Multiparametric simultaneous hybrid 18F-fluorodeoxyglucose positron emission tomography/magnetic resonance imaging (18F-FDG PET/MRI) incorporating intratumoral and peritumoral regions for grading of glioma
Introduction
Optimized management of glioma, the most common primary brain tumor, remains a major global concern (1). According to the 2021 World Health Organization (WHO) classification of central nervous system tumors (2), the diagnosis of gliomas should be an overall “integrated” diagnosis that is reached according to the histopathological denomination, grade, and molecular information. The imaging and neuroradiologist play pivotal roles in glioma diagnosis; the neuroradiologist raises an initial diagnosis of glioma, and the results are confirmed further by histopathological exam, whereas the imaging provides the information of lesion localization, disease extent, and aids tumor grading and surgical planning (3). Patient survival varies greatly depending on tumor grade, with high-grade glioma (HGG, grade 3+4) having a very high mortality rate (e.g., 5-year survival rate <5%), nevertheless, low-grade glioma (LGG, grade 1+2) achieves a survival rate as high as 80% (4). Additionally, the treatment regimens including the degree of resection, postsurgical radiation, or temozolomide combined with radiation are also implemented according to the glioma’s grade. Therefore, accurate pre-operative grading of glioma is critical for clinical decision-making in order to maximize prognosis and patient-tailored precision medicine; however, post-operative histopathological assessment remains the standard for grading gliomas (5). Some patients cannot tolerate surgery or biopsy, in addition, the pathological diagnosis from stereotactic biopsy or surgical resection may be inaccurate due to the tissue heterogeneity and sampling bias (6).
Conventional or advanced magnetic resonance imaging (MRI) have been used to grade gliomas for decades. However, its performance in clinical practice remains unsatisfactory (7). As pathophysiological alterations in tumors are closely associated with aberrant vascularization, hemodynamic imaging, particularly arterial spin labelling (ASL), has been employed to grade glioma due to its advantage of not requiring a tracer (8). Recently, ASL was promoted for employment as a routine clinical setting for glioma (9). Diffusion-weighted imaging (DWI) is a classical and well-known technology for glioma evaluation, but there are no reliable and uniformly accepted threshold values to properly establish grades. Therefore, the value of single imaging techniques remains limited for identifying HGG. Combination of DWI-derived apparent diffusion coefficient (ADC) and ASL has been recommended to simultaneously assess the cellular and vascular properties of gliomas and are viewed as useful biomarkers for grading (10-12). Multiparametric MRI show greater potential in determination of HGGs than does single parameter, but the metabolic behavior of tumors was considered as another valuable biomarker for glioma evaluation.
Positron emission tomography (PET) can visualize biological processes such as cell proliferation, membrane biosynthesis, and glucose consumption by employing various tracers, and thus it has been recommended as standard framework in clinical practice to assist in the management of gliomas (13,14). PET can identify highly metabolically active tissue by measuring uptake of fluorodeoxyglucose (FDG) or other radiotracers, and provide additional insight beyond MRI into the biology of gliomas which may facilitate noninvasive grading of glioma (15). Combining PET and MRI can help procure structural, functional, and metabolic information for glioma in a single examination (16-18), showing additional value to each technique employed alone, especially with the hybrid PET/MRI systems. 18F-fluorodeoxyglucose (18F-FDG), a radiopharmaceutical that is most commonly used as PET tracer for its higher glucose metabolism that tumor cells exhibit compared to healthy tissues (19), shows superior specificity for grading glioma (20,21). Although 18F-fluoro-ethyl-L-tyrosine (FET) has demonstrated higher diagnostic performance for the diagnosis of brain tumor and glioma, it has shown similar performance for distinguishing LGG and HGG in comparison with 18F-FDG (22). Additionally, as 18F-FDG can be applied at all PET sites, we must concede the major convenience of tracer supply in clinical practice. Theoretically, combined parameters from 18F-FDG hybrid PET/MRI may provide sufficient complementary information to improve the grading of gliomas.
Recent studies have found that the peritumoral region (PTR), a region that appears radiologically and macroscopically normal, is crucial for tumor classification (23). Reports have shown that tumor cell infiltration can extend several centimeters beyond the tumor margin (24), thus, a thorough understanding of the actual extent of the region beyond MRI-defined abnormalities is crucial for improving the management of glioma, such as to help determine new therapeutic targets (25). DWI (26) and perfusion imaging (27) have been used to detect alterations in PTR. Combination of ASL, DWI, and 18F-FDG PET parameters from the enhancing tumors and PTR has shown good performance to differentiate HGGs from lymphomas (28). However, multiple parameters derived from the solid component and PTR have not been used for grading gliomas. We hypothesized that multiple parameters derived from 18F-FDG PET/MRI of multiple tumoral regions would improve the grading performance.
Therefore, we combined multiparameters derived from hybrid 18F-FDG PET/MRI of the solid component and PTR to differentiate LGG from HGG, and expected to provide comprehensive physiological and biochemical information that can potentially improve the accuracy of glioma grading. We present this article in accordance with the TRIPOD reporting checklist (available at https://qims.amegroups.com/article/view/10.21037/qims-24-280/rc).
Methods
Patients
The 18F-FDG PET/MRI examination was approved by the Institutional Review Board of Guangzhou Universal Medical Imaging Diagnostic Center (No. 003/2019) and conducted in accordance with the Declaration of Helsinki (as revised in 2013). All patients signed an informed consent form for the 18F-FDG PET/MRI examination. Patients with histologically confirmed gliomas and who had undergone pre-treatment brain 18F-FDG PET/MRI between May 2019 and March 2023 at Guangzhou Universal Medical Imaging Diagnostic Center were retrospectively enrolled. The data applied in this study were exempted from ethical review due to the retrospective nature.
