Prediction of glypican-3 expression in hepatocellular carcinoma using multisequence magnetic resonance imaging-based histology nomograms
Introduction
Hepatocellular carcinoma (HCC) is one of the most prevalent malignant liver tumors and has an incidence that is increasing globally, with a 3- to 5-year survival rate ranging between 5% and 15% (1,2). Additionally, HCC accounts for 75–85% of all liver primary cancers and is the primary pathological type of liver cancer. Despite advances in the treatment of HCC, 80% of patients with HCC are diagnosed in the intermediate to advanced stages, leading to a poor prognosis (3).
The genesis of HCC is often associated with the overexpression of a variety of proteins and receptors. Among these, the levels of the glypican-3 (GPC3) gene and protein expression in HCC tumor tissues have been found to be significantly higher than those in normal liver tissues. GPC3, a glypican, is expressed during embryonic development and in adult ovaries, among other tissues, but not in healthy adult livers (4). One study found that high GPC3 expression is closely associated with serum α-fetoprotein (AFP) levels, and this can serve as a diagnostic and prognostic biomarker for HCC (5). GPC3 has emerged as an effective target for therapy in HCC, and it has been found that patients with high levels of GPC3 have shorter survival than do those with low GPC3 levels (6,7). Consequently, the preoperative assessment of GPC3 expression can be beneficial in clinical decision-making and in enhancing the treatment and prognosis of patients with HCC.
Presently, the preoperative assessment of GPC3 expression primarily relies on the liver biopsy examination, which is an invasive procedure that often fails to capture the overall heterogeneity of the tumor and poses a risk of tumor dissemination. Conventional imaging captures only basic features such as the size, location, and enhancement pattern of lesions and organs (8). As a recent and emerging noninvasive diagnostic modality, radiomics has been used to extract high-throughput radiological data from quantitative image features (9). This technology assists in the diagnosis and prediction of clinical outcomes while allowing for the in-depth characterization of different genetic tumor traits across various clinical scenarios and is thus referred to as virtual biopsy technology. Radiomics technologies have improved the generalization and universality of the noninvasive diagnosis, prognostication, and decision-making in tumor treatment.
Previous studies have demonstrated a close link between GPC3 expression and the histological features of magnetic resonance imaging (MRI). Radiomics models constructed derived from diffusion-weighted histograms, gadoxetic acid-enhanced MRI three-dimensional quantitative analysis, and iterative decomposition of water and fat with echo asymmetry and least-squares estimation quantitation sequence (IDEAL-IQ) have proven efficacious in assessing GPC3 expression levels in intrahepatic HCC (10-14).
However, most of these previous studies have reported the extraction of histological features based on single MRI sequences or enhanced sequences. There is currently no evidence of the efficacy of multisequence MRI imaging for histological analysis in assessing levels of GPC3 expression. Compared to single sequences, multimodality MRI sequences offer more comprehensive information on overall tumor heterogeneity and yield more reliable diagnostic results. Therefore, in this study, our aim was to examine the significance of preoperative multisequence MRI imaging features in predicting levels of GPC3 expression in patients with HCC and to develop nomograms for assessing the clinical utility of predicting GPC3 expression. We present this article in accordance with the TRIPOD reporting checklist (available at https://qims.amegroups.com/article/view/10.21037/qims-24-111/rc).
Methods
General data
This study retrospectively collected the archived data of 395 patients who had undergone radical hepatectomy and were pathologically diagnosed with HCC at the Affiliated Hospital of Hebei University (HBU) from January 2019 to June 2023. A total of 123 eligible cases were selected after inclusion and exclusion criteria were applied, and these cases were used in the construction and testing of prediction models. In addition, the data of 40 patients with pathologically confirmed HCC were downloaded from The Cancer Imaging Archive (TCIA) website (https://dev.cancerimagingarchive.net), with abdominal MRI image data and corresponding extensible markup language (XML) clinical data available for each patient. A total of 20 eligible patients were screened out after inclusion and exclusion criteria to be used as an independent external validation set in evaluating the generalizability of the model. This study was conducted in accordance with the Declaration of Helsinki (as revised in 2013) and was approved by Ethics Committee of the Affiliated Hospital of Hebei University (No. HDFYLL-KY-2024-019). Written informed consent was obtained from all participants.
The inclusion criteria were as follows: (I) a diagnosis of HCC confirmed through histopathology of the primary tumor at the time of surgery or biopsy; (II) complete MRI imaging data; (III) complete clinical data, including age, gender, GPC3 expression status, preoperative serum AFP levels, presence of liver cirrhosis, and the presence of microvascular invasion (MVI) around the tumor; and (IV) for those in the TCIA, a complete plain scan, dynamic enhanced MRI images, and RNA sequencing data from The Cancer Genome Atlas (TCGA) (https://portal.gdc.cancer.gov/).
The exclusion criteria were as follows: (I) previous treatments prior to surgery and (II) low-quality liver MRI images or pathologic tissue images (Figure 1).
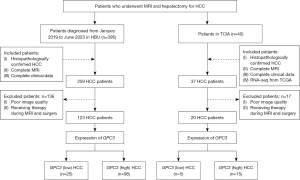
Histopathology
A pathologist with 10 years of clinical experience retrospectively analyzed all HCC samples. To minimize the occurrence of false-positive results, the immunoreactivity profile was assessed by measuring the percentage of GPC3 positivity. In this context, we defined GPC3 high expression as the presence of immunoreactivity in ≥5% of the tumors. Samples with GPC3 immunoreactivity in tumor cells less than 5% were considered to be low expression. We defined MVI as the presence of a tumor thrombus in the small blood vessels surrounding the tumor detectable only under microscopic examination.
