A nomogram for risk stratification of central cervical lymph node metastasis in patients with papillary thyroid carcinoma
Introduction
Thyroid cancer is the sixth most common cancer among women according to the American Cancer Society’s 2023 American Cancer Data Statistics (1). The incidence of central lymph node metastasis (CLNM, level VI) ranges from 12% to 64% (2,3) when first diagnosed with thyroid cancer. Whether to perform prophylactic central lymph node dissection (CLND) for cN0 thyroid cancer is still controversial (4-6), which might lead to overtreatment.
Ultrasound is still the primary method for imaging evaluation of thyroid cancer (7,8). However, due to the influence of gas in the trachea and esophagus (9,10), ultrasound is significantly limited in the evaluation of the central cervical lymph node (11,12). Moreover, several recent studies (13-19) have indicated that the potential complementary role of computed tomography (CT) in assessing lymph node metastasis. Compared to CT, dual-energy CT (DECT) has many advantages, such as low radiation dose, high image quality, multiparameter imaging, quantitative measurement, and so on (20). On the other hand, Appendix 1 detailed that the use of iodine contrast agents did not affect postoperative radioactive iodine therapy.
In recent years, the use of prediction model has been widely recognized by clinicians, which is recommended in clinical practice guidelines (21) and have been applied to predict lymph node metastasis in patients with colorectal cancer (22), endometrial cancer (23), breast cancer (24), gastric cancer (25), and lung adenocarcinoma (26).
In the current study, we hypothesized that first, combined ultrasound and DECT features of the solitary primary thyroid nodule were potentially associated with CLNM. Second, the prediction model would achieve improved performance and risk stratification. Third, the nomogram could guide the formulation of individual treatment plans. The purpose of this study was first to identify independent risk factors for CLNM combining ultrasound and DECT. Second, to develop and validate the prediction model for CLNM and compare it to the model based on ultrasound only. Third, the risk stratification was further verified through a retrospective cohort study to provide information for the clinical transition from prophylactic CLND to therapeutic CLND. We present this article in accordance with the TRIPOD reporting checklist (available at https://qims.amegroups.com/article/view/10.21037/qims-24-284/rc).
Methods
The study was conducted in accordance with the Declaration of Helsinki (as revised in 2013). The study was approved by institutional ethics boards of Tianjin First Central Hospital (No. 2020N220KY) and Binzhou Medical University Hospital (No. LW-24), and individual consent for this retrospective analysis was waived.
Subjects
A total of 5,773 patients with papillary thyroid carcinoma (PTC) who visited Tianjin First Central Hospital (Hospital A) between 2017 and 2019 were retrospectively analyzed. Patients included in this study met the following criteria: first diagnosed with thyroid cancer, met ultrasound-guided fine-needle aspiration biopsy criteria, underwent thyroid surgery with complete postoperative pathological data, complete ultrasound and DECT data and clear images to make a definite diagnosis. Exclusion criteria included: patients with a history of neck radiotherapy or other tumors; patients considered to have multiple lesions on ultrasound examination; patients confirmed to be medullary thyroid carcinoma, follicular thyroid carcinoma, or anaplastic thyroid carcinoma by postoperative pathology. A total of 525 consecutive patients were enrolled to develop the training cohort and were subjected to internal validation (Figure 1).
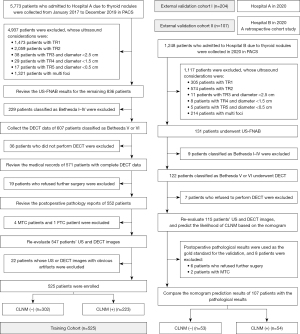
The patients were treated with near-total thyroidectomy or lobectomy accompanied by CLND according to the American Thyroid Association guidelines (27) (see Appendix 2 for the specific principles of operation). The postoperative pathological results were used as the gold standard. In order to ensure the independence of the measured data and the accuracy of the constructed model, all patients included in this study were with a single lesion. The solitary primary thyroid nodule’s ultrasound and DECT parameters were evaluated.
After model development, we used the same criteria to retrospectively analyze medical cases of inpatients who visited Hospital A in 2020 for temporal validation (external validation cohort I). To further validate the prediction model, we conducted a retrospective cohort study of 107 patients admitted to Binzhou Medical University Hospital (Hospital B) in 2020, who were included for geographic validation (external validation cohort II).
Image acquisition
In Hospital A, all data was first scanned with five different Doppler ultrasonic diagnostic apparatuses, then examined by a 64 multidetector row CT scanner (SOMATOM Definition Flash, Siemens Healthcare) using dual-phase contrast-enhanced CT. In Hospital B, the data was scanned with other four different Doppler ultrasonic diagnostic apparatuses. The CT scanner was the same as Hospital A. The specific ultrasound and DECT protocols were detailed in Appendix 3. Ultrasound images parameters from the primary thyroid lesion included: location, diameter, composition, margin, echogenicity, shape, calcification, and ratio of capsular abutment over the lesion perimeter (A/P). In DECT images, we collected the following quantitative parameters from the solitary primary thyroid nodule: iodine concentration (IC) and normalized IC (NIC) of thyroid nodules in the arterial and venous phases. All quantitative parameters were measured independently three times and averaged as results. Measurement methods of related ultrasound and DECT data were introduced in Appendices 4,5 and Figure S1.
