Predictive performance of [18F]F-fibroblast activation protein inhibitor (FAPI)-42 positron emission tomography/computed tomography (PET/CT) in evaluating response of recurrent or metastatic gastrointestinal stromal tumors: complementary or alternative to [18F]fluorodeoxyglucose (FDG) PET/CT?
Introduction
Gastrointestinal stromal tumors (GISTs) represent a subtype of sarcoma originating from Cajal mesenchymal cells or their precursor cells in the myenteric plexus (1), with a current incidence of 6–22/1,000,000/year (2). Approximately 50% of patients experience recurrence or metastasis within 5 years after resection of the primary tumor (3,4). Targeted therapy is the standard-of-care treatment for recurrent or metastatic GISTs, and the detection of treatment resistance and objective responses during follow-up is clinically challenging. When targeted therapy is effective, surgical resection of the locally recurrent or metastatic lesion may minimize damage to vital organ function and prolong survival (5). Imatinib, sunitinib, regorafenib, and ripretinib are the prevalent therapies for recurrent/metastatic GISTs, yet the efficacy in some cases falls short of expectations (6-10). In addition, the biological behavior of GISTs is complex and heterogeneous, making it difficult to assess treatment efficacy. The Response Criteria in Solid Tumors 1.1 (RECIST1.1) is recommended as the primary response assessment criteria for GISTs (11). However, the response of GISTs to targeted therapy is evident after 6 months of treatment (9), and the 6-month progression-free survival after targeted therapy is significantly correlated with the prognosis (12). Therefore, it is crucial to predict the response of targeted therapy early and accurately during the 6-month follow-up, which can help fine-tune therapeutic strategies and reduce unnecessary drug toxicity and the high cost of targeted therapy.
There is an increasing consensus that [18F]fluorodeoxyglucose positron emission tomography/computed tomography ([18F]FDG PET/CT) is highly effective in detecting the recurrence and metastasis (13), monitoring early re-staging (14) and predicting the prognosis of GISTs (15). A study showed that 18% more lesions could be detected in GIST patients by [18F]FDG PET/CT than other modalities [ultrasound, magnetic resonance imaging (MRI), and computed tomography (CT)], positively influencing clinical re-staging (16). However, approximately 13–20% of recurrent or metastatic GISTs present as non-FDG-avid lesions (14,17,18), limiting the value of [18F]-fluorodeoxyglucose ([18F]FDG) in treatment evaluation. Therefore, more specific, and accurate imaging examinations are needed to predict the response of GISTs to targeted therapy.
Fibroblast activation protein (FAP) is a type II transmembrane serine protease that is highly expressed in many epithelial cancer-associated fibroblasts (CAFs) and some mesenchymal-derived tumor cells, playing an essential role in tumor growth and metastasis (19). The healthy gastrointestinal tract and liver show low uptake background in fibroblast activation protein inhibitor(FAPI) PET/CT (20,21), and our previous study consistently revealed that the [18F]F-fibroblast activation protein inhibitor ([18F]FAPI)-42 PET/CT ([18F]FAPI-42 PET/CT) was superior to [18F]FDG PET/CT for imaging recurrent or metastatic GISTs, especially for detecting liver metastases (22). Herein, we aimed to investigate the value of FAPI PET in predicting the treatment response of recurrent or metastatic GISTs, in comparison to [18F]FDG PET/CT. We also intended to explore the model based on PET/CT parameters and clinical parameters to ascertain the optimal utilization of these parameters for enhanced predictive accuracy.
Methods
The study was conducted in accordance with the Declaration of Helsinki (as revised in 2013). The study was approved by the Ethics Committee of The First Affiliated Hospital of Sun Yat-sen University (Ethics Review [2022] No. 257) and registered on the Chinese medical research registration information system (No. MR-44-23-024246). Written informed consent was obtained from each patient prior to each PET/CT scan.
Patients
This study retrospectively analyzed 27 patients with recurrent or metastatic GISTs who underwent baseline [18F]FAPI-42 PET/CT and [18F]FDG PET/CT before switching targeted therapy from September 2021 to June 2022. The inclusion criteria included: (I) recurrent or metastatic GISTs confirmed by pathology or follow-up imaging [the diagnosis references according to our previous study (22)]; (II) patients who underwent baseline [18F]FAPI-42 PET/CT and [18F]FDG PET/CT before switching targeted therapy; (III) patients who underwent contrast-enhanced CT to assess the response after six months of new targeted therapy. The exclusion criteria were as follows: (I) patients who underwent ablation or surgery within 6 months of new targeted therapy; (II) patients who experienced treatment interruption; (III) patients with other malignant tumors.
We evaluated the response of each lesion separately by using contrast-enhanced CT after 6 months of new targeted therapy. The lesions were classified into four groups based on the RECIST 1.1 criteria: complete response (CR), partial response (PR), stable disease (SD), and progressive disease (PD). Lesions classified as CR, PR, and SD were considered non-progression lesions, while lesions classified as PD were considered progression lesions. Gene mutations of lesions and type of targeted therapy were collected during the initial diagnosis.
Radiopharmaceuticals and PET/CT image acquisition
[18F]FAPI‑42 and [18F]FDG were synthesized in the nuclear medicine department of the First Affiliated Hospital of Sun Yat-sen University, as previously documented in our previous study (22).