MRI instruments and methods
The 1.5 T Amira (Siemens Healthineers, Erlangen, Germany) and 3.0 T Discovery 750HD (GE HealthCare, Chicago, IL, USA) MR scanners equipped with a dedicated eight-channel phased-array coil for abdominal imaging were used for imaging the patients. All patients in our cohort underwent an MRI scan with or without contrast, which was performed using an EZEM double-barrel high-pressure injector (E-Z-EM Inc., USA) with gadobenate dimeglumine (Gd-EOB-DTPA) as the contrast agent. The imaging parameters for axial liver acquisition with volume acceleration (LAVA) flex parameters were as follows: repetition time (TR), 3.7 ms; echo time (TE), 1.7 ms; slice thickness, 4 mm; and matrix, 256×256. Meanwhile, the parameters for coronal single-shot fast spin echo (SSFSE) T2-weighted imaging (T2WI) (assisted localization) were as follows: TR, 3,000 ms; TE, 66.7 ms; slice thickness, 5 mm; interslice gap, 1 mm; and matrix, 288×288.
The aforementioned sequences were obtained via breath-holding techniques. T2WI was implemented with respiration-triggered scanning under the following parameters: TR, 3,300–5,000 ms (respiration related); TE, 67.3 ms, slice thickness, 4 mm; interslice gap, 1 mm; and matrix, 320×320. Adjustments in the field of view for conventional MR scanning sequences were contingent upon the dimensions of the respective study participant.
The contrast agent used for enhancement was Gd-EOB-DTPA. The procedure for dynamic imaging with contrast involved using the 3D-LAVA fat-suppression sequence under the following parameters: TE, 2.2; TR, 4.5 ms; layer thickness, 3.0 mm; and matrix 320×192. Moreover, delayed scanning was performed at 15–20 s, 35–40 s, 60 s, 180 s, and 90–120 min after the injection of contrast agent to obtain early arterial, late arterial, portal venous, delayed, and hepatobiliary phase (HBP) images, respectively. The scanning range extended from the apex of diaphragm to the lower margin of right lobe of the liver.
All injections were administered through the forearm vein using a high-pressure injector, with a contrast dose of 0.1 mmol/kg and an injection rate of 2 mL/s. Postcontrast injection, 30 mL of saline was administered at the same rate for lavage.
Radiomics analysis
Image acquisition and region of interest (ROI) segmentation
Two radiologists with 5 years of clinical experience independently evaluated the MRI images. Radiomics analyses of all eligible T2WI and diffusion-weighted imaging (DWI), along with arterial phase (AP), venous phase (VP), delayed phase (DP), and HBP images and the image database management were performed using the United Image Intelligent Research Platform System v. 1.0 (Shanghai Lianying Intelligent Medical Technology Co., Ltd., Shanghai, China) (15).
The ROIs were manually outlined along the contours of the lesions on the enhanced-scan HBP images and replicated onto each serial image to ensure that the size and location of the ROIs matched between the images. The reviewer only knew that the patient had HCC but was blinded to all other information, including the patient’s medical history, laboratory results, and pathology findings.
Interobserver agreement was assessed after the initial independent image analyses. In cases of differences in assessment, the two reviewers discussed among themselves and reached a final consensus. When multiple nodules were observed, only the largest nodule was analyzed. Pathology image matching was performed based on the size and location of the lesion to ensure that the tested specimen correlated with the lesion evaluated on an MRI. Finally, we selected the group characteristics with an intraclass correlation coefficient (ICC) value >0.75 among all the reviewers for follow-up.
Radiomics feature extraction and modeling
First, the original images of lesions from all patients’ MRI sequences (T2WI, DWI, AP, VP, DP, and HBP) were resampled using the maximum–minimum truncation algorithm to minimize the dimensional differences in the image data. The radiomics features of ROIs labeled by each MRI sequence, including first-order, texture, and higher-order features, were then extracted. The texture and higher-order features included the gray-level run-length matrix (GLRLM), gray-level co-occurrence matrix (GLCM), gray-level size zone matrix (GLSZM), and neighboring gray-tone difference matrix (NGTDM) wavelet transforms, as well as Laplacian sharpening.
In the process of using fivefold cross-validation for feature selection, the extracted features were normalized using the z-score method to reduce the dimensional differences between the different features. To prevent overfitting, we conducted an additional round of feature selection. Initially, we retained features with a P value <0.05 according to Pearson correlation coefficient analysis. Subsequently, we performed a deredundant screening using least absolute shrinkage and selection operator (LASSO) analysis, retaining those features that occurred more than twice in five folds. The radiomics score (rad-score) was calculated based on the selected features and their corresponding coefficients using the following formula: rad-score = constant + feature × coefficient.
We used logistic regression (LR) classifiers to develop predictive models for individual sequences and joint sequences. The joint sequences included contrast-enhanced MRI (CE-MRI) (AP + VP + DP + HBP) and multiparametric MRI (mpMRI) (T2WI + DWI + AP + VP + DP + HBP). We evaluated the model’s performance using data from the test set of the cross-validation. Finally, we constructed nomograms based on rad-score and clinical predictors and externally validated the nomograms using data from the TCIA (n=20).
