Deep learning methods for diagnosis of graves’ ophthalmopathy using magnetic resonance imaging
Introduction
Graves’ ophthalmopathy (GO) is an autoimmune disease that commonly appears in 30% of patients with Graves’ disease, which negatively affects the patient’s quality of life (1-4). The diagnosis of GO is mainly based on ophthalmic clinical features such as eyelid retraction and exophthalmos (5,6). However, these symptoms are relatively subjective, and orbital imaging is not often performed in clinical practice unless serious symptoms appear such as double vision (7). Ambiguous ophthalmic characteristics in patients could occasionally lead to an unclear diagnosis. The mechanism of GO may be lymphocyte infiltration and activation inside orbital tissue caused by a cross-reaction of thyroid-stimulating hormone receptor antibodies and antigens (8). With the development of GO, extraocular muscles become infiltrated by inflammatory cells. It is likely to find changes in the muscles on medical imaging. Therefore, it is necessary that objective modalities such as computed tomography (CT) and magnetic resonance imaging (MRI) are applied to improve the accuracy of diagnosis and evaluation of treatment effectiveness.
Compared to CT, the advantages of MRI such as the absence of radiation and the excellent soft tissue resolution, are notable. MRI has been widely applied to the diagnosis of GO, treatment effect evaluation, and follow-up (9). Exophthalmos in the transverse section and extraocular muscle involvement in the coronal section are commonly regarded as diagnostic criteria through radiographic observation. However, the measurement of exophthalmos is relatively complex and prone to error (an accurate measurement of the vertical distance from the front of the eye to the inter-zygomatic line is necessary) (10,11). Extraocular muscle involvement with minor modifications is difficult to differentiate, especially in the early stages of the disease. The inferior rectus muscle is often regarded as the most typically enlarged extraocular muscle in GO (12). However, there are few comprehensive assessment methods of GO that incorporate information from all extraocular muscles. Therefore, to interpret MRI findings and produce objective findings for determining the diagnosis and prognosis of patients with GO, a stable and synthesized method is required.
Artificial intelligence (AI)-based image diagnosis has improved significantly in the medical field during the past few years (13,14), with diagnostic accuracy on par with or exceeding that of human experts for several diseases. The application of AI systems in MRI sequences improves radiologists’ performance in the task of differentiating lesions, especially in the fields of breast and brain (15,16). Although 2 researchers (7,17) previously used deep learning (DL) methods to diagnose GO, they only used CT scans as input, and did not compare their results with those of traditional measurement methods. Besides, it is important to consider that measurements and result reporting are still not standardized, even though several studies have been conducted for GO assessment utilizing various techniques and methodologies (18). In this study, we adopted DL structures that could be applied to the diagnosis of GO and compared with traditional measurement and judgment of radiologists. We present this article in accordance with the TRIPOD reporting checklist (available at https://qims.amegroups.com/article/view/10.21037/qims-24-80/rc).
Methods
Patients
The study was conducted in accordance with the Declaration of Helsinki (as revised in 2013). The study was approved by the Institutional Review Board of The Fifth Affiliated Hospital of Sun Yat-sen University (No. K152-1), and the requirement for individual consent for this retrospective analysis was waived. The dataset was collected from The Fifth Affiliated Hospital of Sun Yat-sen University in May 2023 and 223 patients who were diagnosed with GO and 177 patients who were normal as the control group in our hospital from September 2015 to September 2021 were included. The GO patients were identified using Bartley’s criteria (5). Figure 1 shows the inclusion and exclusion criteria. Patients who were diagnosed with GO and who underwent T1-weighted MRI scans were included in this study. The time from outpatient reception to the MRI examination is less than 3 days. Patients were excluded if: (I) they were younger than 18 years old, (II) had malignant tumors, (III) had other eye diseases, (IV) their MRI quality was inadequate for measurements, or (V) they had a history of treatment for GO. MRI examinations were performed with a 3.0-Tesla Siemens Verio MR Scanner (Siemens AG, Erlangen, Germany). Normal people in the control group were enrolled if they went to the hospital for an exophthalmos assessment by MRI and were confirmed to have no eye disease. They were excluded if: (I) they were younger than 18 years old, (II) had malignant tumors, (III) MRI quality was inadequate for measurements, or (IV) they had abnormal thyroid hormone or thyroid stimulating hormone levels. Finally, all cases were randomly separated into a training group and a validation group at a ratio of 7:3.