Development of the prediction model
The candidate variables with P<0.05 in the univariate analysis were input into the multivariate binary logistic backward stepwise regression analysis to select the independent predictors. A nomogram was constructed based on the results. The specific category description was detailed in Appendix 6.
Additionally, a locally weighted regression (LOESS) curve was drawn according to the total score calculated from the nomogram among the training cohort. Then, based on the inflection point of the LOESS curve to classify all patients into low-risk, intermediate-risk, and high-risk groups.
Validation of the prediction model
The 1,000 bootstrap technique was used for internal validation. Temporal and geographic validation methods were applied for external validation. The nomogram score of the included patients in the external validation cohort II was calculated to predict the probability of CLNM, blinded to the pathological results.
Power calculation
A power calculation was performed to ensure that the external validation cohort was of sufficient size to evaluate the area under the curve (AUC) estimated from the training cohort.
Clinical utility of the prediction model
Decision curve analysis (DCA) was conducted to evaluate the nomogram net benefits with different threshold probabilities in the validation cohorts (28). The predicted probability of CLNM in each patient was calculated based on the nomogram, and risk stratification was performed to assist the clinical decision.
Statistical analysis
All statistical analyses were performed using SPSS 25.0 and R software 4.0.1. R software, OriginPro 9.1, GraphPad Prism 9.0.0, and MedCalc 18.2.1 were used to draw the figures. It was considered that P<0.05 was statistically significant. Multivariate logistic regression was performed to calculate the odds ratio (OR) of the 95% confidence interval (CI) to screen out the independent risk predictors of CLNM. A nomogram was built based on the independent risk predictors. The risk stratification system was established according to the inflection point of the LOESS curve using R software. The performance of the nomogram was estimated using the receiver operating characteristic (ROC) curve and the calibration curve (“rms” package). The DeLong method was used to compare the AUCs of the two constructed models using ultrasound alone and ultrasound combined with DECT. The integrated discrimination improvement index (IDII) and net reclassification index (NRI) were calculated using R software (Predict ABEL package). PASS 15.0 was used to perform the power calculation. DCA was performed by the “dca. R” (decisioncurveanalysis.org).
Results
Patient characteristics
A total of 525 consecutive PTC patients from 2017 to 2019 in Hospital A were included in the training cohort, including 122 males and 403 females. A total of 204 consecutive patients, including 58 males and 146 females in Hospital A in 2020, were collected to form external validation cohort I. Another 107 independent patients, including 38 males and 69 females in Hospital B in 2020, were collected to form external validation cohort II. Baseline information of the training and two external validation cohorts were shown in Table 1 and Tables S1-S3. The distribution of continuous variables in the training cohort was shown in the form of histogram (Figure S2). Heat map of data distribution in the three cohorts was shown in Figure S3. The results of the consistency analysis were shown in Table S4. The cutoff value of each DECT quantitative parameter was displayed in Table S5, and these parameters were converted from continuous variables to categorical variables accordingly.
Table 1
Variables | Training cohort | |||
---|---|---|---|---|
Total (n=525) | CLNM (−) (n=302) | CLNM (+) (n=223) | P | |
Ultrasound | ||||
Diameter† | <0.001¶ | |||
T1a | 322 (61.3) | 220 (72.8) | 102 (45.7) | |
T1b | 152 (29.0) | 62 (20.5) | 90 (40.4) | |
T2 | 44 (8.4) | 19 (6.3) | 25 (11.2) | |
≥ T3 | 7 (1.3) | 1 (0.3) | 6 (2.7) | |
Shape‡ | <0.001⊥ | |||
Wider-than-tall | 317 (60.4) | 202 (66.9) | 115 (51.6) | |
Taller-than-wide | 208 (39.6) | 100 (33.1) | 108 (48.4) | |
Calcification‡ | <0.001⊥ | |||
None or large comet-tail | 207 (39.4) | 192 (63.6) | 15 (6.7) | |
Macrocalcification | 43 (8.2) | 36 (11.9) | 7 (3.1) | |
Rim calcification | 16 (3.0) | 16 (5.3) | 0 | |
Microcalcification | 259 (49.3) | 58 (19.2) | 201 (90.1) | |
A/P§ | <0.001¶ | |||
<25% | 449 (85.5) | 279 (92.4) | 170 (76.2) | |
25–50% | 73 (13.9) | 23 (7.6) | 50 (22.4) | |
>50% | 3 (0.6) | 0 | 3 (1.3) | |
DECT (mg/mL) | ||||
IC IAP | 2.79±0.98 | 2.48±0.93 | 3.21±0.89 | <0.001# |
IC IVP | 3.15±0.96 | 2.87±0.86 | 3.53±0.95 | <0.001# |
†, according to the 8th AJCC staging systems, the diameter was classified into four categories according to the definition of diameter as follows: T1a: ≤1 cm, T1b: 1–2 cm, T2: 2–4 cm, ≥ T3: >4 cm. ‡, refer to American College of Radiology Thyroid Imaging, Reporting, and Data for grouping criteria. §, A/P was graded by values of <25%, 25–50%, or >50%, proven by a previous study. ¶, continuous variables that did not fit to the normal distribution were represented by number (frequency), using the Kolmogorov-Smirnov test. ⊥, categorical variables were represented by number (frequency) using Mann-Whitney U test. #, continuous variables that fitted to the normal distribution were represented by mean ± standard deviation, using the Kolmogorov-Smirnov test. AJCC, American Joint Committee on Cancer; A/P, the ratio of capsular abutment over the lesion perimeter; CLNM, central lymph node metastasis; DECT, dual-energy computed tomography; IAP, in the arterial phase; IC, iodine concentration; IVP, in the venous phase.
