Magnetic resonance imaging–based prognostic model for subsequent distant metastasis in patients with ipsilateral breast tumor recurrence following breast-conserving surgery
Introduction
Breast cancer is the most commonly diagnosed cancer and the leading cause of cancer-related death among women worldwide (1). Clinical trials have suggested that patients with early-stage breast cancer who undergo breast-conserving surgery (BCS) followed by radiotherapy have comparable survival to those who undergo mastectomy (2,3), with the added benefit of enhanced satisfaction and quality of life (4). However, ipsilateral breast tumor recurrence (IBTR) has an annual incidence of approximately 0.5–1.5% in those with invasive carcinoma, with an overall incidence ranging from 5% to 10% after a 10-year follow-up, posing significant challenges in routine clinical practice (3,5,6). Notably, the occurrence of IBTR is psychologically distressing for patients, requiring salvage therapy and resulting in the loss of cosmetic effects, and is additionally associated with a high risk of distant metastasis (DM) (7-9). In population-based real-world studies (10), three distinct clinical outcomes of IBTR after BCS for early-stage breast cancer have been identified, with each being associated with a distinct prognosis: isolated IBTR (55%), IBTR with synchronous DM (30%), and metachronous DM after IBTR surgery (15%). However, in the absence of standardized treatment guidelines, clinicians should strive to predict the clinical course of patients with IBTR solely on retrospective clinical data and to estimate the risk for subsequent recurrence (11). Therefore, there is an increasing need to determine the outcomes after IBTR and identify the patient populations at high risk for a second event. Previous studies have identified various clinicopathological factors that may influence the prognosis of patients with IBTR after BCS, including age, disease-free interval (DFI), biological characteristics, initial disease stage, systemic therapy for primary cancer, radiotherapy, and resectable surgery after IBTR (8,12). However, these factors alone remain insufficient for the accurate prediction of the prognosis of patients with IBTR (9), highlighting the necessity for additional predictive tools.
The use of magnetic resonance imaging (MRI) is widespread for patients with breast cancer due to its superior soft tissue resolution. Several MRI radiological features, including background parenchymal enhancement (BPE), peritumor edema, and nonmass enhancement, have been shown to be associated with the prognosis of patients who undergo BCS for breast cancer (13-17). Furthermore, radiomics, an emerging technology leveraging quantitative analysis to identify correlations between imaging characteristics and clinical outcomes, has demonstrated promising performance in predicting prognosis for various cancers (18-21). Nevertheless, few studies have investigated the radiological and radiomics features related to the prognosis of patients who have undergone IBTR resection.
Therefore, the aim of this study was to develop a predictive model that incorporates routine clinicopathological variables along with MRI qualitative and quantitative features of primary and IBTR tumors to assess the risk of DM in patients with IBTR. In pursuit of this goal, the study proposed a prognostic nomogram to serve as a valuable reference for precision medicine. We present this article in accordance with the TRIPOD reporting checklist (available at https://qims.amegroups.com/article/view/10.21037/qims-23-1831/rc).
Methods
Study population
This study was conducted in accordance with the Declaration of Helsinki (as revised in 2013) and was approved by the Ethics Committee of the Institutional Review Board (IRB) of Fudan University Shanghai Cancer Center (FUSCC) (No. 050432-4-2307E). Individual consent for this retrospective analysis was waived. The analysis included consecutive patients who underwent BCS between 2010 and 2018 at FUSCC in Shanghai, China, and who experienced IBTR without distant metastases during follow-up. All IBTR cases were confirmed surgically, and clinicopathological and pretreatment MRI data of the primary and recurrent tumors were collected. The detailed inclusion and exclusion criteria and enrollment process are shown in Figure 1.
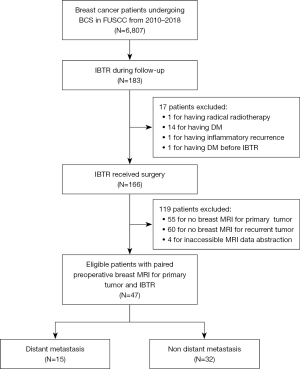
Clinicopathological data collection and follow-up
The factors analyzed in this study encompassed patient and tumor characteristics, including age at initial diagnosis, DFI, lymph node (LN) status, tumor size, histological type, histological grade, estrogen receptor (ER) status, progesterone receptor (PR) status, human epidermal growth factor receptor 2 (HER2) status, Ki-67 index, and lymphovascular invasion (LVI). Additionally, treatment parameters after the initial surgery and IBTR was examined, which included the extent of surgery (repeat lumpectomy and mastectomy), postsurgical radiotherapy, and systemic therapy such as endocrine therapy, chemotherapy, and anti-HER2 therapy. ER and PR statuses were defined as positive if immunohistochemistry (IHC) staining results were greater than 1% (22). HER2 status was considered positive if the IHC staining result was 3+ or if the fluorescent in situ hybridization results showed positive findings (23). Additionally, the DFI was defined as the duration from the initial BCS to the detection of the first IBTR event(s).
The primary endpoint of this study was distant metastasis-free survival (DMFS), defined as the time from the resection of the recurrent tumor to the first occurrence of DM, death from breast cancer, death from non-breast cancer, or death from an unknown cause (24). DM was defined as the presence of disease extending beyond the ipsilateral breast, contralateral breast, or regional LNs as confirmed through pathological evaluation of metastatic lesions. The follow-up period ranged from 1.4 to 101.1 months, and DMFS probabilities were calculated at 2, 3, and 5 years postsurgery.