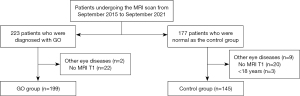
Extraocular muscle measurement
Extraocular muscle involvement including the superior rectus muscle, inferior rectus muscle, medial rectus muscle, and lateral rectus muscle was observed and measured on T1-weighted MRI scans (Figure 2). The axial scans were taken at an angle of 10–15° to the orbitomeatal line. We chose the coronal plane posterior to the eyeball and 5 mm posterior to the eyeball to calculate the area of 4 extraocular muscles. The inferior and superior oblique muscles were not included because of their oblique path to the coronal plane. Areas of 4 extraocular muscles were calculated by 1 radiology resident and reviewed by a radiologist with more than 10 years of experience. Based on the coronal cross-sectional area of the extraocular muscles obtained by conventional measurement methods mentioned above, we constructed logistic regression models to predict GO.
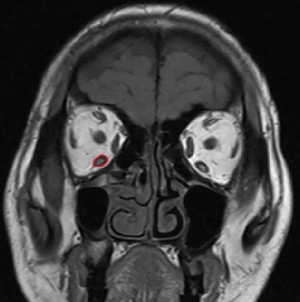
DL models development
To propose DL models that can diagnose GO, we applied a traditional convolutional neural networks (CNNs) model named ResNet101 and a transformer-based architecture named Swin Transformer respectively (19,20). Figure 3 illustrates the construction of pretrained 2 models. In data preprocessing, we placed a square shape of a bounding box manually on the orbit in the coronal plane, ensuring that all extraocular muscles were involved within the box. Coronal planes were the same as the ones chosen to measure muscles. These bounding boxes were resampled to 224×224 pixels as the input of 2 DL models. Transfer learning was applied in 2 models and the swin-tiny-patch4-window7-224 weights were used in Swin Transformer for subsequent training. Swin Transformer’s source code is accessible at https://github.com/microsoft/Swin-Transformer. Python 3.11.0 and PyTorch 2.0 (https://pytorch.org) were used to implement the models. They were trained on a workstation equipped with a single RTX 4090 GPU. In order to elucidate the rationale behind the predictions generated by the network model and the contributions of extraocular muscles towards the diagnosis of GO, a gradient-weighted class activation mapping (Grad-CAM) analysis was utilized.
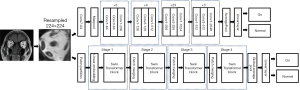
Radiologists’ evaluation
We requested 2 radiologists (average of 5 years’ experience) to conduct an independent diagnosis of GO only relying on T1-weighted MRI scans without clinical information. If the results were inconsistent, a specialist radiologist with more than 15 years’ experience made the final decision. The diagnostic performance was compared to the DL models using the area under the curve (AUC).
Statistical analysis
Statistical analysis was conducted with SPSS 26.0 (IBM Corp., Armonk, NY, USA). The Shapiro-Wilk test was used to determine the normality and homogeneity of variance in the continuous variables, then the independent t-test or Mann-Whitney U test was utilized. Areas of muscles were calculated by 3D-Slicer (version 5.2.0; https://www.slicer.org/). The chi-square test was used to analyze differences in categorical variables in clinical information. The threshold at the greatest Youden index was used to calculate sensitivity, specificity, positivity, and accuracy. The Cohen Kappa coefficient was used to determine consistency between 2 radiologists. AUCs for different models were compared by the DeLong test. We calculated the 95% confidence interval (CI) for this performance by bootstrapping (1,000 bootstrap intervals). A P value <0.05 was considered significant.
