Preoperative computed tomography semantic features in predicting lymph node metastasis of part-solid nodules in non-small cell lung cancer: a multicenter retrospective study
Introduction
Lung cancer remains the malignant tumor type with the highest morbidity and mortality in the world, of which non-small cell lung cancer (NSCLC) accounts for about 85% of all afflicted patients (1-4). Lymph node metastasis (LNM) is a common route of metastasis in lung cancer, and it is an independent risk factor for long-term survival and recurrence of patients with NSCLC: the 5-year survival rate is 49% for N1 disease, 36% for N2 disease, and 20% for N3 disease (5-7). Preoperative prediction of LNM directly informs the method of intraoperative lymph node dissection that is selected. These methods include systematic lymph node dissection, specific lymph node dissection, systematic lymph node sampling, and lymph node sampling, among others (8,9). The appropriate selection of method according to accurate the tumor node metastasis (TNM) staging of lymph node dissection can maximize the removal of tumor cells and avoid excessive dissection.
Computed tomography (CT), as a noninvasive and repeatable imaging examination method, is the most widely applied and commonly used lymph node assessment tool in clinical practice. With the popularization of low-dose CT screening technology, early lung cancer is being discovered in patients with increasing frequency, allowing for timely treatment by surgery (10,11). Pulmonary nodules are classified as solid, pure ground-glass, and part-solid nodules (PSNs) based on CT phenotyping (12). PSNs are defined as nodules containing both ground-glass opacity (GGO) and a solid portion (13). The Fleischner Society indicated in its 2017 report that from a therapeutic point of view, pure and part-solid ground-glass nodules should be considered as a category separate from purely solid lesions (14).
Pathological examination is the gold standard for the diagnosis of LNM in NSCLC, but its reproducibility is quite different, and invasive operation may induce adverse effects, such as tumor cell spread, bleeding, infection, etc. (15-17). The metastasis rate of lymph nodes in PSNs has been known to be low, which makes the intraoperative evaluation of lymph nodes a controversial issue (18). Therefore, a noninvasive method to accurately determine the status of lymph nodes before operation is necessary and urgently needed. Therefore, the purpose of this study was to identify the risk factors of LNM among the preoperative CT semantic features of patients with NSCLC manifesting as PSNs and to establish a predictive model with application value. We present this article in accordance with the STARD reporting checklist (available at https://qims.amegroups.com/article/view/10.21037/qims-23-1631/rc).
Methods
Patients
This retrospective study was conducted in accordance with the Declaration of Helsinki (as revised in 2013) and was approved by the respective ethics committees of Tongde Hospital of Zhejiang Province (approval No. 2022-029-JY), Taizhou Municipal Hospital (approval No. LWYJ2023059), and The First Affiliated Hospital of Bengbu Medical University (approval No. 2021-292). The requirement for individual informed consent for this retrospective analysis was waived.
On the premise that the diagnosis and treatment practices are generally consistent across centers, we used the hospital electronic medical record systems from three centers (Tongde Hospital of Zhejiang Province, Taizhou Municipal Hospital and The First Affiliated Hospital of Bengbu Medical University) and retrieved the files of all consecutive patients with a pathologic diagnosis of primary NSCLC surgically resected from January 2019 to March 2023. A total of 955 patients were enrolled according to the following inclusion criteria: (I) radical surgery and lymph node dissection were performed, and NSCLC was confirmed via postoperative pathology; (II) patients underwent unenhanced chest CT scan within 2 weeks before operation; (III) CT images were satisfactory for analysis; and (IV) the lesion appeared as a PSN. Meanwhile, the exclusion criteria were as follows: (I) patients received radiotherapy or chemotherapy before surgery, (II) the CT scan thickness was more than 2 mm, (III) clinical and pathological data could not be found or were incomplete, and (IV) patients had a history of malignant tumor. According to the presence or absence of LNM, the patients were divided into an LNM group and non-LNM (N-LNM) group (Figure 1). Clinical information including age, gender, smoking history, surgery history, and clinical basic disease was obtained through a search of the medical records.
CT image acquisition
Nonenhanced chest CT scans were performed with seven CT scanners (SOMATOM Definition Flash, FORCE CT, Sensation 16, Definition AS 40, Siemens Healthineers, Erlangen, Germany; Revolution, Optima 680, LightSpeed VCT XT, GE Healthcare, Chicago, IL, USA), and all images were reconstructed with a slice thickness of 0.625–2 mm. All patients were scanned in the supine position, held their arms up and head advanced, performed breath-holding at the end of a deep inhalation, and were scanned from the level of the thoracic entrance to that of the adrenal gland. The scanning parameters are as follows: tube voltage; 120 kV; automatic tube current modulation technology; collimation, 0.625 mm; pitch, 0.98; helical scanning speed, 0.6–0.8 s/r; image matrix, 512×512; and field of view (FOV) under 400 mm × 400 mm. The width of the lung window was 1,500 Hounsfield units (HU), the window level was −400 HU, the width of the mediastinal window was 400 HU, and the window level was 40 HU. After scanning, the data were transmitted to the workstation of the picture archiving and communication system (PACS) system.
