Multiparametric magnetic resonance imaging (MRI)-based radiomics model explained by the Shapley Additive exPlanations (SHAP) method for predicting complete response to neoadjuvant chemoradiotherapy in locally advanced rectal cancer: a multicenter retrospective study
Introduction
Colorectal cancer (CRC) ranks among the most prevalent cancers worldwide, accounting for 10.0% of all cancer cases and 9.4% of cancer-related deaths globally (1). It was projected that in 2023, there would be approximately 153,020 new cases and 52,550 deaths from CRC in the United States (2). Locally advanced rectal cancers (LARCs) represent nearly 30% of diagnosed cases (2). Neoadjuvant chemoradiotherapy (nCRT) followed by radical surgery including total mesorectal excision (TME) is an integral part of the standard treatment for LARCs, significantly improving patient prognosis (3). Moreover, for patients who achieve a pathological complete response (pCR) following neoadjuvant therapy, a “watch-and-wait” nonoperative approach can reduce surgery-related morbidity and functional complications (4,5). However, current assessment of clinical complete response (cCR) primarily relies on post-treatment magnetic resonance imaging (MRI), which classifies patients after treatment and leaves out some patients who could achieve cCR with more appropriate preoperative treatment. Therefore, it is crucial to predict the response to nCRT prior to treatment in order to administer more effective treatments and achieve cCR. Although cCR indicates a favorable response to nCRT, it does not necessarily guarantee a true pCR, as local regrowth rates within 2 years of follow-up range from 7% to 33% (6). Therefore, differentiating pCR from cCR is of paramount importance.
Clinicians currently evaluate response to nCRT based on pathological tumor regression grade (TRG) and downstaging after treatment, but there are limited clinical features available before treatment that can predict tumor response (7). Pathological tissue assessment using sequencing and organoid technology has the potential to identify good response and bad response patients, but its clinical application is hindered by invasiveness and additional costs (8-10). Therefore, there is an urgent need for an accessible, cost-effective, non-invasive, and accurate method to predict the response to nCRT and guide the treatment of LARC patients. Radiomics, which is based on the analysis of medical images, represents a promising tool for response prediction. Numerous studies have demonstrated the efficacy of radiomics based on pre-treatment medical images in predicting the response to chemo(radio)therapy in various cancers (11-15). Although some studies involving rectal cancer have shown promising results, their clinical applications have been limited by small sample sizes, single-center settings, unclear internal mechanisms, or unexplained features of the developed models (16-19).
In this study, we aimed to develop and validate multicenter prediction models that incorporate pre-treatment MRI radiomic features and clinical characteristics for predicting cCR and further identifying pCR from cCR. Additionally, we employed the SHapley Additive exPlanations (SHAP) method to interpret our models, which can elucidate the importance of features in the prediction model and their contributions to individual predictions (20). We present this article in accordance with the TRIPOD reporting checklist (available at https://qims.amegroups.com/article/view/10.21037/qims-24-7/rc).
Methods
Patient cohort
This retrospective study was conducted at 3 centers affiliated to the First Affiliated Hospital, Zhejiang University School of Medicine (Qingchun Center, Yuhang Center, and Zhijiang Center) to investigate a cohort of patients with LARC. The study period ranged from January 2014 to November 2022. The inclusion criteria for the study were as follows: (I) patients with confirmed LARCs based on pre-nCRT MRI scans, with clinical stage T3 or higher, positive nodal status, or both; (II) patients who underwent the standard treatment protocol of long-course radiation therapy (RT), which comprised 50.4 Gy of radiation delivered in 28 fractions (45 Gy in 25 fractions to the pelvic area and 5.4 Gy in 3 fractions to the tumor bed), along with concurrent oral chemotherapy using capecitabine at 825 mg/m2 twice daily; (III) the interval between MRI examination and the initiation of nCRT was less than 2 weeks; (IV) surgery was performed approximately 6 weeks after completing nCRT, and all patients underwent postoperative pathologic examination; (V) pre-nCRT MRI data, including T1-weighted imaging (T1WI) and T2-weighted imaging (T2WI), were available for all included patients. Patients were excluded if they met any of the following criteria: (I) history of previous recurrent rectal cancer or metastatic rectal cancer; (II) incomplete nCRT, absence of surgery or surgery conducted in other hospitals; (III) detection of mucinous adenocarcinoma during postoperative pathologic examination; (IV) presence of poor-quality images or incomplete imaging data. After applying these criteria, a total of 285 patients were included in the study. Among them, 241 patients from the Qingchun Center were designated as the training set, whereas 44 patients from the other centers formed the external validation set. The retrospective research was approved by the Ethics Committee of the First Affiliated Hospital, Zhejiang University School of Medicine (No. IIT2020-1305) and was conducted in accordance with the Declaration of Helsinki (as revised in 2013). The requirement for informed consent was waived by the Ethics Committee because no identifying detail of the participants was included in this research.
Clinical characteristics
The clinical characteristics of the included patients encompassed various factors, such as age, sex, pre-treatment serum tumor marker levels [carcinoembryonic antigen (CEA), carbohydrate antigen 199 (CA199)], systemic immune inflammation index (SII, calculated as the product of platelet count and neutrophil-to-lymphocyte ratio), as well as MRI-predicted tumor invasion parameters, including tumor-node-metastasis (TNM) stage, extramural vascular invasion (EMVI), and circumferential resection margin (CRM). Additionally, the tumor location was also considered as part of the clinical profile of the patients.
Assessment of response
Based on the post-treatment MRI and endoscopic results, cCR was defined as the presence of scars and telangiectasias and the absence of any irregularity, mass, ulceration, or stenosis in endoscopy, and the magnetic resonance tumor regression grade (mrTRG) of 0. The presence of lesion in endoscopic or mrTRG of 1–3 were regarded as non-cCR. The pathological tumor regression was categorized into 4 grades according to the 8th edition of American Joint Committee on Cancer (AJCC) Cancer Staging Manual (21). Grade 0 was considered as pCR, indicating the absence of viable tumor cells. Grades 1–3 were collectively considered as non-pathological complete response (non-pCR), indicating varying degrees of residual tumor cells.
MRI protocol
MRI was performed with a 1.5T scanner at Qingchun center (GE Signa Excite 1.5T; GE Healthcare, Chicago, IL, USA), and 3.0T scanners at Yuhang center (GE Architect 3.0T) and Zhijiang center (GE Architect 3.0T). The imaging protocols included T1-weighted (T1W) and T2-weighted (T2W) turbo spin-echo sequence in the axial planes. Details of MRI characteristics at the 3 centers are shown in Table S1.
Tumor segmentation
The regions of interest (ROIs) encompassing the entire area of rectal cancer were manually delineated in axial T1W and T2W MRIs for each patient by 2 experienced radiologists with more than 5 years of expertise in rectal cancer radiotherapy. This delineation process was performed independently using MITK [version 2021.10; German Cancer research Center (DKFZ), Heidelberg, Germany]. To ensure objectivity, the radiologists remained unaware of the pathological reports during the delineation. The ROIs were meticulously defined, taking care to exclude the normal rectal wall and mucosal edema from the analysis. A visual representation of the ROI delineation can be seen in Figure 1.