Statistical analysis
We used SPSS software version 26.0 (IBM Corp., Armonk, NY, USA) and R software version 3.6.3 (www.rproject.org; The R Foundation for Statistical Computing) for all statistical analyses and visualizations in this study. The Kolmogorov-Smirnov test was used to test the normality of the data, and the Levene method was used for the chi-square test. We used the ICC for evaluating the interrater consistency among all reviewers. We retained radiomic features with ICC values >0.75 for subsequent analysis and processing.
The independent samples t-test or Mann-Whitney test was used for comparisons of continuous variables, while the Pearson χ2 test or Fisher exact probability method was used to compare categorical variables. Univariate and multivariate LR analyses were conducted to evaluate clinical factors that showed significant differences (P<0.05) in correlation with GPC3.
Using receiver operating characteristic (ROC) curves, we evaluated the effectiveness of the radiomics model in distinguishing GPC3 expression in HCC. In addition, we used calibration curves and decision curves to assess the accuracy of the models and computed Brier scores for each model. For statistical analyses, differences were considered statistically significant at a P value of <0.05. The overall methodological flowchart is shown in Figure 2.
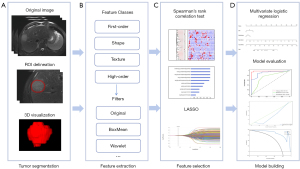
Results
Baseline patient information
Tables 1 and 2 summarize the basic clinical characteristics of 123 patients with HCC in HBU and 20 patients with HCC in the TCIA/TCGA database. The group of patients with HCC and high GPC3 expression included a greater portion of males and those with cirrhosis than did the GPC3-negative group. HCC patients in the HBU center showed significant differences in tumor size (P=0.040), MVI status (P=0.001), and tumor morphology (P=0.002) between the GPC3 high- and low-expression groups (P<0.05). There was a significant difference in the tumor size of patients with HCC (P=0.024) between the GPC3 high- and low-expression groups from the TCIA (P<0.05).
Table 1
Clinical features | HBU | P value | |
---|---|---|---|
GPC3 high (n=98) | GPC3 low (n=25) | ||
Age (years), median ± SD | 58.5±12.1 | 59.6±9.5 | 0.688 |
Gender, n (%) | 0.450 | ||
Male | 80 (81.6) | 22 (88.0) | |
Female | 18 (18.4) | 3 (12.0) | |
AFP, n (%), ng/mL | 0.308 | ||
≤400 | 64 (65.3) | 19 (76.0) | |
>400 | 34 (34.7) | 6 (24.0) | |
Cirrhosis, n (%) | 0.288 | ||
Yes | 66 (67.3) | 14 (56.0) | |
No | 32 (32.7) | 11 (44.0) | |
BCLC classification, n (%) | 0.202 | ||
0 | 23 (23.5) | 6 (24.0) | |
A | 42 (42.9) | 15 (60.0) | |
B | 21 (21.4) | 4 (16.0) | |
C | 12 (12.2) | 0 (0.0) | |
Tumor size (cm), median ± SD | 6.1±4.0 | 4.3±2.6 | 0.040 |
MVI, n (%) | 0.001 | ||
Positive | 52 (53.1) | 4 (84.0) | |
Negative | 46 (46.9) | 21 (16.0) | |
Morphology, n (%) | 0.002 | ||
Regular | 41 (41.8) | 19 (76.0) | |
Irregular | 57 (58.2) | 6 (24.0) | |
Boundary, n (%) | 0.088 | ||
Clear | 52 (53.1) | 18 (72.0) | |
Unclear | 46 (46.9) | 7 (28.0) |
HCC, hepatocellular carcinoma; HBU, Affiliated Hospital of Hebei University; GPC3, glypican-3; SD, standard deviation; AFP, α-fetoprotein; BCLC, Barcelona Clinic Liver Cancer; MVI, microvascular invasion.
Table 2
Clinical features | TCIA | P value | |
---|---|---|---|
GPC3 high (n=15) | GPC3 low (n=5) | ||
Age (years), median ± SD | 63.5±10.8 | 60.6±9.9 | 0.606 |
Gender, n (%) | 0.573 | ||
Male | 11 (73.3) | 3 (60.0) | |
Female | 4 (26.7) | 2 (40.0) | |
Cirrhosis, n (%) | 0.776 | ||
Yes | 11 (73.3) | 4 (80.0) | |
No | 4 (26.7) | 1 (20.0) | |
BCLC classification, n (%) | 0.577 | ||
0 | 2 (13.3) | 0 (0.0) | |
A | 7 (46.7) | 4 (80.0) | |
B | 5 (33.3) | 1 (20.0) | |
C | 1 (6.7) | 0 (0.0) | |
Tumor size (cm), median ± SD | 7.1±3.2 | 3.4±1.2 | 0.024 |
MVI, n (%) | 0.091 | ||
Positive | 12 (80.0) | 2 (40.0) | |
Negative | 3 (20.0) | 3 (60.0) | |
Morphology, n (%) | 0.050 | ||
Regular | 2 (13.3) | 4 (80.0) | |
Irregular | 13 (86.7) | 1 (20.0) | |
Boundary, n (%) | 0.573 | ||
Clear | 4 (26.7) | 2 (60.0) | |
Unclear | 11 (73.3) | 3 (40.0) |
HCC, hepatocellular carcinoma; TCIA, The Cancer Imaging Archive; GPC3, glypican-3; SD, standard deviation; BCLC, Barcelona Clinic Liver Cancer; MVI, microvascular invasion.
