST DE, Siemens Healthineers), and the composition ratios used in this study were −1, −0.6, −0.3, 0, and 0.6. Polychromatic images with composition ratios of −1, −0.6, −0.3, 0, and 0.6 were generated by mixing −100% of 100 kV images and 200% of Sn 140 kV images, −60% of 100 kV images and 160% of Sn 140 kV images, −30% of 100 kV images and 130% of Sn 140 kV images, 0% of 100 kV images and 100% of Sn 140 kV images, and 60% of 100 kV images and 40% of Sn 140 kV images, respectively. A polychromatic image with a composition ratio of 0.6 was equivalent to a 120 kV image according to the vendor’s reference, which can also be regarded as a conventional image (6,22). All images were transmitted to a workstation (Syngo.via, VB20A, Siemens Healthineers, Forchheim, Germany) and analyzed using DE software (Mono, Syngo.via, VB20A, Siemens Healthineers, Forchheim, Germany) to reconstruct virtual monochromatic images (90, 110, 130, 150, and 170 keV). iMAR selected the dental filling mode. All images were analyzed and measured using MM image viewer (Syngo.via, VB20A, Siemens Healthineers).
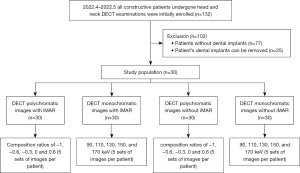
Subjective analysis
Two radiologists who did not participate in the quantitative analysis qualitatively graded the artifact and image quality of each image set using a 5-point Likert scale (with 1 being the best and 5 being the worst). The artifact score depends on the visualization of boundary and details of anatomical structures such as muscle, fat and bone. The score is defined as follows: 1= no artifact, 2= minimal streak artifact that does not affect the structure in question, 3= mild streak artifact that somewhat affects the structure, 4= moderate streak artifact that considerably affects the structure, and 5= severe streak artifact that considerably affects the structure. The image quality is assessed based on the diagnostic interpretability of soft palate, mouth floor, and buccal soft tissue. The evaluation criteria included 1= fully diagnostic, 2= diagnostic without impairment, 3= diagnostic with little impairment, 4= diagnostic with relevant impairment, and 5= nondiagnostic.
Objective analysis
Image measurements were performed by two radiologists with >5 years of experience in diagnostic imaging. Overall, 20 image sets (polychromatic images combined with iMAR, polychromatic images without iMAR, monochromatic images combined with iMAR, and monochromatic images without iMAR) were compared and measured. All the images were present with a window width of 250 and window level of 50. Two slices of the images were selected for each patient: one with the most serious artifacts, referred to as the artifact slice; and the other an adjacent image with no artifacts in the subjective view, considered the normal slice. Following that, the near artifact region of the tongue (within 2 cm from the anterior oropharyngeal wall), far artifact region (the posterior cervical muscle opposite the oral implant), and air region of the posterior cervical of each selected image were measured. The region of interest (ROI) size was 0.5 cm2, and the mean and standard deviation (SD) of each region were recorded. The same position of the other images was measured using MM image viewer (Syngo.via, VB20A, Siemens Healthineers). The contrast-to-noise ratio (CNR) and artifact index (AI) were calculated as follows:
The tongue and muscle ROIs were taken from the same slice.
Statistical analysis
Herein, SPSS (version 25.0; SPSS Inc., IBM Corp., IL, USA) and R language (R, version 4.1.1, R Foundation for Statistical Computing, Vienna, Austria) were used for statistical analysis. The Shapiro-Wilk test was used to assess normal distribution. Normally distributed continuous data were displayed as mean ± SD, and median (interquartile range) was used to represent non-normally distributed continuous data. Intraclass correlation coefficient (ICC) was employed to evaluate the consistency of quantitative data, and the weighted Cohen kappa method (R package: vcd) was used to assess the consistency of the subjective scores. The CT attenuation and SD values of the tongue and posterior neck muscles at the maximum artifact and normal slices were recorded and plotted, and the SD values of air at the normal slice were measured as background noise. The CNR and AI were calculated, and paired t-test compared the quantitative data from artifact and normal slices. A stacked bar chart was used to display the frequency distribution of the subjective ratings. Friedman test was used to assess the differences in the CNR, AI, subjective image quality score, and subjective artifact score between the image sets. The P value was corrected via Bonferroni correction. CT attenuation values, CNR and AI obtained from the maximum artifact slice, subjective image quality score and subjective artifact score were compared in a subgroup analysis. Independent t-test was used to compare differences between the two groups for normal data, and Mann-Whitney U test was used to analyze non-normally distributed data. A P value of <0.05 was considered significant.
Results
Subjective analysis
Table 1 shows the subjective image quality scores of 20 image sets. Radiologists independently scored the MAR effect and overall image quality. The weighted Cohen kappa test demonstrated an excellent interobserver agreement of the subjective scores. The kappa value of the subjective scores ranged from 0.6137 to 0.9681. The image with the optimal metal artifact score was iMAR + DE polychromatic (−0.3) with a median score of 2 (interquartile range: 2–3). Friedman test revealed that DE polychromatic (−0.3) images and DE monochromatic (110 keV) images, and all the iMAR image sets showed no significant difference in the metal artifact scores compared with iMAR + DE polychromatic (−0.3) images (all P>0.05). iMAR + DE monochromatic (110 keV) images exhibited the highest overall image quality score with a median score of 2 (interquartile range: 2–3). The scores of the DE polychromatic (−0.3 and 0) images and all the image sequences using iMAR did not significantly differ from those of iMAR + DE monochromatic (110 keV) image sets (all P>0.05). The stacked bar chart in Figure 2 shows the 5-point scoring results of the radiologists for artifacts and overall image quality in each image set. The metal artifact and image quality scores improved following the use of iMAR. Figure 3 shows the CT images of a patient in the artifact and normal slices wherein it can be intuitively understood that iMAR effectively removed metal artifacts without affecting the quality of the normal image.