Results
The patient characteristics are listed in Table 1. Totals of 199 consecutive cases of GO and 145 cases of normal controls were included in this study. The average age of the GO group was older than that of normal controls. There is no significant difference in gender ratio between the 2 groups. Table 2 illustrates areas of superior, inferior, medial, and lateral rectus muscles on 2 MRI coronal planes in 2 groups. Areas of superior, inferior, medial, and lateral rectus muscles were 0.407±0.187, 0.322±0.147, 0.286±0.088, and 0.305±0.123 mm2, respectively, on the coronal plane posterior to the eyeball. Areas of superior, inferior, medial, and lateral rectus muscles were 0.419±0.182, 0.432±0.167, 0.350±0.103, and 0.345±0.160 mm2, respectively, on the coronal plane 5 mm posterior to the eyeball. Each muscle in the GO group was significantly bigger than the same muscle in the normal group (the corresponding P-value is listed in Table 2).
Table 1
Characteristics | GO | Normal | P value |
---|---|---|---|
Number of participants | 199 | 145 | |
Age (years), mean ± SD | 45.07±13.22 | 41.80±12.97 | 0.017 |
Gender (male/female) | 86/113 | 63/82 | 0.966 |
GO, Graves’ ophthalmopathy; SD, standard deviation.
Table 2
Groups | 0 mm | 5 mm | |||||
---|---|---|---|---|---|---|---|
Normal | GO | P value | Normal | GO | P value | ||
Areas of superior rectus muscles | 0.266±0.062 | 0.407±0.187 | <0.001 | 0.271±0.060 | 0.419±0.182 | <0.001 | |
Areas of inferior rectus muscles | 0.250±0.069 | 0.322±0.147 | <0.001 | 0.337±0.065 | 0.432±0.167 | <0.001 | |
Areas of medial rectus muscles | 0.264±0.047 | 0.286±0.088 | 0.008 | 0.279±0.054 | 0.350±0.103 | <0.001 | |
Areas of lateral rectus muscles | 0.259±0.058 | 0.305±0.123 | <0.001 | 0.300±0.063 | 0.345±0.160 | 0.009 |
Data are presented as means ± standard deviations. GO, Graves’ ophthalmopathy.
Tables 3,4 show the performance of models in the training group (139 GO patients and 101 normal controls) and validation group (60 graves patients and 44 normal controls). In the training group, the AUCs of logistic regression models by superior, inferior, medial, lateral rectus, and all muscles were 0.891 (95% CI: 0.848–0.930), 0.746 (95% CI: 0.684–0.809), 0.771 (95% CI: 0.710–0.828), 0.615 (95% CI: 0.547–0.686), and 0.906 (95% CI: 0.864–0.943). The Swin Transformer achieved an AUC of 0.999 (95% CI: 0.999–1), and its accuracy, sensitivity, and specificity were 0.986 (95% CI: 0.979–0.994), 0.987 (95% CI: 0.978–0.996), and 0.985 (95% CI: 0.972–0.995), respectively, and ResNet101 yielded 1 (95% CI of accuracy, sensitivity, and specificity: 1–1; 95% CI of AUC: 0.999–1) for each index. In the validation group, the AUCs of logistic regression models by superior, inferior, medial, and lateral rectus muscles and all muscles were 0.897 (95% CI: 0.833–0.949), 0.705 (95% CI: 0.598–0.804), 0.799 (95% CI: 0.712–0.876), 0.681 (95% CI: 0.567–0.776), and 0.905 (95% CI: 0.843–0.955), respectively. Swin Transformer achieved an AUC of 0.936 (95% CI: 0.912–0.957), and its accuracy, sensitivity, and specificity were 0.851 (95% CI: 0.815–0.885), 0.817 (95% CI: 0.767–0.863), and 0.898 (95% CI: 0.851–0.938), respectively. ResNet101 yielded an AUC of 0.986 (95% CI: 0.977–0.994), and its accuracy, sensitivity, and specificity were 0.933 (95% CI: 0.906–0.957), 0.979 (95% CI: 0.960–0.996), and 0.869 (95% CI: 0.819–0.923), respectively. Figure 4 shows the ROC curves in the validation group. It was revealed that 2 DL models achieved higher AUC than models of muscles in both groups. AUC was significantly different between ResNet101 and the model of all muscles (Delong test: P<0.001), but not between Swin Transformer and the model of all muscles (Delong test: P=0.291). Figure 5 illustrates the Grad-GAM derived from 2 DL models. Extraocular muscles were highlighted as important regions.