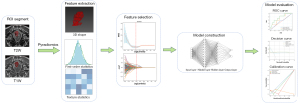
In cases where there were discrepancies in the manual tumor segmentations between the 2 radiologists, a senior radiologist with over 10 years of experience in rectal cancer radiotherapy conducted a final validation. This senior radiologist also remained unaware of the pathological reports to maintain unbiased assessment and ensure the accuracy and consistency of the ROIs.
Radiomic feature extraction
Radiomic features were extracted respectively from original and filtered images, including wavelet, Laplacian of Gaussian (sigma: 2.0, 3.0, 4.0, 5.0), square, squareroot, logarithm, and exponential by PyRadiomics package (version 3.0.1) (22). In order to reduce the disparity among different MR system vendors and acquisition protocols, normalization of the MRI signal intensities for images, discretization with fixed bin width values of 5, and voxel size resampling by 3×3×3 mm3 were performed before feature extraction. Finally, 1,731 features, respectively extracted from each T1WI and T2WI (shown in Table 1), were as follows: (I) shape and size features: n=14; (II) first-order statistics: n=18; (III) texture features including grey-level co-occurrence matrix (GLCM), grey-level run length matrix (GLRLM), grey-level size zone matrix, grey-level dependence matrix (GLDM), neighboring gray tone difference matrix (NGTDM): n=73; and (IV) filter-derived features: filter ‘wavelet’: n=8×91=728; filter ‘LoG’: n=4×91=364; other filter (‘square’, ‘squareroot’, ‘logarithm’, ‘exponential’): n=91×4=364. Subsequently, we used z-score normalization to remove potential batch effects between different centers.
Table 1
Type of feature | Number (total =1,731) |
---|---|
Shape and size feature | 14 |
First-order statistics | 18 |
Texture feature | 73 |
Filter-derived feature | |
Wavelet | 8×91=728 |
LoG | 4×91=364 |
Square | 91 |
Squareroot | 91 |
Logarithm | 91 |
Exponential | 91 |
MRI, magnetic resonance imaging
Feature selection and radiomic model construction
Firstly, the radiomic features with good interobserver reproducibility [intraclass correlation coefficient (ICC) ≥0.75] were included. Then, we used the least absolute shrinkage and selection operator (LASSO) regression to selected features, which can remove unimportant variables by shrinking the coefficient estimates toward zero. In order to identify the best features, we used a 5-fold cross-validation in LASSO regression and then scrambled the data of training set and repeated LASSO regression. After 100 repetitions of above steps, we only selected the features which appeared more than 80 time to build multilayer perceptron (MLP) models. The construction of MLP models involved 5-fold cross-validation to determine the best performance via GridsearchCV package. Finally, the external validation set was used to assess the predictive performance of models for response to nCRT. The predictive models of cCR were based on the entire dataset and predictive models of pCR were built on population that showed cCR.
Statistical analyses
To identify clinical characteristics related to cCR and pCR, we used independent-samples t-test and Mann–Whitney test to analyze variables between the training and external validation set, and univariate and multivariate logistic regression to analyze continuous and categorical variables between different group patients in the training set. Variables with P≤0.05 were picked out as candidates for further model construction. Evaluation of the models included discrimination, calibration, and clinical usefulness. The area under the receiver operating characteristic curve (AUC) was used to evaluate the discrimination ability of models. The Delong test was performed to compare the AUC values among different models. Accuracy, positive predictive value (PPV), and negative predictive value (NPV) were calculated to quantify the discrimination ability of the prediction models. Calibration curves were used to evaluate the consistency between the predicted and actual cCR or pCR rates. Decision curve analysis (DCA) was performed to calculate net benefit (NB) of models in the range of threshold and identify optimal models (23). The SHAP method was used to explain the features’ effects on prediction models and explore relationship of selected features and response to nCRT. A 2-tailed P value <0.05 was considered statistically significant.
The analyses of clinical characteristics were performed using the software SPSS 25.0 (IBM Corp., Armonk, NY, USA). The model construction and assessment were performed using Python version 3.7.0. Code availability: the code can be provided upon request.
Results
Patient characteristics
Patients whose endoscopic results were no lesion and post-MRI assessment were mrTRG0 were grouped into cCR and others were regarded as non-cCR. Of 509 patients with LARCs, 285 who matched the inclusion criteria were selected as the training set (n=241; mean age, 62.58±10.11 years) and external validation set (n=44; mean age 61.16±10.03 years). The patient selection flow chart is shown in Figure 2. Among patients with LARC in training set, 77.54% were male patients and 53.33% were located in middle rectum (Table 2). Most patients were T3 (68.07%) or T4 (31.58%) and involved lymph node (94.04%). EMVI and CRM were 63.86% and 50.18% among patients, respectively. Lateral lymph node involvement appeared in 40.35% of patients, and CEA and CA199 increased in 46.67% and 14.39%, respectively. Besides, comparison of the characteristics of the training set and external validation set, only EMVI showed difference (P=0.04). Among cCR patients, only sex and pathological response showed difference in the training and external validation set (P=0.03 and P=0.04, respectively; Table 3). Further, the clinical pretreatment characteristics of the training sets were analyzed by univariate and multivariate logistic regression to ascertain predictive characteristics. EMVI was significantly associated with cCR (P=0.001; Table S2) in the whole population and T stage was significantly associated with pCR (P=0.050; Table S3) in the cCR population.