Analysis of clinicopathological and MRI features
All patients included in the study underwent preoperative dynamic contrast-enhanced MRI (DCE-MRI) to evaluate both primary and recurrent breast tumors. The DCE-MRI acquisition protocol is described in detail in Appendix 1 (Supplementary Method 1) and Table S1. The data obtained from MRI, including qualitative and quantitative features, were analyzed together with conventional clinicopathological features. The procedures for determining the qualitative characteristics and extracting the quantitative features are described in Appendix 1 (Supplementary Method 2).
Initially, qualitative characteristics were collected based on the lexicon provided by the Breast Imaging Reporting and Data System (BI-RADS). Univariate and multivariate Cox regression analyses were then used to identify significant prognostic factors for clinicopathological and radiological qualitative variables.
After image preprocessing, quantitative features were extracted using the PyRadiomics package (version 3.0; https://pyradiomics.readthedocs.io/en/latest/index.html), with the entire tumor region of interest (ROI) being delineated. In total, 204 features were acquired, including shape, first-order, and texture features. Specifically, 102 features were extracted from the primary tumors, and an additional 102 features were extracted from the recurrent tumors. For the quantitative imaging analysis, all features were standardized to a normal distribution with z-scores before further analysis to eliminate differences in the value scales of the data. A three-step procedure was employed to identify the robust features. First, a reproducibility test was performed, and features with an interclass correlation coefficient <0.75 were excluded. Second, a Pearson correlation analysis was conducted to eliminate redundant features. Correlated features with an absolute value of the Pearson correlation coefficient greater than or equal to 0.95 were excluded. Finally, the least absolute shrinkage and selection operator (LASSO) Cox regression algorithm with penalty parameter tuning via 10-fold cross-validation was employed. Consequently, only four features were selected for the final quantitative analysis, which were then calculated as the radiomics score (Radscore).
Development and performance of the prediction model
Three Cox regression classifier-based prediction models were developed: (I) a clinicoradiological model incorporating significant clinicopathological and radiological variables, (II) a radiomics model incorporating only the Radscore, and (III) a combined model that integrated significant clinicopathological and radiological variables with the Radscore. The models’ performance was evaluated based on discrimination, calibration, and clinical utility. Discrimination performance was quantified using the Harrell concordance index (C-index) and the time-dependent area under the curve (AUC). Internal bootstrap resampling was applied for model verification. Calibration curves were generated to assess model calibration, and decision curve analyses (DCAs) were conducted to evaluate clinical utility. To support clinicians in predicting DMFS quantitatively, a nomogram was developed based on the best-performing model. The nomogram classified patients into high- and low-risk groups using X-tile analyses (25). Kaplan-Meier survival analysis was performed to evaluate the survival differences between the high- and low-risk groups. A flowchart of the proposed study design is shown in Figure 2.
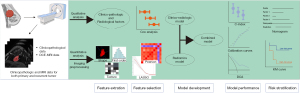
Statistical analysis
To estimate DMFS probabilities, we used the Kaplan-Meier method and compared the results via the log-rank test. Univariate and multivariate Cox regression analyses of qualitative clinicopathological and radiological features were used to identify independent risk factors. Any features with a univariate Cox regression result of P<0.1 were included in the multivariate Cox regression. Furthermore, we used a stepwise regression method based on the Akaike information criterion (AIC) to extract independent prognostic factors. The quantitative feature analysis is described above. We constructed models using the Cox regression classifier, with model discrimination evaluated using the Harrell C-index and compared C-index with a previously described method (26). The time-dependent AUC was derived from every point measured between 24 and 60 months to assess the prognostic accuracy at varying time points. The model fit was assessed using a calibration plot with 500 bootstrap resamples. The clinical utility of the model was evaluated using DCAs. The optimal cutoff point for the nomogram was obtained using X-tile software (version 3.6.1; Yale University School of Medicine, New Haven, CT, USA; RRID:SCR_005602).
Statistical analysis was performed with R software (version 4.2.1; The R Foundation of Statistical Computing; RRID:SCR_001905; http://www.r-project.org) and SPSS software (version 22.0; IBM Corp., Armonk, NY, USA; RRID:SCR_019096). A two-sided P value <0.05 was indicative of a statistically significant difference.
Results
Patient characteristics
A total of 6,807 patients underwent BCS at FUSCC between 2010 and 2018. Of these patients, 183 (2.7%) were diagnosed with IBTR. Ultimately, 47 cases were included in the study (Figure 1), with a mean age at primary breast cancer diagnosis of 46.8 years [standard deviation (SD) 11.7]. The clinicopathological characteristics of the patients are presented in Table 1. The median follow-up duration after IBTR was 22.0 months and ranging from 1.4 to 101.1 months. Out of the included patients, 15 developed metastases during the follow-up period (Table S2), with a median time of 17.2 months (ranging from 1.9 to 37.5 months) from the IBTR resection. The estimated DMFS rates 2, 3, and 5 years after IBTR resection were 69.5% [95% confidence interval (CI): 54.8–84.2%], 62.4% (95% CI: 46.3–78.5%), and 58.2% (95% CI: 41.1–75.3%), respectively.