Table 3
Models | Accuracy (95% CI) | Sensitivity (95% CI) | Specificity (95% CI) | AUC (95% CI) |
---|---|---|---|---|
Superior rectus muscles | 0.813 (0.763–0.858) | 0.842 (0.780–0.901) | 0.772 (0.689–0.857) | 0.891 (0.848–0.930) |
Inferior rectus muscles | 0.654 (0.596–0.713) | 0.813 (0.752–0.874) | 0.436 (0.337–0.530) | 0.746 (0.684,0.809) |
Medial rectus muscles | 0.683 (0.625–0.746) | 0.892 (0.841–0.944) | 0.396 (0.305–0.494) | 0.771 (0.710–0.828) |
Lateral rectus muscles | 0.563 (0.500–0.625) | 0.957 (0.921–0.986) | 0.020 (0.000–0.051) | 0.615 (0.547–0.686) |
All eye muscles | 0.838 (0.788–0.883) | 0.871 (0.810–0.920) | 0.792 (0.708–0.867) | 0.906 (0.864–0.943) |
ResNet101 | 1 (1–1) | 1 (1–1) | 1 (1–1) | 1 (0.999–1) |
Swin Transformer | 0.986 (0.979–0.994) | 0.987 (0.978–0.996) | 0.985 (0.972–0.995) | 0.999 (0.999–1.000) |
DL, deep learning; AUC, area under the curve; CI, confidence interval.
Table 4
Models | Accuracy (95% CI) | Sensitivity (95% CI) | Specificity (95% CI) | AUC (95% CI) |
---|---|---|---|---|
Superior rectus muscles | 0.779 (0.644–0.889) | 0.817 (0.714–0.908) | 0.727 (0.587–0.854) | 0.897 (0.833–0.949) |
Inferior rectus muscles | 0.577 (0.481–0.663) | 0.767 (0.656–0.865) | 0.318 (0.184–0.459) | 0.705 (0.598–0.804) |
Medial rectus muscles | 0.683 (0.587–0.769) | 0.867 (0.779–0.947) | 0.432 (0.286–0.588) | 0.799 (0.712–0.876) |
Lateral rectus muscles | 0.548 (0.452–0.644) | 0.900 (0.823–0.968) | 0.069 (0.0–0.147) | 0.681 (0.567–0.776) |
All eye muscles | 0.817 (0.731–0.885) | 0.850 (0.750–0.934) | 0.773 (0.644–0.889) | 0.905 (0.843–0.955) |
ResNet101 | 0.933 (0.906–0.957) | 0.979 (0.960–0.996) | 0.869 (0.819–0.923) | 0.986 (0.977–0.994) |
Swin Transformer | 0.851 (0.815–0.885) | 0.817 (0.767–0.863) | 0.898 (0.851–0.938) | 0.936 (0.912–0.957) |
DL, deep learning; AUC, area under the curve; CI, confidence interval.
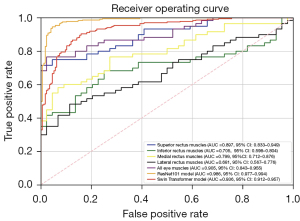
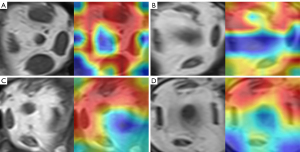
In terms of diagnostic performance for radiologists, the Cohen kappa coefficient for the 2 radiologists was 0.884, and 2 DL models were better than the diagnostic performance of radiologists (AUC: 0.986 and 0.936 vs. 0.818, Delong test: P<0.001), with accuracy, sensitivity, and specificity of 0.808 (95% CI: 0.769–0.844), 0.75 (95% CI: 0.693–0.803), and 0.886 (95% CI: 0.832–0.927), respectively. ResNet101 yielded the best performance; the results are shown in Table 5.
Table 5
Delong test | AUC | P value |
---|---|---|
ResNet101 vs. Swin Transformer | 0.986 vs. 0.936 | <0.001 |
ResNet101 vs. all eye muscles | 0.986 vs. 0.905 | <0.001 |
ResNet101 vs. radiologists | 0.986 vs. 0.818 | <0.001 |
Swin Transformer vs. all eye muscles | 0.936 vs. 0.905 | 0.291 |
Swin Transformer vs. radiologists | 0.936 vs. 0.818 | <0.001 |
AUC, area under the curve.
