Research on ultrasound-based radiomics: a bibliometric analysis
Introduction
The importance of medical imaging in clinical practice has increased significantly by virtue of its noninvasive ability to provide reproducible information pertaining to disease processes, Among the traditional medical imaging modalities, ultrasound has the advantages of being economical, radiation-free, and widely used and has a diversity of imaging modalities and the ability to realize real-time image visualization and acquisition. However, the diagnostic results of ultrasound are highly reliant on the expertise of the operator, may be highly subjective, and lacks a quantitative analysis of the features contained in the image data (1). However, to overcome these uncertainties in diagnostic accuracy, quantitative analysis of the features of the imaging is necessary.
The concept of radiomics was first introduced in 2012 and can be defined as the high-throughput extraction of imaging features from radiographic images (2). These data consist of microscopic features that may be difficult to discern by the naked eye but which can be recognized and quantitatively analyzed by computers to construct diagnostic models (3).
Ultrasound-based radiomics, also known as ultrasomics, is a rapidly growing field, but due to limitations of image instability, the inability to show more than a single section of a lesion, inconsistency in equipment and parameters, and operator dependence, the emergence of ultrasomics has been relatively subdued compared to that other imaging techniques (4). Innovations in medical devices, imaging agents, and imaging analysis and the development of standardized protocols that allow for quantitative imaging have made it possible to obtain genomic and proteomic information from a variety of medical images (2). The combination of ultrasound and radiomics has been investigated in the fields of thyroid (5), breast (6), liver (7), prostate (8), and lung (9) cancer. The addition of multimodal ultrasound, deep learning, and migration learning has further improved the diagnostic efficiency of ultrasonic predictive modeling. The achievements of ultrasomics have inspired further research into this modality, with the number of publications rapidly growing in recent years.
Bibliometrics is an analytic technique that applies commonly used quantitative assessment methods (10), such as mathematics and statistics, to investigate patterns in literature distribution and quantitative relationships. More specifically, bibliometrics collects data on publication counts, citation analysis, citation metrics (e.g., h-index and g-index) etc., which are then examined via publication analysis, collaborative networks, cocitation analysis, keyword analysis, etc. This study relied on CiteSpace (version 6.2) (11), VOSviewer (version 1.6.20) (12), and the “bibliometrix” package in R for the optimization of literature analytics.
The research into ultrasomics is accelerating and diversifying, with this proliferation into specialized fields yielding a boom in the number of publications; therefore, it may be difficult for researchers to stay abreast of the latest advancements in the field and to select research topics of interest, and thus a comprehensive review of the current outlook and trends in this field may be highly beneficial. Bibliometrics, as an emerging tool for publishing analytics, can allow scholars to quickly understand the nuances and emerging trends in a particular area via quantitative analysis and visualization (13). We thus used bibliometric methods to analyze the published literature on ultrasomics, including information on publication, author, country, institution, keywords, and references. These data were then visualized so as to provide a convenient summary, and the development process, trends, hotspots, and frontiers of the research in this field were defined, providing a reference basis for orienting future work in ultrasomics.
Methods
Strategies for literature retrieval
The Web of Science Core Collection (WoSCC) is one of the most comprehensive and authoritative academic information database platforms in the world (14,15) and is thus widely used in bibliometric research (16,17). In addition, the data on WoSCC can be read directly by the bibliometric software CiteSpace and VOSviewer without the need for tedious format conversion.
Literature retrieval in this study was carried out in the WoSCC database with a time span of publication dates from 2016 to 2023. The search formula was as follows: TS = (ultrasound OR ultrasonographic OR ultrasonic OR echotomography OR ultrasomics) AND TS = (radiomic*) AND PY = (2016–2023). The criteria for literature inclusion were as follows: (I) ultrasomics as the topic of study, (II) articles and reviews, and (III) English-language literature. Meanwhile, the exclusion criteria were as follows: (I) conference abstracts, correction, letters, early-access papers, and proceedings papers; (II) literature not published between January 2016 and November 2023; and (III) a subject other than ultrasomics. The literature search was undertaken by two individuals concurrently, and all publications retrieved were assessed and filtered. A third researcher was invited to discuss and arrive at a consensus in case of disagreement between the two researchers in terms of literature screening, data extraction, and quality evaluation. The flowchart of literature selection and analysis is shown in Figure 1.
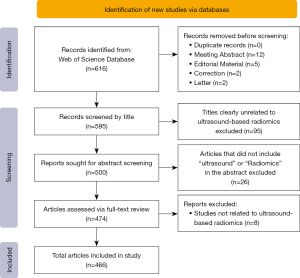
Data analysis
All the data collected from WoSCC were converted into text containing information on authors, research institutions, topics, years, keywords, abstracts, journals, volumes, page numbers, etc. Excel (Microsoft Corp., Redmon, WA, USA) was used to organize the data and generate graphs on publication trends. The “ggplot2” and “bibliometrix” packages in R and Scimago Graphica were used to visualize and analyze the source journals, countries, and institutions of publications.
CiteSpace (version 6.2) and VOSviewer (version 1.6.2) were applied for bibliometric and visualization analysis of the included literature. In bibliometric mapping, each node (or vertices) represents an individual object (18), such as a country, an organization, an author, or a keyword, while node size reflects the frequency of occurrence, with larger nodes indicating a greater frequency. Edges between the nodes represent the collaboration or co-occurrence relationships (18). CiteSpace was originally developed by professor Chaomei Chen and has unique advantages for bibliometric analysis. In CiteSpace, betweenness centrality is a significant parameter and refers to the number of times a node acts as the shortest bridge between two other nodes. The more times a node acts as an “intermediary”, the greater its intermediary centrality, which is an indicator of the importance or influence of a node in the network (19). It is commonly considered that a centrality ≥0.1 indicates an important node and that it is acting as a bridge in network structure; this node type of node is marked by a purple circle in the visualization map. In addition, CiteSpace was used to provide characterization and visualization support for keyword and reference burst analysis for trend identification in this study. VOSviewer is another popular software tool used for mapping bibliometric networks. It has a more user-friendly interface than does CiteSpace, but it does not have the advantage of clustering and analyzing the literature, nor does it have the same level of operability. Therefore, we combined several different analytical tools; VOSviewer was mainly used for the analysis of publication sources, institutions and countries, authors, and references; CiteSpace was primarily used for the clustering analysis of keywords and references; and other graphing tools were employed to refine the content in order to achieve the best presentation of the visualization results.
Data processing
For the analysis of keywords, we imported the data into VOSviewer and then exported the table of keywords from to R software for subsequent visualization and analysis. In managing the data, we manually merged the synonyms to ensure the accuracy of the keyword occurrence frequency. We also noticed that in traditional bibliometric studies, authors’ names in databases or often misspelled, and so we applied a manual review to identify and correct possible spelling errors to ensure the accuracy of the results.
Results
Publishing trends
A total of 617 documents related to ultrasomics published from January 2016 to November 2023 were searched in WoSCC; of these, 595 were retained according to the search criteria, and then 129 were discarded due to not being relevant to the research content according to the screening of titles, abstracts, and keywords. Ultimately, 466 publications were obtained that met the requirements, of which 85 were articles and 381 were reviews. Figure 2A shows the curve of the number of publications, including the annual number and the cumulative number of documents. The whole publication period could be divided into three parts: The first phase was 2016–2017, during which the annual publications were less than 5 per year, with radiomics still being exploratory stage and yet to be refined. The second phase was 2018–2021, during which the number of annual publications increased slightly but still did not surpass 100, with research in the field gradually transitioning from theory to practical application. The third phase was after 2022, when the number of publications exceeded 100, representing a rapid acceleration in growth compared with previous stages; the average annual number of publications at this stage increased by more than 281% compared with the same period of the previous stage and maintained a high level, suggesting the gradual maturity of the research technology and the emergence of the first high production phase. We also regressed the annual cumulative number of publications to discern the pattern of change over time, and the results showed a growth trend of multiplicative power, suggesting that ultrasomics is an area of intense research interest.
