Brain metastasis magnetic resonance imaging-based deep learning for predicting epidermal growth factor receptor (EGFR) mutation and subtypes in metastatic non-small cell lung cancer
Introduction
Non-small cell lung cancer (NSCLC) is the most common and lethal tumor worldwide (1), accounting for 85–90% of all lung cancer cases (2). The epidermal growth factor receptor (EGFR) gene mutation has been identified as a unique therapeutic target, with an incidence of 40–60% among patients with NSCLC (3). Tyrosine kinase inhibitors (TKIs) have been suggested as an effective therapy for patients harboring the EGFR mutation, resulting in satisfactory outcomes with increased survival rates (4-7). Therefore, early identification of the EGFR mutation is critical for appropriate decision-making regarding individualized treatment strategies (8).
EGFR mutation sites located in exons 19 and 21 are the most important subtypes, constituting approximately 90% of all EGFR mutations (9,10). Moreover, the prognostic value of mutations in EGFR exons 19 and 21 differ (11-14). Patients with the 19 or 21 EGFR mutation subtypes often show a higher radiographic response rate to EGFR-TKIs (15) and exhibit longer progression-free survival (PFS) (16,17) and overall survival (OS) times (7) than do those with EGFR mutation sites located in exons 18 or 20. Reports have shown that the median PFS after first-line EGFR-TKI treatment is significantly higher in patients with the exon 19 mutation than in those with the exon 21 mutation (16,18). Osimertinib or afatinib is recommended as the first-line treatment option for patients with the exon 19 mutation. However, dacomitinib or erlotinib in combination with bevacizumab is recommended as the first-line option for patients with the exon 21 mutation (19). Therefore, determining the EGFR mutation site is critical to guiding the selection of a treatment plan.
Many patients with NSCLC present with brain metastasis (BM) from the primary lesion at the time of diagnosis, with an incidence of 30–50% (20,21). Puncture biopsy is the standard clinical procedure for detecting EGFR mutation (22). In the absence of primary tumor, BM is considered an important alternative. However, to prevent potential damage to cranial nerves and the risk of tumor spread, invasive puncture is not clinically recommended for patients with BM. Although brain magnetic resonance imaging (MRI) is the most widely used noninvasive method for evaluating BM (23), MRI lacks specific markers that can help radiologists detect EGFR mutations via visual inspection.
Radiomics is an emerging technique that can quantitatively analyze a large number of imaging features for diagnosis in the assessment of gene mutations (24-27). Previous exploratory studies have demonstrated the value of MRI-based radiomics in determining EGFR mutation status in those with BM (23,28). However, conventional radiomics have some limitations, including the manual segmentation of the tumor and the calculation of hard-coded features (29). In addition, given the diversity in shape and size of BM, it is difficult to respond to the variable and complex textures of tumors by relying solely on traditional handcrafted features. Deep learning algorithms can automatically extract high-dimensional features from medical images and learn the most representative information from raw images; thus, they hold great promise for imaging-based diagnosis (30-35). Hu et al. proposed an effective data augmentation method that uses a generative adversarial network (GAN) to reconstruct missing positron emission tomography (PET) images (36). Additionally, Hu et al. proposed a novel end-to-end network called bidirectional GAN, in which image contexts and latent vectors are effectively used and jointly optimized for brain MR-to-PET synthesis (37). Lei et al. proposed a joint deep learning approach based on a model built using group lasso and correntropy combined with independently recurrent neural network (IndRNN) to predict the clinical scores of patients with Alzheimer disease from brain MRI data at multiple time points (38). Although several studies have shown that deep learning algorithms perform well in detecting EGFR mutation (39-45), all of these have focused on MRI of primary lung cancer and lacked the prediction of EGFR subtypes. Recent studies have predicted EGFR mutation subtypes based on distant metastases originating from primary lung cancer but have only used conventional hand-extracted features in machine learning (46-48). There are challenges in directly applying existing deep learning models to learn representative features from BM owing to its irregular shape and size in MRI. This motivated us to develop a novel deep learning method based on BM for accurately predicting EGFR mutation subtypes. We present this article in accordance with the TRIPOD reporting checklist (available at https://qims.amegroups.com/article/view/10.21037/qims-23-1744/rc).