Prediction model development
Univariate analysis was performed for each variable in the training cohort. Diameter, shape, calcification, A/P, IC in the arterial phase, IC in the venous phase, NIC in the arterial phase, and NIC in the venous phase were statistically associated with CLNM in PTC patients (Table 2).
Table 2
Variables | Univariate analysis | Multivariate analysis | ||||||
---|---|---|---|---|---|---|---|---|
OR | 95% CI | P | OR | 95% CI | P | |||
Sex | 0.672 | 0.447–1.010 | 0.056 | |||||
Age | 0.879 | 0.573–1.349 | 0.555 | |||||
Location | 1.247 | 0.949–1.640 | 0.114 | |||||
Diameter | 2.156 | 1.652–2.814 | <0.001 | 2.113 | 1.431–3.121 | <0.001 | ![]() |
|
Composition | 1.255 | 0.728–2.164 | 0.413 | |||||
Margin | 1.035 | 1.134–2.324 | 0.823 | |||||
Echogenicity | 0.691 | 0.411–1.161 | 0.163 | |||||
Shape | 1.897 | 1.329–2.707 | <0.001 | 3.802 | 2.248–6.430 | <0.001 | ![]() |
|
Calcification | 2.636 | 2.235–3.109 | <0.001 | 2.898 | 2.366–3.549 | <0.001 | ![]() |
|
A/P | 3.727 | 2.228–6.234 | <0.001 | 2.622 | 1.290–5.330 | 0.008 | ![]() |
|
IC IAP >2.4 mg/mL | 3.738 | 2.595–5.383 | <0.001 | 2.354 | 1.440–3.846 | 0.001 | ![]() |
|
IC IVP >3.2 mg/mL | 3.761 | 2.590–5.461 | <0.001 | 2.352 | 1.428–3.874 | 0.001 | ![]() |
|
NIC IAP >0.21 | 4.797 | 3.068–7.502 | <0.001 | |||||
NIC IVP >0.55 | 3.301 | 2.297–4.743 | <0.001 |
A/P, the ratio of capsular abutment over the lesion perimeter; CI, confidence interval; CLNM, central lymph node metastasis; IAP, in the arterial phase; IC, iodine concentration; IVP, in the venous phase; NIC, normalized iodine concentration; OR, odds ratio; PTC, papillary thyroid carcinoma.
Furthermore, a multivariate binary logistic regression analysis identified that diameter (OR, 2.113; 95% CI: 1.431–3.121; P<0.001), shape (OR, 3.802; 95% CI: 2.248–6.430; P<0.001), calcification (OR, 2.898; 95% CI: 2.366–3.549; P<0.001), A/P (OR, 2.622; 95% CI: 1.290–5.330; P=0.008), IC in the arterial phase (OR 2.354; 95% CI: 1.440–3.846; P=0.001), and IC in the venous phase (OR 2.352; 95% CI: 1.428–3.874; P=0.001) were independent risk predictors of CLNM (Table 2). The Hosmer-Lemeshow test showed that the P value was 0.954, indicating that the model had an increased goodness of fit. The results of multiple linear regression showed that the tolerance of all variables was greater than 0.2 and the variance inflation factor was less than 10, so it is considered that there was no multicollinearity among these predictors (Figure S4 and Table S6) (29).
The above six independent predictors were incorporated to produce the nomogram (Figure 2). It showed good discrimination with an AUC of 0.922 (95% CI: 0.895–0.943) (Table 3 and Figure 3A). The good agreement between the nomogram-estimated probability of CLNM and the actual CLNM rate in the training cohort was showed by the calibration curve, with a mean absolute error of 0.015 (Figure 3B and Table S7).
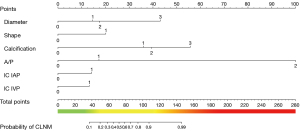
Table 3
Parameters | Training cohort | External validation cohort I | External validation cohort II |
---|---|---|---|
Cutoff value | >0.82 | N/A | N/A |
AUC | 0.922 (0.895–0.943) | 0.912 (0.864–0.947) | 0.861 (0.781–0.920) |
Youden index | 0.7184 | 0.75314 | 0.7002 |
Sensitivity (%) | 84.75 (79.4–89.2) | 86.32 (77.7–92.5) | 88.89 (77.4–95.8) |
Specificity (%) | 87.09 (82.8–90.7) | 88.99 (81.6–94.2) | 81.13 (68.0–90.6) |
PPV (%) | 82.9 (78.2–86.7) | 87.2 (79.9–92.1) | 82.8 (73.2–89.4) |
NPV (%) | 88.6 (85.0–91.4) | 88.2 (81.8–92.5) | 87.7 (76.9–93.9) |
PLR (%) | 6.56 (4.9–8.8) | 7.84 (4.6–13.5) | 4.71 (2.7–8.3) |
NLR (%) | 0.18 (0.1–0.2) | 0.15 (0.09–0.3) | 0.14 (0.06–0.3) |
P | <0.001 | <0.001 | <0.001 |
Data in parentheses are 95% CI. N/A, not applicable; AUC, area under the curve; CI, confidence interval; CLNM, central lymph node metastasis; NLR, negative likelihood ratio; NPV, negative predictive value; PLR, positive likelihood ratio; PPV, positive predictive value; PTC, papillary thyroid carcinoma.