The results of the univariate and multivariate LR analyses are shown in Tables 3 and 4. Using univariate LR analysis, we initially screened all clinical characteristics and found that differences in tumor size [hazard ratio (HR) =1.170, 95% confidence interval (CI): 1.002–1.367; P=0.047], morphology (HR =4.402; 95% CI: 1.617–11.988; P=0.004), and MVI (HR =0.168; 95% CI: 0.054–0.527; P=0.002) were statistically significant (P<0.05). All factors were further analyzed using multivariable forward stepwise LR, which revealed that the independent risk factors for predictive modeling were morphology (HR =3.619; 95% CI: 1.285–10.189; P=0.015) and MVI (HR =0.201; 95% CI: 0.063–0.642; P=0.007).
Table 3
Category | Univariate analysis | |
---|---|---|
HR (95% CI) | P value | |
Age (years) | 1.650 (0.656–6.116) | 0.454 |
Gender | 0.992 (0.955–1.031) | 0.992 |
Tumor size | 1.170 (1.002–1.367) | 0.047* |
Morphology | 4.402 (1.617–11.988) | 0.004* |
Boundary | 2.275 (0.872–5.935) | 0.093 |
AFP | 0.594 (0.217–1.628) | 0.312 |
MVI | 0.168 (0.054–0.527) | 0.002* |
Cirrhosis | 0.617 (0.252–1.511) | 0.291 |
*, indicates a statistical difference (P<0.05). GPC3, glypican-3; HR, hazard ratio; CI, confidence interval; AFP, α-fetoprotein; MVI, microvascular invasion.
Table 4
Category | Multivariate analysis | |
---|---|---|
HR (95% CI) | P value | |
Morphology | 3.619 (1.285–10.189) | 0.015** |
MVI | 0.201 (0.063–0.642) | 0.007** |
**, indicates variables included in the equation after multivariate logistic forward stepwise regression. GPC3, glypican-3; HR, hazard ratio; CI, confidence interval; MVI, microvascular invasion.
Feature selection and establishment of the MRI imaging histology model
We conducted a consistency test on the extracted tumor features and identified 16,377 sequences for each of the histological features that exhibited good consistency (ICC >0.75). After screening, in each sequence model of T2WI, DWI, AP, VP, DP, and HBP, we retained 13, 11, 10, 10, 5, and 5 nonzero coefficient features, respectively. In addition, we retained 5 and 10 nonzero coefficient features in the CE-MRI and mpMRI models, respectively (Figure 3).
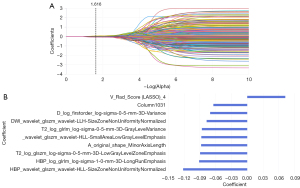
The performance metrics for preoperatively predicting HCC GPC3 expression in the training and test groups of each sequence model are outlined in Tables 5 and 6. Among the single-sequence MRI-based radiomics models, the AP sequence prediction model demonstrated superior performance, with an area under the curve (AUC) of 0.812 (95% CI: 0.623–1) in the test cohort and a sensitivity, specificity, and accuracy of over 70%. In addition, the combined MRI multiple sequences model demonstrated the most promising performance in predicting GPC3 expression in patients with HCC, with AUCs of 0.911 (95% CI: 0.844–0.978) and 0.979 (95% CI: 0.931–1) in the training set and test set, respectively. It also exhibited a sensitivity of 0.947, a specificity of 0.800, and an accuracy of 0.917 in the test set.
Table 5
Training group | Indicator | |||
---|---|---|---|---|
AUC (95% CI) | ACC | SEN | SPE | |
T2WI | 0.914 (0.835–0.994) | 0.753 | 0.723 | 0.875 |
DWI | 0.854 (0.734–0.973) | 0.831 | 0.851 | 0.750 |
AP | 0.873 (0.761–0.985) | 0.779 | 0.790 | 0.733 |
VP | 0.952 (0.891–1) | 0.931 | 0.936 | 0.909 |
DP | 0.986 (0.962–1) | 0.964 | 0.933 | 0.909 |
HBP | 0.807 (0.672–0.941) | 0.815 | 0.849 | 0.684 |
CE-MRI | 0.980 (0.942–1) | 0.901 | 0.890 | 0.944 |
mpMRI | 0.911 (0.844–0.978) | 0.809 | 0.803 | 0.833 |
MRI, magnetic resonance imaging; AUC, area under curve; CI, confidence interval; ACC, accuracy; SEN, sensitivity; SPE, specificity; T2WI, T2-weighted imaging; DWI, diffusion-weighted imaging; AP, arterial phase; VP, venous phase; DP, delayed phase; HBP, hepatobiliary phase; CE-MRI, contrast-enhanced magnetic resonance imaging; mpMRI, multiparametric magnetic resonance imaging.