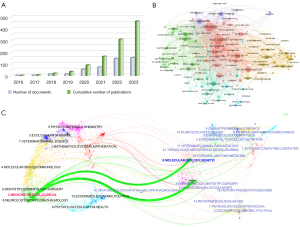
Productive journals
The journals of the publications were visualized using the “bibliometrix” package in R (version 4.1.3). All the articles included in this review were published across 176 journals, of which 9 had more than 10 articles. The most prolific journal was Frontiers in Oncology (60 articles), followed by Cancers (26 articles) and European Radiology (26 articles). Table 1 displays the academic journals that ranked in the top 10 in terms of number of publications, with the highest ranking in terms of impact factor being European Radiology (5.9), followed by Frontiers in Endocrinology (5.2). Figure 2B describes the network of cocited journals’ connections; out of 2,825 cocited journals, the most cited is Radiology (1,069), followed by European Radiology (1,013) and European Journal of Radiology (475). Moreover, we drew dual-visualizations maps to clarify the association between studies and disciplines. The citation relationship between journals and cocited journals is shown in Figure 2C, with clusters of citing journals on the left and groups of cited journals on the right. The map identifies two main links, with the green path being the main citation path, which can be interpreted as indicating that literature categorized as molecular, biology, genetics and health, nursing, and surgery are the primary references for the literature categorized as medicine, medical, and clinical.
Table 1
Rank | Journal | Articles, n |
---|---|---|
1 | Frontiers in Oncology | 60 |
2 | Cancers | 26 |
3 | European Radiology | 26 |
4 | Diagnostics | 19 |
5 | Journal of Ultrasound in Medicine | 16 |
6 | European Journal of Radiology | 12 |
7 | Scientific Reports | 12 |
8 | Frontiers in Endocrinology | 11 |
9 | BMC Medical Imaging | 10 |
10 | Abdominal Radiology | 9 |
Distribution of country/region and institution
A total of 43 countries/regions and institutions participated in the publication of ultrasomics papers. The nodes in the visualization map represent the countries/regions or institutions, and the connecting lines represent the cooperation between them; the size of the nodes is positively correlated with the number of publications, whereas the thickness of the connecting lines represents the closeness of the cooperation relationship between the countries/regions or institutions. By visualizing the number of publications and analyzing the number of citations, it is possible to ascertain the countries/regions and the institutions that have a significant advantage in this domain in order to understand the popularity of this topic on a world-wide scale. This can help to identify researchers or institutions with similar interests or expertise and to highlight potential collaborators or research partners (12).
Countries
We first obtained the converted data in VOSviewer, using Scimago Graphica to draw the country/region cooperation network diagram (Figure 3A). A total of 43 nodes are in the graph. The larger the node indicates that the country has a greater number of papers published; the greater number of linked lines indicates a greater number of internationally collaborative studies performed by the country, and the width of the line represents the closeness of the intercountry collaboration. As seen in the graph in Figure 3, China published the largest volume of literature, followed by the United States and Italy. The countries with the highest number of articles published are shown in Table 2. The results suggest that ultrasomics is a popular theme globally, with significant literature output in various regions around the globe. In terms of citation analysis by country/region, the top three countries/regions in the list of cited counts are China (n=3,273), the United States (n=1,386), and Italy (n=756), respectively, among which China occupies the most important position in the national collaborative network and has close collaborative relationships with neighboring countries.
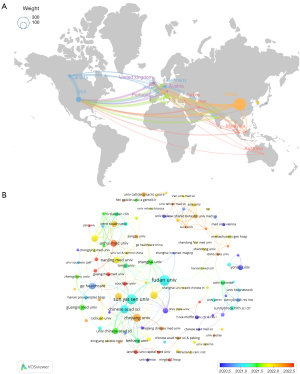
Table 2
Rank | Country | Documents, n | Citations, n |
---|---|---|---|
1 | China | 298 | 3,273 |
2 | USA | 57 | 1,386 |
3 | Italy | 44 | 756 |
4 | The Netherlands | 15 | 356 |
5 | Canada | 19 | 310 |
6 | South Korea | 17 | 250 |
7 | United Kingdom | 16 | 245 |
8 | Switzerland | 13 | 199 |
9 | Germany | 17 | 195 |
10 | Malaysia | 5 | 121 |
Institutions
A total of 696 institutions were identified in the survey, and the top five most productive institutions were Fudan University, Sun Yat-sen University, the Chinese Academy of Sciences, Zhejiang University, and Shanghai Jiao Tong University. Fudan University, which had the highest productivity, published 41 documents. We also conducted h-index analysis of the institutions, and the results showed that Sun Yat-sen University had the highest h-index (18), followed by Fudan University (17). Among the top 10 institutions in h-index, the institution with the highest average number of citations was Institute of Automation, Chinese Academy of Sciences (28.54). The institutional cooperation network diagram (Figure 3B) was obtained by using VOSviewer. It demonstrated that the cooperation between different institutions within China was intense, among which Fudan University, with the highest number of publications, established close cooperation with Sun Yat-sen University, Zhejiang University, and Shanghai Jiao Tong University, among others, whereas the cooperation with institutions abroad was relatively lower; therefore, there are development opportunities for greater cooperation between institutions across different regions. Over time, newly established laboratories have emerged, indicating that ultrasomics has broad prospects for development.
Authors and cocited authors
A total of 2,726 authors were included in the survey for the statistical investigation. We constructed a collaborative network, as shown in Figure 4A, based on the authors who published more than or equal to 3 articles, where the authors’ publications are represented as nodes of different sizes on the graph, and the connecting lines indicate the collaborative relationship between the authors, with the thicker connecting lines indicating a closer coordination between the two authors. Among the top 20 authors, none published fewer than 10 articles, with the most prolific author being Yuanyuan Wang, who published 19 papers over an 8-year span, followed by Wei Wang, has published 16 papers. This shows that these researchers produced significant achievements in this domain; in addition, we also conducted an author cocitation analysis, filtering authors with a minimum repeat count of 15 to draw an author cocitation network diagram (Figure 4B), with 141 authors with more than 15 cocitations. The connecting lines of different widths in the graph represent the cocitation relationship between authors, and the most cocited author is Lambin P (n=233), followed by Gillies RJ (n=187) and van Griethuysen JJM (n=80), suggesting that these articles are a solid theoretical foundation for further investigation.
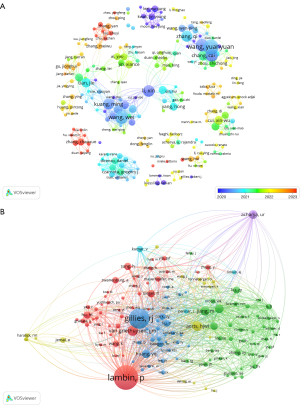
Reference cocitation analysis
There were 15,692 references included in the cocitation analysis, which is commonly used to identify research priorities. In addition, reference burst analysis allows readers to understand the literature in the area of interest for a given period of time. It can provide the researcher with a body of the relevant work in a field and be used to determine the future orientation of research.