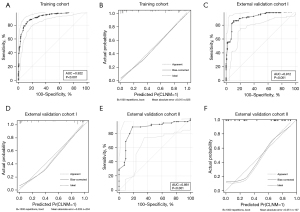
In addition, in the training cohort, the cutoff value of 0.82 was selected to distinguish the presence of CLNM, with a sensitivity of 84.75%, specificity of 87.09%, positive predictive value (PPV) of 82.9%, negative predictive value (NPV) of 88.6%, positive likelihood ratio (PLR) of 6.56, and negative likelihood ratio (NLR) of 0.18 (Table 3).
The Sankey plot showed that the patients in the training cohort had gone through the six risk factors and finally divided into CLNM (−) and CLNM (+) (Figure S5).
Risk stratification system according to the prediction model
A risk stratification system based on the inflection point of the LOESS curve was developed in the training cohort. All patients were divided into low-risk (total points: 0–50), intermediate-risk (total points: 51–100), and high-risk (total points: >100) groups (Figure S6). In the temporal validation cohort, the confusion matrix revealed that the potential utility of CLNM was 9.3%, 34.7%, and 87.9% in the low-risk, intermediate-risk, and high-risk groups, respectively. In the geographic validation cohort, the confusion matrix revealed that the potential utility of CLNM was 17.6%, 28.8%, and 94.7% in the low-risk, intermediate-risk, and high-risk groups, respectively (Figure S7).
Prediction model validation
Good discrimination with an AUC of 0.912 (95% CI: 0.864–0.947) and good calibration with a mean absolute error of 0.033 were both achieved in external validation cohort I (Table 3, Table S7, Figure 3C,3D). A Hosmer-Lemeshow test demonstrated no departure from a good fit, with a P value of 0.829.
In external validation cohort II, the AUC, sensitivity, specificity, PPV, NPV, PLR, and NLR were 0.861 (95% CI: 0.781–0.920), 88.89% (95% CI: 77.4–95.8%), 81.13% (95% CI: 68.0–90.6%), 82.8% (95% CI: 73.2–89.4%), 87.7% (95% CI: 76.9–93.9%), 4.71% (95% CI: 2.7–8.3%), and 0.14% (95% CI: 0.06–0.3%), respectively (Table 3 and Figure 3E). Good calibration was also confirmed, with a mean absolute error of 0.051 (Figure 3F and Table S7).
The predictive performance of the new nomogram was superior to that of the model based on ultrasound only in the external validation cohorts I and II, with AUCs of 0.912 (95% CI: 0.864–0.947) vs. 0.892 (95% CI: 0.841–0.931) and 0.861 (95% CI: 0.781–0.920) vs. 0.741 (95% CI: 0.648–0.821), respectively (Figure 4). In cohort II, the AUC increased by 12%, further demonstrating that DECT complemented ultrasound. Meanwhile, utilization of the DECT parameters improved the predictive value for CLNM in terms of NRI and IDII compared to the prediction model incorporating only the independent ultrasound risk factors (Table S8).
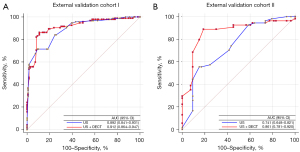
The power calculation
The AUC0 and AUC1 were set at 0.93 and 0.91 according to the AUCs of the training cohort (0.922) and external validation cohorts (0.912 and 0.861). Meanwhile, α was set as 0.05 and false positive rate limited 0.01–0.20. The result showed that the sample size of the validation cohorts needed to be greater than 106 when the target power was 0.90. Therefore, both the sample sizes of the external validation cohorts I and II were sufficient in the current study.
Clinical utility of the prediction model
The decision curve revealed that using nomogram to predict the probability of CLNM in PTC patients would be advantageous if the threshold probability was more significant than 6% (Figure 5).
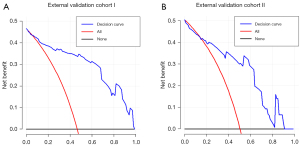
In the external validation cohort II, the patients were scored and risk stratified without knowing the pathological results. Three patients were underestimated in the low-risk group, and two patients were overestimated in the high-risk group (Figure 6, and Figures S8,S9). The possible reasons for the wrong prediction were analyzed in the Discussion section.
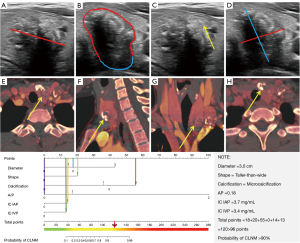
Discussion
There were three significant findings in the current study. First, diameter, shape, calcification, A/P, and IC in the arterial and venous phases were independent risk predictors of CLNM. Second, the new nomogram facilitated the prediction risk of CLNM using a cutoff value of 0.82 (approximately 96 points), with an AUC of 0.922. Based on the LOESS curve, the risk stratification system divided PTC patients into low-risk (0–50 points), intermediate-risk (51–100 points), and high-risk (>100 points) groups. Third, the prediction result of the retrospective cohort study was highly consistent with the three risk groups. These findings might assist in clinical realization of the transition from prophylactic CLND to therapeutic CLND to a greater extent.