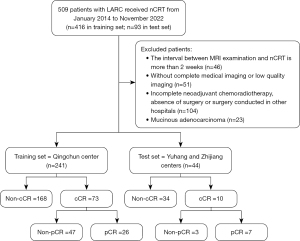
Table 2
Characteristics | Total (n=285) | Training set (n=241) | External validation set (n=44) | P value |
---|---|---|---|---|
Age (mean ± SD, years) | 62.36±10.10 | 62.58±10.11 | 61.16±10.03 | 0.83 |
Sex (percentages) | 0.41 | |||
Male | 221 (77.54) | 189 (78.42) | 32 (72.73) | |
Female | 64 (22.46) | 52 (21.58) | 12 (27.27) | |
Treatment response (percentages) | 0.29 | |||
cCR | 83 (29.12) | 73 (30.29) | 10 (22.73) | |
Non-cCR | 202 (70.88) | 168 (69.71) | 34 (77.27) | |
Location of tumor (percentages) | 0.53 | |||
Low | 87 (30.53) | 70 (29.05) | 17 (38.64) | |
Middle | 152 (53.33) | 129 (53.52) | 23 (52.27) | |
High | 46 (16.14) | 42 (17.43) | 4 (9.09) | |
T stage (percentages) | 0.94 | |||
T2 | 1 (0.35) | 1 (0.41) | 0 (0.00) | |
T3 | 194 (68.07) | 164 (68.05) | 30 (68.18) | |
T4 | 90 (31.58) | 76 (31.54) | 14 (31.82) | |
N stage (percentages) | 0.48 | |||
N0 | 17 (5.96) | 14 (5.81) | 3 (6.82) | |
N1 | 151 (52.98) | 131 (54.36) | 20 (45.45) | |
N2 | 102 (35.79) | 83 (34.44) | 19 (43.18) | |
N3 | 15 (5.26) | 13 (5.39) | 2 (4.55) | |
EMVI* (percentages) | 0.04 | |||
+ | 182 (63.86) | 160 (66.39) | 22 (50.00) | |
− | 103 (36.14) | 81 (33.61) | 22 (50.00) | |
CRM (percentages) | 0.53 | |||
+ | 143 (50.18) | 119 (49.38) | 24 (54.55) | |
− | 142 (49.82) | 122 (50.62) | 20 (45.45) | |
Lateral lymph node (percentages) | 0.94 | |||
+ | 115 (40.35) | 97 (40.25) | 18 (40.91) | |
− | 170 (59.65) | 144 (59.75) | 26 (59.09) | |
CEA (ng/mL) (percentages) | 0.25 | |||
≤5 | 152 (53.33) | 125 (51.87) | 27 (61.36) | |
>5 | 133 (46.67) | 116 (48.13) | 17 (38.64) | |
CA199 (U/mL) (percentages) | 0.54 | |||
≤37 | 244 (85.61) | 205 (85.06) | 39 (88.64) | |
>37 | 41 (14.39) | 36 (14.94) | 5 (11.36) | |
SII (mean ± SD) | 690.98±442.26 | 690.30±452.37 | 694.72±386.90 | 0.95 |
*, P≤0.05, which is considered a statistically significant difference. SD, standard deviation; cCR, clinical complete response; EMVI, extramural vascular invasion; CRM, circumferential resection margin; CEA, carcinoembryonic antigen; CA199, carbohydrate antigen 199; SII, systemic immune-inflammation index.
Table 3
Characteristics | Total (n=83) | Training set (n=73) | External validation set (n=10) | P value |
---|---|---|---|---|
Age (mean ± SD, years) | 61.33±11.13 | 62.03±10.97 | 56.80±11.41 | 0.17 |
Sex* (percentages) | 0.03 | |||
Male | 64 (77.11) | 59 (80.82) | 5 (50.00) | |
Female | 19 (22.89) | 14 (19.18) | 5 (50.00) | |
Pathological response* (percentages) | 0.04 | |||
pCR | 33 (39.76) | 26 (35.62) | 7 (70.00) | |
Non-pCR | 50 (60.24) | 47 (64.38) | 3 (30.00) | |
Location of tumor (percentages) | 0.63 | |||
Low | 25 (30.12) | 21 (28.77) | 4 (40.00) | |
Middle | 45 (54.22) | 40 (54.79) | 5 (50.00) | |
High | 13 (15.66) | 12 (16.44) | 1 (10.00) | |
T stage (percentages) | 0.12 | |||
T3 | 59 (71.08) | 54 (73.97) | 5 (50.00) | |
T4 | 24 (28.92) | 19 (26.03) | 5 (50.00) | |
N stage (percentages) | 0.71 | |||
N0 | 4 (4.82) | 4 (5.48) | 0 (0.00) | |
N1 | 46 (55.42) | 40 (54.79) | 6 (60.00) | |
N2 | 32 (38.55) | 27 (36.99) | 3 (30.00) | |
N3 | 3 (3.61) | 2 (2.74) | 1 (10.00) | |
EMVI (percentages) | 0.53 | |||
+ | 41 (49.40) | 37 (50.68) | 4 (40.00) | |
− | 42 (50.60) | 36 (49.32) | 6 (60.00) | |
CRM (percentages) | 0.43 | |||
+ | 32 (38.55) | 27 (36.99) | 5 (50.00) | |
− | 51 (61.45) | 46 (63.01) | 5 (50.00) | |
Lateral lymph node (percentages) | 0.95 | |||
+ | 34 (40.96) | 30 (41.10) | 4 (40.00) | |
− | 49 (59.04) | 43 (58.90) | 6 (60.00) | |
CEA (ng/mL) (percentages) | 0.56 | |||
≤5 | 47 (56.63) | 44 (60.27) | 3 (30.00) | |
>5 | 36 (43.37) | 29 (39.73) | 7 (70.00) | |
CA199 (U/mL) (percentages) | 0.17 | |||
≤37 | 61 (73.49) | 61 (83.56) | 0 (0.00) | |
>37 | 22 (26.51) | 12 (16.44) | 10 (100.00) | |
SII (mean ± SD) | 655.23±279.43 | 624.92±254.79 | 847.29±386.68 | 0.11 |
*, P≤0.05, which is considered statistically significant difference. cCR, clinical complete response; SD, standard deviation; pCR, pathological complete response; EMVI, extramural vascular invasion; CRM, circumferential resection margin; CEA, carcinoembryonic antigen; CA199, carbohydrate antigen 199; SII, systemic immune-inflammation index.
Feature selection and model construction
T2W and T1W features were selected via LASSO regression respectively. Finally, 3 T2W features and 2 T1W features were identified and used to construct a radiomic model for cCR (Table S4). The only significant clinical characteristic (EMVI) was used together to build prediction model for cCR alone and combined with radiomic features. Furthermore, the selected clinical and radiomic features were used together to build a clinical-radiomic model. For prediction models of pCR, 2 T2W features and 2 T1W features were identified and used to construct radiomic model for pCR. The only significant clinical characteristic (T stage) was used together to build prediction model for pCR alone and combined with radiomic features. All models were built by the MLP classifier and the grid-search method was used to definite the optimal parameters.
Performance of prediction models for cCR and pCR
For prediction models for cCR, the accuracy, PPV, and NPV were 69.71%, 0.00%, and 100.00% for the clinical model, 77.59%, 34.25%, and 96.43% for the radiomic model, and 94.19%, 50.68%, and 88.10% for the clinical-radiomic model in the training set. In the external validation set, the accuracy, PPV, and NPV were 77.27%, 0.00%, and 100.00% for the clinical model, 79.55%, 10.00%, and 100.00% for the radiomic model, and 77.27%, 20.00%, and 94.12% for the clinical-radiomic model (Table 4). The AUC values of the clinical, radiomic, and clinical-radiomic models were 0.613 [95% confidence interval (CI): 0.575–0.653], 0.763 (95% CI: 0.720–0.806), and 0.831 (95% CI: 0.799–0.867), respectively, in the training set (Figure 3A) and 0.565 (95% CI: 0.524–0.615), 0.715 (95% CI: 0.667–0.757), and 0.718 (95% CI: 0.664–0.767), respectively, in the external validation set (Figure 3B). However, the AUC values did not show significant difference in both the clinical model (P=0.15) and radiomic model (P=0.97) compared with the clinical-radiomic model in the external validation set. DCA demonstrated that the clinical-radiomic model could achieve more benefit than a treat-all or treat-none approach, and the clinical-radiomic model had higher NB than the other 2 models (Figure 3C,3D). The calibration curves of radiomic and clinical-radiomic model showed that these 2 models could identify actual cCR patients in the training and external validation sets (Figure 3E,3F).