Table 1
Characteristic | Classification | Patients, n (%) |
---|---|---|
Presentation at primary cancer | ||
Age (years) | ≤40 | 16 (34.0) |
>40 | 31 (66.0) | |
ALN | Negative | 30 (63.8) |
Positive | 17 (36.2) | |
Tumor size (cm) | ≤2 | 25 (53.2) |
>2 | 22 (46.8) | |
Histological subtype | Ductal | 45 (95.7) |
Lobular | 1 (2.1) | |
Others | 1 (2.1) | |
Histological grade | Grade I or II | 15 (31.9) |
Grade III | 26 (55.3) | |
Unknown | 6 (12.8) | |
LVI | Negative | 31 (66.0) |
Positive | 16 (34.0) | |
ER status | Negative | 27 (57.4) |
Positive | 20 (42.6) | |
PR status | Negative | 28 (59.6) |
Positive | 19 (40.4) | |
HER2 status | Negative | 29 (61.7) |
Positive | 18 (38.3) | |
Ki-67 index | ≤20% | 8 (17) |
>20% | 39 (83) | |
Chemotherapy | No | 14 (30.0) |
Yes | 33 (70.0) | |
Radiotherapy | No | 18 (38.3) |
Yes | 29 (61.7) | |
Endocrine therapy | No | 36 (76.6) |
Yes | 11 (23.4) | |
Anti-HER2 therapy | No | 40 (85.1) |
Yes | 7 (14.9) | |
Presentation at IBTR | ||
DFI (years) | ≤2 | 26 (55.3) |
>2 | 21 (44.7) | |
Resection | Lumpectomy | 5 (10.6) |
Mastectomy | 42 (89.4) | |
Tumor size (cm) | ≤2 | 34 (72.3) |
>2 | 13 (27.7) | |
Histological subtype | Ductal | 42 (89.4) |
Lobular | 1 (2.1) | |
Other | 4 (8.5) | |
Histological grade | Grade I or II | 12 (25.5) |
Grade III | 23 (48.9) | |
Unknown | 12 (25.5) | |
ER status | Negative | 32 (68.1) |
Positive | 15 (31.9) | |
PR status | Negative | 37 (78.7) |
Positive | 10 (21.3) | |
HER2 status | Negative | 25 (53.2) |
Positive | 22 (46.8) | |
Ki-67 index | ≤20% | 18 (38.3) |
>20% | 29 (61.7) | |
Chemotherapy | No | 10 (21.3) |
Yes | 37 (78.7) | |
Radiotherapy | No | 41 (87.2) |
Yes | 6 (12.8) | |
Endocrine therapy | No | 32 (68.1) |
Yes | 15 (31.9) | |
Anti-HER2 therapy | No | 31 (66.0) |
Yes | 16 (34.0) |
IBTR, ipsilateral breast tumor recurrence; ALN, axillary lymph node; LVI, lymphovascular invasion; ER, estrogen receptor; PR, progesterone receptor; HER2, human epidermal growth factor receptor 2; DFI, disease-free interval.
Analysis of clinicopathological and MRI features
On univariate analysis, several clinicopathological variables showed a marginal significance with time to metastasis, including age at diagnosis of the primary tumor, LN status of the primary tumor, HER2 status of the primary tumor, and tumor size of IBTR (all P values <0.1), while HER2 status of IBTR was significantly associated with time to metastasis (P<0.05) (Table S3). Of the radiological qualitative features, the BPE grade of IBTR was positively associated with time to metastasis. In multivariate analysis, we identified two independent predictors by employing stepwise analysis with the lowest AIC score: HER2 status of IBTR [hazard ratio (HR) =5.40; P<0.01) and IBTR BPE grade (HR =7.94; P<0.001) (Table 2). For quantitative imaging feature analysis, four features extracted from recurrent tumors were selected using the reproducibility test, correlation test, and LASSO Cox regression (Figure S1). These features were used to calculate the Radscore according to the following formula:
Table 2
Variable | Clinicoradiologic model | Combined model | |||
---|---|---|---|---|---|
Hazard ratio | P value | Hazard ratio | P value | ||
HER2 status (negative vs. positive) | 5.44 (1.54–19.18) | 0.008** | 3.75 (1.07–13.19) | 0.04* | |
BPE grade (moderate or marked vs. minimal or mild) | 7.90 (2.32–26.95) | <0.001*** | 5.96 (1.72–20.65) | 0.005** | |
Radscore | NA | NA | 6.75 (1.47–31.00) | 0.01* |
The numbers in parentheses are the 95% confidence interval. *, P<0.05; **, P<0.01; ***, P<0.001. DMFS, distant metastasis-free survival; HER2, human epidermal growth factor receptor 2; BPE, background parenchymal enhancement; NA, not applicable.
Development and performance of the prediction models
Three models were established using independent predictors and the Radscore. For the clinicoradiological model, the HER2 status of IBTR (HR =5.40; P<0.01) and IBTR BPE grade were included and yielded a C-index of 0.71 in the original cohort and 0.72 in the internal bootstrap validation (Table 3). For the radiomics model compose of the Radscore achieved a C-index of 0.70 and 0.71 in the original cohort and internal bootstrap validation, respectively. The combined model incorporating the independent predictors and Radscore obtained a C-index of 0.77 in the original cohort and 0.80 in the internal bootstrap validation.
