Research into super-resolution in medical imaging from 2000 to 2023: bibliometric analysis and visualization
Introduction
Super-resolution (SR) refers to algorithms that enhance the resolution of low-resolution (LR) images, resulting in high-resolution (HR) images (1). The technology has been extensively applied across a variety of contexts, including for enhancing the spatial resolution of hyperspectral images in satellite and aerial imaging (2), enabling nanoscale optical capture for image enhancement (3), and enhancing image quality and rectifying image artifacts in medical image processing (4). The available hardware-based methods to produce HR images from LR ones typically involve enhancing pixel density through pixel size reduction or sensor size enlargement. In general, image device hardware upgrades tend to be expensive. Hence, improvements are typically achieved through algorithms, such as SR algorithms.
Various medical imaging devices based on different imaging principles have been invented to more intuitively depict the condition of human organs. The advancement and implementation of SR concepts necessitate increased refinement in medical imaging. Additionally, higher resolution enables the early detection of abnormalities and improves the accuracy of assessing the size and morphology of organs and pathologies. During the research process, the majority of studies tend to prioritize postprocessing methods over hardware enhancements as a means to improving resolution. These efforts are motivated by the potential of using SR algorithms to enhance resolution.
Bibliometric analysis is a scientific quantitative research method that employs techniques such as co-word and cluster analysis to organize publications. Its purpose is to summarize the advancements in a given field of research and identify focal points and emerging trends and to use quantitative statistics to evaluate the contributions of authors, journals, institutions, and countries (5). Furthermore, VOSviewer (http://www.vosviewer.com) can be used to visually represent the co-occurrence of keywords and researchers through a network map (6). Recently, there have been multiple bibliometric studies in the fields of medicine and biomedicine that have investigated the existing literature.
Although several reviews on SR in the medical imaging with different focuses have been published (4,7-11), there is still a lack of a comprehensive analysis and visual assessment for the evolution and trends in SR in medical imaging. In this study, we employed bibliometric analysis to characterize the current state of research in SR in medical imaging and examine the related trends. Our objective was to present the current research status and the five primary research directions concerning medical image SR via keyword co-occurrence analysis, identify the research hotspots and future development prospects within this field through keyword heatmaps, and employ bibliometric analysis methods to support newcomers and researchers in selecting their research trajectory and cultivating innovative ideas. This analysis used a publication dataset obtained from the Web of Science Core Collection (WoSCC) database which spanned from 2000 to 2023. The dataset includes comprehensive information from publications, and can be examined via various search tags. Subsequently, we conducted an analysis to characterize the research status and future trends in the field of SR in medical imaging using an array of statistical data obtained through bibliometric methods.
Methods
Data source
The WoSCC database comprises more than 9,000 esteemed research journals worldwide, incorporating numerous features suitable for bibliometric analysis. The data in our analysis corresponded to field tags of the WoSCC: the publication’s information is categorized in terms of AU (author), SO (source), and PY (publication year) tags; the keyword information is categorized in terms of AK (author keywords) and KP (keywords plus) tags; the topic is obtained through the TS (topic) tag; and the abstract is retrieved using the AB (abstract) tag.
Retrieval strategy
A search for publications in the WoSCC database was performed on October 12, 2023, with the following search strategy shown (available in Figure 1): TS= (“super-resolution” OR “super-resolution” OR “superresolution”) AND TS= (“medic*” OR “clinic*” OR “disease” OR “diagnos*” OR “radiology” OR “magnetic resonance imag*” OR “MRI” OR “computed tomography” OR “CT” OR “X-ray” OR “PET” OR “Ultrasound” OR “Ultrason*” OR “digital radiography” OR “computed radiography” OR “Digital Subtraction Angiograph”) NOT WC= (“Astronomy & Astrophysics”). The publication dates spanned from January 1, 2000, to October 11, 2023, and exclusively encompassed full-length papers (Document Type=[article]) written in English. To avoid retrieving papers from the fields of astronomy and astrophysics, as there is a prevalence of X-ray applications in the astronomy and astrophysics, we excluded them from the search results using the Web of Science Categories (WC). All publication data, including title, publication year, author, country/region, affiliation, source, author keywords, and abstract, were extracted from the WoSCC as a TXT file. To optimize retrieval effectiveness, all data searches and downloads were conducted on October 12, 2023.
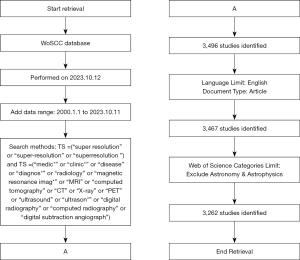
Data analysis and visualization software
The use of bibliometrics was used to elucidate the present state, future possibilities, and knowledge framework of SR in medical imaging. Bibliometric analysis usually involves building bibliometric maps and visually displaying them. Co-occurrence information presents the frequency of bibliographic co-occurrences and enables hierarchical clustering based on these co-occurrences. In this study, the clusters were visualized via graphs.
Bibliometrics are valuable in tracking the progression of trends of particularly influential publications. Bibliometric visualization software is widely used for the extraction and analysis of publication data and for creation of knowledge maps. In this study, VOSviewer (version 1.6.19) was used to conduct the co-occurrence, coauthorship, and network analysis. We also drew maps of the coauthorship and author keyword networks and created an overlay visualization map (6).
Specifically, we conducted an analysis of the top 100 keywords in terms of frequency to perform co-occurrence analysis and identify the hotspots in the field of SR in medical imaging. The co-occurrence network was visualized via VOSviewer, with each node being represented as a labelled circle, the size of the circle correlating with the frequency of the co-occurring items, the color denoting the cluster assignment, and the length and thickness of the node links indicating the correlation and strength of connections, respectively. Our visualization presents the 1,000 most influential connections between nodes.
Results
Annual worldwide publications on SR in medical imaging
Based on our specific search strategy of the WoSCC database, a total of 3262 publications from 2000 to 2023 were identified for inclusion. Figure 2 illustrates the quantity change of annual publications in SR in medical imaging. Over the past two decades, interest in this field has significantly increased, with annual worldwide publications rising from 13 in 2000 to 366 in 2023. The annual publication output for SR in medical imaging was lower than 100 from 2000 to 2012, but steadily increased from 102 to 182 between 2013 and 2017. Surprisingly, from 2018 to 2023, the figure rose above 200, peaking at 437 in 2022.
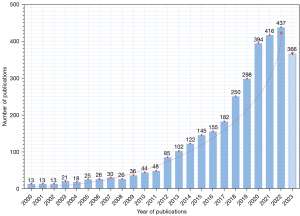
Journals distribution and the 10 most-cited articles
A total of 3,262 articles on SR in medical imaging were published across 1,057 different journals. The top 20 most productive journals in this field accounted for 25.05% (817/3,262) of all publications, as listed in Table 1. Scientific Reports demonstrated the highest level of productivity compared to the other journals, with 86 publications, while NeuroImage received the highest number of citations, totaling 4,678, and was followed by IEEE Transactions on Medical Imaging and IEEE Transactions on Ultrasonics, Ferroelectrics and Frequency Control, which published 74 and 56 articles, respectively.