Discussion
This is a novel article to apply DL methods to MRI diagnosis of GO. We evaluated whether 2 DL models could adequately distinguish individuals with GO from normal controls compared with traditional measurements and decisions of radiologists. The ResNet101 model’s performance showed a higher AUC 0.986 (95% CI: 0.979–0.994) than traditional measurements 0.905 (95% CI: 0.843–0.955) and radiologists 0.818 (95% CI: 0.781–0.854). Since DL models are very quick to judge GO, our findings imply that using AI to aid with diagnosis may minimize the error rate and relieve doctors’ burden in actual clinical use. Estcourt et al. (21) revealed that the period elapsed between the onset of symptoms and the diagnosis of GO was more than 12 months in 26% of respondents and just 25% of patients received recommendations to a specialized GO clinic. The diagnosis and referral were both delayed. In GO treatment, early detection is crucial to preventing patients’ irreversible damage (proptosis, sight impairment) (22). However, Some GO cases do not have thyroid dysfunction and clinical symptoms are late onset or absent. If the model takes patient MRIs of early GO as input and then is trained and validated, it would play an important role in early detection of GO.
MRIs can be used to evaluate the extraocular muscle and other eye components. Similar to CT examinations, extraocular muscles can be examined primarily using volumetry, diameter, thickness, diffusion, and signal intensity ratio. Although the volumetry, diameter, and thickness results were fairly comparable to the CT results, the utilization of MRI allows the investigator to approach the disease using different methodologies, such as diffusion and signal intensity ratio. Diffusion tensor imaging investigations can detect microstructural changes in extraocular muscles and suggest disease activity using mean diffusivity measures for medial extraocular muscles (23). Diffusion-weighted imaging of the extraocular muscles is employed for diagnosis, with the apparent diffusion coefficient as a metric (24). Chen et al. (25) found that the medial rectus muscle is the best place to obtain the metric, and Kilicarslan et al. (26) discovered that the metric also correlates with ophthalmologic tests, making the method a promising option. The diffusion-weighted imaging sequence can be used in a routine examination, providing more information about the disease. Indicating disease activity, the signal intensity ratio of the T1 and T2 scans was also encouraging. Since T1 and T2 pictures are commonly recorded sequentially, 2 studies (27,28) have provided light on the methodology used to signal disease activity in extraocular muscles, which aids in determining the optimal treatment approach.
Proptosis of GO is not measured uniformly, which resulted in a very slight the difference in length between the 2 groups, especially in the mild GO. Therefore, we chose the coronal plane as the level of observation and input of DL. It is also the main reference for radiologists to diagnose GO. Generally, there is no numerical type of standard for eye muscle enlargement, which leads radiologists to rely primarily on empirical judgment. A diagnosis of GO can be suspected if 1 or 2 thickened eye muscles. However, in the early stages of GO muscle thickening is not apparent and there is no comprehensive model for evaluating muscle enlargement. In our study, we performed logistic regression to diagnose GO, which includes all muscles that can be used as a reference. The performance of models achieved AUCs of 0.906 (95% CI: 0.864–0.943) and 0.905 (95% CI: 0.843–0.955) in the training group and validation group, respectively. This indicated that the model has good generalization ability to predict unseen data. DL models have made significant advancements in image recognition tasks in recent years. They provide reliable medical picture analysis and interpretation, whereas human vision can be subjective and sensitive to inter-observer variation. Furthermore, DL models can assess medical pictures in-depth, taking into account all of the pixels, areas, and patterns in the image. There are many features that the human eye cannot analyze or miss easily. In this study, because of the good contrast on T1-weighted images by extraocular muscles with the surrounding tissue, DL models were suitable for our tasks.