Table 1
Variable | Metal artifact | Total image quality | |||||
---|---|---|---|---|---|---|---|
Kappa | Artifact slice | P value | Kappa | Artifact slice | P value | ||
DE polychromatic | |||||||
−1 | 0.7766 | 4 [3−5] | <0.001 | 0.9398 | 4 [3−5] | <0.001 | |
−0.6 | 0.8373 | 4 [3−4.25] | <0.001 | 0.7929 | 4 [3−4] | <0.001 | |
−0.3 | 0.9563 | 3 [3−4] | 0.081 | 0.8618 | 3 [3−4] | 0.103 | |
0 | 0.7129 | 3 [3−4] | 0.013 | 0.8975 | 3 [3−4] | 0.091 | |
0.6 | 0.9681 | 4 [3−5] | <0.001 | 0.9133 | 4 [3−4.25] | <0.001 | |
iMAR + DE polychromatic | |||||||
−1 | 0.8921 | 3 [2−3] | >0.99 | 0.9359 | 3 [2.75−3] | 0.959 | |
−0.6 | 0.8834 | 3 [2−3] | >0.99 | 0.9619 | 3 [2−3] | >0.99 | |
−0.3 | 0.9516 | 2 [2−3] | − | 0.92 | 2.5 [2−3] | >0.99 | |
0 | 0.6137 | 3 [2−3] | >0.99 | 0.7872 | 3 [2−3] | >0.99 | |
0.6 | 0.9065 | 3 [3−4] | 0.331 | 0.9571 | 3 [3−4] | 0.202 | |
DE monochromatic | |||||||
90 keV | 0.8760 | 4 [3−4] | <0.001 | 0.8561 | 3.5 [3−4] | <0.001 | |
110 keV | 0.8723 | 3 [3−3] | 0.570 | 0.8743 | 3 [3−3] | <0.001 | |
130 keV | 0.9263 | 3 [3−4] | 0.001 | 0.8554 | 3 [3−4] | 0.001 | |
150 keV | 0.9608 | 4 [3−4] | <0.001 | 0.8488 | 4 [3−4] | <0.001 | |
170 keV | 0.8005 | 4 [3−4] | <0.001 | 0.9601 | 4 [3−4] | <0.001 | |
iMAR + DE monochromatic | |||||||
90 keV | 0.9372 | 3 [2−3] | >0.99 | 0.9527 | 3 [3−3] | >0.99 | |
110 keV | 0.8940 | 3 [2−3] | >0.99 | 0.9581 | 2 [2−3] | − | |
130 keV | 0.9458 | 3 [2−3] | >0.99 | 0.9552 | 3 [2−3] | >0.99 | |
150 keV | 0.8699 | 3 [2−3] | >0.99 | 0.8684 | 3 [2−3] | >0.99 | |
170 keV | 0.8983 | 3 [2−3] | >0.99 | 0.7692 | 3 [2−3] | >0.99 |
The score is shown as the median [interquartile range]. The kappa value is weighted Cohen kappa. Each image set was compared with the optimal image set using Friedman test. P value was adjusted by Bonferroni-adjusted. P>0.05 is considered to have no significant difference. DE, dual energy; iMAR, iterative metal artifact reduction algorithm.
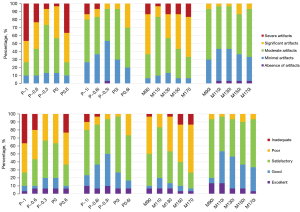
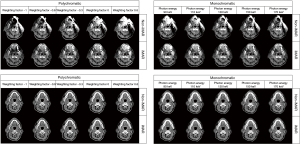
Objective analysis
The CT and SD values of the tongue and posterior neck muscles were measured in 20 sets of image sequences (Table 2), and the consistency of the data was analyzed. ICC revealed that the interobserver agreement of the objective measurement data was very high and that the data results ranged from 0.919 to 1.000. There was no significant difference in the CT values of the tongue between the artifact and normal slices in the DE polychromatic (−1 and −0.6) (P=0.635 and 0.433), DE monochromatic (150 and 170 keV) images (P=0.239 and 0.486), but there was significant difference in other images (all P<0.05). In addition, notably, the SD of the DE polychromatic (−1 and −0.6) images in the artifact slice data was considerable (CT value, 15.1±105.7 and 36.4±64.4 HU, respectively), and the CT value distribution was highly scattered. There were no significant differences in the sequence CT values for the posterior cervical muscles that were not near the metal implants.