Totally, 131 patients were initially enrolled. The inclusion criteria were as follows: (I) complete 18F-FDG PET/MRI data including T1-weighted imaging (T1WI), T2-weighted imaging (T2WI), T2-weighted fluid-attenuated inversion recovery (T2-FLAIR), DWI, ASL, and 18F-FDG PET before surgery; (II) histopathologically-confirmed glioma without other brain tumors; and (III) no history of treatment, including steroid treatment, radiotherapy, or chemotherapy. The exclusion criteria were as follows: (I) ambiguous grade, especially between grade II and III; (II) incomplete exam or poor image quality (such as motion artifacts); (III) unusual glioma location (e.g., pineal gland, close to the lateral ventricle which may be contaminated by cerebrospinal fluid) that could influence data analysis; (IV) lesions below 5–10 mm; and (V) obvious hemorrhage or large amount of calcification. Tumor grading was based on the 2016 World Health Organization classification, ascertained during operation or via stereotactic brain biopsy. Grades I and II were grouped together as LGG, whereas grade III and IV were grouped together as HGG.
The flowchart for patient enrollment is shown in Figure 1. The participants’ clinical and conventional imaging features, including age, sex, location, cystic degeneration/necrosis, range of involvement, mass effect, and the detailed histopathology were collected from the hospital electronic system. Finally, 41 patients with HGGs [mean age, 51.54 years (range, 29–79 years)] and 35 patients with LGGs [mean age, 45.06 years (range, 23–69 years)] met the inclusion criteria.
PET/MRI image acquisition
PET/MRI images were obtained on an integrated 3T PET/MRI system (Biograph mMRI; Siemens Healthcare, Erlangen, Germany) with a 12-channel, PET-compatible receiver head coil. All patients had fasting blood glucose level <8.3 mmol/L (150 mg/dL) and had fasted for at least 6 hours prior to examination. Before imaging, FDG was administered intravenously according to the patient’s weight, with a standard of 3.70–5.55 MBq/kg. After 40 minutes of uptake in a dimly lit room with eyes open, the patient was positioned for brain imaging.
The comprehensive protocol included the following MRI sequences acquired simultaneously during PET:
- 3D-T1WI-magnetization-prepared rapid gradient echo (MPRAGE): repetition time/echo time (TR/TE), 2,000/2.3 ms; slice thickness/gap, 5/1.5 mm; field of view (FOV), 250×250 mm2; matrix, 256×256;
- T2WI: TR/TE, 5,000/91 ms; slice thickness/gap, 5/1.5 mm; FOV, 230×201 mm2; matrix, 384×307;
- T2-FLAIR: TR/TE, 9,000/102 ms; slice thickness/gap, 5/1.5 mm; FOV, 230×201 mm2; matrix, 256×230;
- Single-shot echo-planar DWI (b=0 and 1,000 s/mm2): TR/TE, 5,900/85 ms; slice thickness/gap, 5/1.5 mm; FOV, 230×201 mm2; matrix, 146×146;
- ASL: TR/TE, 2,500/13 ms; slice thickness/gap, 8/2 mm; FOV, 256×256 mm2; matrix, 64×64. The CBF maps were automatically generated after the scan.
Brain PET images were simultaneously acquired with the MRI acquisition without re-positioning. The attenuation correction method of tissue segmentation and iterative reconstruction algorithm with 21 subsets, 3 iterations, and 4 mm (full width at half maximum) post-filtering was used.
Image analysis
Images were transferred to a postprocessing workstation (Syngovia 3.0, Siemens Healthcare, Germany) and independently interpreted by 2 experienced radiologists (P.L. and G.H.J. with 15 and 20 years of experience in neuroradiology, respectively) who were blinded to tumor grade and patient identity. Location, necrosis, and cystic degeneration were qualitatively assessed on conventional MRI. As the relative values, obtained by normalizing the ROI to the normal appearing (contralateral) tissue using mirrored ROI, may help improve accuracy in tumor grading (29). Thus, we used the relative values (the values of ROI divided by the value of the mirrored ROI from the contralateral normal-appearing brain regions) of PET, CBF, and ADC for quantitative assessment. The delineation of the margin was performed by the previous 2 neuroradiologists; any disagreements about the placement of ROIs within each lesion and the definition of the solid component of the glioma were resolved by consensus or with the assistance of a senior radiologist with over 30 years of experience in brain tumor diagnosis. The pipeline of the process of the measurements of multiple parameters in our study are shown in Figure 2 and as follows:
- The cross-section with the largest tumor area and best demarcation of the lesions was chosen, and the T2-FLAIR and T2WI images were used as visual aid.
- The solid component of glioma was first identified, on combination of the T2-FLAIR (a slightly higher signal intensity than normal brain tissue but lower than edema) and PET images, PTR with 5, 10, 15, and 20 mm adjacent to the margin of the solid region were defined (Figure 2A), 5 round ROIs with a diameter of 5 mm were placed in the solid part without overlap, and the average values were recorded for analysis. Areas adjacent to hemorrhage, vessels, and cystic components were carefully avoided and excluded. Another 4 ROIs were then copy-and-pasted onto the PTR at different distances with 5, 10, 15, and 20 mm adjacent to the margin of the solid region. Additionally, the ROIs were copied into the contralateral normal-appearing tissue as mirrored ROI (Figure 2B).
- ROIs were copied onto maps of ADC maps (Figure 2C), and CBF maps (Figure 2D).
- The maximum standardized uptake value (SUVmax), CBF, and minimum apparent diffusion coefficient (ADCmin) were recorded for each ROI. The relative values of these multiparameters, including relative maximum standardized uptake value (rSUVmax), relative cerebral blood flow (rCBF), and relative minimum apparent diffusion coefficient (rADCmin), which were generated by the values of an ROI from the tumor divided by the value of the mirrored ROI, were measured for analysis. Additionally, in order to be interpreted conveniently, rSUVmax values from ROIs at distinct locations was recorded as follows: rSUVmax-solid, rSUVmax-5, rSUVmax-10, rSUVmax-15, and rSUVmax-20. Similarly, the rCBF and minimum ADC values were recorded: rCBFsolid, rCBF5, rCBF10, rCBF15 and rCBF20; rADCmin-solid, rADCmin-5, rADCmin-10, rADCmin-15, and rADCmin-20.
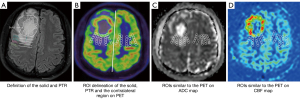
Statistical analysis
Statistical analysis was performed on SPSS software (version 26, IBM Corp., Armonk, NY, USA). A P value <0.05 was considered statistically significant.