Methods
Patients
This study was conducted in accordance with the Declaration of Helsinki (as revised in 2013) and was approved by the institutional ethics board of Liaoning Cancer Hospital and Institute (No. 20200819YG), and the requirement for individual consent in this retrospective analysis was waived. The inclusion criteria were as follows: (I) pathologically confirmed BM from primary NSCLC, (II) gene-based sequencing analysis of pathological biopsy specimens to confirm the EGFR mutation status and location of EGFR mutation sites, and (III) complete preoperative BM MRI. The exclusion criteria were as follows: (I) presence of other tumors and (II) receiving radiochemotherapy. Finally, 160 from Liaoning Cancer Hospital and Institute (center 1) and 72 patients Shengjing Hospital of China Medical University (center 2) who met the inclusion criteria were enrolled between January 2018 and December 2021. Center 2 data constituted the external validation set to validate the models. Clinical characteristics, including performance status (PS), sex, smoking status, age, serum carcinoembryonic antigen (CEA) levels, neuron-specific enolase (NSE) levels, and cytokeratin (CYFRA) levels were retrieved from the electronic medical record systems for statistical analysis. In this study, we segmented lung cancer BM and constructed a deep learning multiscale feature fusion network (MSF-Net) model to predict EGFR mutations and subtypes. Gradient-weighted class activation mapping (Grad-CAM) was used to demonstrate the areas most affected by the MSF-Net model. A detailed flowchart of this process is shown in Figure 1.
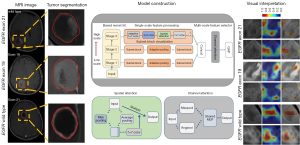
Acquisition of MRI and segmentation of the regions of interest (ROIs)
Patients from centers 1 and 2 were scanned using a MAGNETOM Trio 3.0 T MRI scanner, (Siemens Healthineers, Erlangen) or a 3.0 T MAGNETOM Skyra (Siemens Healthineers), respectively. The parameters for T1-weighted (T1w)-contrast enhanced (CE) MRI were as follows: T1-CE [time to repetition (TR) =500 ms; time to echo [(TE) =9 ms], sagittal slice thickness =4 mm, and scan interval =4.4 mm. Gadolinium-diethylenetriamine penta-acetic acid (Gd-DTPA) was used as the contrast medium for T1w-CE MRI. The parameters for T2w MRI were as follows: TR/TE =3,630/87 ms, slice thickness =5 mm, pixel spacing =0.359×0.359 mm, acquisition matrix =320×259, and field of view =576×640 mm. Brain MRI scans were saved in a Digital Imaging and Communications in Medicine (DICOM) format.
We invited a senior radiologist with 4 years of experience to manually segment the metastatic lesions in each MRI slice using ITK-Snap (v. 3.6.0), thus generating ROIs for extracting radiological features. All ROIs were validated by a senior clinician with 14 years of work experience to ensure that they were correct.
Establishment of the MSF-Net
We developed MSF-Net using residual network (ResNet) 50 (49) as the backbone network, which aggregates three types of feature information at various scales. We used the learning weights obtained from the fivefold cross-validation as our base model parameters because of the fast training speed and parameter efficiency of ResNet 50. The details of MSF-Net are as follows.
Single-scale feature refinement
MSF-Net divides feature mapping into various stages and forms a special single-scale feature processing branch for each stage. The feature processing branch is stacked with attention modules, introducing depthwise convolutions to reduce the number of network parameters. To identify the most discriminative and effective features of BM, the spatial attention module is composed of two pooling strategies (average and max pooling) (50). The operation of the spatial attention module is described in detail as follows.
, as the features, are input into the spatial attention module. The channel information of a feature map is aggregated using two pooling operations to generate two 2D maps. The two generated maps are then concatenated and applied to a standard convolution, which is followed by sigmoid activation. This process can be expressed as follows:
where σ denotes the sigmoid function and denotes a convolution operation with the filter size of 7×7. The map can be used to identify the informative regions by multiplying M and X point wise, as follows:
where P is the final refined output.