Imaging evaluation
Blinded to the clinicopathologic data, two senior thoracic imaging diagnostic physicians independently interpreted the following CT features: the location, shape, and solid component proportion of the nodules; lobulation; spiculation; air cavity; pulmonary disease; air bronchogram type; and pleural contact type. Lobulation involves the irregular undulation of the nodule margin (19). Spiculation is defined as linear strands extending from the nodule surface into the lung parenchyma without reaching a pleural surface (20) that is caused by the contraction and traction of the surrounding interlobular septum when the tumor cells invade and extend outward. In this study, according to the shape and size of the air cavity appearing inside the nodule, the air cavity was classified into three types: vacuole, cavity, and cavum. A bubble small than 5 mm was considered to be a vacuole, while a thin-walled air cavity with a diameter ≥5 mm was considered to be a cavity. The cavum was the thick-walled air cavity. Pulmonary disease included emphysema, bullae, and ventilation-perfusion imbalance. Air bronchogram types were classified into bronchial nondeformation, bronchial deformation, and adjacent bronchiectasis. Pleural contact types included direct contact and pleural traction by tags with indentation or not. A pleural tag was defined as a line or lines extending from a nodule to the pleural surface due to thickening of the interlobular septum (21). Mean diameter was measured with the average of the long and short diameters. To avoid areas of blood vessels, calcification, or cystic degeneration, we delineated the region of interest (ROI) on the highest visible attenuation of lesions and measured the mean of CT attenuation as the CTmean value. All measurements of lesions in this study were performed in the axial plane, and any disagreement in describing semantic features was resolved by a consensus read (Figure 2).
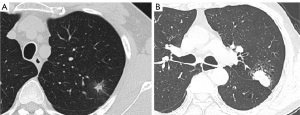
Training and validation of the prediction model
The included patients were divided into a training group and validation group via random10-fold cross-validation. The data of the in the LNM group and N-LNM group were then analyzed and compared to ascertain if there were any significant differences. Combined Model 1 was developed based on factors that were significantly different in the results of univariate analysis. Combined Model 2 included all the independent risk factors from multivariate analysis, and the imaging predictors among the independent factors were separately fused into another model, the image predictor model. Each independent imaging factor was also used in an individual model, which was then compared to the three combined models.
Statistical analysis
All statistical analyses were performed using the SPSS version 25.0.0 software (64-bit; IBM Corp., Armonk, NY, USA) and R software version 4.2.2 (The R Foundation for Statistical Computing; https://www.r-project.org). The normality of data was determined using the Kolmogorov-Smirnov test. In the univariate difference analysis, the quantitative data conforming to a normal distribution are expressed as the mean ± standard deviation and were compared using the independent samples t-test; the quantitative data not conforming to a normal distribution are expressed as the median with interquartile range and were compared using the Mann-Whitney test; the Chi-square test and Fisher exact probability test were used to compare the differences of the qualitative data. Multivariate logistic regression was used to adjust the variables with differences in the univariate difference analysis to eliminate confounding factors, the independent risk factors were then screened out, and different prediction models were established. Ten-fold cross-validation was used for model training and validation. The efficacy of each model was evaluated using receiver operating characteristic (ROC) curves, and the area under the curve (AUC) was also calculated. The Delong test was used to compare the differences between the models. A P value of less than 0.05 was considered to indicate statistical significance.
Results
Clinical characteristics of patients in the training group
Among the 799 cases patients in the training group, 58 (7.3%) had pathological LNM (mean age 60.9±9.4 years; age range 41–91 years; 34 females and 24 males), including 56 cases of adenocarcinoma and 2 cases of squamous carcinoma; the remaining 741 cases (92.7%) had N-LNM (mean age 60.7±9.7 years; age range 18–86 years; 476 females and 265 males), including 738 cases of adenocarcinoma and 3 cases of adenosquamous carcinoma. A total of 9,702 lymph nodes were detected in postoperative samples from the 799 patients, including 9,478 (97.7%) negative lymph nodes and 224 (2.3%) cancerous lymph nodes (Figure 3).
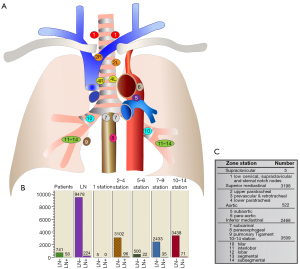
In the univariate difference analysis, there was a significant difference in the distribution of smoking status between the two groups (P=0.021), and it was found to be an independent risk factor for LNM after multivariate logistic regression (P=0.049). There was no significant difference in the distribution of gender, age, surgical history, or clinical basic disease between the LNM group and N-LNM group (P>0.05). The analytical results of clinical data for all patients are summarized in Table 1.