Table 1
Rank | Journal | WoS category | Impact factor | Publication proportion | Citation, n |
---|---|---|---|---|---|
1 | Scientific Reports | Multidisciplinary sciences | 4.6 | 86 (2.64%) | 1,802 |
2 | IEEE Transactions on Medical Imaging | Radiology, nuclear medicine & medical imaging | 10.6 | 74 (2.27%) | 3,319 |
3 | IEEE Transactions on Ultrasonics Ferroelectrics and Frequency Control | Acoustics | 3.6 | 56 (1.72%) | 1,448 |
4 | Magnetic Resonance in Medicine | Radiology, nuclear medicine & medical imaging | 3.3 | 53 (1.62%) | 1,368 |
5 | IEEE Access | Computer science, information systems | 3.9 | 51 (1.56%) | 575 |
6 | NeuroImage | Radiology, nuclear medicine & medical imaging | 5.7 | 48 (1.47%) | 4,678 |
7 | Medical Physics | Radiology, nuclear medicine & medical imaging | 3.8 | 45 (1.38%) | 636 |
8 | Nature Communications | Multidisciplinary sciences | 16.6 | 44 (1.35%) | 1,872 |
9 | Physics in Medicine and Biology | Radiology, nuclear medicine & medical imaging | 3.5 | 41 (1.26%) | 865 |
10 | Proceedings of the National Academy of Sciences of the United States of America | Multidisciplinary sciences | 11.1 | 39 (1.20%) | 2,163 |
11 | Medical Image Analysis | Radiology, nuclear medicine & medical imaging | 10.9 | 36 (1.10%) | 1,367 |
12 | PLOS One | Multidisciplinary sciences | 3.7 | 36 (1.10%) | 1,291 |
13 | Optics Express | Optics | 3.8 | 31 (0.95%) | 836 |
14 | Biomedical Signal Processing and Control | Engineering, biomedical | 5.1 | 29 (0.89%) | 128 |
15 | Computer Methods and Programs in Biomedicine | Computer science, theory & methods | 6.1 | 28 (0.86%) | 301 |
16 | Nuclear Instruments & Methods in Physics Research Section A: Accelerators Spectrometers Detectors and Associated Equipment | Physics, nuclear | 1.4 | 27 (0.83%) | 505 |
17 | Sensors | Engineering, electrical & electronic | 3.9 | 26 (0.80%) | 271 |
18 | Multimedia Tools and Applications | Computer science, theory & methods | 3.6 | 24 (0.74%) | 108 |
19 | Magnetic Resonance Imaging | Radiology, nuclear medicine & medical imaging | 2.5 | 23 (0.71%) | 536 |
20 | ACS Nano | Chemistry, physical | 17.1 | 20 (0.61%) | 614 |
WoS, Web of Science.
The articles received a total of 77,180 citations, with a median of 24 citations. Table 2 displays the 10 most frequently cited articles, with the citation number ranging from 305 to 1,383 citations. The most cited article was “Robust determination of the fibre orientation distribution in diffusion MRI: non-negativity constrained super-resolved spherical deconvolution” (12) (1,383 citations), which was published in NeuroImage in 2007.
Table 2
Rank | Title | Source | Total citations | PY |
---|---|---|---|---|
1 | Robust determination of the fibre orientation distribution in diffusion MRI: non-negativity constrained super-resolved spherical deconvolution (12) | NeuroImage | 1,383 | 2007 |
2 | Ultrafast ultrasound localization microscopy for deep super-resolution vascular imaging (13) | Nature | 698 | 2015 |
3 | Lewy pathology in Parkinson’s disease consists of crowded organelles and lipid membranes (14) | Nature Neuroscience | 444 | 2019 |
4 | Resolving crossing fibres using constrained spherical deconvolution: Validation using diffusion-weighted imaging phantom data (15) | NeuroImage | 428 | 2008 |
5 | Super-resolution: a comprehensive survey (1) | Machine Vision and Applications | 408 | 2014 |
6 | Anatomically constrained neural networks (ACNNs): Application to cardiac image enhancement and segmentation (16) | IEEE Transactions on Medical Imaging | 377 | 2018 |
7 | Convolutional neural networks for inverse problems in imaging: A review (17) | IEEE Signal Processing Magazine | 364 | 2017 |
8 | Super-resolution microscopy reveals that mammalian mitochondrial nucleoids have a uniform size and frequently contain a single copy of mtDNA (18) | Proceedings of the National Academy of Sciences of the United States of America | 348 | 2011 |
9 | Super-resolution in medical imaging (7) | Computer Journal | 320 | 2009 |
10 | Medical image synthesis with deep convolutional adversarial networks (19) | IEEE Transactions on Biomedical Engineering | 305 | 2018 |
PY, publication year.
Coauthorship status of countries/regions
SR in medical imaging has garnered worldwide interest, with 81 countries/regions devoting to research to this subject in total, as demonstrated in Figure 3. Table 3 presents the top 10 countries with the highest productivity based on their national scientific research strength, with the United States leading with 1,017 publications and 31,301 citations, followed by China with 867 publications and 14,134 citations, and England with 326 publications and 9,753 citations. VOSviewer was used to conduct coauthorship analysis to characterize the international collaboration in this field. The resulting network, as shown in Figure 3, included 75 out of 81 countries or regions, categorized into three distinct clusters, each represented by a different color. The red cluster is the largest, containing 32 countries, and is centered around England, France, Germany, and Italy. The United States has extensive collaboration with numerous countries (n=54), wields significant scientific research influence in this field, and is followed by England (n=53), China (n=47), France (n=44), and Germany (n=43).
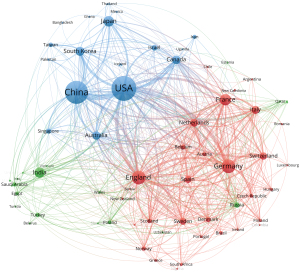
Table 3
Rank | Countries | Publications, n | Citations, n |
---|---|---|---|
1 | USA | 1,017 | 31,301 |
2 | China | 867 | 14,134 |
3 | England | 326 | 9,753 |
4 | Germany | 318 | 9,376 |
5 | France | 215 | 7,559 |
6 | Japan | 188 | 2,844 |
7 | India | 179 | 2,543 |
8 | South Korea | 144 | 3,953 |
9 | Canada | 125 | 3,849 |
10 | Australia | 119 | 5,661 |
Coauthorship status of institutions
Our analysis indicated that 3,562 institutions published research on SR in medical imaging. Table 4 presents the rankings of the top 10 institutions based on their productivity. The Chinese Academy of Sciences published the highest number of articles, with 124 publications and 2,758 citations, followed by King’s College London (58 publications and 2,034 citations), and Harvard Medical School (57 publications and 1,426 citations). After institutions that published fewer than 14 articles were eliminated, 100 institutions remained. VOSviewer was used to conduct coauthorship analysis for these 100 institutions. Figure 4 illustrates the network of institutions, depicting 100 institutions divided into 6 clusters, each represented by a distinct color. The largest cluster, highlighted in red, comprises 27 institutions focused on the Chinese Academy of Sciences, the University of Chinese Academy of Sciences, and Shanghai Jiao Tong University. In terms of the number of partners, Harvard Medical School has the highest count, followed by the University of Oxford, the Chinese Academy of Sciences and the University College London, with 39, 37, 32, 29 partners, respectively.
Table 4
Rank | Institutions | Country | Publications, n | Citations, n |
---|---|---|---|---|
1 | Chinese Academy of Sciences | China | 124 | 2,758 |
2 | King’s College London | England | 58 | 2,034 |
3 | Harvard Medical School | USA | 57 | 1,426 |
4 | University College London | England | 50 | 1,259 |
5 | Stanford University | USA | 47 | 1,507 |
6 | University of Illinois | USA | 47 | 1,326 |
7 | Imperial College London | England | 45 | 1,490 |
8 | University of Oxford | England | 44 | 1,943 |
9 | University of Cambridge | England | 43 | 1,616 |
10 | University of Chinese Academy of Sciences | China | 40 | 556 |
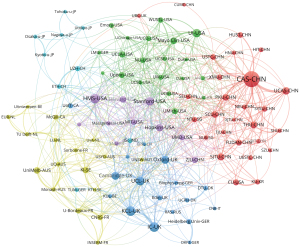
Coauthorship status of authors
Our analysis revealed that 17,026 authors made 20,905 contributions to publish the 3,262 retrieved articles, with an average of 6.4 authors contributing per article in total. The top 20 most productive authors, with Dinggang Shen (20 publications, 671 citations) ranked first, Pengfei Song (19 publications, 499 citations) ranked second, and Clemens F. Kaminski (15 publications, 773 citations) ranked third, are presented in Table 5. In this study, coauthorship analysis was conducted through VOSviewer, with a cutoff of four articles published by the authors being used. Out of the 17,026 authors, 367 met the criterion, and Figure 5 presents the author’s coauthorship network. The coauthorship network assigned 129 authors into 15 groups, each distinguished by a different color. The largest red group encompassed 16 authors and centered around Joshua D. Trzasko, Chengwu Huang, and Wei Zhang, with 17, 14, and 14, and 10 cooperating partners, respectively.