Previous studies have demonstrated that based on CT images, DL models can be used for GO diagnosis and severity evaluation (7,17). However, both of these studies lacked a direct comparison of DL models with traditional measurements. Hanai et al. (7) chose the maximum diameter to assess the extraocular muscles; by this, some useful information would be missed, and muscle cross-section is usually irregular. In our study, measurements and model inputs were in the same coronal planes, and radiologists also diagnosed only from T1-weighted images. Furthermore, CT may not be indicated for evaluation of treatment efficacy and follow-up because of radiation, especially for high severity of GO. Kvetny et al. (9) discovered that the thickness of muscles on T1-weighted sequences was substantially related to the patient’s clinical activity score (CAS) and active illness duration. Tortora et al. (29) investigated the signal intensity of muscles on short tau inversion recovery (STIR) and T1-weighted post-contrast sequences associated with CAS. DL methods have the potential to evaluate activity and severity in GO based on MRI multiple sequences, and this is important for the subsequent selection of treatment options. Further research would focus on multisequence MRI to evaluate activity assessment of GO and the effectiveness of treatment relying on DL methods.
CNNs have been widely employed in medical imaging disciplines such as detection, classification, and semantic segmentation (30). ResNet is an advanced CNN which has excellent model performance in image classification and recognition. Although transformer-based models may be more interpretable than typical CNN and have gradually been applied in the computer vision field in recent years (20), the advantages of Swin Transformer have not been reflected on specific tasks and the transformer model usually requires large samples. In our study, ResNet101 outperformed Swin Transformer in terms of AUC (0.986 vs. 0.936). This variability in model performance is largely influenced by datasets and sizes. Another possible reason is our study mainly focused on changes in muscle morphology, a relatively uncomplicated task. Gai et al. (31) also highlighted the superior effectiveness of CNNs. Appropriate networks should be selected based on different types of medical tasks.
There are some limitations to our study. First, this study involved a single center, the sample size was relatively small; multi-institution datasets and independent external validation groups would be considered to confirm the generalization of the 2 DL models. Second, because not every patient underwent the coronal MRI T2-weighted scans and MRI coronal contrast-enhanced T1-weighted scans, models constructed by T2-weighted or other sequences and comparison of different sequences were lacking. T2 MRI sequence had the advantage of the hyperintense signal of edema. Ollitrault et al. (32) showed that Dixon-T2-weighted scans had greater sensitivity and specificity, as well as fewer artifacts, than a standard technique when assessing GO. Third, the measurement of extraocular muscle involvement and input of 2 DL models depended on 2-dimensional MRI images. More information was provided if axial and sagittal planes were considered as references and the model’s efficacy may be improved as parameters increase from different views. Furthermore, finding and cropping is time-consuming; in further study, we would utilize AI segmentation to automate cutting to achieve fully automated diagnosis, and if the outcomes are good, we would conduct a prospective study to support the actual clinical value.
In conclusion, we have proposed DL models based on MRI to diagnose GO relying on T1-weighted MRI in terms of diagnostic efficiency. In regular clinical use, the models may provide a useful diagnostic reference for diagnosing GO.
Acknowledgments
Funding: This work was supported by
Footnote
Reporting Checklist: The authors have completed the TRIPOD reporting checklist. Available at https://qims.amegroups.com/article/view/10.21037/qims-24-80/rc
Conflicts of Interest: All authors have completed the ICMJE uniform disclosure form (available at https://qims.amegroups.com/article/view/10.21037/qims-24-80/coif). The authors have no conflicts of interest to declare.
Ethical Statement: The authors are accountable for all aspects of the work in ensuring that questions related to the accuracy or integrity of any part of the work are appropriately investigated and resolved. The study was conducted in accordance with the Declaration of Helsinki (as revised in 2013). The study was approved by the Institutional Review Board of The Fifth Affiliated Hospital of Sun Yat-sen University (No. K152-1), and the requirement for individual consent for this retrospective analysis was waived.
Open Access Statement: This is an Open Access article distributed in accordance with the Creative Commons Attribution-NonCommercial-NoDerivs 4.0 International License (CC BY-NC-ND 4.0), which permits the non-commercial replication and distribution of the article with the strict proviso that no changes or edits are made and the original work is properly cited (including links to both the formal publication through the relevant DOI and the license). See: https://creativecommons.org/licenses/by-nc-nd/4.0/.