Cocitation
Out of the 15,692 cocited documents found, the top 10 most frequently cited papers are shown in Table 3. Gillies RJ’s “Radiomics: images are more than pictures, they are data” published in Radiology was the most frequently cited article with 175 citations, followed by Lambin P’s “Radiomics: extracting more information from medical images using advanced feature analysis”, with 119 citations. In addition, we also performed a cluster analysis of the reference cocitation network diagram, and all nodes could be classified into 12 major clusters, as seen in Figure 5A, the references of which are detailed in Table S1. The clusters were as follows: #0, papillary thyroid cancer; #1 breast cancer; #2, hepatocellular carcinoma (HCC); #3, artificial intelligence; #4, potential biomarkers; #5, biological behavior; #6, comparative assessment; #7, neoadjuvant chemotherapy; #8, head and neck squamous carcinoma; #9, probing; #10, urology; and #11, ultrasound. The clusters with the stronger centrality were papillary thyroid cancer, biological behavior, potential biomarkers, and comparative assessment, suggesting that the studies in these areas serve a bridging role between newer and older research. According to the timeline, it can be speculated that the current frontiers of the field are breast, thyroid, and hepatocellular cancers, as well as their associated neoadjuvant chemotherapies, with the research focus gradually shifting from technology and algorithms to specific clinical applications.
Table 3
Rank | Title | Author | Citations, n |
---|---|---|---|
1 | Radiomics: images are more than pictures, they are data (doi: 10.1148/radiol.2015151169) |
Robert J. Gillies et al. (20) | 175 |
2 | Radiomics: extracting more information from medical images using advanced feature analysis (doi: 10.1016/j.ejca.2011.11.036) | Philippe Lambin et al. (2) | 119 |
3 | Radiomics: the bridge between medical imaging and personalized medicine (doi: 10.1038/nrclinonc.2017.141) | Philippe Lambin et al. (21) | 110 |
4 | Computational radiomics system to decode the radiographic phenotype (doi: 10.1158/0008-5472.CAN-17-0339) | Joost J. M. van Griethuysen et al. (22) | 80 |
5 | Decoding tumour phenotype by noninvasive imaging using a quantitative radiomics approach (doi: 10.1038/ncomms5006) | Hugo J. W. L. Aerts et al. (23) | 57 |
6 | Radiomics analysis on ultrasound for prediction of biologic behavior in breast invasive ductal carcinoma (doi: 10.1016/j.clbc.2017.08.002) | Yi Guo et al. (24) | 48 |
7 | Development and validation of a radiomics nomogram for preoperative prediction of lymph node metastasis in colorectal cancer (doi: 10.1200/jco.2015.65.9128) | Yan-Qi Huang et al. (25) | 47 |
8 | Radiomics: the process and the challenges (doi: 10.1016/j.mri.2012.06.010) | Virendra Kumar et al. (26) | 43 |
9 | Ultrasound-based radiomics score: a potential biomarker for the prediction of microvascular invasion in hepatocellular carcinoma (doi: 10.1007/s00330-018-5797-0) | Hang-Tong Hu et al. (27) | 43 |
10 | 2015 American Thyroid Association Management Guidelines for Adult Patients with Thyroid Nodules and Differentiated Thyroid Cancer: The American Thyroid Association Guidelines Task Force on Thyroid Nodules and Differentiated Thyroid Cancer (doi: 10.1089/thy.2015.0020) | Bryan R. Haugen et al. (28) | 42 |
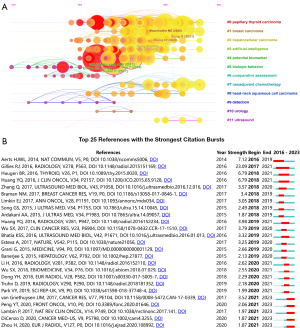
Reference burst
In addition, we identified the top 25 papers in terms of strongest citation explosion based on the citation explosion value (Figure 5B, with references in Table S2). The first citation burst began in 2016, and the strength of the citation bursts of the top 25 papers ranged from 1.87 to 23.09. The strongest and longest-lasting citation burst was from an article published by Gillies et al. (20) in the journal Radiology, entitled “Radiomics: images are more than pictures, they are data”. This paper explains the concept of radiomics, the basic process of performing radiomics, the key points of each step, and the continuing difficulties faced, aiming to encourage more radiologists to engage in radiomic studies and to create more high-quality clinical decision support tools. Finally, the authors summarize the current status of the development in radiomics and their outlook on the future of the discipline, suggesting that radiomics is an approach that holds great promises but needs further validation in a multicenter setting and in laboratory studies (2).
Keyword analysis
Keywords are those words or phrases chosen for the purpose of literature citation and retrieval to reflect the topic of the essay, representing a highly condensed expression of the study subject. By analyzing the keywords, we can discern the core components and research hotspots of a given field, and by clustering, we can highlight the key nodes and important connections; overall, keyword analysis can reveal the major themes in a given field and track its evolution.
Co-occurrence
Keyword cooccurrence identifies recurring themes, topics, and subject areas in the literature; maps knowledge networks; aids researchers in identifying emerging trends; and facilitates collaboration. Meanwhile, co-occurrence analysis provides a systematic approach to identifying relevant literature and key concepts in a given field of study, which helps in the writing of a literature review (29,30). Figure 6A shows the 20 most frequently occurring keywords, from which the overall research in ultrasomics can be classified the into two general areas. One is the study of the technical operations, and includes keywords such as machine learning, deep learning, artificial intelligence, features, texture features, classifiers, and nomograms. The other is the study of the clinical applications of ulstrasomics models and includes keywords such as diagnostic, predictive, managerial, recurrence, neoplastic, nodal, and benign. In addition, we used the “ggplot2” software package in R to produce heat maps of the top 20 keywords with the highest frequency of occurrence in different time periods (Figure 6B), which were normalized to the frequency of occurrence and displayed as a tube chart with different colors. In Figure 6, the brighter the grid color is, the higher the frequency of the keyword in that year, and it can be seen that a greater number of keywords appears as the time passes, which indicates that the field of research has gradually broadened and become multidirectional. Around 2016, ultrasomics was in its infancy, and the related research mainly concerned texture analysis, the theory of radiomics, and prostate cancer; in 2020, with the greater depth of theoretical research and the increasing maturity of practical technology, the keywords changed, and the amount of literature related to joint diagnosis of ultrasound imaging and radiomics proliferated, with the main keywords being diagnosis, lymph node, and thyroid; after 2020, the evolution of new algorithms and the upgrading of equipment provided a solid foundation for the development of ultrasomics, and the research focus broadened and diversified.
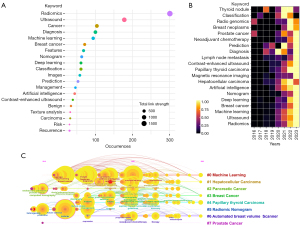
Keyword clusters
According to the cluster analysis of the keywords, a visualization network of keyword clustering was constructed, as shown in Figure 6C. The keywords could be approximately divided into 8 categories: #0, machine learning; #1, hepatocellular carcinoma; #2, adenocarcinoma; #3, breast cancer; #4, papillary thyroid cancer; #5, nomograms for radiomics; #6, automated breast volume scanner; and #7, prostate cancer. The keyword with the earliest time of emergence was machine learning, which is a feature extraction algorithm based on radiomics and served as the theoretical foundation for the early development of radiomics and commonly used algorithmic models. The research areas that are increasing in popularity continue to be diagnosis, prognosis, and evaluation of the efficacy of diseases such as thyroid cancer, prostate cancer, and HCC.