Single-scale feature fusion
Adaptive pooling is applied for downsampling to ensure that each branch generates the same feature resolution. Single-scale feature mappings are fused to form multiscale feature blocks via concatenation.
Multiscale feature selection
The channel attention module was introduced to distribute the importance of each branch so that MSF-Net can adaptively utilize multiscale information. It is composed of global average pooling (GAP), two consecutive fully connected (FC) layers followed by sigmoid activation, and a multiply layer. GAP is applied to squeeze the global spatial information and generate a channel-wise feature response. , as the fused feature maps, are input into the channel attention module. The c-th element is calculated as follows:
Subsequently, the two consecutive FC layers are used to recalibrate the vector to :
where δ denotes to the rectified linear unit (ReLU) function, and W1 and W2 denote the respective weights in the two FCs. The final output Xout of the block is obtained by rescaling with activation :
Finally, the output features are reduced to one dimension using GAP to avoid overfitting (51). The architecture of the proposed MSF-Net is shown in Figure 2.
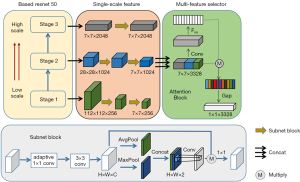
To train MSF-Net, we conducted a degree of preprocessing on the image, and each image was shifted vertically and horizontally, rotated by –30° and +30°, and then scaled and cropped. The ROI of each MRI slice was adjusted to 224×224 pixels for input into the network. During training, the Adam algorithm optimized the model with a learning rate of 0.0001. To avoid overfitting, we added batch normalization layers after the convolution layers and dropped them out to the FC layers. We chose cross-entropy as the loss function:
where denotes the prediction, and denotes the ground truth. The batch size and training epochs were 8 and 200, respectively. All networks were created and trained on a single graphics processing unit (GPU) (GeForce 3090 Ti, Nvidia Corporation, Santa Clara, CA, USA) workstation in Python v. 3.6 (Python Software Foundation, Wilmington, DE, USA).
Selection of handcrafted radiomics features and model construction
In total, 1976 radiomic candidate features were extracted from BM, and a handcrafted radiomic model was constructed using the Pyradiomics package (52). The feature types used were first-order, shape-based, and texture features. To identify the most predictive features, 30 randomly selected patients were used to evaluate the consistency of features expressed by the interclass correlation coefficient (ICC) (53). High ICC values (ICC >0.85) indicated that the results of independent evaluations by different observers were consistent. Next, the retained features were screened using the Mann-Whitney test with P<0.05, and those selected were used for the training set. Least absolute shrinkage and selection operator logistic regression (54) were applied to screen the remaining features to retain the nonzero coefficient features. Handcrafted radiomic models were constructed by incorporating the finally remaining features with logistic regression using the “rms” package in R v. 3.6 (available from URL: https://www.r-project.org; The Foundation of Statistical Computing).
Statistical analysis
Statistical analysis was performed to assess the statistical differences in the clinical characteristics between the wild-type EGFR and EGFR mutations in exon 19 and 21. The Mann-Whitney test and chi-square test were employed for continuous and discrete variables, respectively. The threshold for statistical significance was set at a two-sided P<0.05. Receiver operating characteristic (ROC) analysis was performed in R using the maximum Youden index (55) to assess the performance of the handcrafted radiomics- and deep learning-based models. Micro- and macroaveraged values were used to calculate the average performance of the ROC curves for the three EGFR mutation statuses. Precision, recall, and F1-score, together with their corresponding macro- and weighted-average values, were computed at the patient level. Grad-CAM was employed for visual interpretation of MSF-Net (56).
Results
Clinical characteristics
A total of 160 patients from center 1 were included in the training set. The number of patients with wild-type EGFR, exon 19 mutation, and exon 21 mutation was 76, 41, and 43, respectively. A total of 72 patients from center 2 were used as the external validation set, and the number of patients with wild-type EGFR, exon 19 mutation, and exon 21 mutation was 30, 22, and 20, respectively. Table 1 shows the clinical characteristics of the patients.