Table 2
Variable | ICC | CT attenuation number (HU) | SD (HU) | |||||||||||||
---|---|---|---|---|---|---|---|---|---|---|---|---|---|---|---|---|
Tongue | Muscle | Tongue | Muscle | |||||||||||||
Artifact slice | Normal slice | P | Artifact slice | Normal slice | P | Artifact slice | Normal slice | P | Artifact slice | Normal slice | P | |||||
DE polychromatic | ||||||||||||||||
−1 | 0.993 | 15.1±105.7 | 24.3±13.3 | 0.635 | 55.4±19.1 | 50.0±9.9 | 0.139 | 36.0±13.6 | 20.8±3.9 | <0.001 | 17.6±3.9 | 14.2±2.8 | <0.001 | |||
−0.6 | 0.996 | 36.4±64.4 | 27.2±11.3 | 0.433 | 53.9±14.9 | 50.2±8.9 | 0.180 | 33.4±10.0 | 20.7±4.0 | <0.001 | 17.4±3.8 | 14.1±2.8 | <0.001 | |||
−0.3 | 0.990 | 52.2±39.5 | 29.5±10.4 | 0.003 | 53.1±12.3 | 50.8±8.1 | 0.304 | 27.9±7.8 | 18.5±3.8 | <0.001 | 14.9±3.6 | 12.4±2.5 | 0.001 | |||
0 | 0.996 | 68.3±36.6 | 31.9±10.3 | <0.001 | 52.5±10.6 | 51.9±7.4 | 0.742 | 21.5±8.7 | 13.8±3.8 | <0.001 | 10.1±3.6 | 7.9±1.9 | 0.002 | |||
0.6 | 0.994 | 100.0±90.9 | 36.3±12.3 | <0.001 | 50.6±11.6 | 53.2±8.2 | 0.231 | 31.1±23.5 | 14.7±4.1 | <0.001 | 11.7±4.8 | 8.1±2.1 | <0.001 | |||
iMAR + DE polychromatic | ||||||||||||||||
−1 | 0.995 | 54.1±61.8 | 25.0±14.3 | 0.013 | 55.1±14.9 | 49.9±9.9 | 0.101 | 22.2±4.5 | 21.7±3.9 | 0.511 | 16.5±3.5 | 14.8±2.6 | 0.007 | |||
−0.6 | 0.919 | 57.6±39.3 | 28.5±13.0 | <0.001 | 52.1±13.7 | 50.3±9.0 | 0.503 | 20.2±4.0 | 21.6±3.9 | 0.102 | 16.5±3.3 | 14.8±2.7 | 0.006 | |||
−0.3 | 0.996 | 58.9±32.7 | 30.9±12.0 | <0.001 | 51.1±12.4 | 50.7±8.3 | 0.889 | 18.2±4.2 | 19.1±3.6 | 0.285 | 14.5±3.4 | 12.7±2.5 | 0.004 | |||
0 | 0.996 | 57.7±30.3 | 33.4±11.8 | <0.001 | 50.9±11.7 | 51.8±7.9 | 0.686 | 14.9±4.8 | 14.0±3.6 | 0.381 | 10.4±3.9 | 8.2±1.9 | 0.003 | |||
0.6 | 0.995 | 60.3±40.1 | 38.0±12.4 | 0.005 | 49.0±11.4 | 53.3±8.6 | 0.053 | 16.8±5.7 | 15.1±3.9 | 0.182 | 11.7±4.8 | 8.2±2.0 | <0.001 | |||
DE monochromatic | ||||||||||||||||
90 keV | 0.996 | 82.5±47.5 | 33.7±11.3 | <0.001 | 50.8±10.3 | 51.0±7.8 | 0.922 | 25.5±11.2 | 15.9±3.3 | <0.001 | 11.9±3.3 | 10.0±2.0 | 0.003 | |||
110 keV | 0.997 | 59.9±32.9 | 31.5±11.7 | <0.001 | 51.7±11.2 | 50.4±8.2 | 0.498 | 27.4±6.6 | 19.1±3.3 | <0.001 | 16.2±6.2 | 13.1±2.6 | 0.018 | |||
130 keV | 0.996 | 47.9±44.0 | 30.1±12.2 | 0.031 | 52.3±12.7 | 50.0±8.6 | 0.314 | 32.4±8.3 | 21.4±3.4 | <0.001 | 17.9±3.8 | 15.1±2.9 | 0.058 | |||
150 keV | 0.996 | 41.1±54.0 | 29.5±12.6 | 0.239 | 50.9±16.1 | 49.8±8.8 | 0.679 | 35.5±9.3 | 22.9±3.5 | <0.001 | 19.6±4.1 | 16.3±3.4 | 0.001 | |||
170 keV | 0.996 | 36.7±60.7 | 29.0±13.1 | 0.486 | 52.8±14.3 | 49.6±9.0 | 0.235 | 37.5±10.1 | 23.7±3.5 | <0.001 | 20.6±4.2 | 17.1±3.4 | 0.001 | |||
iMAR + DE monochromatic | ||||||||||||||||
90 keV | 0.996 | 60.4±33.8 | 35.2±12.2 | <0.001 | 49.4±10.7 | 51.0±8.3 | 0.438 | 17.3±4.8 | 16.4±3.1 | 0.372 | 12.4±3.3 | 10.4±2.7 | 0.004 | |||
110 keV | 0.996 | 56.2±32.3 | 32.7±12.9 | <0.001 | 50.2±11.4 | 50.3±8.5 | 0.953 | 20.0±5.0 | 20.0±3.1 | 0.974 | 16.2±6.7 | 14.1±2.6 | 0.078 | |||
130 keV | 0.996 | 53.7±36.4 | 31.3±13.8 | 0.002 | 50.8±12.1 | 50.1±8.8 | 0.774 | 22.1±5.9 | 22.6±3.3 | 0.721 | 17.3±3.1 | 16.2±3.0 | 0.073 | |||
150 keV | 0.922 | 52.6±40.5 | 30.6±14.2 | 0.005 | 51.0±12.7 | 49.8±9.1 | 0.635 | 23.8±6.6 | 24.0±3.4 | 0.842 | 18.8±3.2 | 17.6±3.5 | 0.082 | |||
170 keV | 1.000 | 51.7±43.2 | 30.0±14.6 | 0.009 | 51.1±13.1 | 49.8±9.3 | 0.616 | 24.7±6.9 | 25.0±3.4 | 0.792 | 19.6±3.4 | 18.4±3.7 | 0.095 |
The CT and SD values of the tongue and muscle are reported as mean ± standard deviation. Paired t-test was performed to assess the values of the artifact and normal slices. P<0.05 was considered significant. CT, computed tomography; SD, standard deviation; ICC, intraclass-correlation coefficient; HU, Hounsfield unit; DE, dual energy; iMAR, iterative metal artifact reduction algorithm.