References
- Blandford AD, Zhang D, Chundury RV, Perry JD. Dysthyroid optic neuropathy: update on pathogenesis, diagnosis, and management. Expert Rev Ophthalmol 2017;12:111-21. [Crossref] [PubMed]
- Gillespie EF, Smith TJ, Douglas RS. Thyroid eye disease: towards an evidence base for treatment in the 21st century. Curr Neurol Neurosci Rep 2012;12:318-24. [Crossref] [PubMed]
- Topilow NJ, Tran AQ, Koo EB, Alabiad CR. Etiologies of Proptosis: A review. Intern Med Rev (Wash D C) 2020.
- Eckstein AK, Lösch C, Glowacka D, Schott M, Mann K, Esser J, Morgenthaler NG. Euthyroid and primarily hypothyroid patients develop milder and significantly more asymmetrical Graves ophthalmopathy. Br J Ophthalmol 2009;93:1052-6. [Crossref] [PubMed]
- Bartley GB, Gorman CA. Diagnostic criteria for Graves' ophthalmopathy. Am J Ophthalmol 1995;119:792-5. [Crossref] [PubMed]
- Barrio-Barrio J, Sabater AL, Bonet-Farriol E, Velázquez-Villoria Á, Galofré JC. Graves' Ophthalmopathy: VISA versus EUGOGO Classification, Assessment, and Management. J Ophthalmol 2015;2015:249125. [Crossref] [PubMed]
- Hanai K, Tabuchi H, Nagasato D, Tanabe M, Masumoto H, Miya S, Nishio N, Nakamura H, Hashimoto M. Automated detection of enlarged extraocular muscle in Graves' ophthalmopathy with computed tomography and deep neural network. Sci Rep 2022;12:16036. [Crossref] [PubMed]
- Cockerham KP, Kennerdell JS. Does radiotherapy have a role in the management of thyroid orbitopathy? View 1. Br J Ophthalmol 2002;86:102-4.
- Kvetny J, Puhakka KB, Røhl L. Magnetic resonance imaging determination of extraocular eye muscle volume in patients with thyroid-associated ophthalmopathy and proptosis. Acta Ophthalmol Scand 2006;84:419-23. [Crossref] [PubMed]
- Schmidt P, Kempin R, Langner S, Beule A, Kindler S, Koppe T, Völzke H, Ittermann T, Jürgens C, Tost F. Association of anthropometric markers with globe position: A population-based MRI study. PLoS One 2019;14:e0211817. [Crossref] [PubMed]
- Ma Z, Ozaki H, Ishikawa Y, Jingu K. Improvement of the MRI and clinical features of Asian Graves' ophthalmopathy by radiation therapy with steroids. Jpn J Radiol 2019;37:612-8. [Crossref] [PubMed]
- Crisp M, Starkey KJ, Lane C, Ham J, Ludgate M. Adipogenesis in thyroid eye disease. Invest Ophthalmol Vis Sci 2000;41:3249-55.
- Rajpurkar P, Chen E, Banerjee O, Topol EJ. AI in health and medicine. Nat Med 2022;28:31-8. [Crossref] [PubMed]
- Chen PC, Mermel CH, Liu Y. Evaluation of artificial intelligence on a reference standard based on subjective interpretation. Lancet Digit Health 2021;3:e693-5. [Crossref] [PubMed]
- Jiang Y, Edwards AV, Newstead GM. Artificial Intelligence Applied to Breast MRI for Improved Diagnosis. Radiology 2021;298:38-46. [Crossref] [PubMed]
- Krishnapriya S, Karuna Y. Pre-trained deep learning models for brain MRI image classification. Front Hum Neurosci 2023;17:1150120. [Crossref] [PubMed]
- Lee J, Seo W, Park J, Lim WS, Oh JY, Moon NJ, Lee JK. Neural network-based method for diagnosis and severity assessment of Graves' orbitopathy using orbital computed tomography. Sci Rep 2022;12:12071. [Crossref] [PubMed]
- Luccas R, Riguetto CM, Alves M, Zantut-Wittmann DE, Reis F. Computed tomography and magnetic resonance imaging approaches to Graves' ophthalmopathy: a narrative review. Front Endocrinol (Lausanne) 2024;14:1277961. [Crossref] [PubMed]
- He K, Zhang X, Ren S, Sun J. Deep residual learning for image recognition. Proceedings of the IEEE Conference on Computer Vision and Pattern Recognition (CVPR), 2016:770-8.