Table 6
Test group | Indicator | |||
---|---|---|---|---|
AUC (95% CI) | ACC | SEN | SPE | |
T2WI | 0.704 (0.507–0.901) | 0.629 | 0.643 | 0.571 |
DWI | 0.779 (0.581–0.978) | 0.762 | 0.765 | 0.750 |
AP | 0.812 (0.623–1) | 0.750 | 0.750 | 0.750 |
VP | 0.778 (0.534–1) | 0.733 | 0.750 | 0.667 |
DP | 0.792 (0.358–1) | 0.857 | 0.750 | 0.500 |
HBP | 0.803 (0.600–1) | 0.696 | 0.684 | 0.750 |
CE-MRI | 0.947 (0.853–1) | 0.826 | 0.842 | 0.750 |
mpMRI | 0.979 (0.931–1) | 0.917 | 0.947 | 0.800 |
MRI, magnetic resonance imaging; AUC, area under curve; CI, confidence interval; ACC, accuracy; SEN, sensitivity; SPE, specificity; T2WI, T2-weighted imaging; DWI, diffusion-weighted imaging; AP, arterial phase; VP, venous phase; DP, delayed phase; HBP, hepatobiliary phase; CE-MRI, contrast-enhanced magnetic resonance imaging; mpMRI, multiparametric magnetic resonance imaging.
Construction of GPC3 predictive nomograms
We constructed a predictive nomogram model by integrating tumor morphology, MVI, and mpMRI model rad-score scores (Figure 4). We found that the nomogram model demonstrated superior diagnostic accuracy in predicting GPC3 expression when compared to the other sequence models. The model achieved AUCs of 0.985 (95% CI: 0.959–1) and 0.989 (95% CI: 0.958–1) for the training and test sets, respectively. Additionally, it yielded a sensitivity, specificity, and accuracy of 0.944, 0.800, and 0.913, respectively, for the test set. The external validation AUC was 0.826. The calibration curves indicated that the nomogram demonstrated good agreement between predicted and observed GPC3 expression, with a strong fit between the predicted and actual curves (Brier score =0.029). The decision curve analysis (DCA) indicated that nomograms could yield a significant net gain when the threshold probability ranged from 10% to 95% (Figure 5). This suggests that the nomograms can be potentially used to enhance clinical outcomes by personalizing the prediction of GPC3 expression.
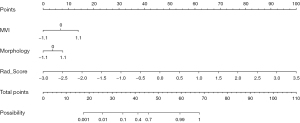
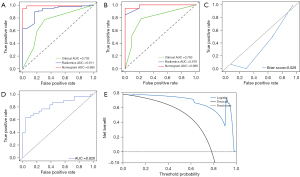
Discussion
The significance of this study lies in its contribution to the research on the noninvasive assessment of GPC3 expression in patients with HCC. We developed a machine learning-based MRI radiomics model for the preoperative prediction of GPC3 expression in patients with HCC. Additionally, we created nomograms that yielded favorable outcomes. We found that tumor morphology and MVI were independent factors associated with GPC3 expression. We also used LR modeling to construct radiomics models and nomograms for different MRI sequences, aiming to evaluate the models’ predictive performance. The nomogram, which integrated clinical factors and radiomics of multisequence MRI images, demonstrated an optimal diagnostic efficacy of 0.989 (95% CI: 0.958–1) in the test set, with a sensitivity of 0.944, a specificity of 0.800, and an accuracy of 0.913. The AUC in the independent external validation cohort was 0.826.
GPC3 is differentially expressed during the aggressive growth of HCC, suggesting that GPC3 may be involved in HCC development. Research on the related mechanism suggests that GPC3 contributes to HCC progression and metastasis by binding to molecules such as growth factors and Wnt signaling proteins, stimulating macrophage recruitment and epithelial-mesenchymal transition (16). It has been observed in clinical practice that most patients with high GPC3 expression and HCC have a poorer prognosis, experiencing higher recurrence rates and lower survival rates (17). Radiomics modeling has gradually become an important tool for predicting GPC3 expression in order to provide early warning or reduce the impact of high GPC3 expression in patients with HCC. However, few studies on using radiomics to predict GPC3 in patients with HCC have been conducted.
Zhang et al. (18) used a combination of radiomic features of Gd-EOB-DTPA in HBP and clinical factors to construct nomograms. Zhao et al. (10) used diffusion-weighted histogram analysis to assess the clinico-radiological variables associated with GPC3 expression. However, these studies only considered the predictive value that a single sequential feature provided to the model. Single-sequence MRI can only reflect the tumor morphology, black-and-white-signal contrast, composition, water molecular diffusion, and other singleton information, while the potential information feedback from the disease is limited and the potential for errors considerable. Multisequence MRI can not only visualize the morphological characteristics of tumors at the macro level but also further evaluate the biological development of tumors through MRI and hepatocellular-specific contrast agents. In this study, we extracted multiple sets of parameter data, including shape, first-order, texture, and higher-order features, from multisequence MRI. This approach allows for a more comprehensive reflection of the overall heterogeneity of the tumor, leading to a more accurate interpretation of the correlation between tumor GPC3 expression and clinico-radiological information (19).
Our results in this study showed that the AP model had the highest AUC in a single sequence, suggesting that AP images are more effective in reflecting tumor heterogeneity. In addition, the combined model demonstrated higher predictive performance than did the single-sequence model. The mpMRI model exhibited the best predictive performance, highlighting the critical value of multiple sequences in providing additional potential information about tumor heterogeneity (20-22).
DWI can noninvasively detect the diffusion of water molecules in living tissues. Although it can detect diffusion-restricted heterogeneity in tumors, but its imaging of radiomics in our study does not show significant incremental value in differentiating the expression of GPC3 in patients with HCC (23). This may be due to the small sample size and geographic variations of the related studies, warranting validation from multicenter large-scale data. Finally, the poor performance of prediction efficiency in external verification may be related to the size of sample data and the complexity of the model. Considering practical factors, future studies can focus on multicenter samples and deep learning model construction to improve the prediction efficiency and repeatability of the model.