Table 5
Rank | Author | Publications, n | Citations, n | Average citations |
---|---|---|---|---|
1 | Shen, Dinggang | 20 | 671 | 34 |
2 | Song, Pengfei | 19 | 499 | 26 |
3 | Kaminski, Clemens F. | 15 | 773 | 52 |
4 | Chen, Shigao | 14 | 467 | 33 |
5 | Tang, Meng-Xing | 14 | 696 | 50 |
6 | Liu, Xin | 13 | 296 | 23 |
7 | Tanter, Mickael | 13 | 1,087 | 84 |
8 | Lowerison, Matthew R. | 12 | 174 | 15 |
9 | Zheng, Hairong | 12 | 129 | 11 |
10 | Chen, Zhong | 12 | 453 | 38 |
11 | Dunsby, Christopher | 12 | 627 | 52 |
12 | Huang, Chengwu | 11 | 249 | 23 |
13 | Cai, Congbo | 11 | 309 | 28 |
14 | Eckersley, Robert J. | 11 | 722 | 66 |
15 | Christensen-Jeffries, Kirsten | 11 | 684 | 62 |
16 | Eldar, Yonina C. | 11 | 520 | 47 |
17 | Schierle, Gabriele S. Kaminski | 10 | 677 | 68 |
18 | Sauer, Markus | 10 | 254 | 25 |
19 | Klenerman, David | 10 | 579 | 58 |
20 | Chen, Lin | 10 | 229 | 23 |
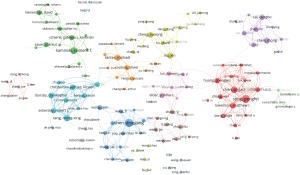
Co-occurrence status of the top 100 most frequent keywords
Keywords serve to represent the fundamental theme of the article. Meanwhile, co-occurrence analysis is employed to accurately represent the essence of the research field using high-frequency keywords. VOSviewer was used to extract all the keywords from the research field and then placed them in the top 100 most distinct groups, as delineated in Table 6. Figure 6 depicts a visual network graph illustrating the top 100 most frequent keywords, categorized into 5 groups according to their co-occurrence relationships. The node size reflects the frequency of keyword occurrence, while the connection between two nodes represents their co-occurrence relationship. At the center of the visual network graph are the most frequently occurring keywords, including super-resolution (697 citations), deep learning (DL) (205 citations), magnetic resonance imaging (MRI) (197 citations), convolutional neural network (CNN) (116 citations), and generative adversarial networks (GANs) (92 citations). VOSviewer was further used to classify keywords into five categories according to their connection strength. In Figure 6, these five clusters are indicated by the colors red, green, blue, yellow, and purple. The following provides explanations of titles categorized by the keywords within the clusters.
Table 6
Cluster | Keyword | Counts | Rank |
---|---|---|---|
1 | Super-resolution | 697 | 1 |
1 | Deep learning | 205 | 2 |
1 | MRI | 197 | 3 |
1 | Convolutional neural network | 116 | 4 |
1 | Generative adversarial networks | 92 | 5 |
1 | Super-resolution reconstruction | 53 | 8 |
1 | Medical imaging | 49 | 11 |
1 | CT | 44 | 12 |
1 | Compressed sensing | 36 | 13 |
1 | Sparse representation | 33 | 19 |
1 | Image segmentation | 30 | 23 |
1 | Image enhancement | 25 | 27 |
1 | Reconstruction | 25 | 28 |
1 | Machine learning | 24 | 30 |
1 | Image processing | 20 | 34 |
1 | Interpolation | 19 | 36 |
1 | COVID-19 | 18 | 37 |
1 | Attention mechanism | 17 | 40 |
1 | Image registration | 17 | 42 |
1 | Optical coherence tomography | 16 | 46 |
1 | Resolution enhancement | 16 | 47 |
1 | Denoising | 15 | 48 |
1 | Image quality | 15 | 51 |
1 | Transfer learning | 15 | 54 |
1 | Artificial intelligence | 13 | 59 |
1 | Breast cancer | 12 | 63 |
1 | Single image super-resolution | 12 | 67 |
1 | Image fusion | 11 | 70 |
1 | Spatiotemporal encoding | 10 | 83 |
1 | Classification | 9 | 85 |
1 | Residual learning | 9 | 88 |
1 | Residual network | 9 | 89 |
1 | Transformer | 9 | 91 |
2 | Image reconstruction | 89 | 6 |
2 | Image resolution | 31 | 21 |
2 | PET | 31 | 22 |
2 | Training | 25 | 29 |
2 | Dictionary learning | 20 | 32 |
2 | Feature extraction | 20 | 33 |
2 | Motion correction | 18 | 39 |
2 | Image restoration | 17 | 43 |
2 | Inverse problems | 17 | 44 |
2 | Three-dimensional displays | 17 | 45 |
2 | Neural network | 14 | 58 |
2 | Image denoising | 13 | 61 |
2 | Medical image processing | 12 | 66 |
2 | Task analysis | 11 | 75 |
2 | Diseases | 10 | 79 |
2 | Medical diagnostic imaging | 10 | 80 |
2 | Generators | 9 | 86 |
2 | Convolution | 8 | 94 |
2 | Noise reduction | 8 | 97 |
2 | Unsupervised learning | 8 | 100 |
3 | Ultrasound imaging | 51 | 9 |
3 | Imaging | 50 | 10 |
3 | Ultrasound | 36 | 15 |
3 | Ultrasound localization microscopy | 35 | 17 |
3 | Microbubbles | 32 | 20 |
3 | Microscopy | 30 | 24 |
3 | Spatial resolution | 27 | 26 |
3 | Super-resolution ultrasound | 20 | 35 |
3 | Fluorescence | 18 | 38 |
3 | Biomedical imaging | 17 | 41 |
3 | Microvasculature | 15 | 52 |
3 | Resolution | 15 | 53 |
3 | Deconvolution | 14 | 55 |
3 | Cancer | 11 | 69 |
3 | Location awareness | 11 | 71 |
3 | Contrast-enhanced ultrasound | 10 | 78 |
3 | Optical imaging | 10 | 81 |
3 | Acoustics | 9 | 84 |
3 | Modulation transfer function | 9 | 87 |
3 | Transducers | 8 | 99 |
4 | Super-resolution microscopy | 89 | 7 |
4 | STED | 36 | 14 |
4 | Alzheimer's disease | 35 | 16 |
4 | Structured illumination microscopy | 30 | 25 |
4 | Fluorescence microscopy | 15 | 50 |
4 | Mitochondria | 14 | 57 |
4 | DSTORM | 13 | 60 |
4 | Electron microscopy | 12 | 64 |
4 | Synapse | 12 | 68 |
4 | Nanoparticles | 11 | 72 |
4 | Neurodegeneration | 11 | 73 |
4 | Protein aggregation | 11 | 74 |
4 | Alpha-synuclein | 10 | 77 |
4 | Parkinson's disease | 10 | 82 |
4 | Tau | 9 | 90 |
4 | Confocal microscopy | 8 | 93 |
4 | Expansion microscopy | 8 | 95 |
4 | Super-resolution fluorescence microscopy | 8 | 98 |
5 | Diffusion MRI | 34 | 18 |
5 | Brain | 23 | 31 |
5 | DTI | 15 | 49 |
5 | High resolution | 14 | 56 |
5 | Tractography | 13 | 62 |
5 | Fetal MRI | 12 | 65 |
5 | Track density imaging | 11 | 76 |
5 | 3D reconstruction | 8 | 92 |
5 | Neuroimaging | 8 | 96 |
MRI, magnetic resonance imaging; CT, computed tomography; COVID-19, coronavirus disease 2019; PET, positron emission tomography; STED, stimulated emission depletion microscopy; DSTORM, direct stochastic optical reconstruction microscopy; DTI, diffusion tensor imaging; 3D, three-dimensional.