Patients underwent [18F]FDG PET/CT and [18F]FAPI-42 PET/CT on two days of the same week. Before taking [18F]FDG PET/CT, patients were required to fast for at least 6 hours and maintain a blood glucose level of less than 10 mmol/L. Patients were given 259±26 MBq [18F]FAPI-42 or 5.18 MBq/kg [18F]FDG intravenously, and the PET/CT scan was performed using a uMI780 scanner (United Imaging Healthcare, Shanghai, China) around 60 min after injection. Parameters for CT were as follows: voltage =120 kV, current =174 mA, CT reconstruction = thickness 1.25 mm, matrix =512×512. Immediately after the completion of the CT scan, PET scanning was performed in the same axial field of view, and 6–8 beds were collected according to the patient’s height in three-dimension (3D) acquisition mode, and each bed was collected for 1 min. The PET image dataset was reconstructed using the ordered subset expectation maximization iterative reconstruction method.
Image analyses
All images were processed by the PETVCAR (PET Volume Computed Assisted Reading) software of the AW4.7 (Advantage workstation 4.7) post-processing workstation. The images were analyzed jointly by two clinically experienced nuclear medicine physicians. The volume of interest (VOI) was then manually adjusted according to the 3 levels of cross-sectional, sagittal, and coronal images to include the lesions in the VOI as accurately as possible at each level. Parameter measurements were performed using a fixed threshold method with a threshold set at 41% of the maximum standardized uptake value (SUVmax) (23). Volumetric segmentation of the lesions was performed automatically by the software on [18F]FAPI-42 PET/CT and [18F]FDG PET/CT images, respectively, and the corresponding metabolic parameters were obtained: the mean standardized uptake value in FAPI PET/CT (SUVmean-FAPI), SUVmean-FDG, the standard uptake value corrected for lean body mass in FAPI PET/CT (SULpeak-FAPI), the standard uptake value corrected for lean body mass in FDG PET/CT (SULpeak-FDG), maximum standardized uptake value in FAPI PET/CT (SUVmax-FAPI), maximum standardized uptake value in FDG PET/CT (SUVmax-FDG), metabolic tumor volume (MTV) and total lesion glycolysis (TLG), FAPI-positive tumor volume (GTV-FAPI), and FAPI-positive total lesion accumulation (TL-FAPI). Tumor-to-blood pool ratio (TBR) was calculated by dividing the tumor SUVmax by the SUVmax of the blood pool (a VOI with a 1.5 cm diameter was placed in the aorta arch). The tumor-to-liver ratio (TLR) was calculated by dividing the tumor SUVmax by the SUVmax of the liver (measured in a 3 cm VOI in all scans while avoiding large vessels.
Statistical analysis
Univariate analysis was performed between the non-progressive and progressive groups using IBM SPSS Statistics software (version 26.0, IBM Corp, Armonk, NY, USA) to screen the independent variables. The Mann-Whitney U test was used to compare quantitative variables. Categorical data were analyzed using Pearson’s Chi-square test or Fisher’s exact probability test. A P value <0.05 was statistically significant.
Multivariate logistic regression models based on PET/CT parameters were developed by different combinations of significant parameters in univariate analyses. The receiver operating characteristic curves (ROC) were constructed using the R (version 4.2.2) package “reportROC”. Delong’s test was used to compare the area under the curve (AUC) of different models to assess the discrimination of the models. A 5-fold cross-validation was used for internal validation. The calibration curves were plotted using the R package “riskRegression”. A smaller Brier score value indicated better model calibration (24). The Hosmer-Lemeshow test was used to assess the goodness of fit of the curves.
Finally, a nomogram of the optimal model was plotted using the R package “rms”.
Results
Patient demographics
A total of 27 patients with recurrent/metastatic GISTs were included in this study (Figure 1), exhibiting a male predominance (n=18), with 13 patients younger than 60 years and 14 patients older than 60 years) (Table 1). The most common primary sites were the jejunum and ileum (17/27, 63.0%), followed by the gastric (6/27, 22.2%), duodenum (3/27, 11.1%), and rectum (1/27, 3.7%). KIT proto-oncogene receptor tyrosine kinase gene (KIT) exon 11 mutation was the most common type of mutation (16/27, 59.3%), followed by wild-type and other mutations (n=6, 22.2%) and KIT exon 9 mutations (n=5, 18.5%). Treatment was switched to the following targeted drugs: imatinib (n=15, 55.6%), sunitinib (n=6, 22.2%), regorafenib (n=4, 14.8%), ripretinib (n=1, 3.7%), and other target drugs (n=1, 3.7%).

Table 1
Parameters | No. of patients (%) |
---|---|
Sex | |
Man | 18 (66.7) |
Woman | 9 (33.3) |
Age (years) | |
≤60 | 13 (48.1) |
>60 | 14 (51.9) |
Primary location | |
Gastric | 6 (22.2) |
Duodenum | 3 (11.1) |
Jejunum and ileum | 17 (63.0) |
Rectum | 1 (3.7) |
Previous treatment | |
Surgery only | 0 (0.0) |
Targeted therapy | 0 (0.0) |
Both surgery and targeted therapy | 27 (100) |
Type of gene mutation | |
KIT exon 9 | 5 (18.5) |
KIT exon 11 | 16 (59.3) |
Wild-type and others | 6 (22.2) |
Targeted therapy | |
Imatinib | 15 (55.6) |
Sunitinib | 6 (22.2) |
Regorafenib | 4 (14.8) |
Ripretinib | 1 (3.7) |
Others | 1 (3.7) |
GISTs, gastrointestinal stromal tumors; KIT, KIT proto-oncogene receptor tyrosine kinase gene.