Continuous variables were expressed as mean ± standard deviation (SD) and compared using independent-sample t-tests. Categorical data were expressed as numbers and percentages (%). The interclass correlation coefficient (ICC) was calculated to assess measurement consistency between the 2 radiologists. ICC ≤0.40 indicated poor consistency, 0.40< ICC ≤0.75 medium consistency, and ICC >0.75 high consistency. Kolmogorov-Smirnov tests were used to assess the normality of quantitative data. Imaging parameters across HGG and LGG groups were compared using the 2-sample t-test or the Mann-Whitney U test. Receiver operating characteristic (ROC) curves for parameters (single or combined) were used to evaluate their efficiency in discriminating HGGs form LGGs.
An individualized nomogram prediction model was constructed to predict the probability of HGG. The nomogram performance was evaluated by discrimination and calibration. The discriminative ability of the prediction model was determined by concordance index (C-index). A visual calibration plot, comparing the predicted and actual probability of HGG, was performed to calibrate the prediction model. The nomogram was subjected to 1,000 bootstrap resamples for internal validation to assess predictive accuracy (30).
The overall workflow of this study is shown in Figure 3.
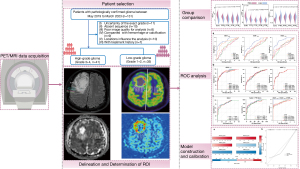
Results
Demographic characteristics and conventional MRI features
Of the 41 HGGs, 21 had glioblastomas, 13 anaplastic astrocytoma, and 7 anaplastic oligodendroglioma. Among 35 LGGs, 23 had astrocytoma and 12 had oligodendrogliomas. Demographic characteristics and conventional MRI features are summarized in Table 1. Age and sex were comparable across groups.
Table 1
Variable | HGG (n=41) | LGG (n=35) | P value |
---|---|---|---|
Age (years), mean ± SD | 51.54±10.54 | 45.06±12.20 | 0.299 |
Gender, n (%) | 0.927 | ||
Male | 18 (43.9) | 15 (42.9) | |
Female | 23 (56.1) | 20 (57.1) | |
Location (n) | |||
Frontal lobe | 9 | 7 | NA |
Parietal lobe | 3 | 5 | NA |
Temporal lobe | 6 | 4 | NA |
Occipital lobe | 13 | 13 | NA |
Multiple lobes | 10 | 6 | NA |
Cystic degeneration/necrosis, n (%) | 0.729 | ||
Yes | 25 (61.0) | 22 (62.9) | |
No | 16 (39.0) | 13 (37.1) | |
Mass effect, n (%) | 0.107 | ||
Yes | 17 (41.5) | 21 (60.0) | |
No | 24 (58.5) | 14 (40.0) | |
IDH mutation, n (%) | 0.377 | ||
Yes | 17 (41.5) | 15 (42.9) | |
No | 18 (43.9) | 11 (31.4) | |
NOS | 6 (14.6) | 9 (25.7) | |
Type [n] | NA | ||
Glioblastoma [21] | Astrocytoma [23] | ||
Anaplastic astrocytoma [13] | Oligodendroglioma [12] | ||
Anaplastic oligodendroglioma [7] |
Statistical significance is indicated by P values less than 0.05. MRI, magnetic resonance imaging; HGG, high-grade glioma; LGG, low-grade glioma; SD, standard deviation; NA, not applicable; IDH, isocitrate dehydrogenase; NOS, not otherwise specified.
Interobserver agreement of the multiparametric measurements
The degree of interobserver agreement between the 2 operators for measuring all hybrid PET/MRI parameters were good to excellent (ICC >0.785) (Table S1).
Differences in rSUVmax, rCBF, and rADCmin between HGG and LGG
Detailed and visualized differences in multiparametric measurements between the HGG and LGG groups are presented in Table S2 and Figure 4. The HGG group showed a tendency for higher rSUVmax, lower rADCmin, and higher rCBF values than did the LGG group, no matter whether there was a statistical difference. Significant inter-group differences were observed mainly in the solid component (all P<0.000, respectively), PTR5 (P<0.000 for rSUVmax and rCBF, P<0.001 for rADCmin), and PTR10 (P<0.000 for rSUVmax, P=0.011 for rADCmin, and P=0.002 for rCBF). Examples of patients with HGG and LGG are shown in Figure 5.
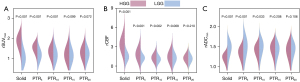
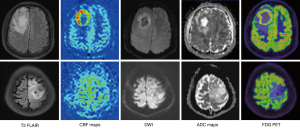
Diagnostic performance analysis
Diagnostic performance of single parameters
ROC curve analysis indicated that rSUVmax-solid performed the best for grading as a single parameter, with the largest area under the curve (AUC) value of 0.884, followed by rADCmin-solid (0.835) and rCBFsolid (0.748). The optimal cut-off value of rSUVmax-solid was 1.388, with a sensitivity and specificity of 87.8% and 85.14%, respectively. The optimal cutoff values, AUC, sensitivity, and specificity are summarized in Table 2, whereas the visualized ROC curves are displayed in Figure 6A.
Table 2
Parameters | AUC (95% CI) | Cutoff value | Sensitivity (%) | Specificity (%) | Youden index |
---|---|---|---|---|---|
rSUVmax | |||||
Solid | 0.884 (0.803–0.927) | 1.388 | 72.7 | 85.1 | 0.578 |
PTR5 | 0.775 (0.695–0.862) | 1.319 | 72.2 | 78.3 | 0.505 |
PTR10 10 mm | 0.617 (0.497–0.706) | 1.178 | 74.6 | 49.1 | 0.238 |
PTR15 15 mm | 0.555 (0.462–0.670) | 1.096 | 64.4 | 52.7 | 0.184 |
PTR20 20 mm | 0.548 (0.505–0.708) | 1.102 | 46.3 | 68.6 | 0.217 |
rADCmin | |||||
Solid | 0.835 (0.768–0.910) | 0.982 | 84.4 | 84.6 | 0.563 |
PTR5 | 0.659 (0.566–0.760) | 0.625 | 94.3 | 42.4 | 0.376 |
PTR10 10 mm | 0.553 (0.495–0.700) | 0.799 | 87.4 | 36.9 | 0.225 |
PTR15 15 mm | 0.678 (0.541–0.740) | 0.678 | 42.0 | 81.1 | 0.322 |
PTR20 20 mm | 0.624 (0.492–0.695) | 0.624 | 22.9 | 93.7 | 0.227 |
rCBF | |||||
Solid | 0.748 (0.680–0.846) | 1.080 | 77.1 | 59.4 | 0.387 |
PTR5 | 0.617 (0.516–0.717) | 1.131 | 59.0 | 56.0 | 0.193 |
PTR10 10 mm | 0.533 (0.429–0.636) | 1.621 | 25.9 | 90.9 | 0.155 |
PTR15 15 mm | 0.597 (0.496–0.698) | 1.332 | 46.3 | 74.5 | 0.207 |
PTR20 20 mm | 0.593 (0.491–0.694) | 1.761 | 37.8 | 91.4 | 0.222 |
AUC, area under the curve; CI, confidence interval; rSUVmax, relative maximum standardized uptake value; PTR5, the 5-mm PTR; PTR10, the 10-mm PTR; PTR15, the 15-mm PTR; PTR20, the 20-mm PTR; PTR, peritumoral zone; rCBF, relative cerebral blood flow; rADCmin, relative minimum apparent diffusion coefficient.