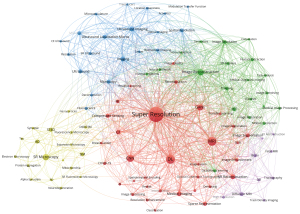
Cluster 1 in red
SR technology plays a crucial role in enhancing the quality and accuracy of medical images by using advanced techniques such as DL, CNN, and GANs. This technology is widely applied in MRI and computed tomography (CT) imaging for tasks such as image enhancement, segmentation, and reconstruction. Moreover, methods such as compressive sensing, sparse representation, machine learning, and transformation learning have been increasingly used in low-dose CT scans and rapid MR scans. Additionally, SR technology has been implemented in super-resolution (SISR) and three-dimensional super-resolution (3DSR). Noteworthy advancements have been made during the coronavirus disease 2019 (COVID-19) through attention mechanisms and image registration techniques in SR reconstruction and optical coherence tomography (20). Meanwhile, the integration of artificial intelligence (AI) into the process of SR reconstruction of chest X-rays has resulted in the enhanced classification accuracy of COVID-19 (21). In essence, this cluster focuses on the integration of SR and AI for improving medical image.
Cluster 2 in green
SR holds significance in medical image processing. Addressing inverse problems involves using dictionary learning and regularization methods to accomplish tasks such as image denoising, restoration, and motion correction. The advancement of image morphology analysis and DL techniques enables enhancement of the positron emission tomography (PET) image resolution affected by hardware constraints through inverse problem processing concepts. In essence, this cluster emphasizes enhancing the quality of PET images through the integration of SR and inverse problem processing concepts.
Cluster 3 in blue
The use of SR in ultrasound has garnered considerable interest, particularly in ultrasound localization microscopy and SR ultrasound. This technology leverages microbubbles as contrast agents to enable the characterization of minute structures such as microvasculature. Through deconvolution operations on ultrasound images to enhance resolution, SR not only aids in early disease detection, such as that of cancer, but also elevates the utility of ultrasound imaging in the realm of biomedical imaging. Moreover, contrast-enhanced ultrasound and fluorescence microscopy facilitate multimodal imaging and comprehensive data acquisition. The fusion of ultrasound and optical imaging not only advances photoacoustic technology but also extends to acoustics and modulation transfer function. By optimizing ultrasound transducers and enhancing image quality, SR technology boosts image spatial resolution, thereby supporting the expansion of ultrasound imaging technology applications. To conclude, this cluster focuses on using ultrasound microbubble methods for achieving SR.
Cluster 4 in yellow
SR plays a pivotal role in microscopy imaging, notably in SR microscopy. Advancements in structure illumination microscopy (SIM) and stimulated emission depletion microscopy (STED) have enabled the HR imaging of microstructures such as protein aggregation and synapses. Techniques such as SR fluorescence microscopy and direct stochastic optical reconstruction microscopy (DSTORM) leverage fluorescence microscopy for the precise imaging of cellular substructures such as mitochondria. In neurodegenerative diseases, including Alzheimer disease (AD) and Parkinson disease (PD), SR facilitates the exploration of intracellular changes, such as protein aggregation, neuronal synapses, and Tau protein accumulation in neurons, aiding in the study of pathological mechanisms and treatment pathways in neurodegenerative diseases. Moreover, the integration of electron microscopy and expansion microscopy is becoming increasingly prominent. By investigating the distribution and functionality of nanoparticles within cells, SR can advance the study of life sciences and neurodegenerative disease treatment and mechanisms. In essence, this cluster is focused on the use of SR microscopy in addressing two distinct diseases.
Cluster 5 in purple
The implementation of SR in diffusion MRI and brain neuroimaging is being increasingly appreciated. The integration of brain diffusion tensor imaging (DTI), tractography, and track density imaging with SR can reveal the intricate structure and connectivity of brain neural pathways, facilitating a comprehensive examination of neural function and structure. In fetal MRI, SR effectively addresses motion artifacts. The near continuous innovation in this field suggests the vast potential of SR in advancing neuroimaging research and propelling the development of neuroscience. In summary, this cluster focuses on how SR functional magnetic resonance imaging (fMRI) of the brain provides rapid acquisition and enhances precision imaging.
Figure 7 illustrates how VOSviewer was used to color code the keywords extracted from the publications based on the average appearing year (AAY) via a heatmap for the analysis of trend progression. Keywords with cooler hues signify earlier occurrences, whereas keywords with warmer hues signify later occurrences. The most recent hotspot words include transformer (AAY: 2022.8889), attention mechanism (AAY: 2022.2353), medical diagnostic imaging (AAY: 2022.1), feature extraction (AAY: 2022), task analysis (AAY: 2021.9011), COVID-19 (AAY: 2021.8333), location awareness (AAY: 2021.8182), and generative adversarial networks (AAY: 2021.5326).
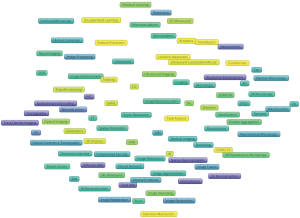
Discussion
Research trends in the field of SR for medical imaging
SR in medical imaging has made significant progress with the development of science and technology. Figure 2 illustrates the temporal evolution of the popularity of SR research, indicating a consistent and substantial growth. The research concerning SR in medical imaging has exhibited a sustained level of excellence over the past 5 years, encompassing 58.6% (1,911/3,262) of the total publications, with a noteworthy emphasis on the significance of AI. Analysis of data from the previous 5 years indicates that the integration of SR with AI has surpasses traditional image processing methods.
Out of the top 20 most productive journals, 7 journals were related to the field of radiology and the field of nuclear medicine and medical imaging, 4 journals were related to multidisciplinary sciences, and 2 journals were related to computer science and information systems. The remaining journals were related to acoustics or chemistry, multidisciplinary engineering, biomedical engineering, electronics or optics, and nuclear physics. This also suggests that SR is a prominent topic in medical imaging.
Over 17,000 authors from 3,562 research institutions across 81 countries published research in SR for medical imaging, aligning with the prevailing trend of comprehensive development. According to Figure 5, Dinggang Shen has been the most prolific author in this field but does not have an extensive collaboration network. Figure 3 illustrates that global collaboration is not constrained by geographical boundaries. The United States, being the most productive country and a central hub of international collaboration, holds a leading position in this field. Among the top 10 most productive institutions, 5 were from England, 3 were from the United States, and the remaining 2 were from China. The institutions of these 3 countries hold a leading position in this field. Figure 4 demonstrates that more than 90% of the institutions contributing to SR in medical imaging were part of a collaborative network. However, collaboration was predominantly between institutions within the same country, with Chinese institutions dominating the red and American institutions dominating the green.
In conclusion, SR has historically been widely used for enhancing medical images. The advent of AI in recent years has introduced novel pathways. Collaborative efforts among leading countries and institutions from the United States, China, and England have been instrumental in achieving advancements in this field.
Examining the state of the art through clustering analysis
According to co-occurrence analysis of the top 100 most frequent keywords, five predominant research directions in focusing on SR in medical imaging were identified (Figure 6), which enabled us to classify the primary knowledge structures and discern research hotspots. In the co-citation analysis presented in Table 6, the top 10 most cited references are categorized within one of the five clusters. In the subsequent sections, we examine the different directions in SR research, drawing from articles obtained through keywords from various clusters.
Cluster 1: SR and AI for medical image enhancement
SR is extensively used in numerous medical imaging modalities, including CT and MRI, due to its capability to enhance LR images into HR ones. The accurate diagnosis of subtle lesions necessitates the extraction of intricate details from HR medical images. Setting aside specific diseases, we focused on summarizing the articles regarding the use of SR with AI for medical image enhancement. The analysis revealed that achieving HR medical images encompasses two dimensions: SISR and 3DSR.
SR in HR CT images from two dimensions
In the context of SISR, the presence of foggy edges and unreadable textures in conventional CT images often hinders the assessment of radiologists or clinicians (22). The integration of different diagnostic results pertaining to CT image characteristic can be challenging, with it being difficult to establish coherent mapping. To address this issue, a transformer model is commonly employed, leveraging the combined training of natural and medical images to attain SISR (23).
Regarding 3DSR, the level of anatomical detail and image segmentation accuracy are typically associated with meeting surgical demands (24). However, CT images are acquired with a certain slice thickness, resulting in anisotropic resolution. The partial volume effects caused by the slice thickness further hinder the accurate segmentation of small structures relative to the pixel size (25). Conversely, the anisotropic resolution along the z-axis direction impacts the precision of 3D segmentation. In response to these challenges, multiple approaches have been proposed, such as Super Resolution Generative Adversarial Networks (SRGAN) (26).