Comparison of PET/CT parameters and clinicopathological indicators between the non-progression group (NPG) and progression group (PG)
A total of 110 lesions (27 in the PG and 83 in the non-progressive group) were retrospectively analyzed in our study (Table 2). For [18F]FDG PET/CT parameters, tumor-to-blood pool SUV ratio (TBR)-FDG (P=0.033) and SUVmean-FDG (P=0.038) in the NPG were lower than that in the PG, while the differences in tumor-to-liver SUV ratio in FDG PET/CT (TLR-FDG), SUVmax-FDG, MTV, and TLG were comparable between the two groups. For [18F]FAPI-42 PET/CT parameters, TLF-FAPI (P=0.049) and SULpeak-FAPI (P=0.010) in the NPG were higher than that in the PG, while the remaining parameters (tumor-to-liver SUV ratio in FAPI PET/CT (TLR-FAPI), tumor-to-blood pool SUV ratio in FAPI PET/CT (TBR-FAPI), SUVmax-FAPI, SUVmean-FAPI, GTV-FAPI were comparable between the two groups. In terms of clinicopathological indicators, the type of targeted drug used was significantly associated with PD (P<0.001). There was a nearly significant difference in the type of gene mutation observed between the non-progression and PGs (P<0.1).
Table 2
Variables | Non-progression group | Progressive group | Total | P |
---|---|---|---|---|
TLR-FDG | 1.46 (1.13, 2.49) | 2.15 (1.28, 4.84) | 1.59 (1.14, 3.03) | 0.075 |
TBR-FDG | 1.89 (1.45, 3.60) | 3.20 (1.76, 5.63) | 2.11 (1.49, 3.89) | 0.033* |
SUVmax-FDG | 2.76 (2.15, 5.71) | 3.89 (2.62, 8.20) | 3.10 (2.27, 6.40) | 0.053 |
SUVmean-FDG | 1.94 (1.44, 3.23) | 2.75 (1.95, 4.84) | 2.15 (1.52, 3.46) | 0.038* |
MTV | 5.52 (1.46, 26.42) | 3.61 (1.20, 24.22) | 4.76 (1.46, 25.00) | 0.567 |
TLG | 10.00 (2.70, 112.70) | 11.30 (2.50, 77.50) | 10.90 (2.68, 79.40) | 0.972 |
SULpeak-FDG | 1.77 (1.17, 3.72) | 2.51 (1.37, 5.20) | 1.87 (1.17, 4.26) | 0.198 |
TLR-FAPI | 3.79 (2.24, 9.34) | 3.07 (1.97, 6.45) | 3.59 (2.18, 8.57) | 0.514 |
TBR-FAPI | 2.85 (1.76, 5.63) | 2.30 (1.51, 4.86) | 2.73 (1.72, 5.17) | 0.314 |
SUVmax-FAPI | 3.22 (1.56, 6.12) | 1.95 (1.30, 4.13) | 2.90 (1.49, 5.73) | 0.057 |
SUVmean-FAPI | 1.75 (1.00, 3.37) | 1.24 (0.81, 2.47) | 1.63 (0.94, 3.00) | 0.056 |
GTV-FAPI | 4.74 (1.60, 35.47) | 2.48 (0.86, 7.84) | 3.97 (1.52, 28.17) | 0.249 |
TLF-FAPI | 8.30 (2.80, 80.10) | 3.60 (1.10, 11.90) | 7.80 (2.40, 60.08) | 0.049* |
SULpeak-FAPI | 1.76 (0.90, 4.27) | 1.02 (0.00, 2.02) | 1.54 (0.79, 3.35) | 0.010* |
Type of gene mutation | 0.076 | |||
KIT exon 9 | 18 | 5 | 23 | |
KIT exon 11 | 54 | 13 | 67 | |
WT and others | 11 | 9 | 20 | |
Total | 83 | 27 | 110 | |
Targeted therapy | <0.001*** | |||
Imatinib | 41 | 2 | 43 | |
Sunitinib | 29 | 7 | 36 | |
Regorafenib | 9 | 10 | 19 | |
Ripretinib | 2 | 3 | 5 | |
Others | 2 | 5 | 7 | |
Total | 83 | 27 | 110 |
Data are presented as M (P25, P75) or number. *, P<0.05; ***, P<0.001. PET/CT, positron emission tomography/computed tomography; TLR-FDG, tumor-to-liver standardized uptake value ratio in FDG PET/CT; TBR-FDG, tumor-to-blood pool standardized uptake value ratio in FDG EPT/CT; SUVmax-FDG, maximum standardized uptake value in FDG PET/CT; SUVmean-FDG, mean standardized uptake value in FDG PET/CT; MTV, metabolic tumor volume; TLG, total lesion glycolysis; SULpeak-FDG, peak standard uptake value corrected for lean body mass in FDG PET/CT; TLR-FAPI, tumor-to-liver SUV ratio in FAPI PET/CT; TBR-FAPI, tumor-to-blood pool standardized uptake value ratio in FAPI PET/CT; SUVmax-FAPI, maximum standardized uptake value in FAPI PET/CT; SUVmean-FAPI, mean standardized uptake value in FAPI PET/CT; GTV-FAPI, FAPI-positive tumor volume; TLF-FAPI, FAPI-positive total lesion accumulation; SULpeak-FAPI, peak standard uptake value corrected for lean body mass in FAPI PET/CT; KIT, KIT proto-oncogene receptor tyrosine kinase gene; WT, wild-type; FDG, fluorodeoxyglucose; FAPI, fibroblast activation protein inhibitor; M (P25, P75), median (interquartile range).