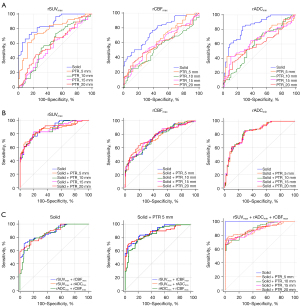
Diagnostic performance of combined parameters at different regions
As shown in Table 3, when combining the multiparametric indices for the center of the solid component and PTR at distinct distances, the AUC values gradually increased, indicating that the diagnostic performance improved when values for PTR at increased distances were combined. The combination of the rSUVmax for the solid component and PTR20 showed the largest AUC of 0.881, with sensitivity and specificity values of 0.906 and 0.745, respectively. The visualized curves are shown in Figure 6B.
Table 3
Parameters | AUC (95% CI) | Sensitivity (%) | Specificity (%) | Youden index |
---|---|---|---|---|
rSUVmax | ||||
Solid | 0.865 (0.803–0.927) | 71.9 | 85.5 | 0.573 |
PTRs+5 | 0.860 (0.793–0.927) | 67.2 | 92.7 | 0.599 |
PTRs+10 | 0.864 (0.798–0.929) | 81.3 | 80.0 | 0.613 |
PTRs+15 | 0.868 (0.802–0.934) | 82.8 | 83.6 | 0.664 |
PTRs+20 | 0.881 (0.819–0.943) | 90.6 | 74.5 | 0.652 |
rADCmin | ||||
Solid | 0.839 (0.768–0.910) | 72.7 | 84.6 | 0.573 |
PTRs+5 | 0.839 (0.768–0.911) | 72.7 | 84.6 | 0.573 |
PTRs+10 | 0.847 (0.775–0.918) | 72.7 | 96.2 | 0.589 |
PTRs+15 | 0.846 (0.775–0.918) | 72.1 | 86.2 | 0.589 |
PTRs+20 | 0.847 (0.776–0.919) | 72.3 | 84.6 | 0.573 |
rCBF | ||||
Solid | 0.763 (0.680–0.846) | 76.9 | 61.8 | 0.387 |
PTRs+5 | 0.782 (0.702–0.861) | 56.9 | 85.5 | 0.424 |
PTRs+10 | 0.783 (0.703–0.862) | 60.0 | 87.3 | 0.473 |
PTRs+15 | 0.792 (0.714–0.870) | 60.0 | 87.3 | 0.473 |
PTRs+20 | 0.813 (0.739–0.888) | 73.8 | 78.2 | 0.529 |
AUC, area under the curve; CI, confidence interval; rSUVmax, relative maximum standardized uptake value; PTRs+5, the solid component and the 5-mm PTR; PTRs+10, the solid component and the 10-mm PTR; PTRs+15, the solid component and the 15-mm PTR; PTRs+20, the solid component and the 20-mm PTR; PTR, peritumoral zone; rCBF, relative cerebral blood flow; rADCmin, relative minimum apparent diffusion coefficient.
As shown in Table 4, when any 2 parameters were combined across DWI, ASL, and 18F FDG-PET for the center of the solid component and PTR5, the combination of rSUVmax with rADCmin or rCBF showed equally excellent diagnostic efficacy, with an AUC of 0.874. However, the 3-way combination of parameters from the solid component and PTR5 had lower AUC values than did the multiparametric combinations for the center only. These visualized curves are shown in Figure 6C.
Table 4
Parameters | AUC (95% CI) | Sensitivity (%) | Specificity (%) | Youden index |
---|---|---|---|---|
Solid | ||||
SUVmax + ADCmin | 0.874 (0.815–0.933) | 60.0 | 100 | 0.600 |
SUVmax + rCBF | 0.874 (0.814–0.934) | 73.8 | 94.5 | 0.684 |
ADCmin + rCBF | 0.860 (0.796–0.925) | 73.8 | 87.3 | 0.611 |
Solid + PTR5 | ||||
SUVmax + ADCmin | 0.788 (0.705–0.871) | 66.2 | 85.5 | 0.516 |
SUVmax + rCBF | 0.791 (0.710–0.872) | 64.6 | 87.3 | 0.519 |
ADCmin + rCBF | 0.693 (0.599–0.788) | 58.5 | 76.4 | 0.348 |
SUVmax + ADCmin + rCBF | ||||
Solid | 0.883 (0.825–0.941) | 68.8 | 10.0 | 0.688 |
PTRs+5 | 0.897 (0.844–0.950) | 71.9 | 94.5 | 0.664 |
PTRs+10 | 0.905 (0.853–0.956) | 73.4 | 94.5 | 0.680 |
PTRs+15 | 0.912 (0.862–0.962) | 87.5 | 71.8 | 0.693 |
PTRs+20 | 0.928 (0.884–0.972) | 75.0 | 94.5 | 0.695 |
AUC, area under the curve; CI, confidence interval; PTR, peritumoral region; SUVmax, maximum standardized uptake value; ADCmin, minimum apparent diffusion coefficient; rCBF, relative cerebral blood flow; PTR5, the 5-mm PTR; PTRs+5, the solid component and the 5-mm PTR; PTRs+10, the solid component and the 10-mm PTR; PTRs+15, the solid component and the 15-mm PTR; PTRs+20, the solid component and the 20-mm PTR.