Figure 4 shows the CT attenuation and SD value distribution of 20 image sets in the tongue and muscles. The distribution of CT attenuation values in the tongue at DE polychromatic (−1 and −0.6) images was very dispersed, and the mean CT attenuation in the artifact slice was larger than that in the normal slice (Figure 4A). For the posterior neck muscles, the CT values of the tongue were similar in the artifact and normal slices (Figure 4B). The SD value of the tongue in the artifact slice was very close to that of the normal slice in the image sets with iMAR, whereas the SD was significantly higher in the artifact slice than that of the normal slice in the image sets without iMAR (Figure 4C). The SD distribution of the posterior cervical muscles was similar in all the images, and the mean SD of the artifact slice was slightly larger than that of the normal slice (Figure 4D).
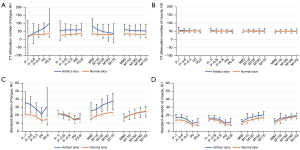
Paired t-test revealed that the CNR of the artifact and normal slices were only significantly different in the DE polychromatic (−1 and 0.6) images (Table 3). There was no significant difference in the AI values between the artifact and normal slices in the image sets with iMAR (P>0.05). The value of AI was the lowest [median (interquartile range): 8.7 (5.9–14.5)] in iMAR + DE polychromatic (0) images. The result of Friedman test revealed that the images obtained using iMAR or the DE polychromatic (0) images exhibited no significant difference with the iMAR + DE polychromatic (0) images (P>0.05). Figure 5 shows that the AI value significantly decreased after using iMAR compared with that of the original image sets.
Table 3
Variable | CNR | AI | |||||||
---|---|---|---|---|---|---|---|---|---|
Artifact slice | Normal slice | P1 | P2 | Artifact slice | Normal slice | P1 | P2 | ||
DE polychromatic | |||||||||
−1 | 3.19 (1.08−9.18) | 2.22 (1.53−3.93) | 0.015 | <0.001 | 28.2 (18.8−41.2) | 14.3 (10.9−17.3) | 0.736 | <0.001 | |
−0.6 | 1.69 (0.69−5.04) | 2.00 (1.48−3.18) | 0.134 | 0.512 | 27.1 (19.7−37.6) | 13.9 (10.8−17.3) | <0.001 | <0.001 | |
−0.3 | 1.29 (0.44−3.25) | 2.00 (1.58−3.31) | 0.555 | >0.99 | 23.2 (16.8−29.8) | 12.8 (9.9−15.6) | <0.001 | <0.001 | |
0 | 2.47 (0.62−4.50) | 3.50 (2.07−5.00) | 0.184 | >0.99 | 17.3 (11.5−21.8) | 10.0 (7.1−14.5) | 0.009 | 0.397 | |
0.6 | 4.60 (1.21−11.28) | 2.45 (1.28−4.20) | 0.003 | 0.002 | 22.8 (13.4−32.5) | 11.4 (8.6−15.9) | 0.002 | <0.001 | |
iMAR + DE polychromatic | |||||||||
−1 | 1.19 (0.51−3.31) | 2.18 (1.15−3.61) | 0.875 | >0.99 | 13.5 (8.4−18.9) | 14.7 (11.3−18.0) | 0.576 | >0.99 | |
−0.6 | 1.25 (0.58−2.65) | 1.89 (0.94−3.04) | 0.331 | >0.99 | 11.0 (6.0−15.7) | 14.9 (11.9−17.8) | 0.063 | >0.99 | |
−0.3 | 1.97 (0.61−2.93) | 2.01 (0.82−3.07) | 0.598 | >0.99 | 11.6 (0−13.6) | 12.9 (9.9−16.7) | 0.279 | >0.99 | |
0 | 1.62 (0.55−3.59) | 2.73 (1.19−4.85) | 0.337 | >0.99 | 8.7 (5.9−14.5) | 10.0 (8.1−14.5) | 0.911 | − | |
0.6 | 1.50 (0.29−4.11) | 2.40 (1.07−4.23) | 0.401 | >0.99 | 10.4 (4.2−15.1) | 11.7 (8.5−15.9) | 0.282 | >0.99 | |
DE monochromatic | |||||||||
90 keV | 2.14 (0.92−4.68) | 2.33 (1.20−3.50) | 0.068 | 0.612 | 20.4 (14.4−25.3) | 11.1 (8.8−15.1) | <0.001 | 0.001 | |
110 keV | 1.00 (0.54−2.50) | 1.81 (1.29−2.86) | 0.160 | − | 23.2 (15.2−27.0) | 13.0 (9.5−16.0) | <0.001 | 0.001 | |
130 keV | 1.02 (0.49−3.34) | 1.57 (1.18−2.51) | 0.632 | >0.99 | 27.8 (19.1−33.9) | 14.3 (11.5−17.4) | <0.001 | <0.001 | |
150 keV | 1.18 (0.39−4.09) | 1.67 (1.11−2.55) | 0.149 | >0.99 | 30.1 (21.0−37.6) | 15.4 (11.6−18.3) | <0.001 | <0.001 | |
170 keV | 1.52 (0.47−3.92) | 1.60 (1.07−2.35) | 0.062 | >0.99 | 30.9 (23.0−41.3) | 15.6 (11.7−19.0) | <0.001 | <0.001 | |
iMAR + DE monochromatic | |||||||||
90 keV | 1.66 (0.31−3.04) | 2.25 (0.77−3.50) | 0.550 | >0.99 | 10.8 (5.5−17.0) | 11.2 (9.6−14.9) | 0.609 | >0.99 | |
110 keV | 1.38 (0.49−3.00) | 1.70 (0.60−2.61) | 0.872 | >0.99 | 12.2 (7.1−15.3) | 13.6 (9.3−16.5) | 0.895 | >0.99 | |
130 keV | 1.60 (0.48−2.63) | 1.65 (0.65−2.45) | 0.734 | >0.99 | 11.6 (6.1−15.7) | 14.8 (11.7−17.7) | 0.566 | >0.99 | |
150 keV | 1.36 (0.49−2.64) | 1.52 (0.66−2.31) | 0.585 | >0.99 | 12.2 (6.7−16.3) | 16.0 (12.0−18.2) | 0.603 | >0.99 | |
170 keV | 1.28 (0.47−2.60) | 1.52 (0.67−2.30) | 0.627 | >0.99 | 12.0 (4.8−17.6) | 16.5 (12.3−19.5) | 0.715 | >0.99 |
The data are shown as median (interquartile range). Paired t-test was used to compare the difference between the artifact and normal slices, and the results are shown in P1. Friedman test was used to compare the paired images with the optimal images, and the results are shown in P2. P1 and P2 <0.05 were considered significant. CNR, contrast-to-noise ratio; AI, artifact index; iMAR, iterative metal artifact reduction algorithm; DE, dual energy.