Collinearity test of [18F]FAPI-42, [18F]FDG PET/CT parameters and clinical indicators
Given that a significant correlation was observed between TBR-FDG and SUVmean-FDG (r=0.86) and between TLF-FAPI and SULpeak-FAPI (r=0.90) (Figure 2), TBR-FDG and SULpeak-FAPI were included in the model. To investigate the value of [18F]FAPI-42 PET/CT in predicting the treatment response of recurrent or metastatic GISTs compared with [18F]FDG PET/CT and explore the model based on PET/CT parameters and clinical parameters to ascertain the optimal utilization of these parameters for enhanced predictive ability. We further included gene mutation in the models, which was not associated with FDG PET/CT and FAPI PET/CT parameters (Table 3). Therefore, TBR-FDG, SULpeak-FAPI, gene mutation type, and targeted drugs were included as variables in the logistic regression model.
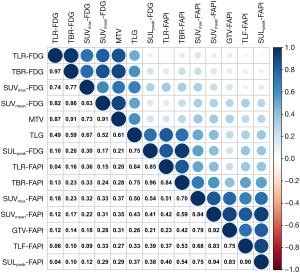
Table 3
Parameters | KIT exon 9 | KIT exon 11 | Wild-type and others | P |
---|---|---|---|---|
TLR-FDG | 1.90 (1.51, 3.35) | 1.37 (0.98, 4.19) | 1.59 (1.19, 2.60) | 0.342 |
TBR-FDG | 2.76 (2.23, 4.82) | 1.76 (1.37, 4.69) | 1.97 (1.55, 3.51) | 0.095 |
SUVmax-FDG | 3.97 (3.37, 6.07) | 2.58 (2.10, 7.71) | 3.51 (2.65, 6.04) | 0.077 |
SUVmean-FDG | 2.65 (1.95, 3.23) | 1.87 (1.36, 4.51) | 2.33 (1.58, 3.44) | 0.107 |
MTV | 2.01 (0.78, 14.47) | 6.88 (1.65, 55.64) | 3.48 (1.19, 10.53) | 0.053 |
TLG | 5.40 (2.80, 21.00) | 21.60 (2.60, 117.4) | 6.95 (2.60, 57.20) | 0.299 |
SULpeak-FDG | 2.15 (1.11, 2.92) | 1.59 (1.57, 4.90) | 2.21 (1.40, 4.34) | 0.518 |
TLR-FAPI | 3.07 (2.28, 16.16) | 4.23 (1.90, 8.21) | 3.14 (2.33, 14.10) | 0.648 |
TBR-FAPI | 2.46 (1.80, 6.67) | 2.91 (1.50, 5.38) | 1.96 (1.71, 3.96) | 0.619 |
SUVmax-FAPI | 2.04 (1.26, 7.27) | 3.33 (1.56, 5.92) | 2.33 (1.40, 5.32) | 0.639 |
SUVmean-FAPI | 1.22 (0.88, 4.12) | 1.98 (0.95, 2.99) | 1.50 (0.94, 2.97) | 0.743 |
GTV-FAPI | 3.00 (0.63, 13.31) | 5.09 (1.80, 40.81) | 1.84 (1.08, 7.97) | 0.095 |
TLF-FAPI | 4.40 (1.30, 25.6) | 8.30 (3.50, 105.30) | 4.10 (1.53, 18.75) | 0.141 |
SULpeak-FAPI | 1.26 (0.75, 5.11) | 1.84 (0.95, 3.33) | 1.15 (0.73, 2.99) | 0.366 |
Data are presented as M (P25, P75). FDG, fluorodeoxyglucose; FAPI, fibroblast activation protein inhibitor; PET/CT, positron emission tomography/computed tomography; KIT, KIT proto-oncogene receptor tyrosine kinase gene; TLR-FDG, tumor-to-liver standardized uptake value ratio in FDG PET/CT; TBR-FDG, tumor-to-blood pool standardized uptake value ratio in FDG EPT/CT; SUVmax-FDG, maximum standardized uptake value in FDG PET/CT; SUVmean-FDG, mean standardized uptake value in FDG PET/CT; MTV, metabolic tumor volume; TLG, total lesion glycolysis; SULpeak-FDG, peak standard uptake value corrected for lean body mass in FDG PET/CT; TLR-FAPI, tumor-to-liver SUV ratio in FAPI PET/CT; TBR-FAPI, tumor-to-blood pool SUV ratio in FAPI PET/CT; SUVmax-FAPI, maximum standardized uptake value in FAPI PET/CT; SUVmean-FAPI, mean standardized uptake value in FAPI PET/CT; GTV-FAPI, FAPI-positive tumor volume; TLF-FAPI, FAPI-positive total lesion accumulation; SULpeak-FAPI, peak standard uptake value corrected for lean body mass in FAPI PET/CT; M (P25, P75), median (interquartile range).
Comparison of models with different parameters and internal validation
Four models were developed with the dependent variable being the progression status, and different combinations of independent variables including TBR-FDG, SULpeak-FAPI, gene mutation type, and targeted drug (model 1: TBR-FDG + SULpeak-FAPI + gene mutation type + targeted drug; model 2: TBR-FDG + SULpeak-FAPI; model 3: TBR-FDG; model 4: SULpeak-FAPI).
The area under the curve of model 1 (AUC =0.865) was significantly higher than model 2 (AUC =0.721, P<0.001), model 3 (AUC =0.637, P<0.001) and model 4 (AUC =0.665, P<0.001) (Figure 3, Table 4). Notably, the AUC of model 3 is similar to that of model 4. Five-fold cross-validation showed that model 1 yielded the highest average AUC among these four models (AUC =0.802). The sensitivity of models 1, 2, 3, and 4 were 70.4%, 81.5%, 59.3%, and 66.7%, and the specificity was 90.4%, 57.8%, 69.9%, and 59.0%, respectively (Table 4). These findings suggest that model 1 exhibited the best discrimination ability.