- Liu Z, Lin Y, Cao Y, Hu H, Wei Y, Zhang Z, Lin S, Guo B. Swin Transformer: Hierarchical Vision Transformer using Shifted Windows. Proceedings of the IEEE/CVF International Conference on Computer Vision (ICCV), 2021:10012-22.
- Estcourt S, Hickey J, Perros P, Dayan C, Vaidya B. The patient experience of services for thyroid eye disease in the United Kingdom: results of a nationwide survey. Eur J Endocrinol 2009;161:483-7. [Crossref] [PubMed]
- Tortora F, Prudente M, Cirillo M, Elefante A, Belfiore MP, Romano F, Cappabianca S, Carella C, Cirillo S. Diagnostic accuracy of short-time inversion recovery sequence in Graves' Ophthalmopathy before and after prednisone treatment. Neuroradiology 2014;56:353-61. [Crossref] [PubMed]
- Chen L, Hu H, Chen W, Wu Q, Zhou J, Chen HH, Xu XQ, Shi HB, Wu FY. Usefulness of readout-segmented EPI-based diffusion tensor imaging of lacrimal gland for detection and disease staging in thyroid-associated ophthalmopathy. BMC Ophthalmol 2021;21:281. [Crossref] [PubMed]
- Abdel Razek AA, El-Hadidy M, Moawad ME, El-Metwaly N, El-Said AA. Performance of apparent diffusion coefficient of medial and lateral rectus muscles in Graves' orbitopathy. Neuroradiol J 2017;30:230-4. [Crossref] [PubMed]
- Chen HH, Hu H, Chen W, Cui D, Xu XQ, Wu FY, Yang T. Thyroid-Associated Orbitopathy: Evaluating Microstructural Changes of Extraocular Muscles and Optic Nerves Using Readout-Segmented Echo-Planar Imaging-Based Diffusion Tensor Imaging. Korean J Radiol 2020;21:332-40. [Crossref] [PubMed]
- Kilicarslan R, Alkan A, Ilhan MM, Yetis H, Aralasmak A, Tasan E. Graves' ophthalmopathy: the role of diffusion-weighted imaging in detecting involvement of extraocular muscles in early period of disease. Br J Radiol 2015;88:20140677. [Crossref] [PubMed]
- Kirsch EC, Kaim AH, De Oliveira MG, von Arx G. Correlation of signal intensity ratio on orbital MRI-TIRM and clinical activity score as a possible predictor of therapy response in Graves' orbitopathy--a pilot study at 1.5 T. Neuroradiology 2010;52:91-7. [Crossref] [PubMed]
- Politi LS, Godi C, Cammarata G, Ambrosi A, Iadanza A, Lanzi R, Falini A, Bianchi Marzoli S. Magnetic resonance imaging with diffusion-weighted imaging in the evaluation of thyroid-associated orbitopathy: getting below the tip of the iceberg. Eur Radiol 2014;24:1118-26. [Crossref] [PubMed]
- Tortora F, Cirillo M, Ferrara M, Belfiore MP, Carella C, Caranci F, Cirillo S. Disease activity in Graves' ophthalmopathy: diagnosis with orbital MR imaging and correlation with clinical score. Neuroradiol J 2013;26:555-64. [Crossref] [PubMed]
- Kaul V, Enslin S, Gross SA. History of artificial intelligence in medicine. Gastrointest Endosc 2020;92:807-12. [Crossref] [PubMed]
- Gai L, Xing M, Chen W, Yi Z, Xu Q. Comparing CNN-based and transformer-based models for identifying lung cancer: which is more effective? Multimedia Tools and Applications 2023; [Crossref]
- Ollitrault A, Charbonneau F, Herdan ML, Bergès O, Zuber K, Giovansili L, Launay P, Savatovsky J, Lecler A. Dixon-T2WI magnetic resonance imaging at 3 tesla outperforms conventional imaging for thyroid eye disease. Eur Radiol 2021;31:5198-205. [Crossref] [PubMed]