A/P was used instead of the extrathyroidal extension to achieve quantitative measurement, consistent with a previous study (30). Diameter was recognized as another independent risk predictor for CLNM, and we considered that the more extensive the PTC lesion, the more aggressive it was (9). Taller-than-wide was an independent risk factor because benign nodules grew parallel to regular tissue planes, whereas malignant nodules grew across normal tissue planes (31,32). Microcalcification appeared as hyperechoic spots ≤1 mm in diameter on ultrasound image and can be named as psammoma bodies (PBs) histologically. Some studies (33-37) have shown that bone morphogenetic protein (BMP)-1 was overexpressed in PBs. BMP-1 may function through the osteopontin-CD44v6 axis, regulating cell matrix interactions and signal transduction, to promote tumor cell adhesion and migration and promote lymph node metastasis (34). Therefore, we speculated that microcalcification was significant for predicting CLNM.
When PTC entered the vascular phase of rapid neovascularization from the slow-growing pre-vascular phase, it indicated that the growth rate of the tumor was accelerated, and the neovascularization of the tumor was significantly increased, but the basement membrane development of the neovascularization was not perfect, making the vascular endothelial gap larger (38). High permeability, which also increased the possibility of tumor cell metastasis and spread (39,40). The IC obtained by DECT could directly reflect the tumor blood flow and was affected by the number of blood vessels (41). It was a highly sensitive parameter for identifying benign and malignant thyroid nodules (42,43). Therefore, we hypothesized that differences in iodine intake might result in different ability of lymph node metastasis. In short, thyroid primary lesion with the above independent risk factors were more likely to cause CLNM in PTC patients; and whether it would cause further transmission needed to be further studied.
One study (44) suggested considering fine needle aspiration as a first step in the evaluation of thyroid nodules. Whether DECT parameters in the results of this study can be correlated with fine needle aspiration to a certain extent remains to be further studied. The correlation between digital pathology (45) and DECT will be a research hotspot in the future. Of course, the pathophysiological mechanism of lymph node metastasis in thyroid cancer is very complex. Previous study (46) has found that image analysis and artificial intelligence have considerable potential in thyroid pathology. Perhaps we can further combine IC and artificial intelligence in the future. On the other hand, whether newly discovered thyroid nodules in deceased donors may cause cancer transmission is an interesting topic (44); whether DECT can be used to distinguish deceased donors’ benign and malignant thyroid nodules remains to be further studied.
In the current study, to improve the reliability and accuracy of the prediction model, we performed temporal and geographic validations. We observed that the AUC of external validation cohort II was lower than that of cohort I and that the risk stratification probabilities of cohorts I and II were different. We analyzed the specific reasons for this. First, we chose patients from another hospital as the test subjects, and individual differences among patients in different cities, such as whether the patient’s residence place was a coastal city, eating habits, living habits, etc., was one aspect we need to consider. Second, we performed a retrospective cohort study. In this part, the radiologists completed the data extraction and total score evaluation without knowing the pathological results, resulting in a higher probability of actual occurrence of CLNM in cohort II than cohort I. Therefore, there was no selection bias or subjective tendency. Even so, the AUC of cohort II reached up to 0.861. The prediction model was adequate for basic clinical decisions.
Of note, there were five cases of overestimation or underestimation of CLNM in the retrospective cohort study. For cases with underestimation of CLNM risk, we found that IC in the arterial and venous phases was high in most of them; however, these patients did not have typical ultrasound features, such as microcalcification. Therefore, we believed that DECT was a powerful supplement but not a substitute for ultrasound, so the two image methods should be closely combined in the diagnosis process. For cases with overestimation of CLNM risk, we found that most were due to an excessive emphasis on ultrasound features. When DECT characteristics were not apparent, the total scores might have exceeded 100 points and be included in the high-risk group. For such patients, we should pay much more attention to DECT images, weigh the image evaluation of ultrasound and DECT, and then make the final diagnosis.
There are some limitations to the current study. First, due to its retrospective nature, potential selection bias may exist. However, we conducted a retrospective cohort study and obtained a higher AUC, which also demonstrated the reliability of our model to a certain extent. Of course, we still need to conduct a large sample prospective study to verify the accuracy and reliability of the nomogram in the future. Second, this study was cross-sectional, and we should pay more attention to patients who have undergone CLND and postoperative pathology confirmed cN0 stage. The recurrence rate in these patients will be the focus of the next research. Third, only the patients with a single lesion were included, whether the constructed nomogram was suitable for patients with multiple lesions still needed to be verified by prospective study. Although there were some limitations listed above, the nomogram and risk stratification system constructed in this study were expected to assist clinicians to predict the occurrence probability of CLNM before surgery accurately, so as to update the surgical plan. To maximize the benefit for patients, we look forward to providing additional accurate analysis for future individualized treatment.
Conclusions
Six important parameters from ultrasound and DECT images, including diameter, shape, calcification, A/P, and IC in the arterial and venous phases, were independent risk predictors of CLNM in PTC patients. In the preoperative diagnosis of CLNM, DECT was a useful supplement to ultrasound. This new nomogram facilitated the CLNM prediction and included risk stratification, assisting the formulation of individualized treatment plans.
Acknowledgments
The authors thank Shuang Xia, MD, PhD, Department of Radiology, Tianjin First Central Hospital for the guidance of research content and image acquisition; Jianhua Gu, MD, PhD, Department of General Surgery, Tianjin First Central Hospital for patient recruitment and guidance of clinical work; Wen Shen, MD, PhD, Department of Radiology, Tianjin First Central Hospital for image acquisition; Fang Sun, MD, Department of Ultrasonography, Binzhou Medical University Hospital for patient recruitment and image acquisition; Xi Zhao, Senior engineer, Siemens for his support for dual-energy CT image post-processing.