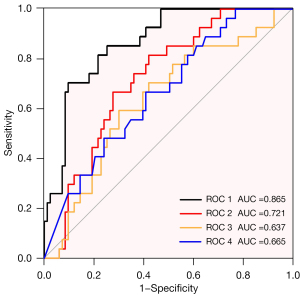
Table 4
Model | Factors | AUC (95% CI) | 5-fold cross-validation | Sensitivity | Specificity | P value (Delong’s test) |
---|---|---|---|---|---|---|
Model 1 | Gene mutation + drug + TBR-FDG + SULpeak-FAPI | 0.865 (0.794–0.936) | 0.802 | 70.4% | 90.4% | Reference |
Model 2 | TBR-FDG + SULpeak-FAPI | 0.721 (0.620–0.821) | 0.552 | 81.5% | 57.8% | <0.001 |
Model 3 | TBR-FDG | 0.637 (0.518–0.757) | 0.552 | 59.3% | 69.9% | <0.001 |
Model 4 | SULpeak-FAPI | 0.665 (0.553–0.777) | 0.615 | 66.7% | 59.0% | <0.001 |
AUC, area under the curve; 95% CI, 95% confidence interval; TBR-FDG, tumor-to-blood pool standardized uptake value ratio in FDG PET/CT; FDG, fluorodeoxyglucose; SULpeak-FAPI, peak standard uptake value corrected for lean body mass in FAPI PET/CT; PET/CT, positron emission tomography/computed tomography; FAPI, fibroblast activation protein inhibitor.
A calibration curve was generated showing that model 1 yielded the most accurate calibration of the four models (as depicted in Figure 4) and had an excellent goodness-of-fit, as determined by the Hosmer-Lemeshow test (P>0.05).
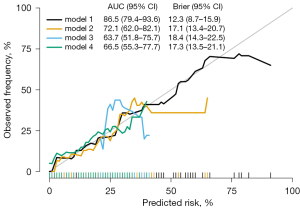
Visualization of the optimal model with a nomogram
A nomogram was used to illustrate model 1 (Figure 5) and facilitate clinical application, which incorporated TBR-FDG, SULpeak-FAPI, gene mutation type, and targeted drug as predictors. When the gene mutation type was wild-type and others (except KIT exon 9 and KIT exon 11), the risk of progression was correspondingly increased. The risk of progression increased with the use of first- to fourth-line targeted agents. Higher TBR-FDG levels were associated with an increased risk of progression, although this was observed in a smaller proportion of cases. Conversely, a lower risk of progression was unexpectedly associated with higher SULpeak-FAPI levels. Two representative cases were presented in Figure 6 to illustrate this relationship.
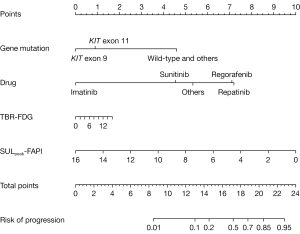
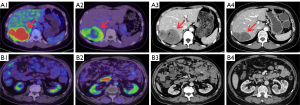
Discussion
In this study, we highlighted that both baseline [18F]FAPI-42 PET/CT and [18F]FDG PET/CT had predictive value in assessing treatment responses for recurrent or metastatic GIST lesions. Furthermore, we determined that the combined predictive capacity of FAPI and FDG PET imaging enhances the precision of response predictions, surpassing the capabilities of each method individually. Significantly, our study revealed that a model integrating baseline [18F]FAPI-42 PET/CT, [18F]FDG PET/CT parameters, gene mutation type, and targeted therapy yielded the most accurate predictions for recurrent metastatic GISTs.
[18F]FDG uptake is a promising indicator for predicting tumor progression. [18F]FDG PET/CT-based imaging parameters such as SUV, SUL, MTV, and TLG have previously been suggested as potential prognostic markers for various tumors (25-29), and high MTV and TLG in GISTs are associated with a risk of poor prognosis (29). However, in our study, only TBR-FDG and SUVmean-FDG were higher in the PG than in the NPG, while there was no difference in MTV and TLG between the progression and NPGs. The possible reason is that the fixed threshold (41% of SUVmax) was not always effective in determining tumor metabolic volume due to noise, tracer uptake of tumor and background homogeneity, and tumor/background ratio (30). Moreover, our findings lead us to hypothesize that the proliferation of GISTs lesions may not be governed by the aggregate volume and glycolytic activity of the tumor cells as a whole. Instead, it appears to be driven by a specific subset of cells characterized by heightened glucose metabolic activity. It is well-established that the measurement of SUVmax is influenced by body composition, time intervals, technical factors, tumor burden, tumor volume, and VOI (31,32). Studies have demonstrated that normalizing the SUVmax of tumors with a suitable reference background can address this heterogeneity in some cases (31). The liver and blood pools are commonly used as reference backgrounds as they maintain a relatively constant SUV level following injection over time (33). In this study, TBR rather than TLR differed significantly between progression and NPGs, likely due to the SUVmax of the blood pool being smaller than that of the liver, resulting in a larger ratio and a more significant difference. In general, baseline [18F]FDG PET/CT parameters (TBR-FDG and SUVmean-FDG) had predictive value in assessing treatment responses for recurrent or metastatic GIST lesions.