Table 1
Characteristic | Training set (center 1) | External validation set (center 2) | |||||||
---|---|---|---|---|---|---|---|---|---|
Wild-type (n=76) | Exon 19 (n=41) | Exon 21 (n=43) | P | Wild-type (n=30) | Exon 19 (n=22) | Exon 21 (n=20) | P | ||
Age (years), mean ± SD | 60.39±7.64 | 55.51±8.68 | 63.27±10.11 | 0.001* | 60.18±7.54 | 60.34±6.57 | 59.78±8.27 | 0.628 | |
Gender, n (%) | 0.032* | 0.005* | |||||||
Male | 36 (47.37) | 20 (48.78) | 22 (51.16) | 14 (46.67) | 10 (45.45) | 10 (50.00) | |||
Female | 40 (52.63) | 21(51.22) | 21 (48.84) | 16 (53.33) | 12 (54.55) | 10 (50.00) | |||
Smoking, n (%) | 0.018* | 0.023* | |||||||
Yes | 42 (55.26) | 24 (58.54) | 19 (44.19) | 12(40.00) | 9 (40.91) | 9 (45.00) | |||
No | 34 (44.74) | 17 (41.46) | 24 (55.81) | 18(60.00) | 13 (59.09) | 11(55.00) | |||
PS score, n (%) | 0.004* | 0.185 | |||||||
0 | 13 (17.11) | 5 (12.20) | 6 (13.95) | 5 (16.67) | 3 (13.64) | 2 (10.00) | |||
1 | 58 (76.32) | 36 (87.80) | 34 (79.07) | 21 (70.00) | 18 (81.82) | 16 (80.00) | |||
2 | 5 (6.57) | 0 (0.00) | 3 (6.98) | 4 (13.33) | 1 (4.54) | 2 (10.00) | |||
KPS score, n (%) | 0.051 | 0.324 | |||||||
70 | 8 (10.53) | 6 (14.63) | 7 (16.28) | 2 (6.67) | 2 (9.09) | 1 ((5.00) | |||
80 | 17 (22.37) | 16 (39.03) | 15 (34.88) | 10 (33.33) | 6 (27.27) | 7 (35.00) | |||
90 | 51 (67.10) | 19 (46.34) | 21 (48.84) | 18 (60.00) | 14 (63.64) | 12 (60.00) | |||
CEA, mean ± SD | 138.24±367.22 | 97.23±267.22 | 88.65±198.24 | 0.167 | 151.89±211.90 | 99.28±131.19 | 87.27±99.31 | 0.213 | |
CYFRA, mean ± SD | 10.28±19.32 | 8.89±9.83 | 9.35±17.29 | 0.711 | 10.36±9.84 | 17.21±10.01 | 15.11±6.79 | 0.674 | |
NSE, mean ± SD | 29.26±29.84 | 30.28±16.76 | 26.73±18.99 | 0.384 | 24.58±6.22 | 22.89±5.88 | 20.16±12.63 | 0.380 |
*, P<0.05. Center 1, Liaoning Provincial Cancer Hospital; center 2, Shengjing Hospital of China Medical University. SD, standard deviation; PS, performance status; KPS, Karnofsky Performance Scale; CEA, carcinoembryonic antigen; CYFRA, cytokeratin; NSE, neuron-specific enolase.
Establishment and evaluation of MSF-Net
Figure 3 shows the loss curves for training and testing, in which the model rapidly converges. As shown in Figure 4, in the training set enrolled from center 1, our MSF-Net generated micro- and macroaveraged areas under the curve (AUCs) of 0.90 and 0.91, respectively. MSF-Net performed well in predicting EGFR mutations and generated the highest AUC (0.92). MSF-Net generated the lowest AUC (0.90) for predicting the EGFR mutation in exon 21. At the patient level, our MSF-Net generated micro- and macroaveraged AUCs of 0.93 and 0.94, respectively. External validation results (Figure 5) showed slight decreases in the diagnostic performance of MSF-Net with AUCs of 0.91, 0.91, and 0.89 for predicting EGFR mutations, exon 19 mutation, and exon 21 mutation, respectively. The micro- and macroaveraged AUCs in the external set were 0.90 and 0.91, respectively.