Table 3
Model | Training cohort | Internal bootstrap validation | P value | |||
---|---|---|---|---|---|---|
C-index | Time-dependent AUC | C-index (95% CI) | Time-dependent AUC | |||
Clinicoradiological | 0.71 | 0.75 | 0.72 (0.55–0.87) | 0.76 | Ref. | |
Radiomics | 0.70 | 0.84 | 0.71 (0.56–0.85) | 0.84 | 0.19 | |
Combined | 0.77 | 0.88 | 0.80 (0.66–0.93) | 0.89 | <0.01* |
The time-dependent AUC represents the median AUC at various time points. All P values were obtained from analyses comparing the C indexes of various models. *, P<0.05. AUC, area under the curve; confidence interval.
For DMFS prediction, the performance of the combined model was superior to that of the clinicoradiological (Z=9.15; P=0.006) and radiomics models (Z=7.47; P=0.01). Using time-dependent AUC analysis, we found that the combined model improved the prediction of DMFS in patients with IBTR as compared with the clinicoradiological and radiomics models at most time points (Figure S2). Moreover, according to the Delong test, the differences of AUCs between the clinicoradiological and combined models were marginally significant for the AUCs at 2 years (P=0.06), 3 years (P=0.05), and 5 years (P=0.07) (Table S4). The corresponding calibration curves for predicting the probability of DM at 2, 3, or 5 years after surgery for each model are presented in Figure 3A-3C and demonstrated satisfactory consistency between the model-predicted survival and the actual observed survival. The DCA indicated that compared to the clinicoradiological and radiomics models, the combined model provided a greater net benefit within a reasonable range of threshold probabilities (Figure 3D).
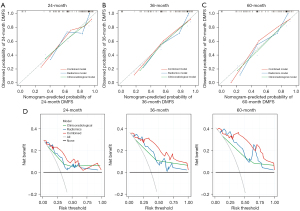
Based on the combined model, a nomogram was constructed to stratify the post-IBTR patients into low- and high-risk groups (Figure 4A). The optimal cutoff point used for stratification was 89.7 according to the X-tile analysis. Kaplan-Meier survival curves showed that the DMFS rates of high-risk patients were poorer than those of low-risk patients (P<0.001; Figure 4B). To further support the proposed nomogram, Figure 4C shows two cases representing both low- and high-risk patients, respectively, illustrating their survival outcomes after IBTR resection.
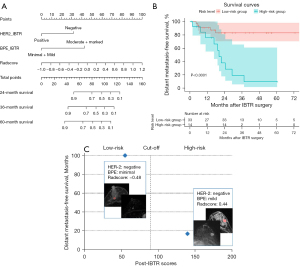
Associations between MRI features and clinicopathological variables
The associations between the MRI features and clinicopathological variables are shown in Table 4. BPE showed significant differences in terms of ER status. Patients with ER-positive tumors were more likely to show high BPE (P<0.05). Furthermore, in order to better understand the relationship between Radscore and clinicopathological characteristics, we used the median of Radscore as the cut-off point to classify patients into low Rad-risk and high Rad-risk. Rad-risk was correlated with the tumor size of IBTR and the change of HER2 status from primary tumor to IBTR (P<0.05). Specifically, patients with high Rad-risk had larger IBTR tumors, while patients with low Rad-risk had proportionately more changes in HER2 status (change in patients with low Rad-risk: 5/24, 20.8%; change in patients with high Rad-risk: 0/23, 0%), with 80% of those experiencing HER2 status change (4/5) switching from negative to positive (Figure 5). There was no statistical difference between BPE and Rad-risk and other pathological characteristics (P>0.05).
Table 4
Characteristic | BPE | Rad-risk | |||||
---|---|---|---|---|---|---|---|
Minimal or mild (n=39) | Moderate or marked (n=8) | P value | Low-risk (n=24) | High-risk (n=23) | P value | ||
Tumor size (cm) | 0.67 | 0.02 | |||||
≤2 | 29 (74.4) | 5 (62.5) | 21 (87.5) | 13 (56.5) | |||
>2 | 10 (25.6) | 3 (37.5) | 3 (12.5) | 10 (43.5) | |||
Histological subtype | 0.30 | 0.69 | |||||
DCIS | 7 (17.9) | 0 (0.0) | 4 (16.7) | 3 (13.0) | |||
IDC | 28 (71.8) | 8 (100.0) | 19 (79.2) | 17 (73.9) | |||
Others | 4 (10.3) | 0 (0.0) | 1 (4.2) | 3 (13.0) | |||
Histological grade | 0.32 | 0.66 | |||||
Grade I/II | 11 (28.2) | 4 (50.0) | 9 (37.5) | 6 (26.1) | |||
Grade III | 21 (53.8) | 4 (50.0) | 11 (45.8) | 14 (60.9) | |||
Unknown | 7 (17.9) | 0 (0.0) | 4 (16.7) | 3 (13.0) | |||
ER status | 0.009 | 0.09 | |||||
Negative | 30 (74.3) | 2 (25.0) | 19 (79.2) | 13 (56.5) | |||
Positive | 9 (25.6) | 6 (75.0) | 5 (20.8) | 10 (43.5) | |||
PR status | 1 | 0.49 | |||||
Negative | 31 (79.5) | 6 (75.0) | 20 (83.3) | 17 (73.9) | |||
Positive | 8 (20.5) | 2 (25.0) | 4 (16.7) | 6 (26.1) | |||
HER2 status | 0.45 | 0.30 | |||||
Negative | 22 (56.4) | 3 (37.5) | 11 (45.8) | 14 (60.9) | |||
Positive | 17 (43.6) | 5 (62.5) | 13 (54.2) | 9 (39.1) | |||
Ki-67 index | 0.69 | 0.77 | |||||
≤20% | 16 (41.0) | 2 (25.0) | 10 (41.7) | 8 (34.8) | |||
>20% | 23 (59.0) | 6 (75.0) | 14 (58.3) | 15 (65.2) |
Data are represented as number (%). MRI, magnetic resonance imaging; BPE, background parenchymal enhancement; DCIS, ductal carcinoma in situ; IDC, invasive ductal carcinoma; ER, estrogen receptor; PR, progesterone receptor; HER2, human epidermal growth factor receptor 2.