Discussion
The results of the bibliometrics analysis suggest that radiomics has been developing rapidly in recent years, with a surge in the number of articles and citations to related journals. Among them, Frontiers in Oncology is the journal with the highest number of publications. Moreover, China was the most prolific country in this regard, followed by the United States and Italy. The leading three institutions in terms of published papers were Fudan University, Sun Yat-sen University, and the Chinese Academy of Sciences, all of which are Chinese science institutions, reflecting China’s leading role in this study area. Yuanyuan Wang was found to be the author with the highest number of publications, with a total of 19 articles published over 8 years, and Philippe Lambin was the most cited author with 233 citations. Visualization of the cocitation analysis of the literature showed that the current foci in this field are papillary thyroid carcinoma (PTC), biological behavior, potential biomarkers, and comparative assessment, among others. The co-occurrence analysis of the keywords allowed us to summarize the core theme of our study—ultrasomics—into two general categories. We could clearly summarize the entire research content of ultrasound-based radiomics into two aspects: one is the study the of the technical imaging, including image acquisition and segmentation, feature extraction and screening, and artificial intelligence model construction; the second is the exploration of typical clinical applications of ultrasomics models in tumor diagnosis, efficacy evaluation, prognosis, and survival prediction. The detailed findings are discussed in the subsequent sections.
Preparation of radiomics models
Radiomics is the practice in which images are transformed into data that can be mined and subsequently analyzed for decision support (20). These data are often combined with other patient information, such as genomics, proteomics, and other clinical biochemical metrics, to develop models that may improve diagnostic, prognostic, and predictive accuracy. Ultrasomics is a branch of radiomics, which can extract a large number of quantitative features from ultrasonic images, including texture, shape, intensity, trend, and wavelet features, etc., which represent different pathological types of diseases, and provide clinicians with comprehensive quantitative phenotypes to help clinical decision-making (26). The application of ultrasomics has been gradually broadened by the updating of ultrasonic technology and the swift development of computerized algorithms, with studies now being conducted in the areas of thyroid (5), breast (31), liver (32), prostate (33), and head and neck tumors (34).
The process of radiomics mainly includes image acquisition and reconstruction, image preprocessing, region of interest (ROI) identification, feature extraction, and quantization, feature filtering, and prediction modeling (20).
Image acquisition and segmentation
Images are the base of ultrasomics, from which all radiomic features are extracted. The collection of image samples can be prospective or retrospective. While prospective collection maximizes image consistency, multicenter prospective studies require clear agreements between participating institutions and standardized practices. Retrospectively collected data must be reconstructed due to the lack of standardization prior to collection (35). Moreover, ultrasound has multiple imaging modalities, and multimodal ultrasomics should be able to solve the problem of matching the pixel points in different imaging modalities (33). Machine learning algorithms require labeling of ROIs, and the current approaches to ROI segmentation can be broadly characterized into three types: automatic segmentation, semiautomatic segmentation, and manual segmentation. The common segmentation algorithms include region growing, level setting, image cutting, active contour (snake) algorithms, semiautomatic segmentation, and livewire methods (35). The method of segmentation and location of ROIs are not fixed but are ultimately determined according to the purpose of the study and the reliability of the results, which can be the tumor, tumor subregions, metastases, paracancerous tissues, or even normal tissues (4). The image quality of ultrasound imaging for feature extraction is highly reliant on the operator, the performance of the equipment, and the accuracy of the delineation of the ROI, however, it is still a great challenge to unifying the image acquisition standard and the parameters of the equipment and eliminate the differences in the results caused by the manual delineation; therefore, the establishment of a unified and standardized image acquisition standard and the development of semiautomatic ROI delineation tools is a hotspot of ultrasomics research.
Feature extraction and filtering
At the nucleus of radiomics is the extraction of high-dimensional feature data used to quantitatively characterize the values of the properties of the volume of interest (20), which can be broadly classified into shape, first-order grayscale histogram, second-order and higher-order texture features, and other features based on filtering and transformations (4). Form-based features, known as semantic features, include summary statistics describing the volume or size of the region (35). First-order features are summary statistics of the histogram of grayscale signal intensity values obtained from an image within a given region and thus are also known as histogram features (36). Second-order features are also often described as texture features, and they describe statistical interrelationships between voxels with similar (or different) contrast values (20). Texture-feature extraction methods often used in medical ultrasound image analyzation include gray-level co-occurrence matrix (GLCM), histogram of oriented gradients (HOG), and local binary pattern (LBP), among others (37). Higher-order features include filters and higher-order images to describe metrics (35). It has been argued that higher-order features and second-order texture features can, to some extent, provide useful information about the heterogeneity within a tumor, thereby improving the efficiency of tumor diagnosis and prediction of treatment response (38). Ultrasound images contain a massive number of features, and establishing a link between the extracted second-order and higher-order features and tumor heterogeneity for better diagnosis and assessment of tumor characteristics is a future line of investigation.
Building artificial intelligence models
Artificial intelligence has shown strong productivity in other disciplines (39) and is gaining ground in studies related to ultrasomics (40). Marya et al. used deep learning algorithms to develop a model that can accurately differentiate autoimmune pancreatitis from pancreatic ductal adenocarcinoma on endoscopic ultrasound images (41). Azizi et al. used a deep learning approach to capture advanced potential features of temporal-enhanced ultrasound for the early detection of prostate cancer (42). In addition, artificial intelligence has achieved satisfactory outcomes in the segmentation of medical images (43) and reconstruction of sonograms (44). The current learning algorithms of artificial intelligence used for model development in ultrasomics include traditional machine learning, deep learning, and transfer learning (4). Machine learning involves using algorithms that learn from and make forecasts about data (45) and includes supervised (46), semisupervised (47), and unsupervised (48) learning. Machine learning solves problems in classification, regression, clustering, and network analysis. The algorithms commonly used in machine learning include k-nearest neighbor, decision tree, random forest, support vector machine (SVM), logistic regression, linear discriminant analysis, multilayer perceptron, and Bayesian models (49). Deep learning is an algorithm in machine learning based on representation learning (50), which uses a variety of nonlinear ways to represent the observed object as a vector of intensity values per pixel or more abstractly as a series of edges, a region of a particular shape, etc. Neural networks (51,52) are a commonly used method in ultrasomics (53). The benefit of deep learning over machine learning is the use of unsupervised or semisupervised methods to extract efficient algorithms to replace the manual acquisition of features, which reduces the uncertainty of manual input and greatly compensates for the shortcomings of the poor robustness of ultrasound images (4). Deep learning’s mode of learning dictates the need for larger input data than that of traditional machine learning. Transfer learning and domain adaptation are processes in which the model learning in one task environment is used to enhance the generalization of models in another task environment (50). This allows for the acceleration and optimization the learning efficiency of the model without the need to start from scratch— as most networks do—and the circumvention of the overfitting problem caused by insufficient training data in traditional machine learning (4). Correctly selecting algorithms that match the size of the data and the characteristics of the disease being studied, constructing classifier models for several different algorithms, and selecting the model with the best classification are currently popular topics in radiologic research.
Applications of radiomics models
Medical imaging, which provides information about pathologic characteristics in a noninvasive, reproducible manner, has grown significantly in importance in clinical decision-making, evolving from a purely diagnostic tool to an essential facilitator of early screening, diagnosis, treatment planning, and disease surveillance (54). Depending on the department in which the predictive model is clinically applied, the application of the model can be described in two aspects: diagnosis and prediction.
Differential diagnosis
Ultrasomics models greatly improve the efficiency and accuracy of tumor diagnosis, which helps clinicians make personalized medical management plans, contributes to the early treatment of tumor patients, and improves the prognosis of patients. In their study, Căleanu et al. (7) extracted the time-intensity curves (TICs) of arterial and portal phases in contrast-enhanced ultrasound (CEUS) images for data mining and model development, using deep neural networks to diagnose liver lesions, which showed a high accuracy rate of 83.36% for benign-malignant differential diagnosis. Fujioka et al. (55) collected 480 images of 96 benign breast masses and 467 images of 144 malignant breast masses as training data. A deep learning model was constructed using the convolutional neural network (CNN) architecture GoogLeNet, and the results indicated that the model had the same or even better diagnostic performance than did that of a radiologist. Moreover, Wu et al. (56) simulated the typical diagnostic workflow used by radiologists to develop a user-friendly framework for the automated diagnosis of thyroid nodules in ultrasound videos. Most of the work in ultrasomics research is still limited to the manual selection of features from stationary images or dynamic videos or through selecting poorly interpretable algorithmic models; thus, in the future, the development of multitask learning frameworks and the enhanced interpretability of the models may emerge as key research objectives.