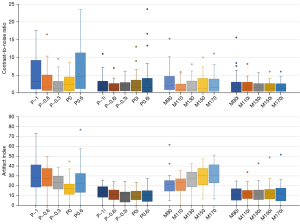
Subgroup analysis
Table 4 shows the comparison between large and small implants for CT attenuation values, CNR and AI. The data showed that the mean and variance of tongue CT attenuation in patients with large implants measured at the artifact level were almost greater than those in images with small implants. However, the two groups of data showed significant differences only for iMAR + DE polychromatic (−0.3, 0 and 0.6) and iMAR + DE monochromatic (90 and 110 keV) (P<0.05). It was observed that the variance of CT values obtained by these techniques was smaller, suggesting that the data were more concentrated, making the difference significant. For CNR and AI, there was no significant difference between the two groups for the techniques combined with iMAR (P>0.05). There was also no difference between the two groups (P>0.05) for any of the techniques in terms of subjective scores.
Table 4
Variable | CT value of tongue (HU) | CNR | AI | ||||||||
---|---|---|---|---|---|---|---|---|---|---|---|
Large (n=14) | Small (n=16) | P | Large (n=14) | Small (n=16) | P | Large (n=14) | Small (n=16) | P | |||
DE polychromatic | |||||||||||
−1 | 14.0±122.3 | 16.1±92.9 | 0.959 | 4.07 (1.2−7.5) | 2.22 (1.0−10.3) | 0.739 | 33.8 (25.0−47.7) | 22.5 (16.6−33.1) | 0.032* | ||
−0.6 | 39.4±73.7 | 33.8±57.4 | 0.814 | 1.96 (0.5−4.6) | 1.40 (0.8−6.8) | 0.901 | 31.1 (25.7−40.6) | 21.6 (15.3−34.8) | 0.034* | ||
−0.3 | 57.4±47.2 | 47.6±32.3 | 0.51 | 0.93 (0.4−3.6) | 1.58 (0.3−3.0) | 0.983 | 25.0 (21.1−31.6) | 19.6 (11.3−28.3) | 0.056 | ||
0 | 74.6±47.3 | 62.8±24.1 | 0.412 | 3.23 (2.3−5.1) | 0.72 (0.3−4.1) | 0.022* | 20.9 (13.7−26.7) | 15.2 (9.7−19.1) | 0.038* | ||
0.6 | 108.9±109.4 | 92.3±73.9 | 0.625 | 7.08 (2.7−11.7) | 2.11 (0.1−10.4) | 0.096 | 29.0 (17.1−45.1) | 18.5 (10.7−26.6) | 0.077 | ||
iMAR + DE polychromatic | |||||||||||
−1 | 64.9±64.9 | 44.6±59.4 | 0.379 | 1.43 (0.5−3.3) | 1.06 (0.3−3.5) | 0.934 | 13.5 (8.4−20.0) | 14.1 (6.8−18.0) | 0.618 | ||
−0.6 | 72.1±39.0 | 44.9±36.1 | 0.058 | 0.93 (0.6−2.2) | 2.03 (0.5−3.0) | 0.36 | 9.9 (0.0−12.7) | 12.1 (7.4−17.2) | 0.108 | ||
−0.3 | 75.5±30.9 | 44.4±27.6 | 0.007** | 1.66 (0.7−2.9) | 1.97 (0.4−3.0) | 0.771 | 10.3 (0.0−12.0) | 12.0 (7.5−16.6) | 0.106 | ||
0 | 75.1±28.7 | 42.5±23.2 | 0.002** | 1.68 (0.7−3.3) | 1.55 (0.2−3.9) | 0.771 | 8.1 (4.4−11.1) | 10.4 (7.2−15.2) | 0.327 | ||
0.6 | 81.1±40.6 | 42.1±30.5 | 0.006** | 2.01 (0.5−4.1) | 1.50 (0.3−4.9) | 0.506 | 9.5 (0.0−15.5) | 11.1 (6.1−16.9) | 0.558 | ||
DE monochromatic | |||||||||||
90 keV | 92.3±55.1 | 74.0±40.5 | 0.305 | 3.27 (1.3−4.6) | 1.59 (0.5−5.9) | 0.454 | 25.1 (16.3−33.5) | 15.8 (11.2−22.1) | 0.006** | ||
110 keV | 66.1±39.9 | 54.5±25.4 | 0.342 | 1.41 (0.6−3.0) | 0.90 (0.3−1.4) | 0.339 | 26.5 (21.9−31.7) | 18.6 (12.8−23.5) | 0.011* | ||
130 keV | 52.2±51.5 | 44.1±37.5 | 0.624 | 0.91 (0.5−3.2) | 1.20 (0.3−3.6) | 0.803 | 30.1 (26.8−36.0) | 21.5 (15.9−32.2) | 0.034* | ||
150 keV | 44.3±62.1 | 38.4±47.8 | 0.771 | 1.77 (0.7−3.6) | 1.06 (0.3−4.7) | 0.533 | 34.1 (29.3−38.8) | 23.0 (17.5−35.6) | 0.036* | ||
170 keV | 39.4±69.1 | 34.4±54.4 | 0.824 | 2.27 (0.9−3.8) | 0.92 (0.3−5.5) | 0.533 | 36.8 (31.0−41.6) | 23.6 (18.3−36.4) | 0.029* | ||
iMAR + DE monochromatic | |||||||||||
90 keV | 79.7±33.2 | 43.6±24.6 | 0.002** | 1.59 (0.6−4.8) | 1.70 (0.2−2.4) | 0.533 | 9.3 (3.9−14.4) | 11.8 (7.3−17.2) | 0.491 | ||
110 keV | 71.6±30.2 | 42.7±28.5 | 0.012* | 1.25 (0.6−2.5) | 1.55 (0.2−3.5) | 0.787 | 10.1 (0.0−15.3) | 12.7 (8.7−15.5) | 0.242 | ||
130 keV | 67.1±36.7 | 41.9±32.9 | 0.057 | 1.40 (0.5−2.2) | 1.64 (0.3−3.1) | 0.589 | 10.6 (0.0−14.7) | 13.0 (9.3−17.4) | 0.242 | ||
150 keV | 64.9±42.1 | 41.9±37.1 | 0.123 | 1.07 (0.5−2.3) | 1.73 (0.4−2.9) | 0.603 | 8.5 (4.7−15.7) | 13.2 (10.1−18.8) | 0.163 | ||
170 keV | 63.1±45.9 | 41.8±39.4 | 0.18 | 1.00 (0.5−2.4) | 1.75 (0.4−3.0) | 0.519 | 9.1 (0.0−16.9) | 13.6 (9.9−20.5) | 0.107 |