Table 4
Model | Training set | External validation set | |||||||
---|---|---|---|---|---|---|---|---|---|
AUC (95% CI) | Accuracy | PPV | NPV | AUC (95% CI) | Accuracy | PPV | NPV | ||
cCR | |||||||||
Clinical model | 0.613 (0.575–0.653) | 69.71% | 0.00% | 100.00% | 0.565 (0.524–0.615) | 77.27% | 0.00% | 100.00% | |
Radiomic model | 0.763 (0.720–0.806) | 77.59% | 34.25% | 96.43% | 0.715 (0.667–0.757) | 79.55% | 10.00% | 100.00% | |
Clinical-radiomic model | 0.831 (0.799–0.867) | 94.19% | 50.68% | 88.10% | 0.718 (0.664–0.767) | 77.27% | 20.00% | 94.12% | |
pCR | |||||||||
Clinical model | 0.613 (0.579–0.645) | 64.38% | 0.00% | 100.00% | 0.381 (0.334–0.414) | 30.00% | 0.00% | 100.00% | |
Radiomic model | 0.979 (0.963–0.988) | 94.52% | 96.15% | 93.62% | 0.762 (0.726–0.810) | 50.00% | 28.57% | 100.00% | |
Clinical-radiomic model | 0.970 (0.957–0.986) | 97.26% | 96.15% | 97.87% | 0.810 (0.775–0.847) | 50.00% | 28.57% | 100.00% |
nCRT, neoadjuvant chemoradiotherapy; AUC, area under the receiver operating characteristic curve; CI, confidence interval; PPV, positive predictive value; NPV, negative predictive value; cCR, clinical complete response; pCR, pathological complete response.
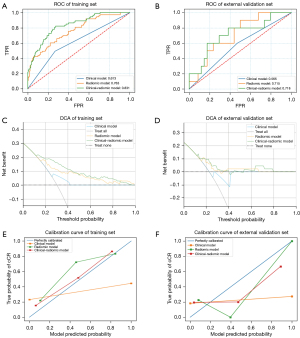
For distinguishing pCR from cCR, the accuracy, PPV, and NPV were 64.38%, 0.00%, and 100.00% for the clinical model, 94.52%, 96.15%, and 93.62% for the radiomic model, and 97.26%, 96.15%, and 97.87% for the clinical-radiomic model, respectively, in the training set. In the external validation set, the accuracy, PPV, and NPV were 30.00%, 0.00%, and 100.00% for the clinical model, 50.00%, 28.57%, and 100.00% for the radiomic model and 50.00%, 28.57%, and 100.00% the clinical-radiomic model, respectively (Table 3). The AUC values of the clinical, radiomic, and clinical-radiomic models were 0.613 (95% CI: 0.579–0.645), 0.979 (95% CI: 0.963–0.988), and 0.970 (95% CI: 0.957–0.986), respectively, in the training set (Figure 4A) and 0.381 (95% CI: 0.334–0.414), 0.762 (95% CI: 0.726–0.810), and 0.810 (95% CI: 0.775–0.847) in the external validation set, respectively (Figure 4B). The high AUC value and relatively low accuracy may be mainly caused by limited and unbalanced samples of pCR. The AUC values did not show significant difference in both the clinical model (P=0.13) and radiomic model (P=0.76) compared with clinical-radiomic model in external validation set. DCA demonstrated that the radiomic model and clinical-radiomic model could achieve more benefit than a treat-all or treat-none approach when the threshold probability was more than 64.2% (Figure 4C,4D). However, the calibration curves of the clinical-radiomic model showed that the models could effectively identify actual pCR patients in the training set, but did not perform well in the external validation set (Figure 4E,4F), which may be caused by limited number of pCR samples in the external validation set.
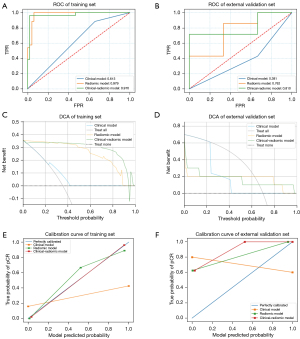
Explanation of prediction models for cCR and pCR
The relationships of the response to nCRT and features, which were used to construct the best predictive model (clinical-radiomic models), were analyzed by SHAP algorithm. In the prediction model of cCR, we identified that EMVI and T1_wavelet-LLL_glrlm_RunEntropy were negatively related to cCR, but T2_exponential_gldm_SmallDependenceLowGrayLevelEmphasis and T2_log-sigma-2-0-mm-3D_glszm_GrayLevelNonUniformityNormalized were positively related to cCR (Figure 5A). The most important features in the clinical-radiomic model were T1_wavelet-LLL_glrlm_RunEntropy (Figure 5B). Besides, we also showed how to interpret the assessment of a single patient on response to nCRT in the model. In assessment of patient 1, the SHAP value was higher than base value, which indicated that this patient was a cCR individual, and the arrows of features exhibited their contribution to assessment of cCR quantitatively (Figure 5C). The value of feature 3 (T1_wavelet-LLL_glrlm_RunEntropy) was −4.54 in this patient and positively contributed to the SHAP value. For patient 2, a non-cCR individual, the SHAP value was obviously lower than base value and the value of feature 3 was 1.26, which decreased the SHAP value.
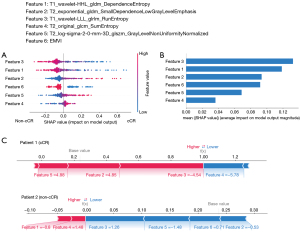
For the prediction model of pCR, T1_wavelet-LLL_firstorder_Range was the most important feature to evaluate pCR and T2_wavelet-HLL_glszm_SizeZoneNonUniformity was the least important feature (Figure 6A,6B). In patient 1, who reached pCR after nCRT, the value of feature 4 (T2_wavelet-HHL_firstorder_Skewness) was 0.87 and made a negative effect on computing the SHAP value, but the value of feature 3 (T2_wavelet-HLL_glszm_SizeZoneNonUniformity) was 0.65 and made a positive effect on computing the SHAP value (Figure 6C). In patient 2, who did not reach pCR after nCRT, the value of feature 4 (T2_wavelet-HHL_firstorder_Skewness) was −1.49 and increased the SHAP value.
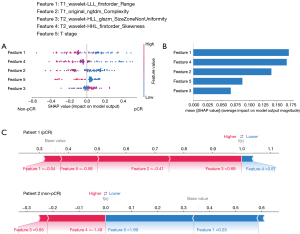
Discussion
Clinical characteristics and MRI have been regularly used to evaluate the condition of patients with LARCs and develop treatment plans. Numerous studies have demonstrated that factors such as EMVI and T stage are associated with the response to nCRT in individuals with CRC (24-26). However, prediction models solely based on clinical characteristics have shown poor performance in discriminating patients with a good response, according to several studies focusing on therapeutic effect evaluation (27-29). The underlying reason for these outcomes may lie in the nature of the rough qualitative assessment of the tumor. For instance, the evaluation of T stage is based on the degree of tumor invasion in the rectum, and higher T stages have been associated with a poorer response (30-32). However, a considerable number of patients with LARCs are at T3 stage, and the traditional T stage system is inadequate in predicting the response among this population. Meanwhile, several studies have demonstrated that tumor size is related to the response to nCRT, with tumors smaller than 3 cm being more likely to achieve pCR regardless of their pretreatment clinical stage (30,33). Although some studies have suggested that the difference in mean tumor size between patients with and without pCR is minor (34,35), a quantitative assessment of the lesion is crucial for precisely judging the response to treatment.