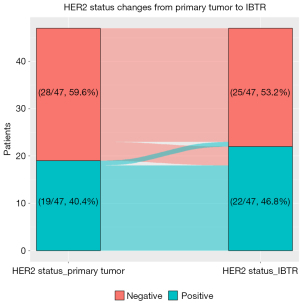
Discussion
Patients with breast cancer who experience IBTR demonstrate an elevated and sustained risk of DM following surgery (27). Risk stratification has emerged as a crucial factor in predicting the eventual development of metachronous distant metastases. Our study sheds light on the importance of both quantitative and qualitative MRI features, specifically those of recurrent tumors, in patients with IBTR. The combined model, in particular, exhibited enhanced prognostic efficacy (C-index 0.77; all P values <0.05) and improved clinical utility compared to the other two models. Furthermore, the combined model effectively stratified IBTR cases into low- and high-risk subgroups, resulting in significant prognostic differentiation (P<0.001). Essentially, our study introduced a noninvasive approach to predicting outcomes in patients with resectable IBTR, which holds profound implications for personalized follow-up.
In this study, we enrolled 47 patients with IBTR from a cohort of 6,807 individuals who underwent BCS. All of these patients had undergone preoperative MRI scans that included matched imaging of primary tumors and recurrent lesions. To the best of our knowledge, this study represents the first attempt to use matched MRI for predicting the subsequent clinical course after IBTR. Our findings revealed a close correlation between the qualitative and quantitative MRI characteristics of recurrent tumors and patient prognosis. However, primary MRI features may not hold significant predictive value for outcomes. Specifically, the Radscore, derived from four quantitative features of recurrent tumors, effectively reflects the risk of postoperative metastasis and demonstrates unique advantages in predicting survival. Furthermore, our study provides evidence supporting that a high BPE grade is an independent, significant risk factor for patients with IBTR (HR =7.94; P<0.001), and these results are consistent with previous studies (14,15). The association between BPE grade and the prognosis of patients with IBTR may stem from the potential misinterpretation of enhancement in multifocal or multicentric malignant lesions as BPE, thereby influencing patient outcomes. However, the pathological origins of BPE remain unclear. Several studies have reported an association between BPE and increased vascular permeability and metabolic activity that is linked to hormones (28). In our study, further analysis indicated that ER-positive patients exhibited higher BPE levels. These results provided further evidence of the hormone-sensitive nature of BPE, indicating a potential role for BPE as an imaging marker of hormonal exposures in the breast (15). Additional research is needed to evaluate the correlation between BPE and prognosis. Consistent with prior studies, our findings suggest that a high BPE grade serves as a significant risk factor for poor outcomes. The addition of MRI at the time of IBTR could be used selectively based on clinical concern.
In order to comprehensively understand the pathological basis of radiomics features, we investigated the correlation between Radscore and pathological variables of recurrent tumors. The results showed that patients with high Rad-risk had larger tumor size. Furthermore, patients with low Rad-risk were more likely to exhibit alterations in HER2 status (change in patients with low Rad-risk: 5/24, 20.8%; change in patients with high Rad-risk: 0/23, 0%), with 80% (4/5) of those experiencing HER2 status change switching from negative to positive. This finding suggests that Rad-risk may be a biomarker for anti-HER2 targeted therapy.
Our study further discovered that HER2-negativity in recurrent tumors is a poor prognostic factor for DM following IBTR surgery, which is consistent with previous studies (8,9,12,29). One possible explanation for this may be the escalating anti-HER2 regimens after IBTR. In the setting of primary breast tumor, 12 out of 19 (63.2%) patients with HER2-positive disease did not receive anti-HER2 therapy (trastuzumab) due to drug accessibility and economic limitations. In contrast, 16 out of 22 (72.7%) patients were administered anti-HER2 treatment. By integrating qualitative and quantitative MRI features with routine clinicopathological variables, our derived model could be interpreted to provide a multimodal and comprehensive understanding of IBTR outcome. Our combined model exhibited a noteworthy C-index of 0.77, outperforming our alternative models and the model described in a previous study (9). Wu et al. developed a model based on 10 clinicopathological factors to predict outcomes in patients with locally recurrent breast cancer, which achieved a final C-index of 0.72 in the validation set (9). Consequently, our combined model demonstrated significant advancements in the accuracy of prognostic prediction for this specific patient cohort. Moreover, the calibration curves for the combined model displayed excellent performance, and DCA indicated that our proposed model yielded greater net benefits for patients compared to the models using either clinicoradiological or radiomics alone. These findings emphasize the potential of MRI-based features in providing supplementary prognostic information alongside clinical characteristics, which aligns with earlier studies (19,30). Huang et al. explored the prognostic potential of radiomics in 282 patients with early-stage no-small cell lung cancer and revealed that the integrated nomogram (C-index =0.720) exhibited superior predictive capabilities compared with the clinical model (C-index =0.629) and radiomics signature (C-index =0.617) (30). An integrated analysis of clinicopathological and MRI imaging information may provide a more comprehensive understanding of patient prognosis.