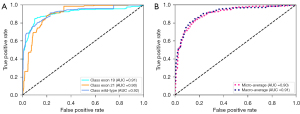
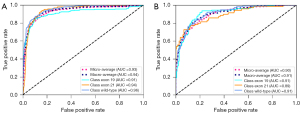
Figure 6 shows an example of the visual interpretation of MSF-Net, indicating the most affected regions. The first row shows the BM MRI, the second row of the heatmap shows the attention region, and the third row shows the ROI. The rightmost color bar shows the focus of MSF-Net with a cutoff value set to 0.5 to reflect the ROI obtained from the heatmap. For each class of the EGFR-mutant subtype, the tumor regions were localized to some extent, suggesting that the tumor regions provided important information for the classification task performed using MSF-Net.
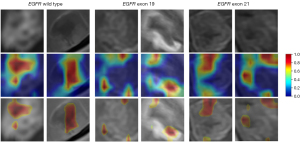
Ablation study
The novelty of our proposed MSF-Net is the multiscale feature fusion and the incorporation of spatial attention and channel attention mechanisms. Ablation experiments were conducted to evaluate the effect of multidimensional features and spatial attention mechanisms on the prediction of EGFR mutations and subtypes. As shown in Table 2, MSF-Net yielded the highest AUC and accuracy values. The ResNet 50 model generated the lowest AUC, accuracy, specificity, and sensitivity among all models. The ablation analysis indicated that each module of MSF-Net contributed to improving the prediction of EGFR mutation and subtypes.
Table 2
Task | Model | AUC | ACC | SPE | SEN |
---|---|---|---|---|---|
EGFR wild-type vs. EGFR exon 19 vs. EGFR exon 21 |
Resnet 50 | 0.72 | 0.69 | 0.79 | 0.76 |
Resnet 50 + SA+ CA | 0.78 | 0.70 | 0.81 | 0.84 | |
Resnet 50 + MF | 0.82 | 0.72 | 0.82 | 0.86 | |
MSF-Net | 0.91 | 0.75 | 0.87 | 0.90 |
ResNet, residual network; AUC, area under the curve; ACC, accuracy; SPE, specificity; SEN, sensitivity; EGFR, epidermal growth factor receptor; SA, spatial attention mechanism; CA, channel attention mechanism; MF, multiscale feature; MSF-Net, multiscale feature fusion network.
Comparison between MSF-Net and handcrafted radiomics
For comparison with the proposed MSF-Net, a handcrafted radiomics model was established based on logistic regression. As shown in Table 3, MSF-Net consistently outperformed the radiomics model in terms of precision, recall, F1-score, AUC, and accuracy for both the primary and external sets. In addition, MSF-Net exhibited a superior performance in dealing with multiclassification problems compared to the manually extracted radiomics features.
Table 3
Performance evaluation | Training set | External validation set | |||
---|---|---|---|---|---|
MSF-Net | Radiomics | MSF-Net | Radiomics | ||
Precision | |||||
Macro-average | 0.81 | 0.72 | 0.78 | 0.61 | |
Weighted-average | 0.82 | 0.78 | 0.77 | 0.60 | |
Recall | |||||
Macro-average | 0.79 | 0.69 | 0.73 | 0.62 | |
Weighted-average | 0.81 | 0.73 | 0.74 | 0.60 | |
F1-score | |||||
Macro-average | 0.79 | 0.71 | 0.74 | 0.61 | |
Weighted-average | 0.81 | 0.72 | 0.74 | 0.60 | |
AUC | |||||
Macro-average | 0.93 | 0.82 | 0.91 | 0.81 | |
Micro-average | 0.94 | 0.83 | 0.9 | 0.80 | |
Accuracy | 0.81 | 0.72 | 0.75 | 0.60 |
MSF-Net, multiscale feature fusion network; AUC, area under the curve.