In contrast to the poor performance of clinical models, radiomic-based models have demonstrated promising abilities in identifying patients with a good response in multiple studies (36-40), with several of them involving features extracted from pretreatment MRI (37,38,40). For instance, Liu et al. developed a powerful radiomics model combining pre-treatment and post-treatment data to predict pCR, achieving an impressive AUC of 0.9756; however, their study was limited to a single center and did not discuss the performance of only pretreatment-MRI based models (37). Song et al. successfully developed and validated a robust radiomic-based model to predict the response using a large-scale multicenter dataset based solely on pretreatment T2 MRI and discovered that the protocol of pretreatment was strongly associated with response to therapy, but they also overlooked the effects of pretreatment MRI on assisting on decision of preoperative treatment (40). To summarize, many previous studies have ignored the importance of pretreatment MRI on making therapy protocol. In addition, a great number of these studies mainly focused on identifying pCR patients, overlooking the clinical reality that patients who achieve cCR are often candidates for “watch-and-wait” management due to the safety of the therapy regimen and the challenges of proving pCR.
To confirm the value of pretreatment MRI in decision-making of preoperative protocol and achieving clinical application of radiomics on assessment on response to treatment, our study firstly set cCR as one of the endpoints and demonstrated that the radiomic-based model outperformed the clinical model, particularly in identifying cCR patients. The external validation results further confirmed the powerful predictive capability of radiomics in assessing the response to nCRT in LARC patients, especially when combined with clinical prognostic factors. Moreover, previous research has indicated that the achievement of cCR is related to the choice of therapeutic regimen (41,42) and our study suggests that radiomics could assist oncologists in selecting more intensive treatments among non-cCR patients to potentially achieve more cCR. Furthermore, we validated the ability of radiomics to differentiate pCR patients among cCR patients based on pre-treatment MRIs, considering the minor lesions often observed on post-treatment MRI. The radiomic-based models displayed strong performance in differentiating pCR patients among cCR patients, highlighting the potential of radiomics as a useful tool for assisting oncologists in identifying patients who would benefit from a “watch-and-wait” strategy. Importantly, radiomic-based models offer several advantages, including non-invasiveness, non-tissue-destructiveness, cost-effectiveness, ease of use, and compatibility with existing clinical workflows (43). These features make radiomics a promising and practical tool in the field of oncology.
With the advancement of radiomics, more complex models, such as support vector machines (SVM) and neural networks, have been increasingly utilized to predict treatment outcomes and assess patients, showing promising performance (44-47). However, the "black-box" nature of these models presents challenges in understanding how factors contribute to predictions and limits their application in the clinical setting (48,49). To address this issue, SHAP method has emerged as a valuable approach for interpreting models and has been widely employed in radiomics studies (50-52). According to the results of SHAP analysis, we found that T1_wavelet-LLL_glrlm_RunEntropy was the most important feature for differentiating cCR patients from non-cCR patients, followed by the features of T1_wavelet-HHL_gldm_DependenceEntropy and T2_exponential_gldm_SmallDependenceLowGrayLevelEmphasis. However, the GLDM and GLRLM were both radiomic features reflecting tissue heterogeneity by quantifying the frequency of occurrence of pairs of pixels with the same gray value and a specified spatial relationship in an image (53), and they evaluated the heterogeneity in a different way, which may reflect different information of tumor internal heterogeneity. For predicting pCR among cCR patients, we found that T1_wavelet-LLL_firstorder_Range and T2_wavelet-HHL_firstorder_Skewness were the 2 most important features and both negatively contributed to pCR, supporting the association between heterogeneity and response to treatment.
Numerous studies have demonstrated that the heterogeneity of tumor cell populations is strongly linked to the response to treatment (54,55) and several studies have established the relationship between radiomic features and tumor cell population heterogeneity (56,57). Our SHAP analysis findings not only confirm the association between tumor heterogeneity and the outcomes of nCRT, but also highlight the varying contributions of different radiomic features, reflecting different aspects of tumor heterogeneity, in assessing the treatment response. This insight could potentially lead to a better understanding of treatment outcomes and assist in refining treatment strategies tailored to individual patients.
The present study had several limitations that should be considered. Firstly, we only collected radiomic features from T1W and T2W imaging, and did not include diffusion-weighted imaging (DWI) due to significant variations between centers (58). Meanwhile, other MR sequences with useful information about treatment response (59-61), such as dynamic contrast-enhanced MR were not used in this study. Future radiomic models incorporating more comprehensive medical imaging modalities should be explored to enhance predictive capabilities. Secondly, the number of patients achieving pCR was relatively low due to the strict requirements for complete multiparametric MRI with high quality. Moreover, patients with cCR on nonsurgical management were not included in our study, which might have affected the representation of the cCR group. Future studies with larger sample sizes and more diverse patient populations are warranted to validate our findings. Thirdly, it is important to acknowledge that this study was retrospective in nature. To establish more robust evidence, prospective studies should be designed and conducted in the future. Lastly, the biological interpretation of radiomic features was based on previous research and the SHAP analysis. Although this analysis provided valuable insights into feature importance and associations, rigorous verification through combined radiomic research with pathological examination and advanced sequencing technology is highly encouraged. Addressing these limitations in future research will further enhance the clinical utility and reliability of radiomics in guiding personalized treatment strategies for LARC patients.
Conclusions
Our study demonstrated that the clinical-radiomic model based on pretreatment features effectively assessed the treatment response to nCRT both in the training and external validation sets. The utilization of pretreatment radiomic features appeared to capture tumor internal heterogeneity to some extent, enabling the identification of patients with cCR and facilitating the tailoring of more appropriate therapy protocols for patients with non-cCR. Additionally, radiomics was shown to be a promising tool for supporting oncologists in identifying “watch-and-wait” patients among those with cCR. Moreover, the integration of radiomics with the SHAP method allowed for a better understanding of the factors influencing treatment response, providing radiation oncologists with valuable insights to personalize nCRT in a targeted manner. Overall, our study highlights the potential of radiomics in the field of oncology, offering a non-invasive and effective approach to predict treatment response and optimize therapeutic strategies for patients with LARCs.
Acknowledgments
Funding: This study was supported by
Footnote
Reporting Checklist: The authors have completed the TRIPOD reporting checklist. Available at https://qims.amegroups.com/article/view/10.21037/qims-24-7/rc
Conflicts of Interest: All authors have completed the ICMJE uniform disclosure form (available at https://qims.amegroups.com/article/view/10.21037/qims-24-7/coif). F.Z. and Y.Z. serve as unpaid editorial board members of Quantitative Imaging in Medicine. F.Z. also serves as a researcher in Alibaba-Zhejiang University Joint Research Center of Future Digital Healthcare. All authors report receiving cloud server from Alibaba Cloud. The authors have no other conflicts of interest to declare.