According to our previous study, fibroblast-activating protein-targeted imaging (FAPI PET/CT) is superior to FDG PET/CT in the imaging of metastatic/recurrent GISTs (22). Moreover, FAPI PET/CT has been found to have predictive value in the efficacy and prognosis of esophageal squamous cell carcinoma (ESCC) (34,35). In this research, we delved into the potential of FAPI PET/CT as a prognostic tool for the therapeutic response in GISTs, assessing its role as either a supplementary or an alternative method to [18F]FDG PET/CT. Notably, the results of this study showed that SULpeak-FAPI and TLF-FAPI based on [18F]FAPI-42 PET/CT were higher in the NPG than that in the PG, which indicates that GISTs exhibiting lower FAP expression are more likely to develop resistance to targeted therapy. This is opposite to the previous study, indicating that the higher the baseline ratio of tumor SUVmax to bood pool SUVmax, the worse the response to chemoradiotherapy and PD-1 inhibitors (34,36). Therefore, our findings, apart from confirming the predictive value of FAPI PET/CT for the response of GISTs, could have a novel that high FAPI uptake (a high level of FAP) does not always induce resistance to the therapy.
Notably, model 3 incorporating only FDG PET/CT parameters and model 4 incorporating only FAPI PET/CT parameters had similar predictive capabilities. To ascertain the optimal utilization of these parameters for enhanced predictive accuracy, we explored the model based on both PET/CT parameters and combined clinical parameters by comparing the value of four models in predicting the response of recurrent or metastatic GISTs and constructed the nomogram based on the best model. The value of combined FDG PET/CT and FAPI PET/CT for predicting the response of recurrent and metastatic GISTs was better than FDG PET/CT or FAPI PET/CT alone. It is widely acknowledged that the efficacy of targeted drugs in patients with GISTs is related to gene mutation type. Further, our study suggested that the performance of the model incorporating TBR-FDG, SULpeak-FAPI, gene mutation, and the targeted drug was superior to the model incorporating FDG PET/CT and FAPI PET/CT parameters alone, which could yield more precise predictions of the response of recurrent metastatic GISTs to targeted therapy.
There are several limitations to this study that need to be addressed. Firstly, the sample size was inevitably small. Secondly, the evaluation was focused on lesions rather than patients, which may be relevant for local recurrence or metastasis but may not provide predictive value for overall patient survival outcomes. Thirdly, the model was not externally validated with a data set in another institution. To overcome these limitations, it is essential to expand the sample size and optimize the model, assess the response of patients, analyze its predictive value on patient prognosis, and conduct multicenter studies for external validation of the model.
Conclusions
Both baseline [18F]FAPI-42 PET/CT and [18F]FDG PET/CT had predictive value in assessing treatment responses for recurrent or metastatic GIST lesions. Furthermore, the combined predictive capacity of FAPI and FDG PET imaging enhances the precision of response predictions, surpassing the capabilities of each method individually. The model integrating baseline [18F]FAPI-42 PET/CT, [18F]FDG PET/CT parameters, gene mutation type, and targeted therapy yielded the most accurate predictions for recurrent metastatic GISTs.
Acknowledgments
Funding: None.
Footnote
Conflicts of Interest: All authors have completed the ICMJE uniform disclosure form (available at https://qims.amegroups.com/article/view/10.21037/qims-24-192/coif). The authors have no conflicts of interest to declare.
Ethical Statement: The authors are accountable for all aspects of the work in ensuring that questions related to the accuracy or integrity of any part of the work are appropriately investigated and resolved. The study was conducted in accordance with the Declaration of Helsinki (as revised in 2013). The study was approved by the Ethics Committee of The First Affiliated Hospital of Sun Yat-sen University (Ethics Review [2022] No. 257). Written informed consent was obtained from each patient prior to each PET/CT scan.
Open Access Statement: This is an Open Access article distributed in accordance with the Creative Commons Attribution-NonCommercial-NoDerivs 4.0 International License (CC BY-NC-ND 4.0), which permits the non-commercial replication and distribution of the article with the strict proviso that no changes or edits are made and the original work is properly cited (including links to both the formal publication through the relevant DOI and the license). See: https://creativecommons.org/licenses/by-nc-nd/4.0/.
References