Additionally, when combining all the 3 functional parameters and integrating the parameters from the solid component and PTR at different distances, the discrimination efficacy gradually increased with increasing distance of PTR. The combination of rSUVmax, rADCmin, and rCBF from the solid component and PTR20 had the largest AUC of 0.928, with corresponding sensitivity and specificity were 94.5% and 69.5%, respectively.
Predictive nomogram for the probability of HGG and its performance
According to the diagnostic performance of the combined multi-parameters from different regions, an individualized nomogram prediction model was constructed to predict HGGs (Figure 7A). The total score was calculated, including the rSUVmax, rADCmin, and rCBF for the solid component and PTR5, and the value of each variable was assigned a score on the point-scale axis. A total score was calculated by adding each single score. By projecting the total score onto the individual variable axes of the nomogram, we were able to estimate the probability of HGG. The nomogram showed good accuracy, with a C-index of 0.906 [95% confidence interval (CI): 0.849–0.963]. Figure 7B displays the calibration curve of the nomogram, which showed that the HGG probabilities predicted by the nomogram agreed well with the actual probabilities.
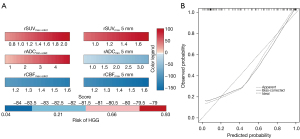
Discussion
Differentiating LGGs from HGGs can be challenging but essential for making optimal treatment decisions. Recent studies found that glioma cells can infiltrate the apparently normal region covering 20 mm around the tumor border as visualized on conventional enhanced MRI. We proposed to use multiple parameters from solid component and PTRs from hybrid 18F-FDG PET/MRI to grade glioma. HGGs showed higher rSUVmax, lower rADCmin, and higher rCBF value relative to LGGs, with significant intergroup differences in rADCmin and rCBF values for the solid component and 5–10 mm-adjacent region. Regardless of single or combined parameters, only those including rSUVmax achieved optimal discrimination performance. When properties of the solid component and PTR at different distances were integrated, the diagnostic efficacy improved with increasing distance of PTR. A nomogram prediction model incorporating 3 parameters from the solid component and PTR5 predicted HGGs with optimal discrimination and excellent calibration. This finding is valuable in clinical practice by permitting patients with glioma to be stratified before surgery.
PET provides information on the tumor metabolism and proliferation in a noninvasive, dynamic, qualitative, and quantitative manner. 18F-FDG remains the most widely used radiotracer to evaluate brain tumors, and its uptake indirectly reflects cell density and tumor aggressiveness (31). In the current study, HGGs showed higher rSUVmax than did LGGs at any measured point including the solid and PTR; especially in the solid component, the AUC was about 0.803–0.927 in differentiating LGGs from HGGs. This may reflect the high glucose avidity in malignant brain tumors, as suggested in previous reports (18,31,32), 18F-FDG-PET is a reliable tool to reflect this difference. However, these previous studies were mainly performed by combining the MRI and 18F-FDG-PET parameters, rather than hybrid 18F-FDG-PET/MRI-derived parameters. Additionally, we applied a relative SUVmax, which may overcome the influence from the background uptake (33) and alleviate some overlap in 18F-FDG uptake to a certain extent. The diagnostic performance in our study was slightly better than that in a previous report (34) in which O-(2-[18F]-fluoroethyl)-L-tyrosine (18F-FET) PET showed an AUC of 0.80–0.83 in differentiating LGGs from HGGs, which may also be ascribed to the relative value applied in this study.
Of note, the HGG and LGG groups differed in the solid component and the 5-mm- and 10-mm-adjacent PTR, suggesting that the greatest metabolic discrepancy between glioma exists mainly in and close to the solid component, where tumor cells proliferate much more actively. This was in line with the theoretical tumor region mode that with the increasing of the distance adjacent to the PTR, the tumor cell density decreased (35). Although there was no between-group difference, rSUVmax in the 15–20-mm-adjacent PTR (Table S2 and Figure 4A) exhibited some alterations, indicating occult metabolic abnormality in the PTR that was considered normative in past decades. Therefore, PTR is an important area that should not be undervalued in the evaluation of gliomas. Importantly, compared to relying on a single parameter from any imaging technique or any tumor region, incorporating rSUVmax with other MRI (DWI or ASL) parameters enhances grading performance. Thus, rSUVmax plays a significant role in differentiation of glioma grades. This research agrees with a previous review reporting that 18F-FDG PET can provide additional information that complements MRI data, thus easing glioma management (18). In this context, we consider that 18F-FDG-PET/MRI can be a vital step in the clinical workflow to distinguish HGGs from LGGs before surgery.
ASL provides microcirculation information by measuring blood flow using magnetically labeled water protons in the arterial blood as an endogenous tracer. rCBF derived from ASL has been viewed as an effective marker for discriminating LGGs from HGGs (36,37); however, it has not been used to assess PTR for glioma grading. In our study, a puzzling result was that rCBF values were higher for HGGs than for LGGs even at areas located 20 mm away from the tumor margin. However, this is not sufficient to distinguish the 2 grades. We suggest that there is aberrant vascularization in a wide area of PTR in both HGGs and LGGs. This could be attributed to the tumorigenic and angiogenic properties of PTR, which promote the growth and invasion of gliomas (38). In a pilot study, it may be worth assessing the merits of rCBF values at 15–20 mm from the conventionally defined tumor margins as a sensitivity marker for PTR. DWI-generated ADC values can quantitatively evaluate tissue cellularity, which is useful because proliferating tumor cells hamper extracellular water diffusion in vivo (39). Nevertheless, the grading performance of PTR based on ADC has not been scrutinized. Our findings revealed significantly decreased rADCmin values for HGGs, consistent with the results of previous studies (40). Similar to the findings in a previous report (26), we found an increase in the rADCmin in PTR, and, with increasing distance of PTR, the rADCmin values also decreased; however, the properties of PTR have not been studied in detail.