SR in HR MRI from two dimensions
In the context of SISR, various factors, including the quality of the MRI device, noise, and low image resolution can negatively impact the quality of MRI images. However, these issues can be mitigated through the application of SR algorithms (27). Traditional methods that rely on interpolation techniques for enhancing the resolution of MRI images tend to compromise the accuracy of the subsequent diagnostic processes (28). Therefore, the advent of DL has revitalized the field of magnetic resonance SR reconstruction technology (29). By leveraging the capacity of deep CNNs (DCNNs) to identify nonlinear relationships, we can enhance the resolution of isotropic pixels in MRI images along the X–Y plane (30). However, pre-processing is often necessary to align the original image with the parameter requirements of the CNN (31), yet it diminishes the correlation of feature information across convolutional layers, thus resulting in suboptimal SR outcomes (32). To overcome these challenges, residual networks have been widely employed.
Regarding 3DSR, thin-layer, as opposed to thick-layer, MRI offers superior interlayer information and enhances the accuracy of structural relationships. Nonetheless, acquiring thin layers tends to be time-consuming and challenging in practical scanning scenarios (33). In order to address these challenges, several reconstruction frameworks have been proposed to reconstruct thin-layer MRI images in both the transverse or sagittal planes. These frameworks have used 3D U-net, GANs, and 3D CNNs (34).
SR medical image enhancement based on imaging principles
Compressed sensing and sparse encoding are frequently discussed in the field of SR. The use of these algorithms in CT indicates their potential to attain the precise reconstructions of imaged objects, and the management a low-dose in CT acquisition through the use of compressed sensing techniques is also promising (35). Compressed sensing may further facilitate high spatial resolution in MRI while reducing scanning time. However, to avoid any loss of fine analytical details, edges, and boundaries resulting from energy leakage and artifacts, the integration of GANs is required (36,37).
Cluster 2: SR and inverse problem processing concepts for PET image processing
Cluster 2 (Figure 6, green cluster) includes 20 high-frequency keywords, such as PET, inverse problems, image reconstruction, image restoration, and neural network.
In this cluster, the majority of keywords related to the concept of inverse problem processing within the domain of medical image processing. The inverse problem is a term commonly used to describe the ill-posed problem in image processing. Examples of typical ill-posed problems in image processing include image denoising, image restoration, and image SR. These types of problems are typically addressed using regularization methods, sparse representation methods such as dictionary learning, and statistical methods. In recent years, the use of image morphological component analysis and DL methods has become prevalent. Given the imaging principles and hardware limitations of PET, the inverse problem processing concept has been broadly applied, particular as it concerns SR in PET image processing.
Use of SR in overcoming hardware limitations
In terms of hardware, the resolution of PET is primarily constrained by the physical size of the crystals or the inherent spatial resolution of the detector (38). From the perspective of imaging principles, the image quality is affected by both the administered dose of 18F-fluorodeoxyglucose and the duration of the acquisition time (39). The costly equipment and dosage constraints together limit the achievable image resolution.
In achieving SR images, hardware upgrades can be avoided by leveraging the spatial location information extracted from the MR images of the input image patches. To account for the spatially variant nature of the blur kernels, this information is subsequently integrated into the CNN as additional inputs (40). Another approach involves combining consecutive acquisitions with an iterative algorithm (41).
Use of SR and PET imaging principles to mitigate radiation exposure
One approach for reducing radiation exposure is obtaining the SR images involved in predicting the standard PET images through the use of multiple MRI sequences as inputs (42). Furthermore, the use of the SRPET-Net allows for the learning of the intricacies and structural attributes of PET images across varying doses, facilitating effective transformation (43).
Sparse representation plays a significant role in PET SR in two distinct respects, as demonstrated by cluster 1. In this method, two dictionaries are extracted from raw PET images, each with different resolutions, and these are subsequently trained separately as part of the optimization process for enhancing image resolution (44). Furthermore, the application of dictionary learning methods can also extend to dose parameters in PET (45).
Cluster 3: SR ultrasound through use of microbubbles
Cluster 3 (Figure 6, blue cluster) includes 20 high frequency keywords, such as ultrasound, ultrasound localization microscopy, microscopy, microbubbles, optical imaging, fluorescence, and cancer.
In ultrasound imaging, aside from hardware conditions, image resolution is primarily limited by wavelength, depth, diffraction phenomena, and acoustic scattering. To address this issue, ultrasonic microbubble technology has emerged. Within this context, the primary application involves acquiring SR ultrasound images using ultrasound microbubble methods (46), while ultrasound localization microscopy is commonly employed for visualizing microbubbles.
Ultrasound microbubble methods for SR
Ultrasonic microbubbles typically consist of a gas-liquid mixture and produce echo signals in the ultrasonic field to improve the contrast and resolution of ultrasound images (13). This technology allows for the observation of fine structures and details at the micrometer level, as well as deep penetration without causing damage to the organizational structure. Its advantages, including high resolution, deep penetration, safety, and real-time capabilities, have made it a widely applied technology in non-invasive biological imaging research and in clinic. In ultrasound vascular imaging, the implementation of ultrafast frame rate algorithms in ultrasound imaging enables the capturing of instantaneous signals from ultrasound microbubbles, leading to accurate extraction of blood flow velocity in the plane. The advancement of this technology has introduced a novel diagnostic approach for those diseases linked to microvascular blood flow, such as stroke and arteriosclerosis (47).
The infusion of microbubble contrast agent into the entire microvascular network requires a specific duration of time. To enhance the visualization of blood vessels, it is necessary to individually locate and track microbubbles for achieving SR. The use of low microbubble concentrations is necessary and provides acquisitions that can last several minutes. However, the current conventional processing pipelines face difficulties in handling the interference caused by multiple nearby microbubbles, which in turn further decreases the concentrations (48).
Integration of DL for optimizing ultrasound microbubble methods
The collected radio frequency (RF) signals of microbubbles often overlap, and deep ultrasound localization microscopy (Deep-ULM) helps clarify their nonlinear image characteristics. This approach provides an improvement over the traditional method mentioned above (49). Additionally, in contrast to the aforementioned methods, CNNs have the ability to directly deconvolute a single ultrasonic RF channel signal, thereby achieving SR (50). Furthermore, the beamformer can accomplish SR at high frame rates by using RF signals as inputs and training them with enhanced networks, including GoogLeNet and U-Net (51).
Algorithm-enhanced ultrasound localization microscopy
Standard ultrasound localization microscopy can be enhanced through the integration of algorithms that incorporate sparsity in a transform domain. For instance, the fast iterative shrinkage-thresholding algorithm (FISTA algorithm, despite requiring numerous iterations for convergence, can extend the capabilities of ultrasound localization microscopy (ULM) (52). Similarly, the Kalman tracking framework, which offer benefits comparable to those of sparsity-based deconvolution techniques, can also serve as an extension to ultrasound localization microscopy (53).
Cluster 4: SR microscopy for AD and PDs
Cluster 4 (Figure 6, yellow cluster) includes 18 high-frequency keywords, such as AD, PD, DSTORM, electron microscopy, structured illumination microscopy, STED, alpha-synuclein, and neurodegeneration.
AD and PD are two neurodegenerative disorders frequently characterized by protein misfolding, disorder, and the formation of insoluble amyloid protein structures. Optical imaging, particularly optical SR imaging, can be used to observe the dynamic processes of biomarkers at the molecular level, which traditional imaging techniques are unable to capture, and SR microscopy has begun to emerge to address this issue.
Diagnostic biomarkers for AD and PD
Amyloid-beta peptides (Aβ) and tau protein aggregates are two biomarkers that can serve as neurological indicators of AD (54). The genetic characteristics of sporadic AD are primarily influenced by variations in the cholesterol transporter apolipoprotein E (apoE) (55).
In PD, both mitochondrial function and morphology are affected, with there being a clear relationship between mitochondrial function and morphology (56). The interaction between alpha-synuclein and mitochondria plays a crucial role in the degeneration observed in various types of PD (57). Studies have been conducted using microscopy-based methods to investigate mitochondrial morphological changes and the interaction with alpha-synuclein.