Funding: This work was supported by
Footnote
Reporting Checklist: The authors have completed the TRIPOD reporting checklist. Available at https://qims.amegroups.com/article/view/10.21037/qims-24-284/rc
Conflicts of Interest: All authors have completed the ICMJE uniform disclosure form (available at https://qims.amegroups.com/article/view/10.21037/qims-24-284/coif). The authors have no conflicts of interest to declare.
Ethical Statement: The authors are accountable for all aspects of the work in ensuring that questions related to the accuracy or integrity of any part of the work are appropriately investigated and resolved. The study was conducted in accordance with the Declaration of Helsinki (as revised in 2013). The study was approved by institutional ethics boards of Tianjin First Central Hospital (No. 2020N220KY) and Binzhou Medical University Hospital (No. LW-24), and individual consent for this retrospective analysis was waived.
Open Access Statement: This is an Open Access article distributed in accordance with the Creative Commons Attribution-NonCommercial-NoDerivs 4.0 International License (CC BY-NC-ND 4.0), which permits the non-commercial replication and distribution of the article with the strict proviso that no changes or edits are made and the original work is properly cited (including links to both the formal publication through the relevant DOI and the license). See: https://creativecommons.org/licenses/by-nc-nd/4.0/.
References
- Siegel RL, Miller KD, Wagle NS, Jemal A. Cancer statistics, 2023. CA Cancer J Clin 2023;73:17-48. [Crossref] [PubMed]
- Cho SY, Lee TH, Ku YH, Kim HI, Lee GH, Kim MJ. Central lymph node metastasis in papillary thyroid microcarcinoma can be stratified according to the number, the size of metastatic foci, and the presence of desmoplasia. Surgery 2015;157:111-8. [Crossref] [PubMed]
- Zhao Q, Ming J, Liu C, Shi L, Xu X, Nie X, Huang T. Multifocality and total tumor diameter predict central neck lymph node metastases in papillary thyroid microcarcinoma. Ann Surg Oncol 2013;20:746-52. [Crossref] [PubMed]
- Carling T, Carty SE, Ciarleglio MM, Cooper DS, Doherty GM, Kim LT, Kloos RT, Mazzaferri EL Sr, Peduzzi PN, Roman SA, Sippel RS, Sosa JA, Stack BC Jr, Steward DL, Tufano RP, Tuttle RM, Udelsman R. American Thyroid Association design and feasibility of a prospective randomized controlled trial of prophylactic central lymph node dissection for papillary thyroid carcinoma. Thyroid 2012;22:237-44. [Crossref] [PubMed]
- So YK, Seo MY, Son YI. Prophylactic central lymph node dissection for clinically node-negative papillary thyroid microcarcinoma: influence on serum thyroglobulin level, recurrence rate, and postoperative complications. Surgery 2012;151:192-8. [Crossref] [PubMed]
- Medas F, Canu GL, Cappellacci F, Anedda G, Conzo G, Erdas E, Calò PG. Prophylactic Central Lymph Node Dissection Improves Disease-Free Survival in Patients with Intermediate and High Risk Differentiated Thyroid Carcinoma: A Retrospective Analysis on 399 Patients. Cancers (Basel) 2020.
- Yeh MW, Bauer AJ, Bernet VA, Ferris RL, Loevner LA, Mandel SJ, Orloff LA, Randolph GW, Steward DL. American Thyroid Association statement on preoperative imaging for thyroid cancer surgery. Thyroid 2015;25:3-14. [Crossref] [PubMed]
- Cong P, Wang XM, Zhang YF. Comparison of artificial intelligence, elastic imaging, and the thyroid imaging reporting and data system in the differential diagnosis of suspicious nodules. Quant Imaging Med Surg 2024;14:711-21. [Crossref] [PubMed]
- Jianming L, Jibin L, Linxue Q. Suspicious ultrasound characteristics correlate with multiple factors that predict central lymph node metastasis of papillary thyroid carcinoma: Significant role of HBME-1. Eur J Radiol 2020;123:108801. [Crossref] [PubMed]
- Xu JM, Xu XH, Xu HX, Zhang YF, Guo LH, Liu LN, Liu C, Bo XW, Qu S, Xing M, Li XL. Prediction of cervical lymph node metastasis in patients with papillary thyroid cancer using combined conventional ultrasound, strain elastography, and acoustic radiation force impulse (ARFI) elastography. Eur Radiol 2016;26:2611-22. [Crossref] [PubMed]
- Wang HL, Zhang S, Xin XJ, Zhao LH, Li CX, Mu JL, Wei XQ. Application of Real-time Ultrasound Elastography in Diagnosing Benign and Malignant Thyroid Solid Nodules. Cancer Biol Med 2012;9:124-7. [Crossref] [PubMed]
- Tong Y, Li J, Huang Y, Zhou J, Liu T, Guo Y, Yu J, Zhou S, Wang Y, Chang C. Ultrasound-Based Radiomic Nomogram for Predicting Lateral Cervical Lymph Node Metastasis in Papillary Thyroid Carcinoma. Acad Radiol 2021;28:1675-84. [Crossref] [PubMed]