Similar to treatment decisions, surveillance strategies should be carefully balanced in terms of risks and benefits (31). Our model effectively stratified patients with IBTR into a low and high risk of subsequent recurrence, demonstrating consistent predictive performance in the postoperative period. These findings have implications for risk stratification and individualized follow-up strategies in those with IBTR. High-risk patients should receive more intensive follow-up, especially after 1 year, accompanied by tailored systemic therapies. Incorporating whole-body imaging techniques, such as positron emission tomography-computed tomography (PET/CT), is a reasonable approach in these cases. Previous studies have highlighted the efficacy of PET/CT in detecting previously unrecognized distant metastases, underscoring its value for postsurgical surveillance (32,33). The incorporation of PET/CT into the postoperative surveillance protocol can enhance the detection of potential metastatic disease and thus lead to improved patient outcomes.
This study involved certain limitations which should be acknowledged. First, we employed a retrospective, single-center design. In addition, the incidence of IBTR after BCS is low, and preoperative MRI for BCS and IBTR surgery is not mandatory in our institution; thus, it was difficult to collect matched MRI scans. As a result, the generalizability of our findings may be limited. Additionally, the lack of external validation emphasizes the need for further validation. Although we used a bootstrap-based internal validation technique to assess the generalizability, prospective multicenter validation is necessary to establish the robustness of our findings. Second, our study confirmed the predictive value of quantitative features derived from DCE-MRI for assessing the metastatic risk in IBTR. Subsequent research endeavors should focus on applying multiparametric MRI. Finally, multiomics analysis is a rapidly evolving field in research (34-36). Our objective is to extract quantitative radiomics features and integrate multiomics data, which may lead to the development of therapeutic targets for mitigating the risk of recurrence in IBTR.
Conclusions
Both qualitative and quantitative MRI features of recurrent lesions demonstrated promising value in predicting the prognosis of patients with IBTR following resection. Furthermore, the integration of clinicopathological features and MRI data exhibited efficacy in predicting metastatic risk and has the potential to inform tailored follow-up strategies. Additional multicenter, prospective studies are necessary to comprehensively investigate the clinical utility of MRI-based characteristics.
Acknowledgments
Funding: This study was supported by grants from
Footnote
Reporting Checklist: The authors have completed the TRIPOD reporting checklist. Available at https://qims.amegroups.com/article/view/10.21037/qims-23-1831/rc
Conflicts of Interest: All authors have completed the ICMJE uniform disclosure form (available at https://qims.amegroups.com/article/view/10.21037/qims-23-1831/coif). The authors have no conflicts of interest to declare.
Ethical Statement: The authors are accountable for all aspects of the work in ensuring that questions related to the accuracy or integrity of any part of the work are appropriately investigated and resolved. This study was conducted in accordance with the Declaration of Helsinki (as revised in 2013) and was approved by the Ethics Committee of the Institutional Review Board (IRB) of Fudan University Shanghai Cancer Center (FUSCC) (No. 050432-4-2307E). The requirement for individual consent of this retrospective analysis was waived.
Open Access Statement: This is an Open Access article distributed in accordance with the Creative Commons Attribution-NonCommercial-NoDerivs 4.0 International License (CC BY-NC-ND 4.0), which permits the non-commercial replication and distribution of the article with the strict proviso that no changes or edits are made and the original work is properly cited (including links to both the formal publication through the relevant DOI and the license). See: https://creativecommons.org/licenses/by-nc-nd/4.0/.