Discussion
Previous research has attempted to predict the EGFR mutation status in patients with lung cancer using deep learning (57-62). However, these studies were based on the chest computed tomography (CT) data of primary lesions. Shi et al. used a deep learning network to predict metastatic origins and EGFR or human epidermal growth factor receptor 2 (HER2) status in patients with BM (63). Haim et al. used a deep learning network to predict EGFR mutation status in lung cancer BM (64). However, these studies only used traditional deep learning networks, did not improve the model, and only predicted the EGFR mutation status but not the EGFR mutation subtypes. In addition, the amount of data in these studies was small, with only 60 and 59 patients included in the studies by Shi et al. and Haim et al., respectively. Jiang et al. proposed a new neural network for predicting EGFR mutation status and mutation subtypes in BM from lung cancer, with an AUC of 0.69 (65). To our knowledge, this is the first proposal of a deep learning method that can perform multiclassification predictions of EGFR mutations and subtypes based on BM. The proposed MSF-Net involves the adaptive integration of multiscale features of different stages of ResNet 50. MSF-Net is divided into three stages, and the introduced spatial attention module in each stage can learn the feature maps specific to each scale to adapt to irregular brain MRI. Subsequently, the shallow and deep branch features are integrated to enable the model to learn more abundant and significant information for classification instead of relying on the previous layer of features (66). The fusion of multiscale features improved AUCs of the model for predicting the EGFR mutation and subtypes from 0.72 to 0.82, which indicates that the fusion of shallow and deep features can improve the predictive capability of the model.
BM is usually irregular in size and has a complex environment containing edematous and necrotic regions (23), which may distract the attention of the network. To solve this problem, we integrated spatial attention and channel attention modules (67) into MSF-Net. The spatial attention module aims to enhance the feature representation of key regions so that important regions containing rich information regarding EGFR mutations can be fully explored and irrelevant background regions weakened (68). In addition, relying only on fused features to predict the classification may result in a model that ignores more significant features at different scales and introduces more noise. Therefore, we attempted to input the features fused by each branch of the model into the channel attention module, which can adaptively learn the channel weights of these feature maps so that the model can recognize effective feature information at each stage (69). Our experimental results showed an improvement in AUC from 0.72 to 0.78 for predicting EGFR mutations and subtypes when our model was added to the attention module. The predictive power of MSF-Net improved substantially when we incorporated multiscale features and attention modules, with the AUC improving from 0.72 to 0.91.
In this study, we developed a traditional handcrafted radiomics model to predict EGFR mutation subtypes and generated an AUC and accuracy of 0.80 and 0.60, respectively. In comparison, our MSF-Net model achieved a significantly higher AUC and accuracy of 0.94 and 0.81 in the training set and 0.90 and 0.75 in the external validation set, respectively. This discrepancy may be due to the fact that the features extracted using radiomics are low-dimensional features, such as shape and texture. These features contain limited information and are not sufficiently effective for multiclassification tasks; the model built using these features has poor generalization ability, making their use problematic for multicenter datasets (70). Our MSF-Net model showed a slightly reduced performance in predicting EGFR mutation subtypes. This is consistent with previous handcrafted feature-based radiomics studies based on the BM of primary NSCLC, which also reported that the predictive ability of exons 19 and 21 of EGFR was significantly lower than that of EGFR mutations (28,65). It is possible that differences between EGFR subtype mutations are caused by changes in metastases at the molecular level, and such subtle differences are difficult to discern via MRI. In contrast, the differences between EGFR mutations and wild-type EGFR are mainly reflected in histomorphological changes, which may directly lead to the heterogeneity of metastases and significant changes in MRI. Our MSF-Net was externally evaluated, generated good performance in the detailed differentiation of EGFR mutations, and provided a basis for the establishment of the optimal personalized diagnosis and treatment strategies in patients with BM.
We applied Grad-CAM for visual interpretation of our MSF-Net. Grad-CAM can analyze and locate the areas of concern of the network for a given category (71). Our results showed that MSF-Net can provide distinct attention to various regions in the BM that are highly associated with the EGFR mutation status and sites. For the prediction of the EGFR wild type, intratumoral areas of metastases are considered important. A possible reason for this is that EGFR mutations can cause changes in tissue morphology that result in the heterogeneity of intratumoral areas (65). For EGFR mutations in exons 19 and 21, irregular tumor contours were considered as the high-response target areas. Therefore, MSF-Net can infer significant areas related to EGFR mutations and mutation subtypes to assist in clinical diagnosis.