Normally distributed data are expressed as mean ± standard deviation. Non-normal data are shown as median (interquartile range). Independent t-test was used to compare the difference of CT attenuation number. Mann-Whitney U test was used for CNR and AI. P<0.05 was considered to show a significant difference. *, P<0.05; **, P<0.01. CT, computed tomography; HU, Hounsfield unit; CNR, contrast-to-noise ratio; AI, artifact index; DE, dual energy; iMAR, iterative metal artifact reduction algorithm.
Discussion
Herein, the quality of polychromatic and monochromatic DECT images obtained with and without using iMAR in patients with dental implants was analyzed. The subjective analysis results revealed that using DECT polychromatic or monochromatic images reduced metal artifacts. Furthermore, iMAR combined with DECT significantly improved image quality and decreased metal artifacts, regardless of implant size. In addition, we found that the combination of iMAR and DE can help doctors diagnose oral masses in patients with dental implants (Figure 6).
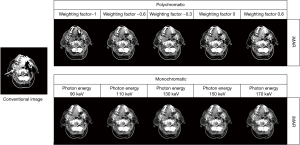
To date, several studies have evaluated the usefulness of MAR and virtual monochromatic images for dental metal artifact and these studies have concluded that the combination yields optimal artifact reduction, compared to each method alone (18-20). Our results partially agree with those results, as iMAR-applied monochromatic images at high keV were superior to iMAR-applied 120-kV-equivalent polychromatic images for MAR. However, our results differ from those of the other studies on the optimal keV images, with the monochromatic images in other studies get the best performance at 130 or 140–200 keV, while in this study, the subjective score of the 110 keV monochromatic image was optimal for the 90–170 keV image sets, whereas the objective analysis results of the 90 keV monochromatic images were optimal; this difference can be explained by the fact that the equipment was from different manufacturers.
The major strength of our study lies in the novel use of polychromatic images with low composition ratios for MAR, rather than routinely using polychromatic images equivalent to 120 kV. Polychromatic images reconstruction is based on linear blending technology. Compared with virtual monochromatic images, which include complex calculations on the postprocessing workstation, linear blending technology is more convenient and faster. However, studies regarding MAR rarely include linear blending technology, and those that do usually only use an image set equivalent to 120 kV for analysis (19,23). Choo et al. innovatively used polychromatic images with multiple composition ratios combined with iMAR for metal artifact removal MAR in knee replacement (21). Inspired by them, we analyzed DE polychromatic and monochromatic images combined with iMAR in patients with dental implants, and the results showed that DE polychromatic images combined with iMAR exhibited the same effect as DE monochromatic images combined with iMAR. Polychromatic images with low weighting factors presumably correspond to images with high kV, while high keV monochromatic images simulate true monoenergetic X-ray examinations. The principles of the two reconstruction algorithms are similar, which may be the reason why these two techniques achieved similar performance. In addition, another innovation of the present study was thte use of the tin prefiltration technique in high kV scanning, i.e., DE polychromatic (0) image. The tin prefiltration technique can considerably filter out low-energy photons, thereby facilitating the reduction of beam-hardening artifacts (21,23). iMAR + DE polychromatic (0) image showed the lowest AI value in this study, which may be attributed to the application of tin prefiltration technique. Furthermore, many patients in our cohort had multiple dental implants, and multiple implants could be regarded as large implants. Therefore, we conducted a subgroup study to analyze the influence of implant size on artifact correction, and the results showed that large implants did increase the change of CT attenuation value compared with small implants. However, the techniques combined with iMAR showed no significant difference between the two groups in the indicators of subjective and objective assessment of image quality, demonstrating excellent robustness.