References
- Siegel RL, Miller KD, Wagle NS, Jemal A. Cancer statistics, 2023. CA Cancer J Clin 2023;73:17-48. [Crossref] [PubMed]
- van Maaren MC, de Munck L, de Bock GH, Jobsen JJ, van Dalen T, Linn SC, Poortmans P, Strobbe LJA, Siesling S. 10 year survival after breast-conserving surgery plus radiotherapy compared with mastectomy in early breast cancer in the Netherlands: a population-based study. Lancet Oncol 2016;17:1158-70. [Crossref] [PubMed]
- Veronesi U, Cascinelli N, Mariani L, Greco M, Saccozzi R, Luini A, Aguilar M, Marubini E. Twenty-year follow-up of a randomized study comparing breast-conserving surgery with radical mastectomy for early breast cancer. N Engl J Med. 2002;347:1227-32. [Crossref] [PubMed]
- Flanagan MR, Zabor EC, Romanoff A, Fuzesi S, Stempel M, Mehrara BJ, Morrow M, Pusic AL, Gemignani ML. A Comparison of Patient-Reported Outcomes After Breast-Conserving Surgery and Mastectomy with Implant Breast Reconstruction. Ann Surg Oncol 2019;26:3133-40. [Crossref] [PubMed]
- Bouganim N, Tsvetkova E, Clemons M, Amir E. Evolution of sites of recurrence after early breast cancer over the last 20 years: implications for patient care and future research. Breast Cancer Res Treat 2013;139:603-6. [Crossref] [PubMed]
- Sanghani M, Truong PT, Raad RA, Niemierko A, Lesperance M, Olivotto IA, Wazer DE, Taghian AG. Validation of a web-based predictive nomogram for ipsilateral breast tumor recurrence after breast conserving therapy. J Clin Oncol 2010;28:718-22. [Crossref] [PubMed]
- Galper S, Blood E, Gelman R, Abner A, Recht A, Kohli A, Wong JS, Smith D, Bellon J, Connolly J, Schnitt S, Winer E, Silver B, Harris JR. Prognosis after local recurrence after conservative surgery and radiation for early-stage breast cancer. Int J Radiat Oncol Biol Phys 2005;61:348-57. [Crossref] [PubMed]
- Sopik V, Lim D, Sun P, Narod SA. Prognosis after Local Recurrence in Patients with Early-Stage Breast Cancer Treated without Chemotherapy. Curr Oncol 2023;30:3829-44. [Crossref] [PubMed]
- Wu HL, Lu YJ, Li JW, Wu SY, Chen XS, Liu GY. Prior Local or Systemic Treatment: A Predictive Model Could Guide Clinical Decision-Making for Locoregional Recurrent Breast Cancer. Front Oncol 2021;11:791995. [Crossref] [PubMed]
- Qu FL, Mao R, Liu ZB, Lin CJ, Cao AY, Wu J, Liu GY, Yu KD, Di GH, Li JJ, Shao ZM. Spatiotemporal Patterns of Loco-Regional Recurrence After Breast-Conserving Surgery. Front Oncol 2021;11:690658. [Crossref] [PubMed]
- Baek SY, Kim J, Chung IY, Ko BS, Kim HJ, Lee JW, Son BH, Ahn SH, Lee SB. Clinical Course and Predictors of Subsequent Recurrence and Survival of Patients With Ipsilateral Breast Tumor Recurrence. Cancer Control 2022;29:10732748221089412. [Crossref] [PubMed]
- Buchholz TA, Ali S, Hunt KK. Multidisciplinary Management of Locoregional Recurrent Breast Cancer. J Clin Oncol 2020;38:2321-8. [Crossref] [PubMed]
- Shin SU, Cho N, Lee HB, Kim SY, Yi A, Kim SY, Lee SH, Chang JM, Moon WK. Neoadjuvant Chemotherapy and Surgery for Breast Cancer: Preoperative MRI Features Associated with Local Recurrence. Radiology 2018;289:30-8. [Crossref] [PubMed]
- Lee SH, Jang MJ, Yoen H, Lee Y, Kim YS, Park AR, Ha SM, Kim SY, Chang JM, Cho N, Moon WK. Background Parenchymal Enhancement at Postoperative Surveillance Breast MRI: Association with Future Second Breast Cancer Risk. Radiology 2023;306:90-9. [Crossref] [PubMed]
- Lim Y, Ko ES, Han BK, Ko EY, Choi JS, Lee JE, Lee SK. Background parenchymal enhancement on breast MRI: association with recurrence-free survival in patients with newly diagnosed invasive breast cancer. Breast Cancer Res Treat 2017;163:573-86. [Crossref] [PubMed]
- Bae MS, Chang JM, Cho N, Han W, Ryu HS, Moon WK. Association of preoperative breast MRI features with locoregional recurrence after breast conservation therapy. Acta Radiol 2018;59:409-17. [Crossref] [PubMed]
- Bae MS, Shin SU, Ryu HS, Han W, Im SA, Park IA, Noh DY, Moon WK, Pretreatment MR. Imaging Features of Triple-Negative Breast Cancer: Association with Response to Neoadjuvant Chemotherapy and Recurrence-Free Survival. Radiology 2016;281:392-400. [Crossref] [PubMed]
- Mayerhoefer ME, Materka A, Langs G, Häggström I, Szczypiński P, Gibbs P, Cook G. Introduction to Radiomics. J Nucl Med 2020;61:488-95. [Crossref] [PubMed]
- Ji GW, Zhu FP, Xu Q, Wang K, Wu MY, Tang WW, Li XC, Wang XH. Radiomic Features at Contrast-enhanced CT Predict Recurrence in Early Stage Hepatocellular Carcinoma: A Multi-Institutional Study. Radiology 2020;294:568-79. [Crossref] [PubMed]