- Serrano C, Martín-Broto J, Asencio-Pascual JM, López-Guerrero JA, Rubió-Casadevall J, Bagué S, García-Del-Muro X, Fernández-Hernández JÁ, Herrero L, López-Pousa A, Poveda A, Martínez-Marín V. 2023 GEIS Guidelines for gastrointestinal stromal tumors. Ther Adv Med Oncol 2023;15:17588359231192388. [Crossref] [PubMed]
- Vallilas C, Sarantis P, Kyriazoglou A, Koustas E, Theocharis S, Papavassiliou AG, Karamouzis MV. Gastrointestinal Stromal Tumors (GISTs): Novel Therapeutic Strategies with Immunotherapy and Small Molecules. Int J Mol Sci 2021;22:493. [Crossref] [PubMed]
- Iwatsuki M, Harada K, Iwagami S, Eto K, Ishimoto T, Baba Y, Yoshida N, Ajani JA, Baba H. Neoadjuvant and adjuvant therapy for gastrointestinal stromal tumors. Ann Gastroenterol Surg 2019;3:43-9. [Crossref] [PubMed]
- Musa J, Kochendoerfer SM, Willis F, Sauerteig C, Harnoss JM, Rompen IF, Grünewald TGP, Al-Saeedi M, Schneider M, Harnoss JC. The GIST of it all: management of gastrointestinal stromal tumors (GIST) from the first steps to tailored therapy. A bibliometric analysis. Langenbecks Arch Surg 2024;409:95. [Crossref] [PubMed]
- Wada N, Takahashi T, Kurokawa Y, Nakajima K, Nishida T, Koh M, Akamaru Y, Motoori M, Kimura Y, Tanaka K, Miyazaki Y, Makino T, Yamasaki M, Eguchi H, Doki Y. Clinical significance of surgical intervention for imatinib-resistant gastrointestinal stromal tumors in the era of multiple tyrosine kinase inhibitors. Surg Today 2021;51:1506-12. [Crossref] [PubMed]
- Casali PG, Blay JY, Abecassis N, Bajpai J, Bauer S, Biagini R, et al. Gastrointestinal stromal tumours: ESMO-EURACAN-GENTURIS Clinical Practice Guidelines for diagnosis, treatment and follow-up. Ann Oncol 2022;33:20-33. [Crossref] [PubMed]
- Hu X, Wang Z, Su P, Zhang Q, Kou Y. Advances in the research of the mechanism of secondary resistance to imatinib in gastrointestinal stromal tumors. Front Oncol 2022;12:933248. [Crossref] [PubMed]
- Demetri GD, Garrett CR, Schöffski P, Shah MH, Verweij J, Leyvraz S, Hurwitz HI, Pousa AL, Le Cesne A, Goldstein D, Paz-Ares L, Blay JY, McArthur GA, Xu QC, Huang X, Harmon CS, Tassell V, Cohen DP, Casali PG. Complete longitudinal analyses of the randomized, placebo-controlled, phase III trial of sunitinib in patients with gastrointestinal stromal tumor following imatinib failure. Clin Cancer Res 2012;18:3170-9. [Crossref] [PubMed]
- Blay JY, Kang YK, Nishida T, von Mehren M. Gastrointestinal stromal tumours. Nat Rev Dis Primers 2021;7:22. [Crossref] [PubMed]
- Blay JY, Serrano C, Heinrich MC, Zalcberg J, Bauer S, Gelderblom H, Schöffski P, Jones RL, Attia S, D'Amato G, Chi P, Reichardt P, Meade J, Shi K, Ruiz-Soto R, George S, von Mehren M. Ripretinib in patients with advanced gastrointestinal stromal tumours (INVICTUS): a double-blind, randomised, placebo-controlled, phase 3 trial. Lancet Oncol 2020;21:923-34. [Crossref] [PubMed]
- Shinagare AB, Jagannathan JP, Kurra V, Urban T, Manola J, Choy E, Demetri GD, George S, Ramaiya NH. Comparison of performance of various tumour response criteria in assessment of regorafenib activity in advanced gastrointestinal stromal tumours after failure of imatinib and sunitinib. Eur J Cancer 2014;50:981-6. [Crossref] [PubMed]
- Le Cesne A, Van Glabbeke M, Verweij J, Casali PG, Findlay M, Reichardt P, Issels R, Judson I, Schoffski P, Leyvraz S, Bui B, Hogendoorn PC, Sciot R, Blay JY. Absence of progression as assessed by response evaluation criteria in solid tumors predicts survival in advanced GI stromal tumors treated with imatinib mesylate: the intergroup EORTC-ISG-AGITG phase III trial. J Clin Oncol 2009;27:3969-74. [Crossref] [PubMed]
- Malle P, Sorschag M, Gallowitsch HJ. FDG PET and FDG PET/CT in patients with gastrointestinal stromal tumours. Wien Med Wochenschr 2012;162:423-9. [Crossref] [PubMed]
- Revheim ME, Hole KH, Mo T, Bruland ØS, Reitan E, Julsrud L, Seierstad T. Multimodal functional imaging for early response assessment in patients with gastrointestinal stromal tumor treated with tyrosine kinase inhibitors. Acta Radiol 2022;63:995-1004. [Crossref] [PubMed]
- Narushima K, Shuto K, Okazumi S, Ohira G, Mori M, Hayano K, Yanagawa N, Matsubara H. Malignant diagnosis and prognostic analysis of 89 GIST patients using preoperative FDG-PET. Sci Rep 2023;13:2266. [Crossref] [PubMed]
- Albano D, Mattia B, Giubbini R, Bertagna F. Role of 18F-FDG PET/CT in restaging and follow-up of patients with GIST. Abdom Radiol (NY) 2020;45:644-51. [Crossref] [PubMed]
- Hahn S, Bauer S, Heusner TA, Ebeling P, Hamami ME, Stahl A, Forsting M, Bockisch A, Antoch G. Postoperative FDG-PET/CT staging in GIST: is there a benefit following R0 resection? Eur J Radiol 2011;80:670-4. [Crossref] [PubMed]
- Choi H, Charnsangavej C, de Castro Faria S, Tamm EP, Benjamin RS, Johnson MM, Macapinlac HA, Podoloff DA. CT evaluation of the response of gastrointestinal stromal tumors after imatinib mesylate treatment: a quantitative analysis correlated with FDG PET findings. AJR Am J Roentgenol 2004;183:1619-28. [Crossref] [PubMed]