Ethical Statement: The authors are accountable for all aspects of the work in ensuring that questions related to the accuracy or integrity of any part of the work are appropriately investigated and resolved. The retrospective research was approved by the Ethics Committee of the First Affiliated Hospital, Zhejiang University School of Medicine (No. IIT2020-1305) and was conducted in accordance with the Declaration of Helsinki (as revised in 2013). The requirement for informed consent was waived by the Ethics Committee because no identifying detail of the participants was included in this research.
Open Access Statement: This is an Open Access article distributed in accordance with the Creative Commons Attribution-NonCommercial-NoDerivs 4.0 International License (CC BY-NC-ND 4.0), which permits the non-commercial replication and distribution of the article with the strict proviso that no changes or edits are made and the original work is properly cited (including links to both the formal publication through the relevant DOI and the license). See: https://creativecommons.org/licenses/by-nc-nd/4.0/.
References
- Sung H, Ferlay J, Siegel RL, Laversanne M, Soerjomataram I, Jemal A, Bray F. Global Cancer Statistics 2020: GLOBOCAN Estimates of Incidence and Mortality Worldwide for 36 Cancers in 185 Countries. CA Cancer J Clin 2021;71:209-49. [Crossref] [PubMed]
- Siegel RL, Miller KD, Wagle NS, Jemal A. Cancer statistics, 2023. CA Cancer J Clin 2023;73:17-48. [Crossref] [PubMed]
- Benson AB, Venook AP, Al-Hawary MM, Azad N, Chen YJ, Ciombor KK, et al. Rectal Cancer, Version 2.2022, NCCN Clinical Practice Guidelines in Oncology. J Natl Compr Canc Netw 2022;20:1139-67. [Crossref] [PubMed]
- Smith JJ, Strombom P, Chow OS, Roxburgh CS, Lynn P, Eaton A, et al. Assessment of a Watch-and-Wait Strategy for Rectal Cancer in Patients With a Complete Response After Neoadjuvant Therapy. JAMA Oncol 2019;5:e185896. [Crossref] [PubMed]
- Polanco PM, Mokdad AA, Zhu H, Choti MA, Huerta S. Association of Adjuvant Chemotherapy With Overall Survival in Patients With Rectal Cancer and Pathologic Complete Response Following Neoadjuvant Chemotherapy and Resection. JAMA Oncol 2018;4:938-43. [Crossref] [PubMed]
- van der Valk MJM, Hilling DE, Bastiaannet E, Meershoek-Klein Kranenbarg E, Beets GL, Figueiredo NL, Habr-Gama A, Perez RO, Renehan AG, van de Velde CJH. IWWD Consortium. Long-term outcomes of clinical complete responders after neoadjuvant treatment for rectal cancer in the International Watch & Wait Database (IWWD): an international multicentre registry study. Lancet 2018;391:2537-45. [Crossref] [PubMed]
- Nagtegaal ID, Glynne-Jones R. How to measure tumour response in rectal cancer? An explanation of discrepancies and suggestions for improvement. Cancer Treat Rev 2020;84:101964. [Crossref] [PubMed]
- Stodolna A, He M, Vasipalli M, Kingsbury Z, Becq J, Stockton JD, Dilworth MP, James J, Sillo T, Blakeway D, Ward ST, Ismail T, Ross MT, Beggs AD. Clinical-grade whole-genome sequencing and 3' transcriptome analysis of colorectal cancer patients. Genome Med 2021;13:33. [Crossref] [PubMed]
- Zheng H, Liu H, Ge Y, Wang X. Integrated single-cell and bulk RNA sequencing analysis identifies a cancer associated fibroblast-related signature for predicting prognosis and therapeutic responses in colorectal cancer. Cancer Cell Int 2021;21:552. [Crossref] [PubMed]
- Hsu KS, Adileh M, Martin ML, Makarov V, Chen J, Wu C, Bodo S, Klingler S, Sauvé CG, Szeglin BC, Smith JJ, Fuks Z, Riaz N, Chan TA, Nishimura M, Paty PB, Kolesnick R. Colorectal Cancer Develops Inherent Radiosensitivity That Can Be Predicted Using Patient-Derived Organoids. Cancer Res 2022;82:2298-312. [Crossref] [PubMed]
- Liu Z, Li Z, Qu J, Zhang R, Zhou X, Li L, Sun K, Tang Z, Jiang H, Li H, Xiong Q, Ding Y, Zhao X, Wang K, Liu Z, Tian J. Radiomics of Multiparametric MRI for Pretreatment Prediction of Pathologic Complete Response to Neoadjuvant Chemotherapy in Breast Cancer: A Multicenter Study. Clin Cancer Res 2019;25:3538-47. [Crossref] [PubMed]
- Zhong L, Dong D, Fang X, Zhang F, Zhang N, Zhang L, Fang M, Jiang W, Liang S, Li C, Liu Y, Zhao X, Cao R, Shan H, Hu Z, Ma J, Tang L, Tian J. A deep learning-based radiomic nomogram for prognosis and treatment decision in advanced nasopharyngeal carcinoma: A multicentre study. EBioMedicine 2021;70:103522. [Crossref] [PubMed]
- Jazieh K, Khorrami M, Saad A, Gad M, Gupta A, Patil P, Viswanathan VS, Rajiah P, Nock CJ, Gilkey M, Fu P, Pennell NA, Madabhushi A. Novel imaging biomarkers predict outcomes in stage III unresectable non-small cell lung cancer treated with chemoradiation and durvalumab. J Immunother Cancer 2022;10:e003778. [Crossref] [PubMed]
- Ligero M, Garcia-Ruiz A, Viaplana C, Villacampa G, Raciti MV, Landa J, et al. A CT-based Radiomics Signature Is Associated with Response to Immune Checkpoint Inhibitors in Advanced Solid Tumors. Radiology 2021;299:109-19. [Crossref] [PubMed]
- Lucia F, Visvikis D, Desseroit MC, Miranda O, Malhaire JP, Robin P, Pradier O, Hatt M, Schick U. Prediction of outcome using pretreatment (18)F-FDG PET/CT and MRI radiomics in locally advanced cervical cancer treated with chemoradiotherapy. Eur J Nucl Med Mol Imaging 2018;45:768-86. [Crossref] [PubMed]
- Petresc B, Lebovici A, Caraiani C, Feier DS, Graur F, Buruian MM. Pre-Treatment T2-WI Based Radiomics Features for Prediction of Locally Advanced Rectal Cancer Non-Response to Neoadjuvant Chemoradiotherapy: A Preliminary Study. Cancers (Basel) 2020.