Table 1
Variable | Univariate | Multivariate | ||||||||
---|---|---|---|---|---|---|---|---|---|---|
Training group | Validation group | P value | P value | |||||||
N-LNM | LNM | P value | N-LNM | LNM | P value | |||||
Total cases | 741 (92.7) | 58 (7.3) | 146 (93.6) | 10 (6.4) | ||||||
Gender | 0.391 | 0.458 | >0.99 | NA | ||||||
Female | 476 (64.2) | 34 (58.6) | 92 (63.0) | 8 (80.0) | ||||||
Male | 265 (35.8) | 24 (41.4) | 54 (37.0) | 2 (20.0) | ||||||
Age (years)* | 60.7±9.7 | 60.9±9.4 | 0.878 | 61.1±10.7 | 60.9±9.2 | 0.964 | 0.741 | NA | ||
Smoker | 0.021 | >0.99 | 0.61 | 0.049 | ||||||
No | 662 (89.3) | 46 (79.3) | 127 (87.0) | 9 (90.0) | ||||||
Yes | 79 (10.7) | 12 (20.7) | 19 (13.0) | 1 (10.0) | ||||||
Surgery history | 0.109 | 0.348 | <0.001 | NA | ||||||
No | 542 (73.1) | 48 (82.8) | 74 (50.7) | 3 (30.0) | ||||||
Yes | 199 (26.9) | 10 (17.2) | 72 (49.3) | 7 (70.0) | ||||||
Clinical basic disease | 0.913 | 0.084 | <0.001 | NA | ||||||
No | 454 (61.3) | 36 (62.1) | 79 (54.1) | 4 (40.0) | ||||||
HTN/HLD/DM | 138 (18.6) | 10 (17.2) | 30 (20.5) | 3 (30.0) | ||||||
Other1 | 77 (10.4) | 5 (8.6) | 13 (8.9) | 3 (30.0) | ||||||
Mixed2 | 72 (9.7) | 7 (12.1) | 24 (16.4) | 0 (0.0) |
*, data are the mean ± standard deviation, and the statistical values are the results of the independent samples t-test. Unless otherwise indicated, the data are qualitative variables, the number of patients are outside the parentheses, the percentages are inside the parentheses, and the statistical values are results of the Chi-square test. 1, other clinical basis diseases except HTN, HLD, and DM; 2, two or more of HTN, HLD, or DM and other clinical basic diseases. N-LNM, non-lymph node metastasis; LNM, lymph node metastasis; NA, not applicable; HTN, hypertension; HLD, hyperlipidemia; DM, diabetes.
CT semantic features of patients
In the univariate analysis of CT semantic features, there were differences pulmonary disease, solid component proportion, spiculation, air cavity, air bronchogram type, pleural contact type, mean diameter, and CTmean value between the LNM group and N-LNM group (P<0.05); however, there were no differences in location, shape, or lobulation between the two groups (P>0.05). After adjustment for confounding factors were made via multivariate logistic regression, pulmonary disease (P=0.011), solid component proportion (P<0.001), pleural contact type (P=0.026), and mean diameter (P<0.001) were found to be independent predictors of LNM (Table 2).
Table 2
Variable | Univariate | Multivariate | ||||||||
---|---|---|---|---|---|---|---|---|---|---|
Training group | Validation group | P value | P value | |||||||
N-LNM | LNM | P value | N-LNM | LNM | P value | |||||
Total cases | 741 (92.7) | 58 (7.3) | 146 (93.6) | 10 (6.4) | ||||||
Pulmonary disease | <0.001** | 0.311** | <0.001** | 0.011 | ||||||
No | 544 (73.4) | 46 (79.3) | 110 (75.3) | 6 (60.0) | ||||||
Emphysema/bullae | 57 (7.7) | 9 (15.5) | 18 (12.3) | 2 (20.0) | ||||||
VPI | 118 (15.9) | 0 (0.0) | 12 (8.2) | 1 (10.0) | ||||||
Mixed | 22 (3.0) | 3 (5.2) | 6 (4.1) | 1 (10.0) | ||||||
Solid component proportion | <0.001 | <0.001** | 0.063 | <0.001 | ||||||
≤25% | 442 (59.6) | 6 (10.3) | 72 (49.3) | 0 (0.0) | ||||||
≤50% | 109 (14.7) | 6 (10.3) | 22 (15.1) | 1 (10.0) | ||||||
≤75% | 95 (12.8) | 14 (24.1) | 23 (15.8) | 1 (10.0) | ||||||
<100% | 95 (12.8) | 32 (55.2) | 29 (19.9) | 8 (80.0) | ||||||
Location | 0.260 | 0.846** | 0.044 | NA | ||||||
Right upper lobe | 233 (31.4) | 20 (34.5) | 59 (40.4) | 6 (60.0) | ||||||
Right middle lobe | 64 (8.6) | 4 (6.9) | 13 (8.9) | 0 (0.0) | ||||||
Right lower lobe | 156 (21.1) | 6 (10.3) | 27 (18.5) | 1 (10.0) | ||||||
Left upper lobe | 196 (26.5) | 21 (36.2) | 25 (17.1) | 2 (20.0) | ||||||
Left lower lobe | 92 (12.4) | 7 (12.1) | 22 (15.1) | 1 (10.0) | ||||||
Shape | >0.99** | >0.99** | 0.231 | NA | ||||||
Round | 8 (1.1) | 0 (0.0) | 1 (0.7) | 0 (0.0) | ||||||
Oval | 34 (4.6) | 2 (3.4) | 12 (8.2) | 0 (0.0) | ||||||
Irregular | 699 (94.3) | 56 (96.6) | 133 (91.1) | 10 (100.0) | ||||||
Lobulation | 0.935 | 0.018 | <0.001 | NA | ||||||
No | 118 (15.9) | 9 (15.5) | 79 (54.1) | 1 (10.0) | ||||||
Yes | 623 (84.1) | 49 (84.5) | 67 (45.9) | 9 (90.0) | ||||||
Spiculation | <0.001 | 0.241 | <0.001 | 0.133 | ||||||
No | 397 (53.6) | 16 (27.6) | 118 (80.8) | 6 (60.0) | ||||||