- Wang X, Xie T, Luo J, Zhou Z, Yu X, Guo X. Radiomics predicts the prognosis of patients with locally advanced breast cancer by reflecting the heterogeneity of tumor cells and the tumor microenvironment. Breast Cancer Res 2022;24:20. [Crossref] [PubMed]
- Wang S, Wang Z, Li R, You C, Mao N, Jiang T, Wang Z, Xie H, Gu Y. Association between quantitative and qualitative image features of contrast-enhanced mammography and molecular subtypes of breast cancer. Quant Imaging Med Surg 2022;12:1270-80. [Crossref] [PubMed]
- Allison KH, Hammond MEH, Dowsett M, McKernin SE, Carey LA, Fitzgibbons PL, Hayes DF, Lakhani SR, Chavez-MacGregor M, Perlmutter J, Perou CM, Regan MM, Rimm DL, Symmans WF, Torlakovic EE, Varella L, Viale G, Weisberg TF, McShane LM, Wolff AC. Estrogen and Progesterone Receptor Testing in Breast Cancer: ASCO/CAP Guideline Update. J Clin Oncol 2020;38:1346-66. [Crossref] [PubMed]
- Wolff AC, Hammond MEH, Allison KH, Harvey BE, Mangu PB, Bartlett JMS, Bilous M, Ellis IO, Fitzgibbons P, Hanna W, Jenkins RB, Press MF, Spears PA, Vance GH, Viale G, McShane LM, Dowsett M. Human Epidermal Growth Factor Receptor 2 Testing in Breast Cancer: American Society of Clinical Oncology/College of American Pathologists Clinical Practice Guideline Focused Update. Arch Pathol Lab Med 2018;142:1364-82. [Crossref] [PubMed]
- Tolaney SM, Garrett-Mayer E, White J, Blinder VS, Foster JC, Amiri-Kordestani L, et al. Updated Standardized Definitions for Efficacy End Points (STEEP) in Adjuvant Breast Cancer Clinical Trials: STEEP Version 2.0. J Clin Oncol 2021;39:2720-31. [Crossref] [PubMed]
- Camp RL, Dolled-Filhart M, Rimm DL. X-tile: a new bio-informatics tool for biomarker assessment and outcome-based cut-point optimization. Clin Cancer Res 2004;10:7252-9. [Crossref] [PubMed]
- Schröder MS, Culhane AC, Quackenbush J, Haibe-Kains B. survcomp: an R/Bioconductor package for performance assessment and comparison of survival models. Bioinformatics 2011;27:3206-8. [Crossref] [PubMed]
- Gennaro M, Di Cosimo S, Ardoino I, Veneroni S, Mariani L, Agresti R, Daidone MG, de Braud F, Apolone G, Biganzoli E, Demicheli R. Dynamics of the hazard for distant metastases after ipsilateral breast tumor recurrence according to estrogen receptor status: An analysis of 2851 patients. Breast 2018;40:131-5. [Crossref] [PubMed]
- Söderqvist G, Isaksson E, von Schoultz B, Carlström K, Tani E, Skoog L. Proliferation of breast epithelial cells in healthy women during the menstrual cycle. Am J Obstet Gynecol 1997;176:123-8. [Crossref] [PubMed]
- Murata T, Yoshida M, Shiino S, Ogawa A, Watase C, Satomi K, Jimbo K, Maeshima A, Iwamoto E, Takayama S, Suto A. A prediction model for distant metastasis after isolated locoregional recurrence of breast cancer. Breast Cancer Res Treat 2023;199:57-66. [Crossref] [PubMed]
- Huang Y, Liu Z, He L, Chen X, Pan D, Ma Z, Liang C, Tian J, Liang C. Radiomics Signature: A Potential Biomarker for the Prediction of Disease-Free Survival in Early-Stage (I or II) Non-Small Cell Lung Cancer. Radiology 2016;281:947-57. [Crossref] [PubMed]
- Lee AY. The Importance of Studying Our Surveillance Strategies. Ann Surg Oncol 2022;29:2745-6. [Crossref] [PubMed]
- Ulaner GA, Castillo R, Goldman DA, Wills J, Riedl CC, Pinker-Domenig K, Jochelson MS, Gönen M. (18)F-FDG-PET/CT for systemic staging of newly diagnosed triple-negative breast cancer. Eur J Nucl Med Mol Imaging 2016;43:1937-44. [Crossref] [PubMed]
- Ulaner GA, Castillo R, Wills J, Gönen M, Goldman DA. (18)F-FDG-PET/CT for systemic staging of patients with newly diagnosed ER-positive and HER2-positive breast cancer. Eur J Nucl Med Mol Imaging 2017;44:1420-7. [Crossref] [PubMed]
- Jiang L, You C, Xiao Y, Wang H, Su GH, Xia BQ, Zheng RC, Zhang DD, Jiang YZ, Gu YJ, Shao ZM. Radiogenomic analysis reveals tumor heterogeneity of triple-negative breast cancer. Cell Rep Med 2022;3:100694. [Crossref] [PubMed]
- Su GH, Jiang L, Xiao Y, Zheng RC, Wang H, Jiang YZ, Peng WJ, Shao ZM, Gu YJ, You C. A Multiomics Signature Highlights Alterations Underlying Homologous Recombination Deficiency in Triple-Negative Breast Cancer. Ann Surg Oncol 2022;29:7165-75. [Crossref] [PubMed]
- Su GH, Xiao Y, Jiang L, Zheng RC, Wang H, Chen Y, Gu YJ, You C, Shao ZM. Radiomics features for assessing tumor-infiltrating lymphocytes correlate with molecular traits of triple-negative breast cancer. J Transl Med 2022;20:471. [Crossref] [PubMed]