- Bordron A, Rio E, Badic B, Miranda O, Pradier O, Hatt M, Visvikis D, Lucia F, Schick U, Bourbonne V. External Validation of a Radiomics Model for the Prediction of Complete Response to Neoadjuvant Chemoradiotherapy in Rectal Cancer. Cancers (Basel) 2022;14:1079. [Crossref] [PubMed]
- Giannini V, Mazzetti S, Bertotto I, Chiarenza C, Cauda S, Delmastro E, Bracco C, Di Dia A, Leone F, Medico E, Pisacane A, Ribero D, Stasi M, Regge D. Predicting locally advanced rectal cancer response to neoadjuvant therapy with (18)F-FDG PET and MRI radiomics features. Eur J Nucl Med Mol Imaging 2019;46:878-88. [Crossref] [PubMed]
- Xu Y, Liu X, Cao X, Huang C, Liu E, Qian S, et al. Artificial intelligence: A powerful paradigm for scientific research. Innovation (Camb) 2021;2:100179. [Crossref] [PubMed]
- Rodríguez-Pérez R, Bajorath J. Interpretation of Compound Activity Predictions from Complex Machine Learning Models Using Local Approximations and Shapley Values. J Med Chem 2020;63:8761-77. [Crossref] [PubMed]
- Amin MB, Edge SB, Greene FL, Byrd DR, Brookland RK, Washington MK, et al. editors. AJCC Cancer Staging Manual, 8th edition. Chicago, USA: Springer, 2017.
- van Griethuysen JJM, Fedorov A, Parmar C, Hosny A, Aucoin N, Narayan V, Beets-Tan RGH, Fillion-Robin JC, Pieper S, Aerts HJWL. Computational Radiomics System to Decode the Radiographic Phenotype. Cancer Res 2017;77:e104-7. [Crossref] [PubMed]
- Van Calster B, Wynants L, Verbeek JFM, Verbakel JY, Christodoulou E, Vickers AJ, Roobol MJ, Steyerberg EW. Reporting and Interpreting Decision Curve Analysis: A Guide for Investigators. Eur Urol 2018;74:796-804. [Crossref] [PubMed]
- Xiao WW, Li M, Guo ZW, Zhang R, Xi SY, Zhang XG, et al. A Genotype Signature for Predicting Pathologic Complete Response in Locally Advanced Rectal Cancer. Int J Radiat Oncol Biol Phys 2021;110:482-91. [Crossref] [PubMed]
- Ying HQ, Sun F, Liao YC, Cai D, Yang Y, Cheng XX. The value of circulating fibrinogen-to-pre-albumin ratio in predicting survival and benefit from chemotherapy in colorectal cancer. Ther Adv Med Oncol 2021;13:17588359211022886. [Crossref] [PubMed]
- Joye I, Debucquoy A, Fieuws S, Wolthuis A, Sagaert X, D'Hoore A, Haustermans K. Can clinical factors be used as a selection tool for an organ-preserving strategy in rectal cancer? Acta Oncol 2016;55:1047-52. [Crossref] [PubMed]
- Lu S, Liu Z, Wang Y, Meng Y, Peng R, Qu R, Zhang Z, Fu W, Wang H. A novel prediction model for pathological complete response based on clinical and blood parameters in locally advanced rectal cancer. Front Oncol 2022;12:932853. [Crossref] [PubMed]
- Mbanu P, Saunders MP, Mistry H, Mercer J, Malcomson L, Yousif S, Price G, Kochhar R, Renehan AG, van Herk M, Osorio EV. Clinical and radiomics prediction of complete response in rectal cancer pre-chemoradiotherapy. Phys Imaging Radiat Oncol 2022;23:48-53. [Crossref] [PubMed]
- Cheng Y, Luo Y, Hu Y, Zhang Z, Wang X, Yu Q, Liu G, Cui E, Yu T, Jiang X. Multiparametric MRI-based Radiomics approaches on predicting response to neoadjuvant chemoradiotherapy (nCRT) in patients with rectal cancer. Abdom Radiol (NY) 2021;46:5072-85. [Crossref] [PubMed]
- Hammarström K, Imam I, Mezheyeuski A, Ekström J, Sjöblom T, Glimelius B. A Comprehensive Evaluation of Associations Between Routinely Collected Staging Information and The Response to (Chemo)Radiotherapy in Rectal Cancer. Cancers (Basel) 2020;13:16. [Crossref] [PubMed]
- Peng H, Wang C, Xiao W, Lin X, You K, Dong J, Wang Z, Yu X, Zeng Z, Zhou T, Gao Y, Wen B. Analysis of Clinical characteristics to predict pathologic complete response for patients with locally advanced rectal cancer treated with neoadjuvant chemoradiotherapy. J Cancer 2018;9:2687-92. [Crossref] [PubMed]
- Tan Y, Fu D, Li D, Kong X, Jiang K, Chen L, Yuan Y, Ding K. Predictors and Risk Factors of Pathologic Complete Response Following Neoadjuvant Chemoradiotherapy for Rectal Cancer: A Population-Based Analysis. Front Oncol 2019;9:497. [Crossref] [PubMed]
- Bitterman DS, Resende Salgado L, Moore HG, Sanfilippo NJ, Gu P, Hatzaras I, Du KL. Predictors of Complete Response and Disease Recurrence Following Chemoradiation for Rectal Cancer. Front Oncol 2015;5:286. [Crossref] [PubMed]
- Garland ML, Vather R, Bunkley N, Pearse M, Bissett IP. Clinical tumour size and nodal status predict pathologic complete response following neoadjuvant chemoradiotherapy for rectal cancer. Int J Colorectal Dis 2014;29:301-7. [Crossref] [PubMed]
- Moureau-Zabotto L, Farnault B, de Chaisemartin C, Esterni B, Lelong B, Viret F, Giovannini M, Monges G, Delpero JR, Bories E, Turrini O, Viens P, Salem N. Predictive factors of tumor response after neoadjuvant chemoradiation for locally advanced rectal cancer. Int J Radiat Oncol Biol Phys 2011;80:483-91. [Crossref] [PubMed]
- Shin J, Seo N, Baek SE, Son NH, Lim JS, Kim NK, Koom WS, Kim S. MRI Radiomics Model Predicts Pathologic Complete Response of Rectal Cancer Following Chemoradiotherapy. Radiology 2022;303:351-8. [Crossref] [PubMed]
- Liu Z, Zhang XY, Shi YJ, Wang L, Zhu HT, Tang Z, Wang S, Li XT, Tian J, Sun YS. Radiomics Analysis for Evaluation of Pathological Complete Response to Neoadjuvant Chemoradiotherapy in Locally Advanced Rectal Cancer. Clin Cancer Res 2017;23:7253-62. [Crossref] [PubMed]
- Mao Y, Pei Q, Fu Y, Liu H, Chen C, Li H, Gong G, Yin H, Pang P, Lin H, Xu B, Zai H, Yi X, Chen BT. Pre-Treatment Computed Tomography Radiomics for Predicting the Response to Neoadjuvant Chemoradiation in Locally Advanced Rectal Cancer: A Retrospective Study. Front Oncol 2022;12:850774. [Crossref] [PubMed]