This study involved certain limitations that should be mentioned. First, we used a 2D MRI slice as the input for our MSF-Net model because of the limited sample size, which might have led to the loss of spatial information. In future studies, adjacent tumor slices should be used as 3D inputs to increase the spatial feature information of the tumor. Second, MSF-Net was only used with T1w-CE and T2W MRI and lacked the inclusion of T2 fluid-attenuated inversion recovery and diffusion-weighted imaging sequences, which will be included in our future work. Third, this study only predicted the EGFR gene mutations; in the future, we will incorporate more data to train the models [such as Kirsten rat sarcoma viral oncogene homologue (KRAS), anaplastic lymphoma kinase (ALK), ROS proto-oncogene 1, receptor tyrosine kinase (ROS1), and T790M]. Finally, automatic detection and segmentation of BM will be addressed in our future work.
Conclusions
We propose an effective MSF-Net for predicting EGFR mutations and subtypes in BM originating from primary NSCLC. In this model, low- and high-dimensional features are fused to improve the classification accuracy. MSF-Net is an end-to-end model that can be used as a noninvasive tool to identify the EGFR mutation status and subtypes, thus aiding clinicians in developing personalized treatment plans.
Acknowledgments
Funding: This study was funded by
Footnote
Reporting Checklist: The authors have completed the TRIPOD reporting checklist. Available at https://qims.amegroups.com/article/view/10.21037/qims-23-1744/rc
Conflicts of Interest: All authors have completed the ICMJE uniform disclosure form (available at https://qims.amegroups.com/article/view/10.21037/qims-23-1744/coif). The authors have no conflicts of interest to declare.
Ethical Statement: The authors are accountable for all aspects of the work in ensuring that questions related to the accuracy or integrity of any part of the work are appropriately investigated and resolved. This study was conducted in accordance with the Declaration of Helsinki (as revised in 2013) and was approved by institutional ethics board of Liaoning Cancer Hospital and Institute (No. 20200819YG). The requirement for individual consent in this retrospective analysis was waived.
Open Access Statement: This is an Open Access article distributed in accordance with the Creative Commons Attribution-NonCommercial-NoDerivs 4.0 International License (CC BY-NC-ND 4.0), which permits the non-commercial replication and distribution of the article with the strict proviso that no changes or edits are made and the original work is properly cited (including links to both the formal publication through the relevant DOI and the license). See: https://creativecommons.org/licenses/by-nc-nd/4.0/.
References
- Siegel RL, Miller KD, Jemal A. Cancer statistics, 2019. CA Cancer J Clin 2019;69:7-34. [Crossref] [PubMed]
- Sung H, Ferlay J, Siegel RL, Laversanne M, Soerjomataram I, Jemal A, Bray F. Global Cancer Statistics 2020: GLOBOCAN Estimates of Incidence and Mortality Worldwide for 36 Cancers in 185 Countries. CA Cancer J Clin 2021;71:209-49. [Crossref] [PubMed]
- Vaid AK, Gupta A, Momi G. Overall survival in stage IV EGFR mutation‑positive NSCLC: Comparing first‑, second‑ and third‑generation EGFR‑TKIs Int J Oncol 2021;58:171-84. (Review). [Crossref] [PubMed]