The limitations and advancements in SR microscopy
Electron microscopy initially observed the aforementioned biomarkers in the research on AD and PD, however, despite the higher resolution it offers, it frequently generates artifacts and distortions during the imaging process, while the field of view and depth are significantly constrained (58).
To address these issues, confocal microscopy, STED, SIM, SR fluorescence microscopy, DSTORM, and expansion microscopy were successively developed and used in the research of AD and PD.
Cluster 5: SR in brain fMRI for rapid acquisition and precise imaging
Cluster 5 (Figure 6, purple cluster) includes 9 high-frequency keywords, including diffusion MRI, DTI, tractography, track density imaging, neuroimaging, and brain, all of which are related to fMRI. Currently, the use of diffusion-weighted imaging (DWI) and DTI is pervasive. Shortening scanning duration to alleviate patient discomfort may compromise image resolution, but the integration of SR with DL effectively addresses this issue.
SR and DL for DWI and DTI
DWI is a well-established technique employed to investigate both the functionality and structure of the white matter. This method commonly involves the use of fiber reconstruction algorithms in combination with tractography (59). DWI allows for the assessment of axonal density in specific regions of the white matter (60). Shortening the scanning time during an actual scan reduces the signal-to-noise ratio of the image, making it impossible to capture HR images with accurate anatomical details. DTI is a data-driven analytical approach that uses DWI data to compute the orientation and connection patterns of fiber bundles. DTI facilitates the depiction of fiber bundle distribution in a variety brain regions and structures. Both techniques are often synergistically employed to acquire a more comprehensive understanding of structural features and connectivity networks.
Therefore, SR has assumed a pivotal role in DWI and tractography imaging. The emergence of DL has led to the development and application of a new approach for enhancing DWI. One example of this is the q-Space DL (q-DL) method, which effectively reduces diffusion gradients while maintaining image quality to fulfil anatomical requirements (61). Building upon this, multimodal SR-q-DL has been proposed for HR tissue microstructure estimation (62). However, the majority of existing methodologies primarily concentrate on deterministic models. Additionally, various approaches have been suggested to accurately characterize uncertain components and accomplish SR diffusion MRI (63).
Algorithms for mitigating fetal MRI artifacts and achieving SR
Fetal brain development encompasses the two crucial aspects of volume growth and structural formation, the observation of which can be facilitated by fetal MRI. Fetal MRI is typically conducted using uterine scans, but these are often affected by motion artifacts, such as breathing, thus reducing image quality.
Despite the widespread use of single-shot RF pulse sequences, artifacts can still affect the interlayer anatomical information, leading to deformation and distortion. The application of interlayer SR can effectively address these issues (64). The use of faster echo planar imaging (EPI) sequences is not feasible due to the geometric distortion induced by the main B0 field, which disrupts the data consistency on which diffusion and functional MRI rely (65). Interslice motion results in significant motion artifacts that are visible in out-of-plane views. Acquiring slices sequentially does not allow for the representation of a 3D volume (66). Slice-to-volume registration (SVR) method can effectively resolve motion artifacts during MR scanning and obtain HR images (67). However, traditional registration and correction methods are incapable of resolving significant motion artifacts during fetal scanning. An emerging method “Affinity Fusion-Based Framework for Iteratively Random Motion Correction” (AFFIRM) that uses iterative fusion algorithms may offer a solution for random motion artifacts (68). Additionally, a stable SR algorithm with a single parameter and CNN can be employed to first complete registration and SR and then segment the entire brain (69).
Emerging frontiers in SR for medical imaging
Figure 7 illustrates the hotspots in the field of SR for medical imaging that have emerged within the past 5 years, including DL, GANs, attention mechanisms, medical diagnostic imaging, task analysis, transfer learning, feature extraction, location awareness, and 3D displays. With the rapid advancement of computing power, SR has transitioned from traditional algorithms to AI methods, such as CNN and GANs. These approaches not only enhance the quality of medical images but also provide valuable references for diagnosis through techniques such as feature extraction. Additionally, the use of GANs and compressed sensing in SR in medical imaging to facilitate easy and rapid access to medical images represents a new direction within this field.
Limitations
We acknowledge that the bibliometric method has inherent limitations. All publications included in this article are indexed in the WoSCC database. However, despite WoSCC being the most commonly used and authoritative comprehensive database, there is still a possibility that other articles from Scopus, PubMed, or Google Scholar were not included in our analysis. To rectify this limitation, we intend to employ a broader range of search engines to comprehensively retrieve publications in subsequent studies. Another limitation is the absence of quality evaluation of publications, which resulted in assigning equal weight to high-quality and low-quality publications. Additionally, there is a potential issue in accurately extracting authors’ names using VOSviewer. Different name spellings or the usage of multiple names by authors might have led to inaccurate research results for these individuals.
Conclusions
Overall, the yearly publication volume of SR has shown a consistent increase over the past two decades, with the most rapid growth observed in the past five years. This trend suggests that the utilization of SR has gained popularity as the technology has advanced, particularly in hardware and AI implementation.
Our analysis revealed that all the top 20 journals in the field of medical imaging, excluding multidisciplinary journals, highlight the importance and research value of SR. We observed that international cooperation in the field of SR is chiefly spearheaded by the United States, China, Germany, and England, while the collaboration among institutions tends to be more domestic. Countries, regions and institutions cluster exhibit strong tendencies toward international collaboration. The domain of medical imaging research is increasingly emphasizing multicenter and multimodal studies, highlighting the crucial role of potential collaborative relationships in funding and joint projects. Productive authors possess a robust research foundation, conduct coherent studies, and offer valuable research concepts for the scholarly community. The clustering of authors shows that collaborative efforts are centered around the leading researchers, while other researchers have also focused on similar research concepts. Analysis of keyword frequencies and co-occurrence networks confirmed the widespread and effective integration of AI in the field of SR. Notably, our findings align with highly cited articles, reinforcing the significance of the five key research areas identified in our study.
In summary, this analysis provides a comprehensive and robust foundation for researchers to use as a reference for future studies.
Acknowledgments
Funding: None.
Footnote
Conflicts of Interest: All authors have completed the ICMJE uniform disclosure form (available at https://qims.amegroups.com/article/view/10.21037/qims-24-67/coif). The authors have no conflicts of interest to declare.
Ethical Statement: The authors are accountable for all aspects of the work in ensuring that questions related to the accuracy or integrity of any part of the work are appropriately investigated and resolved.
Open Access Statement: This is an Open Access article distributed in accordance with the Creative Commons Attribution-NonCommercial-NoDerivs 4.0 International License (CC BY-NC-ND 4.0), which permits the non-commercial replication and distribution of the article with the strict proviso that no changes or edits are made and the original work is properly cited (including links to both the formal publication through the relevant DOI and the license). See: https://creativecommons.org/licenses/by-nc-nd/4.0/.
References
- Nasrollahi K, Moeslund TB. Super-resolution: A comprehensive survey. Mach Vis Appl 2014;25:1423-68.
- Chen C, Wang Y, Zhang N, Zhang Y, Zhao Z. A Review of Hyperspectral Image Super-Resolution Based on Deep Learning. Remote Sens 2023;15:2853.
- Li H, Song W, Zhao Y, Cao Q, Wen A. Optical Trapping, Sensing, and Imaging by Photonic Nanojets. Photonics 2021;8:434.
- Chen Z, Pawar K, Ekanayake M, Pain C, Zhong S, Egan GF. Deep Learning for Image Enhancement and Correction in Magnetic Resonance Imaging-State-of-the-Art and Challenges. J Digit Imaging 2023;36:204-30. [Crossref] [PubMed]
- Agarwal A, Durairajanayagam D, Tatagari S, Esteves SC, Harlev A, Henkel R, et al. Bibliometrics: tracking research impact by selecting the appropriate metrics. Asian J Androl 2016;18:296-309. [Crossref] [PubMed]
- van Eck NJ, Waltman L. Software survey: VOSviewer, a computer program for bibliometric mapping. Scientometrics 2010;84:523-38. [Crossref] [PubMed]
- Greenspan H. Super-Resolution in Medical Imaging. The Computer Journal 2009;52:43-63.