Yes | 344 (46.4) | 42 (72.4) | 28 (19.2) | 4 (40.0) | ||||||
Air cavity | 0.002** | 0.071** | 0.278** | 0.504 | ||||||
No | 573 (77.3) | 38 (65.5) | 107 (73.3) | 5 (50.0) | ||||||
Vacuole | 104 (14.0) | 13 (22.4) | 27 (18.5) | 5 (50.0) | ||||||
Cavity | 62 (8.4) | 4 (6.9) | 12 (8.2) | 0 (0.0) | ||||||
Cavum | 2 (0.3) | 3 (5.2) | 0 (0.0) | 0 (0.0) | ||||||
Air bronchogram type | 0.014** | 0.162** | <0.001 | 0.312 | ||||||
No | 546 (73.7) | 37 (63.8) | 102 (69.9) | 6 (60.0) | ||||||
Without BD | 27 (3.6) | 8 (13.8) | 20 (13.7) | 0 (0.0) | ||||||
With BD | 128 (17.3) | 10 (17.2) | 23 (15.8) | 4 (40.0) | ||||||
Adjacent bronchiectasis | 40 (5.4) | 3 (5.2) | 1 (0.7) | 0 (0.0) | ||||||
Pleural contact type | <0.001 | 0.058** | <0.001 | 0.026 | ||||||
No contact | 172 (23.2) | 6 (10.3) | 35 (24.0) | 0 (0.0) | ||||||
Direct contact | 341 (46.0) | 17 (29.3) | 38 (26.0) | 4 (40.0) | ||||||
Pleural traction without indentation | 130 (17.5) | 5 (8.6) | 32 (21.9) | 1 (10.0) | ||||||
Pleural traction with indentation | 32 (4.3) | 14 (24.1) | 14 (9.6) | 0 (0.0) | ||||||
Direct contact and traction | 66 (8.9) | 16 (27.6) | 27 (18.5) | 5 (50.0) | ||||||
Mean diameter*** | 16.0 (12.0, 20.5) | 23.2 (16.2, 30.6) | <0.001 | 15.0 (11.0, 22.1) | 31.7 (22.4, 37.6) | <0.001 | 0.475 | <0.001 | ||
CTmean value | 19.3±98.3 | 50.6±26.1 | <0.001* | 34.6 (17.4, 60.6) | 32.1 (21.5, 44.6) | 0.764*** | 0.003* | 0.522 |
*, data are the mean ± standard deviation, and the statistical values are the results of the independent samples t-test; **, the statistical values are the results of the Fisher exact probability test; ***, data do not conform to normal distribution, the median are outside parentheses, the lower quartile and the upper quartile are in parentheses, and the statistical values are the results of the Mann-Whitney test. Unless otherwise indicated, the data are qualitative variables, the number of patients are outside the parentheses, the percentages are inside the parentheses, and the statistical values are the results of the Chi-square test. CT, computed tomography; N-LNM, non-lymph node metastasis; LNM, lymph node metastasis; VPI, ventilation-perfusion imbalance; BD, bronchial deformation; CTmean, mean of CT attenuation.
Development and comparison of the prediction models
Combined model 1 was established based on the 9 risk factors that were statistically different in the univariate analysis, while combined model 2 included all independent risk factors. Pulmonary disease, solid component proportion, pleural contact type, and mean diameter were incorporated as CT semantic features among the independent predictors into the third combined model, named the image predictor model. Regarding the performance of the three combined models and individual models (Table 3), in the training group, the combined model 1 yielded an AUC of 0.880 [95% confidence interval (CI): 0.777–0.891], with a sensitivity of 81.2%, a specificity of 78.5%, and an accuracy of 78.7%; combined model 2 had an AUC of 0.876 (95% CI: 0.796–0.892), with a sensitivity of 77.0%, a specificity of 77.6%, and an accuracy of 77.5%; the image predictor model yielded an AUC of 0.868 (95% CI: 0.807–0.889), with a sensitivity of 80.4%, a specificity of 78.4%, and an accuracy of 78.6%. Solid component proportion was the single-feature model with the best ROC among the four individual models, with an AUC of 0.815 (95% CI: 0.734–0.844), and a sensitivity, specificity, and accuracy of 80.9%, 72.7%, and 73.3%, respectively; in the validation group, combined model 1 yielded an AUC of 0.853 (95% CI: 0.777–0.891), and a sensitivity, specificity, and accuracy of 72.9%, 77.8%, and 77.5%, respectively; combined model 2 had an AUC of 0.861 (95% CI: 0.796–0.893), with a sensitivity of 76.0% and a specificity and accuracy of 77.2%; the image predictor model yielded an AUC of 0.857 (95% CI: 0.807–0.888), and the sensitivity, specificity, and accuracy were all 77.6%. The results of the Delong test showed that there was no significant difference in diagnostic efficacy between the three combined models (all P values >0.05), but the solid component proportion had a significantly different performance to those of the three combined models (all P values <0.01). The ROC curves of the seven models in the training and validation groups are displayed in Figure 4.