Regarding the research design of studies involving on removing metal artifacts, most studies analyze the surrounding conditions of metal implants, such as the volume of low- and high-density artifacts around metals, number of pixels affected by metal artifacts, or distribution of attenuation values in hypodense and hyperdense artifacts. Few studies have analyzed the artifact of the position far from the metal on the artifact slice and compared the normal image with the artifact slice (20). This study measured the CT values of the tongue (near the metal) as well as those of the posterior neck muscles (far from the metal) in the artifact and normal slices and compared the two positions in the same slice as well as the same position in the two slices. The results showed the difference of CT value and noise of each image set as well as the performance of metal artifacts in different positions, demonstrating the ability of iMAR and DE techniques to remove metal artifacts. We found that the SD value of the posterior neck muscle in some image sets was significantly higher in the artifact slice than in the normal slice, indicating that there was still some noise at a position far away from the metal in the artifact removal process, probably due to the overcorrection of the algorithm. Several studies have noted that MAR introduces new artifacts or causes some image distortion and blurring, which is also slightly reflected in the current study; however, this did not affect the diagnosis (1,8,18,24). In addition, the metal artifact correction may delete important information of the image, but we do not have a suitable method to evaluate it, and thus we look forward to having more evaluation methods in the future.
There are some limitations to this study. First, owing to the limited number of patients enrolled, more in-depth subgroup analysis could not be performed, such as the artifact removal effect of unilateral and bilateral metal implants and the grouping of dental implants with different metal materials (8,25,26). Second, the present study only used unenhanced data from head and neck CT image sets, and enhanced data can be analyzed in the future; in addition, there were not many patients with lesions observed, future studies need to evaluate the artifact reduction in assessment of pathologies of the surrounding soft tissue, e.g., squamous cell carcinoma.
Conclusions
To conclude, iMAR effectively reduces metal artifacts in the CT images of patients with dental implants, and better image quality can be obtained by combining DECT polychromatic or monochromatic images with iMAR.
Acknowledgments
The authors thank all physicians, nurses, radiographers, other team members, and patients who were involved in this study.
Funding: None.
Footnote
Reporting Checklist: The authors have completed the STROBE reporting checklist. Available at https://qims.amegroups.com/article/view/10.21037/qims-24-19/rc
Conflicts of Interest: All authors have completed the ICMJE uniform disclosure form (available at https://qims.amegroups.com/article/view/10.21037/qims-24-19/coif). L.L. and L.H. are employees of Siemens Healthineers, Ltd., but they were not involved in the analysis of the data and had no control of data or information submitted for publication. The other authors have no conflicts of interest to declare.
Ethical Statement: The authors are accountable for all aspects of the work in ensuring that questions related to the accuracy or integrity of any part of the work are appropriately investigated and resolved. The study was conducted in accordance with the Declaration of Helsinki (as revised in 2013). The study was approved by the Institutional Ethics Committee of Liuzhou Municipal Liutie Central Hospital (No. KY2023-055-01) and individual consent for this retrospective analysis was waived.
Open Access Statement: This is an Open Access article distributed in accordance with the Creative Commons Attribution-NonCommercial-NoDerivs 4.0 International License (CC BY-NC-ND 4.0), which permits the non-commercial replication and distribution of the article with the strict proviso that no changes or edits are made and the original work is properly cited (including links to both the formal publication through the relevant DOI and the license). See: https://creativecommons.org/licenses/by-nc-nd/4.0/.
References
- Laukamp KR, Lennartz S, Neuhaus VF, Große Hokamp N, Rau R, Le Blanc M, Abdullayev N, Mpotsaris A, Maintz D, Borggrefe J. CT metal artifacts in patients with total hip replacements: for artifact reduction monoenergetic reconstructions and post-processing algorithms are both efficient but not similar. Eur Radiol 2018;28:4524-33.
- Katsura M, Sato J, Akahane M, Kunimatsu A, Abe O. Current and Novel Techniques for Metal Artifact Reduction at CT: Practical Guide for Radiologists. Radiographics 2018;38:450-61. [Crossref] [PubMed]
- Mori I, Machida Y, Osanai M, Iinuma K. Photon starvation artifacts of X-ray CT: their true cause and a solution. Radiol Phys Technol 2013;6:130-41. [Crossref] [PubMed]
- Lee MJ, Kim S, Lee SA, Song HT, Huh YM, Kim DH, Han SH, Suh JS. Overcoming artifacts from metallic orthopedic implants at high-field-strength MR imaging and multi-detector CT. Radiographics 2007;27:791-803. [Crossref] [PubMed]
- Wang Y, Peng Y, Wang T, Li H, Zhao Z, Gong L, Peng B. The evolution and current situation in the application of dual-energy computed tomography: a bibliometric study. Quant Imaging Med Surg 2023;13:6801-13. [Crossref] [PubMed]
- Behrendt FF, Schmidt B, Plumhans C, Keil S, Woodruff SG, Ackermann D, Mühlenbruch G, Flohr T, Günther RW, Mahnken AH. Image fusion in dual energy computed tomography: effect on contrast enhancement, signal-to-noise ratio and image quality in computed tomography angiography. Invest Radiol 2009;44:1-6. [Crossref] [PubMed]