Multiparametric imaging is a promising tool for distinguishing tumor characteristics. 18F-FDG PET/MRI not only provides glucose metabolism information via 18F-FDG PET imaging but also enables the simultaneous acquisition of multiple quantitative MRI during PET imaging, providing a more comprehensive assessment of the glioma grading. Our study verified that a combination of multiple parameters derived from 18F FDG-PET/MRI (rSUVmax, rADCmin, or rCBF) yielded better results than did use of a single parameter or incorporation of any 2 parameters alone. In particular, those including rSUVmax achieved optimal discrimination performance; our results further supported the recommendation of the Neuro-Oncology working group for the clinical use of PET imaging in glioma (32). These findings suggest that integrated PET/MRI may be particularly useful for grading gliomas, providing comprehensive morphological and metabolic information in a single examination. Furthermore, a single 18F-FDG PET/MRI examination was less influenced by the unaligned images from multimodality images, providing a robust result (41).
Interestingly, when the 3 18FDG-PET/MRI-based parameters were combined for distinct tumor regions, the combination of parameters derived from the solid component of the tumor and PTR20 displayed the best performance in differentiating HGGs form LGGs, with an AUC of 0.928 (95% CI: 0.884–0.972). Pallud et al. (42) collected biopsy samples within and beyond tumor margins, finding a significant density of tumor cells at distances of 10–20 mm beyond the conventional imaging-defined limits. Similarly, Gerin et al. (43) discovered tumor cell infiltration in up to 20 mm of parenchyma around conventional MRI-defined abnormalities. However, the aforementioned MRI acquisition protocols were mostly performed using conventional MRI sequences such as T2WI. Zhang et al. (28) reported that a multiparametric measurement of the enhancing tumors and PTR diagnostic model based on 18F-FDG PET/MRI is superior to a single parameter method in differentiating of HGG from the primary central nervous system lymphoma. Thus, our nomogram may be an important predictive tool to determine patient-specific probabilities of HGGs with optimal discrimination, with a C-index of 0.906 (95% CI: 0.849–0.963).
Our study had several limitations. First, this was a single-center, retrospective study with a small sample size. We could not separate subgroups, such as according to IDH mutation and other characteristics, as this would have reduced statistical power. Thus, the results may have been statistically biased. A large cohort from multiple centers is required for validation. Second, this retrospective study was conducted from 2019. As a result, the pathological results of the included samples were based on the 2016 CNS WHO classification (4th edition) instead of the latest edition (5th edition) released in 2021. Third, multiparametric measures for PTR do not provide direct evidence regarding the histopathology of the tumor tissue. This is also a clinical challenge that may be overcome to some degree by conducting animal studies. Fourth, due to ethical constraints by the Internal Ethics Committee of the Hospital, we could not perform both FDG and FET-PET for evaluation, although some reports have mentioned that the 18F-FET possessed some advantages over 18F-FDG-PET. Meanwhile, a systematic review and meta-analysis (22) found that both tracers were able to distinguish between LGGs and HGGs. Furthermore, we used the relative value of the SUVmax, which may alleviate the disadvantage of the 18F-FDG to some degree.
Conclusions
The combination of rSUVmax, rCBF, and rADCmin derived from hybrid PET/MRI of the solid component and PTR facilitated more accurate discrimination of LGGs from HGGs than did a single parameter alone. 18F-FDG PET/MRI has particular value in estimating the glioma histologic grade, which is a potential tool to guide the initiation of proper treatment and improve patients’ outcomes. Thus, multiparametric 18F-FDG PET/MRI can be a vital step in the clinical workflow to distinguish HGGs from LGGs before surgery. Moreover, the cellular and metabolic features of PTR in both HGGs and LGGs are crucial for identifying new therapeutic targets (25).
Acknowledgments
Part of the results in this article was submitted to 2023 RSNA and accepted as oral presentation (abstract #14105).
Funding: This work was supported by
Footnote
Reporting Checklist: The authors have completed the TRIPOD reporting checklist. Available at https://qims.amegroups.com/article/view/10.21037/qims-24-280/rc
Conflicts of Interest: All authors have completed the ICMJE uniform disclosure form (available at https://qims.amegroups.com/article/view/10.21037/qims-24-280/coif). P.L. reports a grant from the Science and Technology Planning Project of Guangzhou (No. 2023A03J0276) and the National Natural Science Foundation of China (No. 82102004) during the conduct of the study. G.H.J. reports a grant from the National Natural Science Foundation of China (No. 82271948) and the Key Laboratory Construction Project of the Guangzhou Science and Technology (No. 202201020373) during the conduct of the study. The other authors have no conflicts of interest to declare.
Ethical Statement: The authors are accountable for all aspects of the work in ensuring that questions related to the accuracy or integrity of any part of the work are appropriately investigated and resolved. The study was conducted in accordance with the Declaration of Helsinki (as revised in 2013) and approved by the Institutional Review Board of Guangzhou Universal Medical Imaging Diagnostic Center (No. 003/2019). All patients signed an informed consent form for the 18F-FDG PET/MRI examination. The data applied in this study were exempted from ethical review due to the retrospective nature.
Open Access Statement: This is an Open Access article distributed in accordance with the Creative Commons Attribution-NonCommercial-NoDerivs 4.0 International License (CC BY-NC-ND 4.0), which permits the non-commercial replication and distribution of the article with the strict proviso that no changes or edits are made and the original work is properly cited (including links to both the formal publication through the relevant DOI and the license). See: https://creativecommons.org/licenses/by-nc-nd/4.0/.