- Lepcha DC, Goyal B, Dogra A, Goyal V. Image super-resolution: A comprehensive review, recent trends, challenges and applications. Inf Fusion 2023;91:230-60.
- Li Y, Sixou B, Peyrin F. A Review of the Deep Learning Methods for Medical Images Super resolution Problems. IRBM 2021;42:120-33.
- Christensen-Jeffries K, Couture O, Dayton PA, Eldar YC, Hynynen K, Kiessling F, O'Reilly M, Pinton GF, Schmitz G, Tang MX, Tanter M, van Sloun RJG. Super-resolution Ultrasound Imaging. Ultrasound Med Biol 2020;46:865-91. [Crossref] [PubMed]
- Yang H, Wang Z, Liu X, Li C, Xin J, Wang Z. Deep learning in medical image superresolution: a review. Appl Intell 2023;53:20891-916.
- Tournier JD, Calamante F, Connelly A. Robust determination of the fibre orientation distribution in diffusion MRI: non-negativity constrained super-resolved spherical deconvolution. Neuroimage 2007;35:1459-72. [Crossref] [PubMed]
- Errico C, Pierre J, Pezet S, Desailly Y, Lenkei Z, Couture O, Tanter M. Ultrafast ultrasound localization microscopy for deep super-resolution vascular imaging. Nature 2015;527:499-502. [Crossref] [PubMed]
- Shahmoradian SH, Lewis AJ, Genoud C, Hench J, Moors TE, Navarro PP, et al. Lewy pathology in Parkinson's disease consists of crowded organelles and lipid membranes. Nat Neurosci 2019;22:1099-109. [Crossref] [PubMed]
- Tournier JD, Yeh CH, Calamante F, Cho KH, Connelly A, Lin CP. Resolving crossing fibres using constrained spherical deconvolution: validation using diffusion-weighted imaging phantom data. Neuroimage 2008;42:617-25. [Crossref] [PubMed]
- Oktay O, Ferrante E, Kamnitsas K, Heinrich M, Bai W, Caballero J, Cook SA, de Marvao A, Dawes T, O'Regan DP, Kainz B, Glocker B, Rueckert D. Anatomically Constrained Neural Networks (ACNNs): Application to Cardiac Image Enhancement and Segmentation. IEEE Trans Med Imaging 2018;37:384-95. [Crossref] [PubMed]
- McCann MT, Jin KH, Unser M. Convolutional Neural Networks for Inverse Problems in Imaging: A Review. IEEE Signal Processing Magazine 2017;34:85-95. [Crossref] [PubMed]
- Kukat C, Wurm CA, Spåhr H, Falkenberg M, Larsson NG, Jakobs S. Super-resolution microscopy reveals that mammalian mitochondrial nucleoids have a uniform size and frequently contain a single copy of mtDNA. Proc Natl Acad Sci U S A 2011;108:13534-9. [Crossref] [PubMed]
- Nie D, Trullo R, Lian J, Wang L, Petitjean C, Ruan S, Wang Q, Shen D. Medical Image Synthesis with Deep Convolutional Adversarial Networks. IEEE Trans Biomed Eng 2018;65:2720-30. [Crossref] [PubMed]
- Qiu DF, Cheng YH, Wang XS. Residual Dense Attention Networks for COVID-19 Computed Tomography Images Super-resolution. IEEE Transactions on Cognitive and Developmental Systems 2023;15:904-13.
- Sun H, Ren G, Teng X, Song L, Li K, Yang J, Hu X, Zhan Y, Wan SBN, Wong MFE, Chan KK, Tsang HCH, Xu L, Wu TC, Kong FS, Wang YXJ, Qin J, Chan WCL, Ying M, Cai J. Artificial intelligence-assisted multistrategy image enhancement of chest X-rays for COVID-19 classification. Quant Imaging Med Surg 2023;13:394-416. [Crossref] [PubMed]
- Chi J, Sun Z, Wang H, Lyu P, Yu X, Wu C. CT image super-resolution reconstruction based on global hybrid attention. Comput Biol Med 2022;150:106112. [Crossref] [PubMed]
- Han X, Xie Z, Chen Q, Li X, Yang H. Learning the degradation distribution for medical image superresolution via sparse swin transformer. Comput Graph 2023;114:168-78.
- Kim C, Bekar O, Seo H, Park SM, Lee D. Computed tomography vertebral segmentation from multi-vendor scanner data. J Comput Des Eng 2022;9:1650-64.
- van Aarle W, Batenburg KJ, Van Gompel G, Van de Casteele E, Sijbers J. Super-resolution for computed tomography based on discrete tomography. IEEE Trans Image Process 2014;23:1181-93. [Crossref] [PubMed]
- Ge R, Shi F, Chen Y, Tang S, Zhang H, Lou X, Zhao W, Coatrieux G, Gao D, Li S, Mai X. Improving anisotropy resolution of computed tomography and annotation using 3D super-resolution network. Biomed Signal Process Control 2023;82:104590.
- Sert E, Özyurt F, Doğantekin A. A new approach for brain tumor diagnosis system: Single image super resolution based maximum fuzzy entropy segmentation and convolutional neural network. Med Hypotheses 2019;133:109413. [Crossref] [PubMed]
- Li Y, Song B, Guo J, Du X, Guizani M. Super-Resolution of Brain MRI Images Using Overcomplete Dictionaries and Nonlocal Similarity. IEEE Access 2019;7:25897-907.
- Chun J, Zhang H, Gach HM, Olberg S, Mazur T, Green O, Kim T, Kim H, Kim JS, Mutic S, Park JC. MRI super-resolution reconstruction for MRI-guided adaptive radiotherapy using cascaded deep learning: In the presence of limited training data and unknown translation model. Med Phys 2019;46:4148-64. [Crossref] [PubMed]
- Wu Q, Li Y, Sun Y, Zhou Y, Wei H, Yu J, Zhang Y. An Arbitrary Scale Super-Resolution Approach for 3D MR Images via Implicit Neural Representation. IEEE J Biomed Health Inform 2023;27:1004-15. [Crossref] [PubMed]
- Zareapoor M, Jain DK, Yang J. Local spatial information for image super-resolution. Cogn Syst Res 2018;52:49-57.
- Qiu DF, Zheng LX, Zhu JQ, Huang DT. Multiple improved residual networks for medical image super-resolution. Future Gener Comput Syst 2021;116:200-8.
- Li Z, Yu J, Wang Y, Zhou H, Yang H, Qiao Z. DeepVolume: Brain Structure and Spatial Connection-Aware Network for Brain MRI Super-Resolution. IEEE Trans Cybern 2021;51:3441-54. [Crossref] [PubMed]
- Gu J, Li Z, Wang Y, Yang H, Qiao Z, Yu J. Deep Generative Adversarial Networks for Thin-Section Infant Image Reconstruction. IEEE Access 2019;7:68290-304.
- Mojica E, Pertuz S, Arguello H. High-resolution coded-aperture design for compressive X-ray tomography using low resolution detectors. Opt Commun 2017;404:103-9.
- Malczewski K. Magnetic resonance image enhancement using highly sparse input. Magn Reson Imaging 2020;74:1-13. [Crossref] [PubMed]
- Datta S, Dandapat S, Deka B. A deep framework for enhancement of diagnostic information in CSMRI reconstruction. Biomed Signal Process Control 2022;71:103117.