- Liu X, Ouyang D, Li H, Zhang R, Lv Y, Yang A, Xie C. Papillary thyroid cancer: dual-energy spectral CT quantitative parameters for preoperative diagnosis of metastasis to the cervical lymph nodes. Radiology 2015;275:167-76. [Crossref] [PubMed]
- Lee JH, Ha EJ, Kim JH. Application of deep learning to the diagnosis of cervical lymph node metastasis from thyroid cancer with CT. Eur Radiol 2019;29:5452-7. [Crossref] [PubMed]
- Cho SJ, Suh CH, Baek JH, Chung SR, Choi YJ, Lee JH. Diagnostic performance of CT in detection of metastatic cervical lymph nodes in patients with thyroid cancer: a systematic review and meta-analysis. Eur Radiol 2019;29:4635-47. [Crossref] [PubMed]
- Zhou Y, Su GY, Hu H, Ge YQ, Si Y, Shen MP, Xu XQ, Wu FY. Radiomics analysis of dual-energy CT-derived iodine maps for diagnosing metastatic cervical lymph nodes in patients with papillary thyroid cancer. Eur Radiol 2020;30:6251-62. [Crossref] [PubMed]
- He M, Lin C, Yin L, Lin Y, Zhang S, Ma M. Value of Dual-Energy Computed Tomography for Diagnosing Cervical Lymph Node Metastasis in Patients With Papillary Thyroid Cancer. J Comput Assist Tomogr 2019;43:970-5. [Crossref] [PubMed]
- Lee Y, Kim JH, Baek JH, Jung SL, Park SW, Kim J, Yun TJ, Ha EJ, Lee KE, Kwon SY, Yang KS, Na DG. Value of CT added to ultrasonography for the diagnosis of lymph node metastasis in patients with thyroid cancer. Head Neck 2018;40:2137-48. [Crossref] [PubMed]
- Xing Z, Qiu Y, Yang Q, Yu Y, Liu J, Fei Y, Su A, Zhu J. Thyroid cancer neck lymph nodes metastasis: Meta-analysis of US and CT diagnosis. Eur J Radiol 2020;129:109103. [Crossref] [PubMed]
- Ren L, Rajendran K, McCollough CH, Yu L. Quantitative accuracy and dose efficiency of dual-contrast imaging using dual-energy CT: a phantom study. Med Phys 2020;47:441-56. [Crossref] [PubMed]
- Collins GS, Reitsma JB, Altman DG, Moons KG. Transparent Reporting of a multivariable prediction model for Individual Prognosis or Diagnosis (TRIPOD): the TRIPOD statement. Ann Intern Med 2015;162:55-63.
- Huang YQ, Liang CH, He L, Tian J, Liang CS, Chen X, Ma ZL, Liu ZY. Development and Validation of a Radiomics Nomogram for Preoperative Prediction of Lymph Node Metastasis in Colorectal Cancer. J Clin Oncol 2016;34:2157-64. [Crossref] [PubMed]
- Kang S, Kang WD, Chung HH, Jeong DH, Seo SS, Lee JM, Lee JK, Kim JW, Kim SM, Park SY, Kim KT. Preoperative identification of a low-risk group for lymph node metastasis in endometrial cancer: a Korean gynecologic oncology group study. J Clin Oncol 2012;30:1329-34. [Crossref] [PubMed]
- Nakauchi C, Naoi Y, Shimazu K, Tsunashima R, Nishio M, Maruyama N, Shimomura A, Kagara N, Shimoda M, Kim SJ, Noguchi S. Development of a prediction model for lymph node metastasis in luminal A subtype breast cancer: the possibility to omit sentinel lymph node biopsy. Cancer Lett 2014;353:52-8. [Crossref] [PubMed]
- Pyo JH, Shin CM, Lee H, Min BH, Lee JH, Kim SM, Choi MG, Lee JH, Sohn TS, Bae JM, Kim KM, Kim HS, Jung SH, Kim JJ, Kim S. JHP and CMS contributed equally as the first authors of this study. A Risk-prediction Model Based on Lymph-node Metastasis for Incorporation Into a Treatment Algorithm for Signet Ring Cell-type Intramucosal Gastric Cancer. Ann Surg 2016;264:1038-43. [Crossref] [PubMed]
- Gu Y, She Y, Xie D, Dai C, Ren Y, Fan Z, Zhu H, Sun X, Xie H, Jiang G, Chen C. A Texture Analysis-Based Prediction Model for Lymph Node Metastasis in Stage IA Lung Adenocarcinoma. Ann Thorac Surg 2018;106:214-20. [Crossref] [PubMed]
- Haugen BR, Alexander EK, Bible KC, Doherty GM, Mandel SJ, Nikiforov YE, Pacini F, Randolph GW, Sawka AM, Schlumberger M, Schuff KG, Sherman SI, Sosa JA, Steward DL, Tuttle RM, Wartofsky L. 2015 American Thyroid Association Management Guidelines for Adult Patients with Thyroid Nodules and Differentiated Thyroid Cancer: The American Thyroid Association Guidelines Task Force on Thyroid Nodules and Differentiated Thyroid Cancer. Thyroid 2016;26:1-133. [Crossref] [PubMed]
- Kerr KF, Brown MD, Zhu K, Janes H. Assessing the Clinical Impact of Risk Prediction Models With Decision Curves: Guidance for Correct Interpretation and Appropriate Use. J Clin Oncol 2016;34:2534-40. [Crossref] [PubMed]
- Dormann CF, Elith J, Bacher S, Buchmann C, Carl G, Carré G, Marquéz JRG, Gruber B, Lafourcade B, Leitão PJ, Münkemüller T, McClean C, Osborne PE, Reineking B, Schröder B, Skidmore AK, Zurell D, Lautenbach S. Collinearity: a review of methods to deal with it and a simulation study evaluating their performance. Ecography 2013;36:27-46.