Table 3
Model type | Threshold | AUC (95% CI) | Sensitivity (%) | Specificity (%) | Accuracy (%) | P value |
---|---|---|---|---|---|---|
Training group | ||||||
Combined model 1 | 0.532 | 0.880 (0.777–0.891) | 81.2 | 78.5 | 78.7 | NA |
Combined model 2 | 0.462 | 0.876 (0.796–0.892) | 77.0 | 77.6 | 77.5 | NA |
Image predictor model | 0.462 | 0.868 (0.807–0.889) | 80.4 | 78.4 | 78.6 | NA |
Pulmonary disease | 0.480 | 0.521 (0.423–0.557) | 76.5 | 26.3 | 29.8 | NA |
Solid component proportion | 0.607 | 0.815 (0.734–0.844) | 80.9 | 72.7 | 73.3 | NA |
Pleural contact type | 0.653 | 0.687 (0.654–0.714) | 60.3 | 66.0 | 65.6 | NA |
Mean diameter | 0.531 | 0.736 (0.725–0.737) | 61.1 | 78.4 | 73.8 | NA |
Validation group | ||||||
Combined model 1 | 0.563 | 0.853 (0.777–0.891) | 72.9 | 77.8 | 77.5 | 0.2334* |
Combined model 2 | 0.554 | 0.861 (0.796–0.893) | 76.0 | 77.2 | 77.2 | 0.5177** |
Image predictor model | 0.575 | 0.857 (0.807–0.888) | 77.6 | 77.6 | 77.6 | 0.7995*** |
Pulmonary disease | 0.799 | 0.521 (0.423–0.557) | 76.4 | 26.3 | 29.8 | 0.0026# |
Solid component proportion | 0.504 | 0.814 (0.788–0.835) | 81.0 | 72.7 | 73.3 | 0.0006# |
Pleural contact type | 0.622 | 0.713 (0.674–0.753) | 59.3 | 66.0 | 65.7 | 0.0032# |
Mean diameter | 0.577 | 0.736 (0.721–0.747) | 63.3 | 74.1 | 73.3 | NA |
*, data are the results of Delong test between combined model 1 and combined model 2; **, data are the results of Delong test between combined model 2 and image predictor model; ***, data are the results of Delong test between image predictors model and combined model 1; #, data are the results of Delong test of solid component proportion and combined model 1, combined model 2, and image predictor model. Combined model 1 was established using 9 factors that were statistically different in the univariate analysis; combined model 2 included all independent risk factors from multivariate analysis; image predictor model involved all independent imaging predictors (pulmonary disease, solid component proportion, pleural contact, and mean diameter). AUC, area under the curve; CI, confidence interval; NA, not applicable.
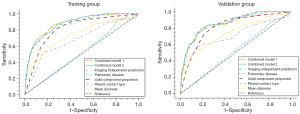
Discussion
In this retrospective study, we analyzed the risk factors of LNM in 955 patients with NSCLC from multiple centers whose disease manifest as PSNs on CT findings. Of all the lymph nodes detected [9,702] in the training group, only 224 (2.3%) were cancerous, indicating a low incidence of LNM in part-solid NSCLC. According to the distribution of the examined lymph nodes in each station, we found that LNM occurred at stations 2–4 (superior mediastinal) with the highest probability, followed by the lung parenchyma, and no LNM occurred in the supraclavicular region in station 1; for N-LNM, the lymph nodes were mainly located in the N1 region, followed by the superior mediastinal region, and few lymph nodes were detected in the supraclavicular region and aortic region.
Regarding CT semantic features, the solid component proportion was an independent risk factor for LNM. The ratio of solid component of nodule to tumor in the LNM group was usually higher than 60% (32/53); therefore, the solid component of nodule was an adverse factor for LNM, which also been confirmed in other studies (22,23). Moreover, the CTmean in the LNM group (50.6±26.1) was significantly higher than that in the N-LNM group (19.3±98.3), and this may be related to the solid component proportion of nodules; there was a positive correlation between the solid component and CT attenuation value, and most nodules in the LNM group had a larger proportion of solid component. Perhaps, this was one of reasons why the mean value of CT lost its significance in multivariate logistic regression. Regarding pleural contact type, a study of 478 patients peripheral solid NSCLC with a largest short diameter >5 mm found that type II pleural involvement (a linear or cord-like pleural tag or tumor abutting to the pleura with a broad base observed on both lung and mediastinal window images) was an independent predictor of occult LNM (24). Similarly, in our study, nodules were more likely to come into direct contact with the pleural than show no contact, both in patients with LNM and N-LNM. However, for patients with LNM, pleural tags were more likely to cause indentation, and the probability of direct contact with the pleural concomitant with pleural tags was also higher than that in patients with N-LNM (27.6% vs. 8.9%). There are abundant lymphatic vessels on the surface of the subpleural lymphatic plexus (25); we can thus infer that when the lesion is in contact with the pleura, the tumor cells first enter the lymphatic vessels, proliferate with lymph fluid in the lymphatic circulation, and enter the lymph node station to complete the invasion.
Emphysema is characterized pathologically by the presence of diffuse chronic inflammation of the lung parenchyma, oxidative stress, and lung destruction (26). A previous study reported (27) emphysema to be an independent risk factor for lung cancer, with a greater severity of emphysema being associated with a higher incidence of lung cancer; our study also examined the correlation between emphysema and LNM and found that as it pertains to pulmonary disease, the patients with LNM were more likely experience concomitant emphysema or bullae than were patients with N-LNM. Some studies have reported (28,29) that a longer mean diameter is an independent risk factor for LNM. DuComb et al. retrospectively studied 332 patients with T1 NSCLC and found that among patients with LNM, the most common pathological type is adenocarcinoma, which is consistent with the results of our study (56/58). In addition, they found that neither tumor diameter nor location was a risk factor for LNM (30). In our study, location was also not found to be a significant factor for metastases, while mean diameter was found to be an independent risk factor for the occurrence of LNM, which is possibly due to the different inclusion and exclusion criteria applied: our study included no restriction on tumor size, while that by DuComb et al. enrolled patients with T1 (8–30 mm).