Disease classification and typing
Correct disease typing can inform the selection of clinical treatment options, and artificial intelligence-assisted ultrasound image analysis can discern the subtle differences between different types of disease and assist clinicians in clarifying the phenotype of the condition. Wang et al. (5) recruited 75 patients with no mutation in the BRAFV600E gene series and 63 patients with mutation in the BRAFV600E gene series and randomized them into a training group (n=96) and a test group (n=42). Based on gray-scale and elastic ultrasound images, 13 radiomics features were extracted, and the model had a superior predictive value for BRAFV600E gene mutations in patients with PTC. Jiang et al. (57) used a dataset of 4,828 ultrasound images from 1,275 patients with primary breast cancer as training samples to predict the four St. Gallen molecular subtypes using deep CNN and, secondarily, to identify luminal disease from nonluminal disease based on the ground truth of the immunohistochemical analysis of the whole tumor surgical specimen, both of which demonstrated excellent diagnostic performance. Different tumor subtypes vary greatly in presentation, treatment response, and survival outcomes, and since most of the recent studies on predictive phenotypes are retrospective, further prospective studies with large samples are still needed to demonstrate the reliability of the models.
Prediction of survival
The noninvasive prediction of patient prognosis can guide the modification of clinical management strategies. Liu et al. (58) constructed a radiofrequency ablation (RFA) and surgical resection (SR) nomogram by combining radiomic features and significant clinical variables for individualized 2-year progression-free survival (PFS) prediction, subsequently applying the radiomics model and the nomogram to each enrolled patient to investigate whether there was a possibility for therapeutic optimization and the magnitude of the expected prognostic improvement. The nomogram showed good predictive accuracy and ultimately benefited patients by changing treatment regimens based on predicted outcomes. Yao et al. (59) enrolled 111 patients with ovarian epithelial cancer (OEC) who underwent preoperative transvaginal ultrasound and were randomly divided into a training set and a test set. The ROI of the tumor was defined by manually drawing the tumor contour, and the results showed that patients with OEC had a good prognosis based on the radiomics features, which helped to establish a significant clinical variable for prediction of PFS at 5 years. Ultrasomics can more accurately predict PFS after clinical interventions, allowing clinicians to develop personalized treatment plans according to histological features from images. Presently, the bulk of images are single slices that best represent the nature of the tumor but cannot reflect the entire lesion and may overlook certain details; therefore, radiomics based on three-dimensional ultrasound may become an attractive area of research in the future.
Prediction of lymph node metastasis
Lymph node invasion is an important reference for the grading and staging of malignant tumors, and preoperative prediction of lymph node metastasis can affect the choice of surgical plan. An increasing number of studies have shown that ultrasomics modeling can noninvasively and accurately predict lymph node status. Chang et al. (60) recruited 3,359 patients with PTC who had undergone total thyroidectomy or thyroid lobectomy at two medical centers and used multivariate logistic regression to construct a comprehensive nomogram combining deep learning, clinical features, and ultrasound features to predict central lymph node metastasis (CLNM) in patients with PTC. The nomogram showed good predictive value and could help surgeons make appropriate surgical decisions in the treatment of PTC. Jiang et al. (61) extracted and selected relevant radiomic features for axillary lymph node (ALN) from shear wave elastography (SWE) and corresponding B-mode ultrasound (BMUS) images, performed proportional ratio sequential logistic regression using radiomics features and clinical data, and subsequently developed an ordinal nomogram for preoperative assessment load in early breast cancer, which provided incremental information for risk stratification that allowed for the more precise treatment based on a mold. Elastography has good predictive value in the diagnosis of solid tumors, and the combination of elastography with gray-scale ultrasound, CEUS, and clinical factors in constructing radiomics models may be promising avenue of research.
Prediction of recurrence
Ultrasomics models can provide clinicians with an easy-to-use, visual, and personalized tool that helps to predict tumor recurrence at an early stage and to act accordingly, which is important for obtaining good patient outcomes. Zhang et al.’s (62) retrospective study included 172 patients with HCC who underwent hepatic resection with at least 1 year of follow-up and partitioned the dataset. Based on CEUS, a hybrid model was constructed using the residual net 50 (ResNet-50) architecture and incorporating clinicopathologic features to predict early recurrence of HCC after hepatic resection, which was diagnostically more efficient than was the single-feature model. In another report, Wang et al. (63) retrospectively analyzed the clinical imaging data of 159 patients with pathologically confirmed prostate cancer who underwent radical prostatectomy and divided the patients into a biochemical recurrence (BCR) group (n=59) and a control group (n=100). They then established radiomics modeling based on CEUS, magnetic resonance imaging (MRI), and CEUS-combined with MRI, respectively, with the combined model demonstrating the best performance. These deep learning-based radiomics models have good performance in clinical practice, indicating that studies into the processing of ultrasound images using deep learning is a burgeoning trend in research.
Prediction and evaluation of therapeutic efficacy
Awareness of an individual’s likelihood of achieving pathologic complete remission (pCR) prior to treatment allows clinicians to develop individually optimized treatment plans. Cai et al. (64) retrospectively collected data from patients who underwent ultrasound and tomosynthesis prior to the initiation of neoadjuvant systemic therapy (NAST), evaluated and compared different models based on input variables, and ultimately proposed a multimodal machine learning algorithm with pretreatment clinical and ultrasomics features to predict the response to neoadjuvant breast cancer therapy. This integrated algorithm, compared with the clinical algorithm, had a significantly higher area under the curve in the prediction of response. Liu et al. (65) retrospectively collected data from 130 patients with HCC undergoing transarterial chemoembolization (TACE) for the first time who underwent an ultrasound examination within 1 week prior to surgery, constructed and validated a deep learning radiomics-based CEUS model (R-DLCEUS), a machine-learning radiomics-based CEUS model with TICs (R-TIC), and a machine learning radiomics-based B-mode image model (R-BMode) to predict the response (objective response and nonresponse) to TACE. The results showed that all three models exhibited excellent robustness, indicating that the deep learning radiomics-based approach can effectively use CEUS videos to achieve the accurate and personalized prediction of the treatment response of TACE.
Frontiers in ultrasomics
According to the heatmap of the top 20 keywords over time, in the past three years, the research into ultrasomics has diversified, gradually extending out of differential diagnosis to the areas of disease prognosis, neoadjuvant chemotherapy, and radiogenomics. In Park et al.’s (66) study, computer-aided ultrasound was shown to be able to estimate the disease-free interval of routine patients with PTC, thus contributing to the development of personalized treatment and follow-up planning. The deep learning-based radiomics system developed by Jiang et al. (6) could assess the efficacy of neoadjuvant chemotherapy for pre and posttreatment pathology of breast cancer in pCR, which can provide valuable information for individualized medical treatment to improve the patient’s quality of life and is a successful application of the precision medicine concept in the field of breast diseases. Park et al.’s findings correlating morphological and vascular ultrasound features of breast cancer with their RNA sequencing results (67) suggest that microvascular ultrasound features that are easily assessed in routine practice can reflect important genetic alterations associated with breast cancer and angiogenesis; additionally, they can provide information for superior prognostic prediction and potential therapeutic targeting. The emerging fields of ultrasomics mentioned above are well confirmed, but most studies have used retrospective datasets and single institutions, and thus prospective studies are still needed for further validation. In addition, the combined application of ultrasomics features with clinical information, genomics, proteomics, etc. is still in the nascent stage of exploration and awaiting further contributions from interested researchers.