- Jin C, Yu H, Ke J, Ding P, Yi Y, Jiang X, Duan X, Tang J, Chang DT, Wu X, Gao F, Li R. Predicting treatment response from longitudinal images using multi-task deep learning. Nat Commun 2021;12:1851. [Crossref] [PubMed]
- Song M, Li S, Wang H, Hu K, Wang F, Teng H, Wang Z, Liu J, Jia AY, Cai Y, Li Y, Zhu X, Geng J, Zhang Y, Wan X, Wang W. MRI radiomics independent of clinical baseline characteristics and neoadjuvant treatment modalities predicts response to neoadjuvant therapy in rectal cancer. Br J Cancer 2022;127:249-57. [Crossref] [PubMed]
- Appelt AL, Pløen J, Vogelius IR, Bentzen SM, Jakobsen A. Radiation dose-response model for locally advanced rectal cancer after preoperative chemoradiation therapy. Int J Radiat Oncol Biol Phys 2013;85:74-80. [Crossref] [PubMed]
- Gash KJ, Baser O, Kiran RP. Factors associated with degree of tumour response to neo-adjuvant radiotherapy in rectal cancer and subsequent corresponding outcomes. Eur J Surg Oncol 2017;43:2052-9. [Crossref] [PubMed]
- Bera K, Braman N, Gupta A, Velcheti V, Madabhushi A. Predicting cancer outcomes with radiomics and artificial intelligence in radiology. Nat Rev Clin Oncol 2022;19:132-46. [Crossref] [PubMed]
- Sun K, Shi L, Qiu J, Pan Y, Wang X, Wang H. Multi-phase contrast-enhanced magnetic resonance image-based radiomics-combined machine learning reveals microscopic ultra-early hepatocellular carcinoma lesions. Eur J Nucl Med Mol Imaging 2022;49:2917-28. [Crossref] [PubMed]
- Mukherjee S, Patra A, Khasawneh H, Korfiatis P, Rajamohan N, Suman G, Majumder S, Panda A, Johnson MP, Larson NB, Wright DE, Kline TL, Fletcher JG, Chari ST, Goenka AH. Radiomics-based Machine-learning Models Can Detect Pancreatic Cancer on Prediagnostic Computed Tomography Scans at a Substantial Lead Time Before Clinical Diagnosis. Gastroenterology 2022;163:1435-46.e3. [Crossref] [PubMed]
- Zheng X, Yao Z, Huang Y, Yu Y, Wang Y, Liu Y, Mao R, Li F, Xiao Y, Wang Y, Hu Y, Yu J, Zhou J. Deep learning radiomics can predict axillary lymph node status in early-stage breast cancer. Nat Commun 2020;11:1236. [Crossref] [PubMed]
- Xu Y, Hosny A, Zeleznik R, Parmar C, Coroller T, Franco I, Mak RH, Aerts HJWL. Deep Learning Predicts Lung Cancer Treatment Response from Serial Medical Imaging. Clin Cancer Res 2019;25:3266-75. [Crossref] [PubMed]
- Hauser K, Kurz A, Haggenmüller S, Maron RC, von Kalle C, Utikal JS, et al. Explainable artificial intelligence in skin cancer recognition: A systematic review. Eur J Cancer 2022;167:54-69. [Crossref] [PubMed]
- Nensa F, Demircioglu A, Rischpler C. Artificial Intelligence in Nuclear Medicine. J Nucl Med 2019;60:29S-37S. [Crossref] [PubMed]
- Manikis GC, Ioannidis GS, Siakallis L, Nikiforaki K, Iv M, Vozlic D, Surlan-Popovic K, Wintermark M, Bisdas S, Marias K. Multicenter DSC-MRI-Based Radiomics Predict IDH Mutation in Gliomas. Cancers (Basel) 2021.
- Wang Y, Lang J, Zuo JZ, Dong Y, Hu Z, Xu X, Zhang Y, Wang Q, Yang L, Wong STC, Wang H, Li H. The radiomic-clinical model using the SHAP method for assessing the treatment response of whole-brain radiotherapy: a multicentric study. Eur Radiol 2022;32:8737-47. [Crossref] [PubMed]
- Zhang R, Hong M, Cai H, Liang Y, Chen X, Liu Z, Wu M, Zhou C, Bao C, Wang H, Yang S, Hu Q. Predicting the pathological invasiveness in patients with a solitary pulmonary nodule via Shapley additive explanations interpretation of a tree-based machine learning radiomics model: a multicenter study. Quant Imaging Med Surg 2023;13:7828-41. [Crossref] [PubMed]
- Abbasian Ardakani A, Bureau NJ, Ciaccio EJ, Acharya UR. Interpretation of radiomics features-A pictorial review. Comput Methods Programs Biomed 2022;215:106609. [Crossref] [PubMed]
- Braun R, Anthuber L, Hirsch D, Wangsa D, Lack J, McNeil NE, et al. Single-Cell-Derived Primary Rectal Carcinoma Cell Lines Reflect Intratumor Heterogeneity Associated with Treatment Response. Clin Cancer Res 2020;26:3468-80. [Crossref] [PubMed]
- Qi J, Sun H, Zhang Y, Wang Z, Xun Z, Li Z, et al. Single-cell and spatial analysis reveal interaction of FAP(+) fibroblasts and SPP1(+) macrophages in colorectal cancer. Nat Commun 2022;13:1742. [Crossref] [PubMed]
- Tomaszewski MR, Gillies RJ. The Biological Meaning of Radiomic Features. Radiology 2021;298:505-16. [Crossref] [PubMed]
- Li G, Li L, Li Y, Qian Z, Wu F, He Y, Jiang H, Li R, Wang D, Zhai Y, Wang Z, Jiang T, Zhang J, Zhang W. An MRI radiomics approach to predict survival and tumour-infiltrating macrophages in gliomas. Brain 2022;145:1151-61. [Crossref] [PubMed]
- Schurink NW, van Kranen SR, Roberti S, van Griethuysen JJM, Bogveradze N, Castagnoli F, El Khababi N, Bakers FCH, de Bie SH, Bosma GPT, Cappendijk VC, Geenen RWF, Neijenhuis PA, Peterson GM, Veeken CJ, Vliegen RFA, Beets-Tan RGH, Lambregts DMJ. Sources of variation in multicenter rectal MRI data and their effect on radiomics feature reproducibility. Eur Radiol 2022;32:1506-16. [Crossref] [PubMed]
- Ter Maat LS, van Duin IAJ, Elias SG, van Diest PJ, Pluim JPW, Verhoeff JJC, de Jong PA, Leiner T, Veta M, Suijkerbuijk KPM. Imaging to predict checkpoint inhibitor outcomes in cancer. A systematic review. Eur J Cancer 2022;175:60-76. [Crossref] [PubMed]
- Mu W, Jiang L, Zhang J, Shi Y, Gray JE, Tunali I, Gao C, Sun Y, Tian J, Zhao X, Sun X, Gillies RJ, Schabath MB. Non-invasive decision support for NSCLC treatment using PET/CT radiomics. Nat Commun 2020;11:5228. [Crossref] [PubMed]
- Feng Y, Gong J, Hu T, Liu Z, Sun Y, Tong T. Radiomics for predicting survival in patients with locally advanced rectal cancer: a systematic review and meta-analysis. Quant Imaging Med Surg 2023;13:8395-412. [Crossref] [PubMed]