- Kim KS, Lee JM, Kim SH, Kim KW, Kim SJ, Cho SH, Han JK, Choi BI. Image fusion in dual energy computed tomography for detection of hypervascular liver hepatocellular carcinoma: phantom and preliminary studies. Invest Radiol 2010;45:149-57. [Crossref] [PubMed]
- Große Hokamp N, Laukamp KR, Lennartz S, Zopfs D, Abdullayev N, Neuhaus VF, Maintz D, Borggrefe J. Artifact reduction from dental implants using virtual monoenergetic reconstructions from novel spectral detector CT. Eur J Radiol 2018;104:136-42. [Crossref] [PubMed]
- Zeng Y, Deng K, Yang H, Tan Y, Liu J, Geng D, Zhang J. Noise-optimised virtual monoenergetic imaging of dual-energy CT: effect on metal artefact reduction in patients with lumbar internal fixation. Eur Spine J 2019;28:1783-92. [Crossref] [PubMed]
- Laukamp KR, Zopfs D, Wagner A, Lennartz S, Pennig L, Borggrefe J, Ramaiya N, Große Hokamp N. CT artifacts from port systems: Virtual monoenergetic reconstructions from spectral-detector CT reduce artifacts and improve depiction of surrounding tissue. Eur J Radiol 2019;121:108733. [Crossref] [PubMed]
- Foti G, Longo C, D'Onofrio M, Natali S, Piovan G, Oliboni E, Iacono V, Guerriero M, Zorzi C. Dual-Energy CT for Detecting Painful Knee Prosthesis Loosening. Radiology 2023;306:e211818. [Crossref] [PubMed]
- Guggenberger R, Winklhofer S, Osterhoff G, Wanner GA, Fortunati M, Andreisek G, Alkadhi H, Stolzmann P. Metallic artefact reduction with monoenergetic dual-energy CT: systematic ex vivo evaluation of posterior spinal fusion implants from various vendors and different spine levels. Eur Radiol 2012;22:2357-64. [Crossref] [PubMed]
- Park J, Kim SH, Han JK. Combined application of virtual monoenergetic high keV images and the orthopedic metal artifact reduction algorithm (O-MAR): effect on image quality. Abdom Radiol (NY) 2019;44:756-65. [Crossref] [PubMed]
- Bolstad K, Flatabø S, Aadnevik D, Dalehaug I, Vetti N. Metal artifact reduction in CT, a phantom study: subjective and objective evaluation of four commercial metal artifact reduction algorithms when used on three different orthopedic metal implants. Acta Radiol 2018;59:1110-8. [Crossref] [PubMed]
- Long Z, DeLone DR, Kotsenas AL, Lehman VT, Nagelschneider AA, Michalak GJ, Fletcher JG, McCollough CH, Yu L. Clinical Assessment of Metal Artifact Reduction Methods in Dual-Energy CT Examinations of Instrumented Spines. AJR Am J Roentgenol 2019;212:395-401. [Crossref] [PubMed]
- Chae HD, Hong SH, Shin M, Choi JY, Yoo HJ. Combined use of virtual monochromatic images and projection-based metal artifact reduction methods in evaluation of total knee arthroplasty. Eur Radiol 2020;30:5298-307. [Crossref] [PubMed]
- Mohammadinejad P, Baffour FI, Adkins MC, Yu L, McCollough CH, Fletcher JG, Glazebrook KN. Benefits of iterative metal artifact reduction and dual-energy CT towards mitigating artifact in the setting of total shoulder prostheses. Skeletal Radiol 2021;50:51-8. [Crossref] [PubMed]
- Laukamp KR, Zopfs D, Lennartz S, Pennig L, Maintz D, Borggrefe J, Große Hokamp N. Metal artifacts in patients with large dental implants and bridges: combination of metal artifact reduction algorithms and virtual monoenergetic images provides an approach to handle even strongest artifacts. Eur Radiol 2019;29:4228-38. [Crossref] [PubMed]
- Zhou Y, Lei L, Wang Z, Cao W, Qin M, Dong S, Dang J, Zhou Z. Utility of spectral CT with orthopedic metal artifact reduction algorithms for (125)I seeds implantation in mediastinal and hepatic tumors. Quant Imaging Med Surg 2024;14:698-710. [Crossref] [PubMed]
- Risch F, Decker JA, Popp D, Sinzinger A, Braun F, Bette S, Jehs B, Haerting M, Wollny C, Scheurig-Muenkler C, Kroencke TJ, Schwarz F. Artifact Reduction From Dental Material in Photon-Counting Detector Computed Tomography Data Sets Based on High-keV Monoenergetic Imaging and Iterative Metal Artifact Reduction Reconstructions-Can We Combine the Best of Two Worlds? Invest Radiol 2023;58:691-6. [Crossref] [PubMed]
- Choo HJ, Lee SJ, Kim DW, Lee YJ, Baek JW, Han JY, Heo YJ. Comparison of the Quality of Various Polychromatic and Monochromatic Dual-Energy CT Images with or without a Metal Artifact Reduction Algorithm to Evaluate Total Knee Arthroplasty. Korean J Radiol 2021;22:1341-51. [Crossref] [PubMed]
- Graser A, Johnson TR, Chandarana H, Macari M. Dual energy CT: preliminary observations and potential clinical applications in the abdomen. Eur Radiol 2009;19:13-23. [Crossref] [PubMed]
- Hackenbroch C, Schüle S, Halt D, Zengerle L, Beer M. Metal Artifact Reduction With Tin Prefiltration in Computed Tomography: A Cadaver Study for Comparison With Other Novel Techniques. Invest Radiol 2022;57:194-203. [Crossref] [PubMed]
- Huang JY, Kerns JR, Nute JL, Liu X, Balter PA, Stingo FC, Followill DS, Mirkovic D, Howell RM, Kry SF. An evaluation of three commercially available metal artifact reduction methods for CT imaging. Phys Med Biol 2015;60:1047-67. [Crossref] [PubMed]
- Neuhaus V, Große Hokamp N, Abdullayev N, Rau R, Mpotsaris A, Maintz D, Borggrefe J. Metal artifact reduction by dual-layer computed tomography using virtual monoenergetic images. Eur J Radiol 2017;93:143-8. [Crossref] [PubMed]
- Große Hokamp N, Hellerbach A, Gierich A, Jordan DW, Visser-Vandewalle V, Maintz D, Haneder S. Reduction of Artifacts Caused by Deep Brain Stimulating Electrodes in Cranial Computed Tomography Imaging by Means of Virtual Monoenergetic Images, Metal Artifact Reduction Algorithms, and Their Combination. Invest Radiol 2018;53:424-31. [Crossref] [PubMed]