Radiomics has been enthusiastically pursued by radiologists and computer engineers since it was first proposed in 2012. The application of radiomics can alleviate the inherent subjectivity of manual image interpretation and provide a data-driven approach for objectively evaluating the characteristics of lesions. Ultrasomics had a relatively late start due to the specificity of its imaging modality and has only gained traction in recent years. However, with the advancement in image-processing techniques, the improvement of equipment resolution, and the refinement of artificial intelligence algorithms, an increasing number of high-quality clinical prediction models are appearing, providing improved clinical decision-making.
In the future, breakthroughs in multimodal fusion and dynamic video processing will enrich radiomics with new ideas for model development, and new technology will overcome the limitation of selecting only a single slice from ultrasound images. Moreover, novel developments in contrast and three-dimensional (3D) ultrasomics have provided more useful feature information for predictive modeling, and artificial intelligence algorithms can enable more in-depth and accurate interpretation of images; thus, they are uniquely suited to the extraction and selection of features, which has led to the emergence of computer-aided design systems, which have demonstrated good performance in disease typing, early disease detection, segmentation of medical images, and reconstruction of sonograms. In addition, the multiomics research of genomics, proteomics, and metabolomics has become a hotspot in the research of tumor molecular biology, and the integration of radiomics and other multiomics approaches will integrate the macroscopic imaging features of tumors and microscopic biological information to jointly aid in the diagnosis of tumors, prognosis. and evaluation of therapeutic efficacy. A good prediction model often requires the training and preparation of a large amount of imaging data, and the standardization and sharing of imaging data is an inevitable trend in the development of radiomics, requiring the joint efforts of multidisciplinary fields such as information technology, biomedicine, and clinical medicine.
The progression of ultrasomics represents a success in the context of precision medicine. It is hoped that ultrasomics will be able to provide patients with a more personalized, higher-quality, and cost-effective healthcare environment in the future.
Limitations
In this study, we used bibliometrics to identify the hotspots and cutting-edge research in the ultrasomics, systematically characterized the structure of knowledge, and visually mapped the literature in the field so as to provide scholars with research ideas through the provision of an overall framework of ultrasomics knowledge. However, there are some limitations to this study which should be addressed. In the author cocitation analysis, the data downloaded from the WoSCC only provided the abbreviation of the reference author’s name, and we could not be sure which author this abbreviated name referred to, which might have influenced the reliability of the results. Moreover, all of the literature was collected from a single database, the WoSCC, and so relevant literature from other databases might have been missed. In addition, our study only included articles published after November 2023, and thus some of the more recent research hotspots might have been omitted.
Conclusions
We conducted a comprehensive analysis of publications related to ultrasomics using bibliometric tools to characterize the current status and research hotspots in the field. Ultrasomics is gradually emerging in the diagnosis, prediction, and prognostic assessment of diseases and may greatly facilitate clinical decision-making and contribute to the development of precision medicine. However, there remain significant problems in ultrasomics, which need to be solved via multidisciplinary cooperation between the fields of biomedicine, information technology, statistics, and clinical medicine. It is hoped that in the future, multiomics and data sharing can be combined to establish better clinical decision-making tools.
Acknowledgments
Funding: This study was supported by
Footnote
Conflicts of Interest: All authors have completed the ICMJE uniform disclosure form (available at https://qims.amegroups.com/article/view/10.21037/qims-23-1867/coif). The authors have no conflicts of interest to declare.
Ethical Statement: The authors are accountable for all aspects of the work in ensuring that questions related to the accuracy or integrity of any part of the work are appropriately investigated and resolved.
Open Access Statement: This is an Open Access article distributed in accordance with the Creative Commons Attribution-NonCommercial-NoDerivs 4.0 International License (CC BY-NC-ND 4.0), which permits the non-commercial replication and distribution of the article with the strict proviso that no changes or edits are made and the original work is properly cited (including links to both the formal publication through the relevant DOI and the license). See: https://creativecommons.org/licenses/by-nc-nd/4.0/.
References
- Rix A, Lederle W, Theek B, Lammers T, Moonen C, Schmitz G, Kiessling F. Ad-vanced Ultrasound Technologies for Diagnosis and Therapy. J Nucl Med 2018;59:740-6. [Crossref] [PubMed]
- Lambin P, Rios-Velazquez E, Leijenaar R, Carvalho S, van Stiphout RG, Granton P, Zegers CM, Gillies R, Boellard R, Dekker A, Aerts HJ. Radiomics: extracting more information from medical images using advanced feature analysis. Eur J Cancer 2012;48:441-6. [Crossref] [PubMed]
- Peng Z, Wang Y, Wang Y, Jiang S, Fan R, Zhang H, Jiang W. Application of radiomics and machine learning in head and neck cancers. Int J Biol Sci 2021;17:475-86. [Crossref] [PubMed]
- Jia Y, Yang J, Zhu Y, Nie F, Wu H, Duan Y, Chen K. Ultrasound-based radiomics: current status, challenges and future opportunities. Med Ultrason 2022;24:451-60. [Crossref] [PubMed]
- Wang YG, Xu FJ, Agyekum EA, Xiang H, Wang YD, Zhang J, Sun H, Zhang GL, Bo XS, Lv WZ, Wang X, Hu SD, Qian XQ. Radiomic Model for Determining the Value of Elasticity and Grayscale Ultrasound Diagnoses for Predicting BRAF(V600E) Mutations in Papillary Thyroid Carcinoma. Front Endocrinol (Lausanne) 2022;13:872153. [Crossref] [PubMed]
- Jiang M, Li CL, Luo XM, Chuan ZR, Lv WZ, Li X, Cui XW, Dietrich CF. Ultrasound-based deep learning radiomics in the assessment of pathological complete response to neoadjuvant chemotherapy in locally advanced breast cancer. Eur J Cancer 2021;147:95-105. [Crossref] [PubMed]
- Căleanu CD, Sîrbu CL, Simion G. Deep Neural Architectures for Contrast Enhanced Ultrasound (CEUS) Focal Liver Lesions Automated Diagnosis. Sensors (Basel) 2021;21:4126. [Crossref] [PubMed]
- Wu P, Liu Y, Li Y, Liu B. Robust Prostate Segmentation Using Intrinsic Properties of TRUS Images. IEEE Trans Med Imaging 2015;34:1321-35. [Crossref] [PubMed]
- Tang W, Zhang Y, Yu XL, Zhang WZ, Chen PJ, Wang Y, Zhang X, Yang GY. Diagnostic value of the dual-modal imaging radiomics model for subpleural pulmonary lesions. Eur J Radiol 2023;166:111000. [Crossref] [PubMed]
- Rousseau R. Library science: Forgotten founder of bibliometrics. Nature 2014;510:218. [Crossref] [PubMed]
- Chen C, Hu Z, Liu S, Tseng H. Emerging trends in regenerative medicine: a scientometric analysis in CiteSpace. Expert Opin Biol Ther 2012;12:593-608. [Crossref] [PubMed]
- van Eck NJ, Waltman L. Software survey: VOSviewer, a computer program for bibliometric mapping. Scientometrics 2010;84:523-38. [Crossref] [PubMed]
- Chen C. CiteSpace II: Detecting and visualizing emerging trends and transient pat-terns in scientific literature. J Am Soc Inf Sci Technol 2005;57:359-77. [Crossref]
- Singh VK, Singh P, Karmakar M, Leta J, Mayr P. The journal coverage of Web of Science, Scopus and Dimensions: A comparative analysis. Scientometrics 2021;126:5113-42. [Crossref]
- Zhao X, Wang S, Wang X. Characteristics and Trends of Research on New Energy Vehicle Reliability Based on the Web of Science. Sustainability 2018;10:3560. [Crossref]
- Wang L, Wang J, Zhang Y, Zhang H. Current perspectives and trends of the research on hypertensive nephropathy: a bibliometric analysis from 2000 to 2023. Ren Fail 2024;46:2310122. [Crossref] [PubMed]
- Yuan G, Yang Y, Lin Y, Lin J, Wu Y. Current status and development trends in CKD with frailty research from 2000 to 2021: a bibliometric analysis. Ren Fail 2024;46:2292142. [Crossref] [PubMed]
- van Eck NJ, Waltman, L. Visualizing Bibliometric Networks. In: Ding Y, Rousseau R, Wolfram D. editors. Measuring Scholarly Impact. Springer, 2014:285-320.