Radiomics is beneficial for addressing tumor heterogeneity, as it can enhance traditional qualitative diagnosis in conventional imaging by compiling pixel-level data profiles from disease images. This allows for the mapping of the differential information that is imperceptible to the human eye (24-26). Most of the features in the prediction model that we constructed were processed using a local binary mode, with which each voxel was compared with its neighboring voxels, and the resulting comparison was saved as a binary number for the quantification of tumor heterogeneity characteristics.
We selected 10 imaging and radiomic features associated with GPC3 expression. These parameters describe the magnitude of voxel variation, adjacent spacing, orientation, homogeneous region characteristics, gray-level correlation, and grayscale relationship with neighboring pixels in an image. They assess textural differences in tumor images in terms of the uniformity of gray-level distribution, correlation, and complexity (27). Among these, three are wavelet features, while the remaining features consist of three texture and four first-order features. The screening indices were based on the GLSZM and GLRLM features, which describe the distance between different pixels or voxels within a specified neighborhood and provide information about the spatial distribution of consecutive pixels of the same gray level.
The HBP_wavelet_glszm_wavelet-HLL-SizeZoneNonUniformityNormalized feature carried the highest weight among the selected features. Derived from the HBP sequence, it reflects the diagnostic value of the HBP sequence features in the expression of GPC3. It describes the homogeneous region of GPC3 high expression in the HCC lesion site, indicating the heterogeneity of the lesion structure. In addition to this, the rad-score derived from radiomic features and their correlation coefficients has been used as a common measurement tool to improve the understanding of the relationship between clinical imaging, histological features, and tumor biological behaviors (28). In this study, we found that the prediction models constructed using clinical factors and the rad-score demonstrated good discrimination ability in the training, test cohorts, and external validation cohorts. This underscores the utility of radiomics imaging in the preoperative assessment of GPC3 expression in patients with HCC.
Our univariate regression analysis results revealed that tumor size and border were significant predictors of GPC3, but the multivariate analysis did not reveal any statistical significance. This finding was in contrast to previous studies. Dong et al. (11) reported that irregular tumor margins are a significant indicator of GPC3 and can reflect the biological characteristics of malignant tumors invading nontumor parenchyma that result in poorly defined tumor boundaries. In addition, Liu et al. (29) reported that the sensitivity of GPC3 expression and AFP level for diagnosing intrahepatic lesions was nearly identical. They also found that these indicators were effective in differentiating between HCC, cholangiocarcinoma, and cholangiocarcinoma combined with HCC in diagnostic models. However, in our study, the AFP levels did not show a significant difference, which may be attributable to the small sample size of our study.
In the multivariate regression analysis, both MVI and tumor morphology were found to be independent biomarkers for predicting GPC3. In several studies, irregular HCC tumor morphology and MVI were associated with early recurrence and a poor prognosis for HCC (30-32). In this study, we found that the incidence of HCC-MVI was higher in patients with high GPC3 expression than in GPC3-negative patients. Additionally, HCC lesions in the high-expression patients were often morphologically irregular, which may be explained by the higher tumor invasiveness of HCC in these patients. This invasiveness leads to a tendency to expand into the surrounding normal tissues and results in changes in the biological behavior and morphology of the tumor (33,34). These results support there being an association between GPC3 and malignant HCC, suggesting that GPC3 may contribute to tumor spread and recurrence by promoting the tumor microenvironment.
There are several limitations to our study that should be addressed. First, we employed a single-center retrospective analysis with a small and geographically diverse sample size, and most patients with HCC had a high GPC3 expression, which might have biased the results. Second, the data that we used for the external validation were primarily from public datasets. It is necessary to confirm whether these datasets can account for the occurrence of high GPC3 expression in HCC in the Chinese population through a large-sample, multicenter study. Moreover, there is little research on HCC-related radiogenomics, and thus the potential relationship between imaging features and tumor biological function needs to be verified by a large number of relevant studies. Future studies can focus more on the construction of multicenter samples and large models based on deep learning to improve the predictive efficiency and repeatability of models and achieve clinical transformation, which an provide accurate judgment of complex new data for various diseases.
Conclusions
MRI imaging, radiomics, tumor morphology, and MVI can noninvasively predict GPC3 expression in patients with HCC. Nomograms that integrate these indicators with clinical factors may provide valuable information for customizing individualized treatment plans for patients diagnosed with HCC before operation.
Acknowledgments
We are particularly grateful to all the people who have given us help in completing this study.
Funding: The study was supported by
Footnote
Reporting Checklist: The authors have completed the TRIPOD reporting checklist. Available at https://qims.amegroups.com/article/view/10.21037/qims-24-111/rc
Conflicts of Interest: All authors have completed the ICMJE uniform disclosure form (available at https://qims.amegroups.com/article/view/10.21037/qims-24-111/coif). J.J.C. is an employee of Beijing United Imaging Healthcare Co., Ltd. The other authors have no conflicts of interest to declare.
Ethical Statement: The authors are accountable for all aspects of the work in ensuring that questions related to the accuracy or integrity of any part of the work are appropriately investigated and resolved. This study was conducted in accordance with the Declaration of Helsinki (as revised in 2013) and was approved by the Ethics Committee of the Affiliated Hospital of Hebei University (No. HDFYLL-KY-2024-019). Written informed consent was obtained from all participants.