Research also indicates that model-based schemes can make better utilize radiographic information to predict lymph node diseases (31-33). Das et al. integrated clinical parameters and radiomics features extracted from three ROIs of gross tumor volume (GTV), peritumoral volume (PTV), and LNs using different methods to create a variety of nomograms for predicting preoperative LNM in adenocarcinoma, and the compared the predictive efficacy of each model (34). The results showed that the AUC of radiological features based on GTV, PTV, and LN in the external verification cohort were 0.74,0.72, and 0.64, respectively, while the AUC of the integrated GTV and PTV (GPTV) was 0.75 in the external validation cohort. GPTV combined with LN yielded an AUC of 0.76, and the strongest predictive power was achieved by the integrated nomogram of clinical parameters and CT radiomics information from GTV, PTV, and LN, with an AUC of 0.79 (95% CI: 0.66–0.93). Our study evaluated the predictive ability of three combined models in identifying LNM. The results showed that compared with combined model 1 (including 9 risk factors) and combined model 2 (including all independent factors), the image predictor model incorporating pulmonary disease, solid component proportion, pleural contact type, and mean diameter had the highest diagnostic accuracy in validation group; moreover, its efficacy was better than that of any of the single-feature models. The image predictor model only incorporated the CT semantic features among the significant independent factors identified via multivariate logistic regression; this model included fewer fusion factors but demonstrated higher predictive ability, which may have profound implications: CT semantic features have a good ability to predict LNM in patients with NSCLC and can provide preoperative guidance for clinical practice. Due to the poor image quality caused by respiratory movement and heartbeat, the prediction of LNM is limited in lung magnetic resonance imaging (35). Positron emission tomography (PET) is another imaging method for mediastinal staging, but its high cost, relatively low rate of lymph node involvement, and high false-negative rate have hinder its routine implementation (36). Martinez-Zayas et al. conducted a multicenter prospective validation of two retrospectively developed diagnostic models, called HAL and HOMER. In their study, they included 1,799 patients with NSCLC who underwent endobronchial ultrasound-guided transbronchial fine needle aspiration staging and PET-CT scans. HOMER was used to predict N0, N1, or N2–3 (three classifier), while HAL was used to predict N2/3 or N0/1 (two classifier). Their results showed that HAL and HOMER had good multicenter discrimination: HAL had an AUC of 0.873, while HOMER yielded an AUC of 0.837 for predicting N1–3 and AUC of 0.876 for predicting N2–3 (37).
Several potential limitations of this study merit comment. (I) Given the retrospective design of this study, potential selection bias may hinder the comparability and reproducibility of the results. (II) In the evaluation of CT image features, this study mainly focused on the characteristics of nodules. The image evaluation of lymph nodes may be considered in subsequent research for more in-depth exploration. (III) As we analyzed image features based on subjective description and measurement, there might have been inconsistency in the models related to the semantic features. In addition, these models were only preliminarily evaluated and validated in this study, and the performance of the models will be further improved and externally validated in future research. The development of radiomics has made it possible to transform images into image quantitative feature data, and this may allow for tumor characteristics to be more objectively and quantitatively described. Therefore, studies examining the prognostic prediction and survival analysis of patients with LNM via radiomics are anticipated.
Conclusions
Smoking history, pulmonary disease, solid component proportion, pleural contact type, and mean diameter were found to be independent risk factors for LNM in patients with NSLCLC consisting of PSNs. Furthermore, the image predictor model proposed in this study demonstrated encouraging diagnostic efficacy for LNM, indicating that CT semantic features can guide clinical practice to some extent before operation and has promising application value.
Acknowledgments
Funding: This study was supported by
Footnote
Reporting Checklist: The authors have completed the STARD reporting checklist. Available at https://qims.amegroups.com/article/view/10.21037/qims-23-1631/rc
Conflicts of Interest: All authors have completed the ICMJE uniform disclosure form (available at https://qims.amegroups.com/article/view/10.21037/qims-23-1631/coif). The authors report that this study was supported by the Medical Health Science and Technology Project of Zhejiang Province (Nos. 2023KY1321 and 2022KY702), the Key Research and Development Program of Anhui Province (No. 2022e07020033). The authors have no other conflicts of interest to declare.
Ethical Statement: The authors are accountable for all aspects of the work in ensuring that questions related to the accuracy or integrity of any part of the work are appropriately investigated and resolved. This retrospective study was conducted in accordance with the Declaration of Helsinki (as revised in 2013) and was approved by the respective ethics committees of Tongde Hospital of Zhejiang Province (approval No. 2022-029-JY), Taizhou Municipal Hospital (approval No. LWYJ2023059), and The First Affiliated Hospital of Bengbu Medical University (approval No. 2021-292). The requirement for individual informed consent for this retrospective analysis was waived.
Open Access Statement: This is an Open Access article distributed in accordance with the Creative Commons Attribution-NonCommercial-NoDerivs 4.0 International License (CC BY-NC-ND 4.0), which permits the non-commercial replication and distribution of the article with the strict proviso that no changes or edits are made and the original work is properly cited (including links to both the formal publication through the relevant DOI and the license). See: https://creativecommons.org/licenses/by-nc-nd/4.0/.