References
- He Y, Cai Y, Liu J, Ding H, Li X, Tian S, Li Z. Systematic Analysis of a Pyroptosis-Related Signature to Predict the Prognosis and Immune Microenvironment of Lower-Grade Glioma. Cells 2022;11:3980. [Crossref] [PubMed]
- Louis DN, Perry A, Wesseling P, Brat DJ, Cree IA, Figarella-Branger D, Hawkins C, Ng HK, Pfister SM, Reifenberger G, Soffietti R, von Deimling A, Ellison DW. The 2021 WHO Classification of Tumors of the Central Nervous System: a summary. Neuro Oncol 2021;23:1231-51. [Crossref] [PubMed]
- McNamara C, Mankad K, Thust S, Dixon L, Limback-Stanic C, D'Arco F, Jacques TS, Löbel U. 2021 WHO classification of tumours of the central nervous system: a review for the neuroradiologist. Neuroradiology 2022;64:1919-50. [Crossref] [PubMed]
- Ostrom QT, Cioffi G, Gittleman H, Patil N, Waite K, Kruchko C, Barnholtz-Sloan JS. CBTRUS Statistical Report: Primary Brain and Other Central Nervous System Tumors Diagnosed in the United States in 2012-2016. Neuro Oncol 2019;21:v1-v100. [Crossref] [PubMed]
- Gu W, Fang S, Hou X, Ma D, Li S. Exploring diagnostic performance of T2 mapping in diffuse glioma grading. Quant Imaging Med Surg 2021;11:2943-54. [Crossref] [PubMed]
- Du N, Shu W, Li K, Deng Y, Xu X, Ye Y, Tang F, Mao R, Lin G, Li S, Fang X. An initial study on the predictive value using multiple MRI characteristics for Ki-67 labeling index in glioma. J Transl Med 2023;21:119. [Crossref] [PubMed]
- Martucci M, Russo R, Schimperna F, D'Apolito G, Panfili M, Grimaldi A, Perna A, Ferranti AM, Varcasia G, Giordano C, Gaudino S. Magnetic Resonance Imaging of Primary Adult Brain Tumors: State of the Art and Future Perspectives. Biomedicines 2023;11:364. [Crossref] [PubMed]
- Guida L, Stumpo V, Bellomo J, van Niftrik CHB, Sebök M, Berhouma M, Bink A, Weller M, Kulcsar Z, Regli L, Fierstra J. Hemodynamic Imaging in Cerebral Diffuse Glioma-Part A: Concept, Differential Diagnosis and Tumor Grading. Cancers (Basel) 2022;14:1432. [Crossref] [PubMed]
- Lindner T, Bolar DS, Achten E, Barkhof F, Bastos-Leite AJ, Detre JA, et al. Current state and guidance on arterial spin labeling perfusion MRI in clinical neuroimaging. Magn Reson Med 2023;89:2024-47. [Crossref] [PubMed]
- Alkanhal H, Das K, Poptani H. Diffusion- and Perfusion-Weighted Magnetic Resonance Imaging Methods in Nonenhancing Gliomas. World Neurosurg 2020;141:123-30. [Crossref] [PubMed]
- Hales PW, d'Arco F, Cooper J, Pfeuffer J, Hargrave D, Mankad K, Clark C. Arterial spin labelling and diffusion-weighted imaging in paediatric brain tumours. Neuroimage Clin 2019;22:101696. [Crossref] [PubMed]
- Hashido T, Saito S, Ishida T. Radiomics-Based Machine Learning Classification for Glioma Grading Using Diffusion- and Perfusion-Weighted Magnetic Resonance Imaging. J Comput Assist Tomogr 2021;45:606-13. [Crossref] [PubMed]
- Roach JR, Plaha P, McGowan DR, Higgins GS. The role of [18F]fluorodopa positron emission tomography in grading of gliomas. J Neurooncol 2022;160:577-89.
- Zhang-Yin JT, Girard A, Bertaux M. What Does PET Imaging Bring to Neuro-Oncology in 2022? A Review. Cancers (Basel) 2022;14:879. [Crossref] [PubMed]
- Çelebi F, Yaghouti K, Cindil E, Dogusoy GB, Tokat Y, Balcı C. The Role of 18F-FDG PET/MRI in the Assessment of Primary Intrahepatic Neoplasms. Acad Radiol 2021;28:189-98. [Crossref] [PubMed]
- Almansory KO, Fraioli F. Combined PET/MRI in brain glioma imaging. Br J Hosp Med (Lond) 2019;80:380-6. [Crossref] [PubMed]
- Overcast WB, Davis KM, Ho CY, Hutchins GD, Green MA, Graner BD, Veronesi MC. Advanced imaging techniques for neuro-oncologic tumor diagnosis, with an emphasis on PET-MRI imaging of malignant brain tumors. Curr Oncol Rep 2021;23:34. [Crossref] [PubMed]
- Quartuccio N, Laudicella R, Vento A, Pignata S, Mattoli MV, Filice R, Comis AD, Arnone A, Baldari S, Cabria M, Cistaro A. The Additional Value of (18)F-FDG PET and MRI in Patients with Glioma: A Review of the Literature from 2015 to 2020. Diagnostics (Basel) 2020;10:357. [Crossref] [PubMed]
- Ferda J, Ferdová E, Hes O, Mraček J, Kreuzberg B, Baxa J. PET/MRI: Multiparametric imaging of brain tumors. Eur J Radiol 2017;94:A14-25. [Crossref] [PubMed]
- Katsanos AH, Alexiou GA, Fotopoulos AD, Jabbour P, Kyritsis AP, Sioka C. Performance of 18F-FDG, 11C-Methionine, and 18F-FET PET for Glioma Grading: A Meta-analysis. Clin Nucl Med 2019;44:864-9. [Crossref] [PubMed]
- Yoon JH, Kim JH, Kang WJ, Sohn CH, Choi SH, Yun TJ, Eun Y, Song YS, Chang KH. Grading of cerebral glioma with multiparametric MR imaging and 18F-FDG-PET: concordance and accuracy. Eur Radiol 2014;24:380-9. [Crossref] [PubMed]
- Dunet V, Pomoni A, Hottinger A, Nicod-Lalonde M, Prior JO. Performance of 18F-FET versus 18F-FDG-PET for the diagnosis and grading of brain tumors: systematic review and meta-analysis. Neuro Oncol 2016;18:426-34. [Crossref] [PubMed]
- Giambra M, Messuti E, Di Cristofori A, Cavandoli C, Bruno R, Buonanno R, Marzorati M, Zambuto M, Rodriguez-Menendez V, Redaelli S, Giussani C, Bentivegna A. Characterizing the Genomic Profile in High-Grade Gliomas: From Tumor Core to Peritumoral Brain Zone, Passing through Glioma-Derived Tumorspheres. Biology (Basel) 2021;10:1157. [Crossref] [PubMed]