Open Access Statement: This is an Open Access article distributed in accordance with the Creative Commons Attribution-NonCommercial-NoDerivs 4.0 International License (CC BY-NC-ND 4.0), which permits the non-commercial replication and distribution of the article with the strict proviso that no changes or edits are made and the original work is properly cited (including links to both the formal publication through the relevant DOI and the license). See: https://creativecommons.org/licenses/by-nc-nd/4.0/.
References
- Bureau of Medical Administration, National Health Commission of the People's Republic of China. Standardization for diagnosis and treatment of hepatocellular carcinoma (2022 edition). Chin J Hepatol 2022;30:367-88. [PubMed]
- Singal AG, Lampertico P, Nahon P. Epidemiology and surveillance for hepatocellular carcinoma: New trends. J Hepatol 2020;72:250-61. [Crossref] [PubMed]
- Villanueva A. Hepatocellular Carcinoma. N Engl J Med 2019;380:1450-62. [Crossref] [PubMed]
- Nicolosi A, Gaia S, Risso A, Rosso C, Rolle E, Abate ML, Olivero A, Armandi A, Ribaldone DG, Carucci P, Fagoonee S, Pellicano R, Saracco GM, Bugianesi E, Caviglia GP. Serum glypican-3 for the prediction of survival in patients with hepatocellular carcinoma. Minerva Gastroenterol (Torino) 2022;68:378-86. [Crossref] [PubMed]
- Debes JD, Romagnoli PA, Prieto J, Arrese M, Mattos AZ, Boonstra AOn Behalf Of The Escalon Consortium. Serum Biomarkers for the Prediction of Hepatocellular Carcinoma. Cancers (Basel) 2021;13:1681. [Crossref] [PubMed]
- Xu D, Su C, Sun L, Gao Y, Li Y. Performance of Serum Glypican 3 in Diagnosis of Hepatocellular Carcinoma: A meta-analysis. Ann Hepatol 2019;18:58-67. [Crossref] [PubMed]
- Jeon Y, Kim H, Jang ES, Hong S, Kim JW, Yoon YS, Cho JY, Han HS, Jeong SH. Expression profile and prognostic value of glypican-3 in post-operative South Korean hepatocellular carcinoma patients. APMIS 2016;124:208-15. [Crossref] [PubMed]
- Guiot J, Vaidyanathan A, Deprez L, Zerka F, Danthine D, Frix AN, Lambin P, Bottari F, Tsoutzidis N, Miraglio B, Walsh S, Vos W, Hustinx R, Ferreira M, Lovinfosse P, Leijenaar RTH. A review in radiomics: Making personalized medicine a reality via routine imaging. Med Res Rev 2022;42:426-40. [Crossref] [PubMed]
- Bera K, Braman N, Gupta A, Velcheti V, Madabhushi A. Predicting cancer outcomes with radiomics and artificial intelligence in radiology. Nat Rev Clin Oncol 2022;19:132-46. [Crossref] [PubMed]
- Zhao J, Gao S, Sun W, Grimm R, Fu C, Han J, Sheng R, Zeng M. Magnetic resonance imaging and diffusion-weighted imaging-based histogram analyses in predicting glypican 3-positive hepatocellular carcinoma. Eur J Radiol 2021;139:109732. [Crossref] [PubMed]
- Dong SY, Sun W, Xu B, Wang WT, Yang YT, Chen XS, Zeng MS, Rao SX. Quantitative image features of gadoxetic acid-enhanced MRI for predicting glypican-3 expression of small hepatocellular carcinoma ≤3 cm. Clin Radiol 2023;78:e764-72. [Crossref] [PubMed]
- Chen R, Bai Y, Liu T, Zhang G, Han Y, Chen L, Gao H, Wei W, Wang M. Evaluation of Glypican-3 Expression in Hepatocellular Carcinoma by Using IDEAL IQ Magnetic Resonance Imaging. Acad Radiol 2021;28:e227-34. [Crossref] [PubMed]
- Gu D, Xie Y, Wei J, Li W, Ye Z, Zhu Z, Tian J, Li X. MRI-Based Radiomics Signature: A Potential Biomarker for Identifying Glypican 3-Positive Hepatocellular Carcinoma. J Magn Reson Imaging 2020;52:1679-87. [Crossref] [PubMed]
- Chong H, Gong Y, Zhang Y, Dai Y, Sheng R, Zeng M. Radiomics on Gadoxetate Disodium-enhanced MRI: Non-invasively Identifying Glypican 3-Positive Hepatocellular Carcinoma and Postoperative Recurrence. Acad Radiol 2023;30:49-63. [Crossref] [PubMed]
- Yu JH, Cho SG, Jin YJ, Lee JW. The best predictive model for hepatocellular carcinoma in patients with chronic hepatitis B infection. Clin Mol Hepatol 2022;28:351-61. [Crossref] [PubMed]
- Zhou F, Shang W, Yu X, Tian J. Glypican-3: A promising biomarker for hepatocellular carcinoma diagnosis and treatment. Med Res Rev 2018;38:741-67. [Crossref] [PubMed]
- Fu SJ, Qi CY, Xiao WK, Li SQ, Peng BG, Liang LJ. Glypican-3 is a potential prognostic biomarker for hepatocellular carcinoma after curative resection. Surgery 2013;154:536-44. [Crossref] [PubMed]