- Freeman LC. Centrality in social networks conceptual clarification. Social Networks 1978;1:215-39. [Crossref]
- Gillies RJ, Kinahan PE, Hricak H. Radiomics: Images Are More than Pictures, They Are Data. Radiology 2016;278:563-77. [Crossref] [PubMed]
- Lambin P, Leijenaar RTH, Deist TM, Peerlings J, de Jong EEC, van Timmeren J, Sanduleanu S, Larue RTHM, Even AJG, Jochems A, van Wijk Y, Woodruff H, van Soest J, Lustberg T, Roelofs E, van Elmpt W, Dekker A, Mottaghy FM, Wildberger JE, Walsh S. Radiomics: the bridge between medical imaging and personalized medicine. Nat Rev Clin Oncol 2017;14:749-62. [Crossref] [PubMed]
- van Griethuysen JJM, Fedorov A, Parmar C, Hosny A, Aucoin N, Narayan V, Beets-Tan RGH, Fillion-Robin JC, Pieper S, Aerts HJWL. Computational Radiomics System to Decode the Radiographic Phenotype. Cancer Res 2017;77:e104-7. [Crossref] [PubMed]
- Aerts HJ, Velazquez ER, Leijenaar RT, Parmar C, Grossmann P, Carvalho S, Bussink J, Monshouwer R, Haibe-Kains B, Rietveld D, Hoebers F, Rietbergen MM, Leemans CR, Dekker A, Quackenbush J, Gillies RJ, Lambin P. Decoding tumour phenotype by noninvasive imaging using a quantitative radiomics approach. Nat Commun 2014;5:4006. [Crossref] [PubMed]
- Guo Y, Hu Y, Qiao M, Wang Y, Yu J, Li J, Chang C. Radiomics Analysis on Ultrasound for Prediction of Biologic Behavior in Breast Invasive Ductal Carcinoma. Clin Breast Cancer 2018;18:e335-44. [Crossref] [PubMed]
- Huang YQ, Liang CH, He L, Tian J, Liang CS, Chen X, Ma ZL, Liu ZY. Development and Validation of a Radiomics Nomogram for Preoperative Prediction of Lymph Node Metastasis in Colorectal Cancer. J Clin Oncol 2016;34:2157-64. [Crossref] [PubMed]
- Kumar V, Gu Y, Basu S, Berglund A, Eschrich SA, Schabath MB, Forster K, Aerts HJ, Dekker A, Fenstermacher D, Goldgof DB, Hall LO, Lambin P, Balagurunathan Y, Gatenby RA, Gillies RJ. Radiomics: the process and the challenges. Magn Reson Imaging 2012;30:1234-48. [Crossref] [PubMed]
- Hu HT, Wang Z, Huang XW, Chen SL, Zheng X, Ruan SM, Xie XY, Lu MD, Yu J, Tian J, Liang P, Wang W, Kuang M. Ultrasound-based radiomics score: a potential biomarker for the prediction of microvascular invasion in hepatocellular carcinoma. Eur Radiol 2019;29:2890-901. [Crossref] [PubMed]
- Haugen BR, Alexander EK, Bible KC, Doherty GM, Mandel SJ, Nikiforov YE, Pacini F, Randolph GW, Sawka AM, Schlumberger M, Schuff KG, Sherman SI, Sosa JA, Steward DL, Tuttle RM, Wartofsky L. 2015 American Thyroid Association Management Guidelines for Adult Patients with Thyroid Nodules and Differentiated Thyroid Cancer: The American Thyroid Association Guidelines Task Force on Thyroid Nodules and Differentiated Thyroid Cancer. Thyroid 2015;26:1-133. [Crossref] [PubMed]
- He S, Zhao Y, Fan Y, Zhao X, Yu J, Xie J, Wang C, Su J. Research Trends and Hotspots Analysis Related to Monocarboxylate Transporter 1: A Study Based on Bibliometric Analysis. Int J Environ Res Public Health 2019;16:1091. [Crossref] [PubMed]
- Yue T, Liu H, Long R, Chen H, Gan X, Liu J. Research trends and hotspots related to global carbon footprint based on bibliometric analysis: 2007-2018. Environ Sci Pollut Res Int 2020;27:17671-91. [Crossref] [PubMed]
- Gu J, Jiang T. Ultrasound radiomics in personalized breast management: Current status and future prospects. Front Oncol 2022;12:963612. [Crossref] [PubMed]
- Cao LL, Peng M, Xie X, Chen GQ, Huang SY, Wang JY, Jiang F, Cui XW, Dietrich CF. Artificial intelligence in liver ultrasound. World J Gastroenterol 2022;28:3398-409. [Crossref] [PubMed]
- Wildeboer RR, Mannaerts CK, van Sloun RJG, Budäus L, Tilki D, Wijkstra H, Salomon G, Mischi M. Automated multiparametric localization of prostate cancer based on B-mode, shear-wave elastography, and contrast-enhanced ultrasound radiomics. Eur Radiol 2020;30:806-15. [Crossref] [PubMed]
- DeJohn CR, Grant SR, Seshadri M. Application of Machine Learning Methods to Improve the Performance of Ultrasound in Head and Neck Oncology: A Literature Review. Cancers (Basel) 2022;14:665. [Crossref] [PubMed]
- Yin R, Jiang M, Lv WZ, Jiang F, Li J, Hu B, Cui XW, Dietrich CF. Study Processes and Applications of Ultrasomics in Precision Medicine. Front Oncol 2020;10:1736. [Crossref] [PubMed]
- Smith CP, Czarniecki M, Mehralivand S, Stoyanova R, Choyke PL, Harmon S, Turkbey B. Radiomics and radiogenomics of prostate cancer. Abdom Radiol (NY) 2019;44:2021-9. [Crossref] [PubMed]
- Huang X, Chen M, Liu P, Du Y. Texture Feature-Based Classification on Transrectal Ultrasound Image for Prostatic Cancer Detection. Comput Math Methods Med 2020;2020:7359375. [Crossref] [PubMed]
- Lubner MG, Smith AD, Sandrasegaran K, Sahani DV, Pickhardt PJ. CT Texture Analysis: Definitions, Applications, Biologic Correlates, and Challenges. Radiographics 2017;37:1483-503. [Crossref] [PubMed]
- Sepúlveda-Oviedo EH, Travé-Massuyès L, Subias A, Pavlov M, Alonso C. Fault diagnosis of photovoltaic systems using artificial intelligence: A bibliometric approach. Heliyon 2023;9:e21491. [Crossref] [PubMed]
- Wang Y, Ge X, Ma H, Qi S, Zhang G, Yao Y. Deep Learning in Medical Ultrasound Image Analysis: A Review. IEEE Access 2021;9:54310-24.