- Zboralski D, Hoehne A, Bredenbeck A, Schumann A, Nguyen M, Schneider E, et al. Preclinical evaluation of FAP-2286 for fibroblast activation protein targeted radionuclide imaging and therapy. Eur J Nucl Med Mol Imaging 2022;49:3651-67. [Crossref] [PubMed]
- Giesel FL, Kratochwil C, Lindner T, Marschalek MM, Loktev A, Lehnert W, Debus J, Jäger D, Flechsig P, Altmann A, Mier W, Haberkorn U. (68)Ga-FAPI PET/CT: Biodistribution and Preliminary Dosimetry Estimate of 2 DOTA-Containing FAP-Targeting Agents in Patients with Various Cancers. J Nucl Med 2019;60:386-92. [Crossref] [PubMed]
- Hamson EJ, Keane FM, Tholen S, Schilling O, Gorrell MD. Understanding fibroblast activation protein (FAP): substrates, activities, expression and targeting for cancer therapy. Proteomics Clin Appl 2014;8:454-63. [Crossref] [PubMed]
- Wu C, Zhang X, Zeng Y, Wu R, Ding L, Xia Y, Chen Z, Zhang X, Wang X. [18F]FAPI-42 PET/CT versus [18F]FDG PET/CT for imaging of recurrent or metastatic gastrointestinal stromal tumors. Eur J Nucl Med Mol Imaging 2022;50:194-204. [Crossref] [PubMed]
- Boellaard R, Delgado-Bolton R, Oyen WJ, Giammarile F, Tatsch K, Eschner W, et al. FDG PET/CT: EANM procedure guidelines for tumour imaging: version 2.0. Eur J Nucl Med Mol Imaging 2015;42:328-54. [Crossref] [PubMed]
- Rufibach K. Use of Brier score to assess binary predictions. J Clin Epidemiol 2010;63:938-9; author reply 939. [Crossref] [PubMed]
- Ayati N, Sadeghi R, Kiamanesh Z, Lee ST, Zakavi SR, Scott AM. The value of (18)F-FDG PET/CT for predicting or monitoring immunotherapy response in patients with metastatic melanoma: a systematic review and meta-analysis. Eur J Nucl Med Mol Imaging 2021;48:428-48. [Crossref] [PubMed]
- Zhu K, Su D, Wang J, Cheng Z, Chin Y, Chen L, Chan C, Zhang R, Gao T, Ben X, Jing C. Predictive value of baseline metabolic tumor volume for non-small-cell lung cancer patients treated with immune checkpoint inhibitors: A meta-analysis. Front Oncol 2022;12:951557. [Crossref] [PubMed]
- Breen WG, Hathcock MA, Young JR, Kowalchuk RO, Bansal R, Khurana A, Bennani NN, Paludo J, Villasboas Bisneto JC, Wang Y, Ansell SM, Peterson JL, Johnston PB, Lester SC, Lin Y. Metabolic characteristics and prognostic differentiation of aggressive lymphoma using one-month post-CAR-T FDG PET/CT. J Hematol Oncol 2022;15:36. [Crossref] [PubMed]
- Tao X, Li N, Wu N, He J, Ying J, Gao S, Wang S, Wang J, Wang Z, Ling Y, Tang W, Zhang Z. The efficiency of (18)F-FDG PET-CT for predicting the major pathologic response to the neoadjuvant PD-1 blockade in resectable non-small cell lung cancer. Eur J Nucl Med Mol Imaging 2020;47:1209-19. [Crossref] [PubMed]
- Hwang SH, Jung M, Jeong YH, Jo K, Kim S, Wang J, Cho A. Prognostic value of metabolic tumor volume and total lesion glycolysis on preoperative (18)F-FDG PET/CT in patients with localized primary gastrointestinal stromal tumors. Cancer Metab 2021;9:8. [Crossref] [PubMed]
- Zhang YY, Song L, Zhao MX, Hu K. A better prediction of progression-free survival in diffuse large B-cell lymphoma by a prognostic model consisting of baseline TLG and %ΔSUV(max). Cancer Med 2019;8:5137-47. [Crossref] [PubMed]
- Hofheinz F, Bütof R, Apostolova I, Zöphel K, Steffen IG, Amthauer H, Kotzerke J, Baumann M, van den Hoff J. An investigation of the relation between tumor-to-liver ratio (TLR) and tumor-to-blood standard uptake ratio (SUR) in oncological FDG PET. EJNMMI Res 2016;6:19. [Crossref] [PubMed]
- Wang C, Zhao K, Hu S, Huang Y, Ma L, Li M, Song Y. The PET-Derived Tumor-to-Liver Standard Uptake Ratio (SUV (TLR)) Is Superior to Tumor SUVmax in Predicting Tumor Response and Survival After Chemoradiotherapy in Patients With Locally Advanced Esophageal Cancer. Front Oncol 2020;10:1630. [Crossref] [PubMed]
- Chiaravalloti A, Danieli R, Abbatiello P, Di Pietro B, Travascio L, Cantonetti M, Guazzaroni M, Orlacchio A, Simonetti G, Schillaci O. Factors affecting intrapatient liver and mediastinal blood pool 18F-FDG standardized uptake value changes during ABVD chemotherapy in Hodgkin's lymphoma. Eur J Nucl Med Mol Imaging 2014;41:1123-32. [Crossref] [PubMed]
- Hu X, Zhou T, Ren J, Duan J, Wu H, Liu X, Mu Z, Liu N, Wei Y, Yuan S. Response Prediction Using (18)F-FAPI-04 PET/CT in Patients with Esophageal Squamous Cell Carcinoma Treated with Concurrent Chemoradiotherapy. J Nucl Med 2023;64:625-31. [Crossref] [PubMed]
- Zhao L, Pang Y, Chen S, Chen J, Li Y, Yu Y, Huang C, Sun L, Wu H, Chen H, Lin Q. Prognostic value of fibroblast activation protein expressing tumor volume calculated from [68 Ga]Ga-FAPI PET/CT in patients with esophageal squamous cell carcinoma. Eur J Nucl Med Mol Imaging 2023;50:593-601. [Crossref] [PubMed]
- Rong X, Lv J, Liu Y, Wang Z, Zeng D, Li Y, Li S, Wu J, Shen Z, Shi M, Liao W, Wu Z, Wang C. PET/CT Imaging of Activated Cancer-Associated Fibroblasts Predict Response to PD-1 Blockade in Gastric Cancer Patients. Front Oncol 2021;11:802257. [Crossref] [PubMed]