- Lemée JM, Clavreul A, Aubry M, Com E, de Tayrac M, Eliat PA, Henry C, Rousseau A, Mosser J, Menei P. Characterizing the peritumoral brain zone in glioblastoma: a multidisciplinary analysis. J Neurooncol 2015;122:53-61. [Crossref] [PubMed]
- Silva M, Vivancos C, Duffau H. The Concept of «Peritumoral Zone» in Diffuse Low-Grade Gliomas: Oncological and Functional Implications for a Connectome-Guided Therapeutic Attitude. Brain Sci 2022;12:504. [Crossref] [PubMed]
- Pauleit D, Langen KJ, Floeth F, Hautzel H, Riemenschneider MJ, Reifenberger G, Shah NJ, Müller HW. Can the apparent diffusion coefficient be used as a noninvasive parameter to distinguish tumor tissue from peritumoral tissue in cerebral gliomas? J Magn Reson Imaging 2004;20:758-64. [Crossref] [PubMed]
- Jensen RL, Mumert ML, Gillespie DL, Kinney AY, Schabel MC, Salzman KL. Preoperative dynamic contrast-enhanced MRI correlates with molecular markers of hypoxia and vascularity in specific areas of intratumoral microenvironment and is predictive of patient outcome. Neuro Oncol 2014;16:280-91. [Crossref] [PubMed]
- Zhang S, Wang J, Wang K, Li X, Zhao X, Chen Q, Zhang W, Ai L. Differentiation of high-grade glioma and primary central nervous system lymphoma: Multiparametric imaging of the enhancing tumor and peritumoral regions based on hybrid (18)F-FDG PET/MRI. Eur J Radiol 2022;150:110235. [Crossref] [PubMed]
- Alsaedi A, Doniselli F, Jäger HR, Panovska-Griffiths J, Rojas-Garcia A, Golay X, Bisdas S. The value of arterial spin labelling in adults glioma grading: systematic review and meta-analysis. Oncotarget 2019;10:1589-601. [Crossref] [PubMed]
- Jin C, Cao J, Cai Y, Wang L, Liu K, Shen W, Hu J. A nomogram for predicting the risk of invasive pulmonary adenocarcinoma for patients with solitary peripheral subsolid nodules. J Thorac Cardiovasc Surg 2017;153:462-469.e1. [Crossref] [PubMed]
- Song PJ, Lu QY, Li MY, Li X, Shen F. Comparison of effects of 18F-FDG PET-CT and MRI in identifying and grading gliomas. J Biol Regul Homeost Agents 2016;30:833-8.
- Albert NL, Weller M, Suchorska B, Galldiks N, Soffietti R, Kim MM, la Fougère C, Pope W, Law I, Arbizu J, Chamberlain MC, Vogelbaum M, Ellingson BM, Tonn JC. Response Assessment in Neuro-Oncology working group and European Association for Neuro-Oncology recommendations for the clinical use of PET imaging in gliomas. Neuro Oncol 2016;18:1199-208. [Crossref] [PubMed]
- Smeraldo A, Ponsiglione AM, Soricelli A, Netti PA, Torino E. Update on the Use of PET/MRI Contrast Agents and Tracers in Brain Oncology: A Systematic Review. Int J Nanomedicine 2022;17:3343-59. [Crossref] [PubMed]
- Verger A, Filss CP, Lohmann P, Stoffels G, Sabel M, Wittsack HJ, Kops ER, Galldiks N, Fink GR, Shah NJ, Langen KJ. Comparison of (18)F-FET PET and perfusion-weighted MRI for glioma grading: a hybrid PET/MR study. Eur J Nucl Med Mol Imaging 2017;44:2257-65. [Crossref] [PubMed]
- Eyüpoglu IY, Buchfelder M, Savaskan NE. Surgical resection of malignant gliomas-role in optimizing patient outcome. Nat Rev Neurol 2013;9:141-51. [Crossref] [PubMed]
- Ningning D, Haopeng P, Xuefei D, Wenna C, Yan R, Jingsong W, Chengjun Y, Zhenwei Y, Xiaoyuan F. Perfusion imaging of brain gliomas using arterial spin labeling: correlation with histopathological vascular density in MRI-guided biopsies. Neuroradiology 2017;59:51-9. [Crossref] [PubMed]
- White CM, Pope WB, Zaw T, Qiao J, Naeini KM, Lai A, Nghiemphu PL, Wang JJ, Cloughesy TF, Ellingson BM. Regional and voxel-wise comparisons of blood flow measurements between dynamic susceptibility contrast magnetic resonance imaging (DSC-MRI) and arterial spin labeling (ASL) in brain tumors. J Neuroimaging 2014;24:23-30. [Crossref] [PubMed]
- Lo Greco MC, Milazzotto R, Liardo RLE, Acquaviva G, La Rocca M, Altieri R, Certo F, Barbagallo GM, Basile A, Foti PV, Palmucci S, Pergolizzi S, Pontoriero A, Spatola C. Relapsing High-Grade Glioma from Peritumoral Zone: Critical Review of Radiotherapy Treatment Options. Brain Sci 2022;12:416. [Crossref] [PubMed]
- Chawla S, Krejza J, Vossough A, Zhang Y, Kapoor GS, Wang S, O'Rourke DM, Melhem ER, Poptani H. Differentiation between oligodendroglioma genotypes using dynamic susceptibility contrast perfusion-weighted imaging and proton MR spectroscopy. AJNR Am J Neuroradiol 2013;34:1542-9. [Crossref] [PubMed]
- Yang X, Xing Z, She D, Lin Y, Zhang H, Su Y, Cao D. Grading of IDH-mutant astrocytoma using diffusion, susceptibility and perfusion-weighted imaging. BMC Med Imaging 2022;22:105. [Crossref] [PubMed]
- Shang F, Tan Z, Gong T, Tang X, Sun H, Liu S. Evaluation of parametrial infiltration in patients with IB-IIB cervical cancer by a radiomics model integrating features from tumoral and peritumoral regions in (18) F-fluorodeoxy glucose positron emission tomography/MR images. NMR Biomed 2023; Epub ahead of print. [Crossref]
- Pallud J, Varlet P, Devaux B, Geha S, Badoual M, Deroulers C, Page P, Dezamis E, Daumas-Duport C, Roux FX. Diffuse low-grade oligodendrogliomas extend beyond MRI-defined abnormalities. Neurology 2010;74:1724-31. [Crossref] [PubMed]
- Gerin C, Pallud J, Deroulers C, Varlet P, Oppenheim C, Roux FX, Chrétien F, Thomas SR, Grammaticos B, Badoual M. Quantitative characterization of the imaging limits of diffuse low-grade oligodendrogliomas. Neuro Oncol 2013;15:1379-88. [Crossref] [PubMed]