- Zhang N, Wu M, Zhou Y, Yu C, Shi D, Wang C, Gao M, Lv Y, Zhu S. Radiomics nomogram for prediction of glypican-3 positive hepatocellular carcinoma based on hepatobiliary phase imaging. Front Oncol 2023;13:1209814. [Crossref] [PubMed]
- Vosshenrich J, Zech CJ, Heye T, Boldanova T, Fucile G, Wieland S, Heim MH, Boll DT. Response prediction of hepatocellular carcinoma undergoing transcatheter arterial chemoembolization: unlocking the potential of CT texture analysis through nested decision tree models. Eur Radiol 2021;31:4367-76. [Crossref] [PubMed]
- Sun K, Shi L, Qiu J, Pan Y, Wang X, Wang H. Multi-phase contrast-enhanced magnetic resonance image-based radiomics-combined machine learning reveals microscopic ultra-early hepatocellular carcinoma lesions. Eur J Nucl Med Mol Imaging 2022;49:2917-28. [Crossref] [PubMed]
- Madani SP, Mirza-Aghazadeh-Attari M, Mohseni A, Pawlik T, Kamel IR. Diffuse infiltrative hepatocellular carcinoma: Multimodality imaging manifestations. J Surg Oncol 2023;127:385-93. [Crossref] [PubMed]
- Luo ZW, Yu HY, Liu HX, Li ZM, Wang T, Zang YC, Zhou XM. The value of radiomics models based on hepatobiliary phase images of Gd-EOB-DTPA enhanced MRI in prediction of microvascular invasion classification in hepatocellular carcinoma. Chin J Magn Reson Imaging 2023;14:95-101,114.
- Ai Z, Han Q, Huang Z, Wu J, Xiang Z. The value of multiparametric histogram features based on intravoxel incoherent motion diffusion-weighted imaging (IVIM-DWI) for the differential diagnosis of liver lesions. Ann Transl Med 2020;8:1128. [Crossref] [PubMed]
- Kim HY, Lampertico P, Nam JY, Lee HC, Kim SU, Sinn DH, et al. An artificial intelligence model to predict hepatocellular carcinoma risk in Korean and Caucasian patients with chronic hepatitis B. J Hepatol 2022;76:311-8. [Crossref] [PubMed]
- Wang Y, Gao B, Xia C, Peng X, Liu H, Wu S. Development of a novel tumor microenvironment-related radiogenomics model for prognosis prediction in hepatocellular carcinoma. Quant Imaging Med Surg 2023;13:5803-14. [Crossref] [PubMed]
- Liu J, Cheng D, Liao Y, Luo C, Lei Q, Zhang X, Wang L, Wen Z, Gao M. Development of a magnetic resonance imaging-derived radiomics model to predict microvascular invasion in patients with hepatocellular carcinoma. Quant Imaging Med Surg 2023;13:3948-61. [Crossref] [PubMed]
- Rimola J. Heterogeneity of Hepatocellular Carcinoma on Imaging. Semin Liver Dis 2020;40:61-9. [Crossref] [PubMed]
- Saini A, Breen I, Pershad Y, Naidu S, Knuttinen MG, Alzubaidi S, Sheth R, Albadawi H, Kuo M, Oklu R. Radiogenomics and Radiomics in Liver Cancers. Diagnostics (Basel) 2018;9:4. [Crossref] [PubMed]
- Liu S, Wang M, Zheng C, Zhong Q, Shi Y, Han X. Diagnostic value of serum glypican-3 alone and in combination with AFP as an aid in the diagnosis of liver cancer. Clin Biochem 2020;79:54-60. [Crossref] [PubMed]
- Chong HH, Yang L, Sheng RF, Yu YL, Wu DJ, Rao SX, Yang C, Zeng MS. Multi-scale and multi-parametric radiomics of gadoxetate disodium-enhanced MRI predicts microvascular invasion and outcome in patients with solitary hepatocellular carcinoma ≤ 5 cm. Eur Radiol 2021;31:4824-38. [Crossref] [PubMed]
- Mao Y, Wang J, Zhu Y, Chen J, Mao L, Kong W, Qiu Y, Wu X, Guan Y, He J. Gd-EOB-DTPA-enhanced MRI radiomic features for predicting histological grade of hepatocellular carcinoma. Hepatobiliary Surg Nutr 2022;11:13-24. [Crossref] [PubMed]
- Kong C, Zhao Z, Chen W, Lv X, Shu G, Ye M, Song J, Ying X, Weng Q, Weng W, Fang S, Chen M, Tu J, Ji J. Prediction of tumor response via a pretreatment MRI radiomics-based nomogram in HCC treated with TACE. Eur Radiol 2021;31:7500-11. [Crossref] [PubMed]
- Khatib SA, Wang XW. Causes and functional intricacies of inter- and intratumor heterogeneity of primary liver cancers. Adv Cancer Res 2022;156:75-102. [Crossref] [PubMed]
- Yang C, Zhang S, Cheng Z, Liu Z, Zhang L, Jiang K, Geng H, Qian R, Wang J, Huang X, Chen M, Li Z, Qin W, Xia Q, Kang X, Wang C, Hang H. Multi-region sequencing with spatial information enables accurate heterogeneity estimation and risk stratification in liver cancer. Genome Med 2022;14:142. [Crossref] [PubMed]