- Qiu K, Du Y, Liu J, Guan JL, Chao H, Diao J. Super-resolution observation of lysosomal dynamics with fluorescent gold nanoparticles. Theranostics 2020;10:6072-81. [Crossref] [PubMed]
- Yoshimura T, Hasegawa A, Kogame S, Magota K, Kimura R, Watanabe S, Hirata K, Sugimori H. Medical Radiation Exposure Reduction in PET via Super-Resolution Deep Learning Model. Diagnostics (Basel) 2022;12:872. [Crossref] [PubMed]
- Song TA, Chowdhury SR, Yang F, Dutta J. Super-Resolution PET Imaging Using Convolutional Neural Networks. IEEE Trans Comput Imaging 2020;6:518-28. [Crossref] [PubMed]
- Kennedy JA, Israel O, Frenkel A, Bar-Shalom R, Azhari H. Super-resolution in PET imaging. IEEE Trans Med Imaging 2006;25:137-47. [Crossref] [PubMed]
- Wang Y, Zhang P, An L, Ma G, Kang J, Shi F, Wu X, Zhou J, Lalush DS, Lin W, Shen D. Predicting standard-dose PET image from low-dose PET and multimodal MR images using mapping-based sparse representation. Phys Med Biol 2016;61:791-812. [Crossref] [PubMed]
- Liu K, Yu H, Zhang M, Zhao L, Wang X, Liu S, Li H, Yang K. A Lightweight Low-dose PET Image Super-resolution Reconstruction Method based on Convolutional Neural Network. Curr Med Imaging 2023;19:1427-35. [Crossref] [PubMed]
- Li T, Jiang C, Gao J, Yang Y, Liang D, Liu X, Zheng H, Hu Z. Low-count PET image restoration using sparse representation. Nucl Instrum Methods Phys Res A 2018;888:222-7.
- Xu Y, Li Z, Zhang X, Fan W, Zhou C, Que D, Yuan J, He Q, Liang D, Liu X, Zheng H, Hu Z, Zhang N. Low-dose PET image denoising based on coupled dictionary learning. Nucl Instrum Methods Phys Res A 2021;1020:165908.
- Huang B, Bates M, Zhuang X. Super-resolution fluorescence microscopy. Annu Rev Biochem 2009;78:993-1016. [Crossref] [PubMed]
- Couture O, Hingot V, Heiles B, Muleki-Seya P, Tanter M. Ultrasound Localization Microscopy and Super-Resolution: A State of the Art. IEEE Trans Ultrason Ferroelectr Freq Control 2018;65:1304-20. [Crossref] [PubMed]
- Milecki L, Poree J, Belgharbi H, Bourquin C, Damseh R, Delafontaine-Martel P, Lesage F, Gasse M, Provost J. A Deep Learning Framework for Spatiotemporal Ultrasound Localization Microscopy. IEEE Trans Med Imaging 2021;40:1428-37. [Crossref] [PubMed]
- van Sloun RJG, Solomon O, Bruce M, Khaing ZZ, Wijkstra H, Eldar YC, Mischi M. Super-Resolution Ultrasound Localization Microscopy Through Deep Learning. IEEE Trans Med Imaging 2021;40:829-39. [Crossref] [PubMed]
- Blanken N, Wolterink JM, Delingette H, Brune C, Versluis M, Lajoinie G. Super-Resolved Microbubble Localization in Single-Channel Ultrasound RF Signals Using Deep Learning. IEEE Trans Med Imaging 2022;41:2532-42. [Crossref] [PubMed]
- Lu JY, Lee PY, Huang CC. Improving Image Quality for Single-Angle Plane Wave Ultrasound Imaging With Convolutional Neural Network Beamformer. IEEE Trans Ultrason Ferroelectr Freq Control 2022;69:1326-36. [Crossref] [PubMed]
- van Sloun RJG, Cohen R, Eldar YC. Deep Learning in Ultrasound Imaging. Proceedings of the IEEE 2020;108:11-29.
- Yan J, Zhang T, Broughton-Venner J, Huang P, Tang MX. Super-Resolution Ultrasound Through Sparsity-Based Deconvolution and Multi-Feature Tracking. IEEE Trans Med Imaging 2022;41:1938-47. [Crossref] [PubMed]
- Zhang WI, Antonios G, Rabano A, Bayer TA, Schneider A, Rizzoli SO. Super-Resolution Microscopy of Cerebrospinal Fluid Biomarkers as a Tool for Alzheimer's Disease Diagnostics. J Alzheimers Dis 2015;46:1007-20. [Crossref] [PubMed]
- Wang H, Kulas JA, Wang C, Holtzman DM, Ferris HA, Hansen SB. Regulation of beta-amyloid production in neurons by astrocyte-derived cholesterol. Proc Natl Acad Sci U S A 2021;118:e2102191118. [Crossref] [PubMed]
- Rocha S, Freitas A, Guimaraes SC, Vitorino R, Aroso M, Gomez-Lazaro M. Biological Implications of Differential Expression of Mitochondrial-Shaping Proteins in Parkinson's Disease. Antioxidants (Basel) 2017;7:1. [Crossref] [PubMed]
- Valdinocci D, Kovarova J, Neuzil J, Pountney DL. Alpha-Synuclein Aggregates Associated with Mitochondria in Tunnelling Nanotubes. Neurotox Res 2021;39:429-43. [Crossref] [PubMed]
- Johansson B, Oasa S, Muntsant Soria A, Tiiman A, Söderberg L, Amandius E, Möller C, Lannfelt L, Terenius L, Giménez-Llort L, Vukojević V. The interwoven fibril-like structure of amyloid-beta plaques in mouse brain tissue visualized using super-resolution STED microscopy. Cell Biosci 2023;13:142. [Crossref] [PubMed]
- Dyrby TB, Lundell H, Burke MW, Reislev NL, Paulson OB, Ptito M, Siebner HR. Interpolation of diffusion weighted imaging datasets. Neuroimage 2014;103:202-13. [Crossref] [PubMed]
- Calamante F, Smith RE, Tournier JD, Raffelt D, Connelly A. Quantification of voxel-wise total fibre density: Investigating the problems associated with track-count mapping. Neuroimage 2015;117:284-93. [Crossref] [PubMed]
- Qin Y, Liu Z, Liu C, Li Y, Zeng X, Ye C. Super-Resolved q-Space deep learning with uncertainty quantification. Med Image Anal 2021;67:101885. [Crossref] [PubMed]
- Qin Y, Li Y, Zhuo Z, Liu Z, Liu Y, Ye C. Multimodal super-resolved q-space deep learning. Med Image Anal 2021;71:102085. [Crossref] [PubMed]
- Tanno R, Worrall DE, Kaden E, Ghosh A, Grussu F, Bizzi A, Sotiropoulos SN, Criminisi A, Alexander DC. Uncertainty modelling in deep learning for safer neuroimage enhancement: Demonstration in diffusion MRI. Neuroimage 2021;225:117366. [Crossref] [PubMed]
- Cordero-Grande L, Ortuno-Fisac JE, Del Hoyo AA, Uus A, Deprez M, Santos A, Hajnal JV, Ledesma-Carbayo MJ. Fetal MRI by Robust Deep Generative Prior Reconstruction and Diffeomorphic Registration. IEEE Trans Med Imaging 2023;42:810-22. [Crossref] [PubMed]
- Kuklisova-Murgasova M, Lockwood Estrin G, Nunes RG, Malik SJ, Rutherford MA, Rueckert D, Hajnal JV. Distortion Correction in Fetal EPI Using Non-Rigid Registration With a Laplacian Constraint. IEEE Trans Med Imaging 2018;37:12-9. [Crossref] [PubMed]
- Gholipour A, Estroff JA, Warfield SK. Robust super-resolution volume reconstruction from slice acquisitions: application to fetal brain MRI. IEEE Trans Med Imaging 2010;29:1739-58. [Crossref] [PubMed]
- Uus AU, Grigorescu I, van Poppel MPM, Steinweg JK, Roberts TA, Rutherford MA, Hajnal JV, Lloyd DFA, Pushparajah K, Deprez M. Automated 3D reconstruction of the fetal thorax in the standard atlas space from motion-corrupted MRI stacks for 21-36 weeks GA range. Med Image Anal 2022;80:102484. [Crossref] [PubMed]
- Shi W, Xu H, Sun C, Sun J, Li Y, Xu X, Zheng T, Zhang Y, Wang G, Wu D. AFFIRM: Affinity Fusion-Based Framework for Iteratively Random Motion Correction of Multi-Slice Fetal Brain MRI. IEEE Trans Med Imaging 2023;42:209-19. [Crossref] [PubMed]
- Ebner M, Wang G, Li W, Aertsen M, Patel PA, Aughwane R, Melbourne A, Doel T, Dymarkowski S, De Coppi P, David AL, Deprest J, Ourselin S, Vercauteren T. An automated framework for localization, segmentation and super-resolution reconstruction of fetal brain MRI. Neuroimage 2020;206:116324. [Crossref] [PubMed]