- Mu J, Liang X, Li F, Liu J, Zhang S, Tian J. Ultrasound features of extranodal extension in the metastatic cervical lymph nodes of papillary thyroid cancer: a case-control study. Cancer Biol Med 2018;15:171-7. [Crossref] [PubMed]
- Yoon SJ, Yoon DY, Chang SK, Seo YL, Yun EJ, Choi CS, Bae SH. "Taller-than-wide sign" of thyroid malignancy: comparison between ultrasound and CT. AJR Am J Roentgenol 2010;194:W420-4. [Crossref] [PubMed]
- Liang F, Li X, Ji Q, He D, Yang M, Xu Z. Revised Thyroid Imaging Reporting and Data System (TIRADS): imitating the American College of Radiology TIRADS, a single-center retrospective study. Quant Imaging Med Surg 2023;13:3862-72. [Crossref] [PubMed]
- Wu Q, Zhang YM, Sun S, Li JJ, Wu J, Li X, Zhu S, Wei W, Sun SR. Clinical and sonographic assessment of cervical lymph node metastasis in papillary thyroid carcinoma. J Huazhong Univ Sci Technolog Med Sci 2016;36:823-7. [Crossref] [PubMed]
- Sun Y, Fang S, Dong H, Zhao C, Yang Z, Li P, Wang J. Correlation between osteopontin messenger RNA expression and microcalcification shown on sonography in papillary thyroid carcinoma. J Ultrasound Med 2011;30:765-71. [Crossref] [PubMed]
- Bai Y, Zhou G, Nakamura M, Ozaki T, Mori I, Taniguchi E, Miyauchi A, Ito Y, Kakudo K. Survival impact of psammoma body, stromal calcification, and bone formation in papillary thyroid carcinoma. Mod Pathol 2009;22:887-94. [Crossref] [PubMed]
- Ferreira LB, Gimba E, Vinagre J, Sobrinho-Simões M, Soares P. Molecular Aspects of Thyroid Calcification. Int J Mol Sci 2020;21:7718. [Crossref] [PubMed]
- Karaman S, Detmar M. Mechanisms of lymphatic metastasis. J Clin Invest 2014;124:922-8. [Crossref] [PubMed]
- Galrão AL, Camargo RY, Friguglietti CU, Moraes L, Cerutti JM, Serrano-Nascimento C, Suzuki MF, Medeiros-Neto G, Rubio IG. Hypermethylation of a New Distal Sodium/Iodide Symporter (NIS) enhancer (NDE) is associated with reduced NIS expression in thyroid tumors. J Clin Endocrinol Metab 2014;99:E944-52. [Crossref] [PubMed]
- Vergez S, Sarini J, Percodani J, Serrano E, Caron P. Lymph node management in clinically node-negative patients with papillary thyroid carcinoma. Eur J Surg Oncol 2010;36:777-82. [Crossref] [PubMed]
- Zhou Y, Su GY, Hu H, Tao XW, Ge YQ, Si Y, Shen MP, Xu XQ, Wu FY. Radiomics from Primary Tumor on Dual-Energy CT Derived Iodine Maps can Predict Cervical Lymph Node Metastasis in Papillary Thyroid Cancer. Acad Radiol 2022;29:S222-31. [Crossref] [PubMed]
- Tawfik AM, Michael Bucher A, Vogl TJ. Dual-Energy Computed Tomography Applications for the Evaluation of Cervical Lymphadenopathy. Neuroimaging Clin N Am 2017;27:461-8. [Crossref] [PubMed]
- Haugen BR. 2015 American Thyroid Association Management Guidelines for Adult Patients with Thyroid Nodules and Differentiated Thyroid Cancer: What is new and what has changed? Cancer 2017;123:372-81. [Crossref] [PubMed]
- Jiang L, Liu D, Long L, Chen J, Lan X, Zhang J. Dual-source dual-energy computed tomography-derived quantitative parameters combined with machine learning for the differential diagnosis of benign and malignant thyroid nodules. Quant Imaging Med Surg 2022;12:967-78. [Crossref] [PubMed]
- Eccher A, Girolami I, D'Errico A, Zaza G, Carraro A, Montin U, Boggi U, Scarpa A, Brunelli M, Martignoni G, Segev D, Rossi ED, Pantanowitz L. Management of Thyroid Nodules in Deceased Donors With Comparison Between Fine Needle Aspiration and Intraoperative Frozen Section in the Setting of Transplantation. Prog Transplant 2019;29:316-20. [Crossref] [PubMed]
- Eccher A, Girolami I, Brunelli M, Novelli L, Mescoli C, Malvi D, D'Errico A, Luchini C, Furian L, Zaza G, Cardillo M, Boggi U, Pantanowitz L. Digital pathology for second opinion consultation and donor assessment during organ procurement: Review of the literature and guidance for deployment in transplant practice. Transplant Rev (Orlando) 2020;34:100562. [Crossref] [PubMed]
- Girolami I, Marletta S, Pantanowitz L, Torresani E, Ghimenton C, Barbareschi M, Scarpa A, Brunelli M, Barresi V, Trimboli P, Eccher A. Impact of image analysis and artificial intelligence in thyroid pathology, with particular reference to cytological aspects. Cytopathology 2020;31:432-44. [Crossref] [PubMed]