References
- Sung H, Ferlay J, Siegel RL, Laversanne M, Soerjomataram I, Jemal A, Bray F. Global Cancer Statistics 2020: GLOBOCAN Estimates of Incidence and Mortality Worldwide for 36 Cancers in 185 Countries. CA Cancer J Clin 2021;71:209-49. [Crossref] [PubMed]
- Bray F, Ferlay J, Soerjomataram I, Siegel RL, Torre LA, Jemal A. Global cancer statistics 2018: GLOBOCAN estimates of incidence and mortality worldwide for 36 cancers in 185 countries. CA Cancer J Clin 2018;68:394-424. [Crossref] [PubMed]
- Morgensztern D, Ng SH, Gao F, Govindan R. Trends in stage distribution for patients with non-small cell lung cancer: a National Cancer Database survey. J Thorac Oncol 2010;5:29-33. [Crossref] [PubMed]
- Siegel RL, Giaquinto AN, Jemal A. Cancer statistics, 2024. CA Cancer J Clin 2024;74:12-49. [Crossref] [PubMed]
- Tang WF, Wu M, Bao H, Xu Y, Lin JS, Liang Y, et al. Timing and Origins of Local and Distant Metastases in Lung Cancer. J Thorac Oncol 2021;16:1136-48. [Crossref] [PubMed]
- Asamura H, Chansky K, Crowley J, Goldstraw P, Rusch VW, Vansteenkiste JF, Watanabe H, Wu YL, Zielinski M, Ball D, Rami-Porta R; International Association for the Study of Lung Cancer Staging and Prognostic Factors Committee, Advisory Board Members, and Participating Institutions. The International Association for the Study of Lung Cancer Lung Cancer Staging Project: Proposals for the Revision of the N Descriptors in the Forthcoming 8th Edition of the TNM Classification for Lung Cancer. J Thorac Oncol 2015;10:1675-84.
- Rami-Porta R, Asamura H, Travis WD, Rusch VW. Lung cancer - major changes in the American Joint Committee on Cancer eighth edition cancer staging manual. CA Cancer J Clin 2017;67:138-55.
- Watanabe S, Asamura H. Lymph node dissection for lung cancer: significance, strategy, and technique. J Thorac Oncol 2009;4:652-7.
- Zhong WZ, Liu SY, Wu YL. Numbers or Stations: From Systematic Sampling to Individualized Lymph Node Dissection in Non-Small-Cell Lung Cancer. J Clin Oncol 2017;35:1143-5.
- de Koning HJ, van der Aalst CM, de Jong PA, Scholten ET, Nackaerts K, Heuvelmans MA, et al. Reduced Lung-Cancer Mortality with Volume CT Screening in a Randomized Trial. N Engl J Med 2020;382:503-13. [Crossref] [PubMed]
- Aberle DR, Adams AM, Berg CD, Black WC, Clapp JD, Fagerstrom RM, Gareen IF, Gatsonis C, Marcus PM, Sicks JD. Reduced lung-cancer mortality with low-dose computed tomographic screening. N Engl J Med 2011;365:395-409. [Crossref] [PubMed]
- Wu G, Woodruff HC, Shen J, Refaee T, Sanduleanu S, Ibrahim A, Leijenaar RTH, Wang R, Xiong J, Bian J, Wu J, Lambin P. Diagnosis of Invasive Lung Adenocarcinoma Based on Chest CT Radiomic Features of Part-Solid Pulmonary Nodules: A Multicenter Study. Radiology 2020;297:451-8. [Crossref] [PubMed]
- Yanagawa M, Tsubamoto M, Satoh Y, Hata A, Miyata T, Yoshida Y, Kikuchi N, Kurakami H, Tomiyama N. Lung Adenocarcinoma at CT with 0.25-mm Section Thickness and a 2048 Matrix: High-Spatial-Resolution Imaging for Predicting Invasiveness. Radiology 2020;297:462-71. [Crossref] [PubMed]
- Bankier AA, MacMahon H, Goo JM, Rubin GD, Schaefer-Prokop CM, Naidich DP. Recommendations for Measuring Pulmonary Nodules at CT: A Statement from the Fleischner Society. Radiology 2017;285:584-600. [Crossref] [PubMed]
- Tournoy KG, Annema JT, Krasnik M, Herth FJ, van Meerbeeck JP. Endoscopic and endobronchial ultrasonography according to the proposed lymph node map definition in the seventh edition of the tumor, node, metastasis classification for lung cancer. J Thorac Oncol 2009;4:1576-84.