- Marya NB, Powers PD, Chari ST, Gleeson FC, Leggett CL, Abu Dayyeh BK, Chandrasekhara V, Iyer PG, Majumder S, Pearson RK, Petersen BT, Rajan E, Sawas T, Storm AC, Vege SS, Chen S, Long Z, Hough DM, Mara K, Levy MJ. Utilisation of artificial intelligence for the development of an EUS-convolutional neural network model trained to enhance the diagnosis of autoimmune pancreatitis. Gut 2021;70:1335-44. [Crossref] [PubMed]
- Azizi S, Bayat S, Yan P, Tahmasebi A, Nir G, Kwak JT, Xu S, Wilson S, Iczkowski KA, Lucia MS, Goldenberg L, Salcudean SE, Pinto PA, Wood B, Abolmaesumi P, Mousavi P. Detection and grading of prostate cancer using temporal enhanced ultrasound: combining deep neural networks and tissue mimicking simulations. Int J Comput Assist Radiol Surg 2017;12:1293-305. [Crossref] [PubMed]
.Wang Z Zhang Z Zheng JQ Huang B Voiculescu I Yang G-Z Deep Learning in Medical Ultrasound Image Segmentation: a Review. arXiv: 2002.07703,2020 .- Perdios D, Besson A, Arditi M, Thiran JP. A deep learning approach to ultra-sound image recovery. 2017 IEEE International Ultrasonics Symposium (IUS), Washington, DC, USA, 2017:1-4.
- Lo YC, Rensi SE, Torng W, Altman RB. Machine learning in chemoinformatics and drug discovery. Drug Discov Today 2018;23:1538-46. [Crossref] [PubMed]
- Aamir S, Rahim A, Aamir Z, Abbasi SF, Khan MS, Alhaisoni M, Khan MA, Khan K, Ahmad J. Predicting Breast Cancer Leveraging Supervised Machine Learning Techniques. Comput Math Methods Med 2022;2022:5869529. [Crossref] [PubMed]
- Al-Azzam N, Shatnawi I. Comparing supervised and semi-supervised Machine Learning Models on Diagnosing Breast Cancer. Ann Med Surg (Lond) 2021;62:53-64. [Crossref] [PubMed]
- Abbasi S, Hajabdollahi M, Khadivi P, Karimi N, Roshandel R, Shirani S, Samavi S. Classification of diabetic retinopathy using unlabeled data and knowledge distillation. Artif Intell Med 2021;121:102176. [Crossref] [PubMed]
- Deo RC. Machine Learning in Medicine. Circulation 2015;132:1920-30. [Crossref] [PubMed]
- LeCun Y, Bengio Y, Hinton G. Deep learning. Nature 2015;521:436-44. [Crossref] [PubMed]
- Li L, Lin X, Liao T, Ouyang R, Li M, Yuan J, Ma J. Clinical application of convolutional neural network for mass analysis on mammograms. Quant Imaging Med Surg 2023;13:8413-22. [Crossref] [PubMed]
- Liu Z, Li H, Li W, Zhuang D, Zhang F, Ouyang W, Wang S, Bertolaccini L, Alskaf E, Pan X. Noncontact remote sensing of abnormal blood pressure using a deep neural network: a novel approach for hypertension screening. Quant Imaging Med Surg 2023;13:8657-68. [Crossref] [PubMed]
- Shin HC, Roth HR, Gao M, Lu L, Xu Z, Nogues I, Yao J, Mollura D, Summers RM. Deep Convolutional Neural Networks for Computer-Aided Detection: CNN Architectures, Dataset Characteristics and Transfer Learning. IEEE Trans Med Imaging 2016;35:1285-98. [Crossref] [PubMed]
- Zhang D, Zhang XY, Duan YY, Dietrich CF, Cui XW, Zhang CX. An overview of ultrasound-derived radiomics and deep learning in liver. Med Ultrason 2023;25:445-52. [Crossref] [PubMed]
- Fujioka T, Kubota K, Mori M, Kikuchi Y, Katsuta L, Kasahara M, Oda G, Ishiba T, Nakagawa T, Tateishi U. Distinction between benign and malignant breast masses at breast ultrasound using deep learning method with convolutional neural network. Jpn J Radiol 2019;37:466-72. [Crossref] [PubMed]
- Wu X, Tan G, Luo H, Chen Z, Pu B, Li S, Li K. A knowledge-interpretable multi-task learning framework for automated thyroid nodule diagnosis in ultrasound videos. Med Image Anal 2024;91:103039. [Crossref] [PubMed]
- Jiang M, Zhang D, Tang SC, Luo XM, Chuan ZR, Lv WZ, Jiang F, Ni XJ, Cui XW, Dietrich CF. Deep learning with convolutional neural network in the assessment of breast cancer molecular subtypes based on US images: a multicenter retrospective study. Eur Radiol 2021;31:3673-82. [Crossref] [PubMed]
- Liu F, Liu D, Wang K, Xie X, Su L, Kuang M, Huang G, Peng B, Wang Y, Lin M, Tian J, Xie X. Deep Learning Radiomics Based on Contrast-Enhanced Ultrasound Might Optimize Curative Treatments for Very-Early or Early-Stage Hepatocellular Carcinoma Patients. Liver Cancer 2020;9:397-413. [Crossref] [PubMed]
- Yao F, Ding J, Hu Z, Cai M, Liu J, Huang X, Zheng R, Lin F, Lan L. Ultrasound-based radiomics score: a potential biomarker for the prediction of progression-free survival in ovarian epithelial cancer. Abdom Radiol (NY) 2021;46:4936-45. [Crossref] [PubMed]
- Chang L, Zhang Y, Zhu J, Hu L, Wang X, Zhang H, Gu Q, Chen X, Zhang S, Gao M, Wei X. An integrated nomogram combining deep learning, clinical characteristics and ultrasound features for predicting central lymph node metastasis in papillary thyroid cancer: A multicenter study. Front Endocrinol (Lausanne) 2023;14:964074. [Crossref] [PubMed]
- Jiang M, Li CL, Luo XM, Chuan ZR, Chen RX, Tang SC, Lv WZ, Cui XW, Dietrich CF. Radiomics model based on shear-wave elastography in the assessment of axillary lymph node status in early-stage breast cancer. Eur Radiol 2022;32:2313-25. [Crossref] [PubMed]
- Zhang H, Huo F. Prediction of early recurrence of HCC after hepatectomy by contrast-enhanced ultrasound-based deep learning radiomics. Front Oncol 2022;12:930458. [Crossref] [PubMed]
- Wang Y, Feng G, Wang J, An P, Duan P, Hu Y, Ye Y, Li Y, Qin P, Song P. Contrast-Enhanced Ultrasound-Magnetic Resonance Imaging Radiomics Based Model for Predicting the Biochemical Recurrence of Prostate Cancer: A Feasibility Study. Comput Math Methods Med 2022;2022:8090529. [Crossref] [PubMed]
- Cai L, Sidey-Gibbons C, Nees J, Riedel F, Schäfgen B, Togawa R, Killinger K, Heil J, Pfob A, Golatta M. Can multi-modal radiomics using pretreatment ultrasound and tomosynthesis predict response to neoadjuvant systemic treatment in breast cancer? Eur Radiol 2024;34:2560-73. [Crossref] [PubMed]
- Liu D, Liu F, Xie X, Su L, Liu M, Xie X, Kuang M, Huang G, Wang Y, Zhou H, Wang K, Lin M, Tian J. Accurate prediction of responses to transarterial chemoembolization for patients with hepatocellular carcinoma by using artificial intelligence in contrast-enhanced ultrasound. Eur Radiol 2020;30:2365-76. [Crossref] [PubMed]
- Park VY, Han K, Lee E, Kim EK, Moon HJ, Yoon JH, Kwak JY. Association Between Radiomics Signature and Disease-Free Survival in Conventional Papillary Thyroid Carcinoma. Sci Rep 2019;9:4501. [Crossref] [PubMed]
- Park AY, Seo BK, Han MR. Breast Ultrasound Microvascular Imaging and Radiogenomics. Korean J Radiol 2021;22:677-87. [Crossref] [PubMed]