- Visser MPJ, van Grimbergen I, Hölters J, Barendregt WB, Vermeer LC, Vreuls W, Janssen J. Performance insights of endobronchial ultrasonography (EBUS) and mediastinoscopy for mediastinal lymph node staging in lung cancer. Lung Cancer 2021;156:122-8. [Crossref] [PubMed]
- El-Sherief AH, Lau CT, Carter BW, Wu CC. Staging Lung Cancer: Regional Lymph Node Classification. Radiol Clin North Am 2018;56:399-409. [Crossref] [PubMed]
- Ye B, Cheng M, Li W, Ge XX, Geng JF, Feng J, Yang Y, Hu DZ. Predictive factors for lymph node metastasis in clinical stage IA lung adenocarcinoma. Ann Thorac Surg 2014;98:217-23. [Crossref] [PubMed]
- Yang G, Nie P, Zhao L, Guo J, Xue W, Yan L, Cui J, Wang Z. 2D and 3D texture analysis to predict lymphovascular invasion in lung adenocarcinoma. Eur J Radiol 2020;129:109111. [Crossref] [PubMed]
- Zwirewich CV, Vedal S, Miller RR, Müller NL. Solitary pulmonary nodule: high-resolution CT and radiologic-pathologic correlation. Radiology 1991;179:469-76. [Crossref] [PubMed]
- Hsu JS, Han IT, Tsai TH, Lin SF, Jaw TS, Liu GC, Chou SH, Chong IW, Chen CY. Pleural Tags on CT Scans to Predict Visceral Pleural Invasion of Non-Small Cell Lung Cancer That Does Not Abut the Pleura. Radiology 2016;279:590-6. [Crossref] [PubMed]
- Li W, Zhou F, Wan Z, Li M, Zhang Y, Bao X, Zhang L, Shi J. Clinicopathologic features and lymph node metastatic characteristics in patients with adenocarcinoma manifesting as part-solid nodule exceeding 3 cm in diameter. Lung Cancer 2019;136:37-44. [Crossref] [PubMed]
- Cho JY, Leem CS, Kim Y, Kim ES, Lee SH, Lee YJ, Park JS, Cho YJ, Lee JH, Lee CT, Yoon HI. Solid part size is an important predictor of nodal metastasis in lung cancer with a subsolid tumor. BMC Pulm Med 2018;18:151. [Crossref] [PubMed]
- He XQ, Luo TY, Li X, Huo JW, Gong JW, Li Q. Clinicopathological and computed tomographic features associated with occult lymph node metastasis in patients with peripheral solid non-small cell lung cancer. Eur J Radiol 2021;144:109981. [Crossref] [PubMed]
- Lee E, Biko DM, Sherk W, Masch WR, Ladino-Torres M, Agarwal PP. Understanding Lymphatic Anatomy and Abnormalities at Imaging. Radiographics 2022;42:487-505. [Crossref] [PubMed]
- Yang X, Wisselink HJ, Vliegenthart R, Heuvelmans MA, Groen HJM, Vonder M, Dorrius MD, de Bock GH. Association between Chest CT-defined Emphysema and Lung Cancer: A Systematic Review and Meta-Analysis. Radiology 2022;304:322-30. [Crossref] [PubMed]
- Hunsaker AR. Emphysema as a Predictor of Lung Cancer: Implications for Lung Cancer Screening. Radiology 2022;304:331-2. [Crossref] [PubMed]
- Wang L, Jiang W, Zhan C, Shi Y, Zhang Y, Lin Z, Yuan Y, Wang Q. Lymph node metastasis in clinical stage IA peripheral lung cancer. Lung Cancer 2015;90:41-6. [Crossref] [PubMed]
- Zhong Y, Yuan M, Zhang T, Zhang YD, Li H, Yu TF. Radiomics Approach to Prediction of Occult Mediastinal Lymph Node Metastasis of Lung Adenocarcinoma. AJR Am J Roentgenol 2018;211:109-13. [Crossref] [PubMed]
- DuComb EA, Tonelli BA, Tuo Y, Cole BF, Mori V, Bates JHT, Washko GR, San José Estépar R, Kinsey CM. Evidence for Expanding Invasive Mediastinal Staging for Peripheral T1 Lung Tumors. Chest 2020;158:2192-9. [Crossref] [PubMed]
- Verdial FC, Madtes DK, Hwang B, Mulligan MS, Odem-Davis K, Waworuntu R, Wood DE, Farjah F. Prediction Model for Nodal Disease Among Patients With Non-Small Cell Lung Cancer. Ann Thorac Surg 2019;107:1600-6. [Crossref] [PubMed]
- Guang Y, Wan F, He W, Zhang W, Gan C, Dong P, Zhang H, Zhang Y. A model for predicting lymph node metastasis of thyroid carcinoma: a multimodality convolutional neural network study. Quant Imaging Med Surg 2023;13:8370-82. [Crossref] [PubMed]
- Feng M, Zhao Y, Chen J, Zhao T, Mei J, Fan Y, Lin Z, Yao J, Bu H. A deep learning model for lymph node metastasis prediction based on digital histopathological images of primary endometrial cancer. Quant Imaging Med Surg 2023;13:1899-913. [Crossref] [PubMed]
- Das SK, Fang KW, Xu L, Li B, Zhang X, Yang HF. Integrative nomogram of intratumoral, peritumoral, and lymph node radiomic features for prediction of lymph node metastasis in cT1N0M0 lung adenocarcinomas. Sci Rep 2021;11:10829. [Crossref] [PubMed]
- Peerlings J, Troost EG, Nelemans PJ, Cobben DC, de Boer JC, Hoffmann AL, Beets-Tan RG. The Diagnostic Value of MR Imaging in Determining the Lymph Node Status of Patients with Non-Small Cell Lung Cancer: A Meta-Analysis. Radiology 2016;281:86-98. [Crossref] [PubMed]
- Zhong Y, She Y, Deng J, Chen S, Wang T, Yang M, Ma M, Song Y, Qi H, Wang Y, Shi J, Wu C, Xie D, Chen CMulti-omics Classifier for Pulmonary Nodules (MISSION) Collaborative Group. Deep Learning for Prediction of N2 Metastasis and Survival for Clinical Stage I Non-Small Cell Lung Cancer. Radiology 2022;302:200-11. [Crossref] [PubMed]
- Martinez-Zayas G, Almeida FA, Yarmus L, Steinfort D, Lazarus DR, Simoff MJ, et al. Predicting Lymph Node Metastasis in Non-small Cell Lung Cancer: Prospective External and Temporal Validation of the HAL and HOMER Models. Chest 2021;160:1108-20. [Crossref] [PubMed]