- Yang JJ, Zhou C, Huang Y, Feng J, Lu S, Song Y, Huang C, Wu G, Zhang L, Cheng Y, Hu C, Chen G, Zhang L, Liu X, Yan HH, Tan FL, Zhong W, Wu YL. Icotinib versus whole-brain irradiation in patients with EGFR-mutant non-small-cell lung cancer and multiple brain metastases (BRAIN): a multicentre, phase 3, open-label, parallel, randomised controlled trial. Lancet Respir Med 2017;5:707-16. [Crossref] [PubMed]
- Sun L, Guo YJ, Song J, Wang YR, Zhang SL, Huang LT, Zhao JZ, Jing W, Han CB, Ma JT. Neoadjuvant EGFR-TKI Therapy for EGFR-Mutant NSCLC: A Systematic Review and Pooled Analysis of Five Prospective Clinical Trials. Front Oncol 2021;10:586596. [Crossref] [PubMed]
- Ramalingam SS, Vansteenkiste J, Planchard D, Cho BC, Gray JE, Ohe Y, et al. Overall survival with osimertinib in un-treated, EGFR- mutated advanced NSCLC. N Engl J Med 2020;382:41-50. [Crossref] [PubMed]
- Zheng H, Zhang Y, Zhan Y, Liu S, Lu J, Feng J, Wu X, Wen Q, Fan S. Prognostic analysis of patients with mutant and wild-type EGFR gene lung adenocarcinoma. Cancer Manag Res 2019;11:6139-50. [Crossref] [PubMed]
- Kim HS, Yoon YC, Kwon S, Lee JH, Ahn S, Ahn HS. Dynamic Contrast-enhanced MR Imaging Parameters in Bone Metastases from Non-Small Cell Lung Cancer: Comparison between Lesions with and Lesions without Epidermal Growth Factor Receptor Mutation in Primary Lung Cancer. Radiology 2017;284:815-23. [Crossref] [PubMed]
- Chan SK, Gullick WJ, Hill ME. Mutations of the epidermal growth factor receptor in non-small cell lung cancer -- search and destroy. Eur J Cancer 2006;42:17-23. [Crossref] [PubMed]
- Shi Y, Li J, Zhang S, Wang M, Yang S, Li N, Wu G, Liu W, Liao G, Cai K, Chen L, Zheng M, Yu P, Wang X, Liu Y, Guo Q, Nie L, Liu J, Han X. Molecular Epidemiology of EGFR Mutations in Asian Patients with Advanced Non-Small-Cell Lung Cancer of Adenocarcinoma Histology - Mainland China Subset Analysis of the PIONEER study. PLoS One 2015;10:e0143515. [Crossref] [PubMed]
- Zhang Y, Sheng J, Kang S, Fang W, Yan Y, Hu Z, Hong S, Wu X, Qin T, Liang W, Zhang L. Patients with exon 19 deletion were associated with longer progression-free survival compared to those with L858R mutation after first-line EGFR-TKIs for advanced non-small cell lung cancer: a meta-analysis. PLoS One 2014;9:e107161. [Crossref] [PubMed]
- Zeng L, Xiao L, Jiang W, Yang H, Hu D, Xia C, Li Y, Zhou C, Xiong Y, Liu L, Liao D, Guan R, Li K, Wang J, Zhang Y, Yang N, Mansfield AS. Investigation of efficacy and acquired resistance for EGFR-TKI plus bevacizumab as first-line treatment in patients with EGFR sensitive mutant non-small cell lung cancer in a Real world population. Lung Cancer 2020;141:82-8. [Crossref] [PubMed]
- Liu X, Hong L, Nilsson M, Hubert SM, Wu S, Rinsurongkawong W, Lewis J, Spelman A, Roth J, Swisher S, He Y, Jack Lee J, Fang B, Heymach JV, Zhang J, Le X. Concurrent use of aspirin with osimertinib is associated with improved survival in advanced EGFR-mutant non-small cell lung cancer. Lung Cancer 2020;149:33-40. [Crossref] [PubMed]
- Renaud S, Seitlinger J, Guerrera F, Reeb J, Beau-Faller M, Voegeli AC, Siat J, Clément-Duchêne C, Tiotiu A, Santelmo N, Costardi L, Ruffini E, Falcoz PE, Vignaud JM, Massard G. Prognostic Value of Exon 19 Versus 21 EGFR Mutations Varies According to Disease Stage in Surgically Resected Non-small Cell Lung Cancer Adenocarcinoma. Ann Surg Oncol 2018;25:1069-78. [Crossref] [PubMed]
- Carey KD, Garton AJ, Romero MS, Kahler J, Thomson S, Ross S, Park F, Haley JD, Gibson N, Sliwkowski MX. Kinetic analysis of epidermal growth factor receptor somatic mutant proteins shows increased sensitivity to the epidermal growth factor receptor tyrosine kinase inhibitor, erlotinib. Cancer Res 2006;66:8163-71. [Crossref] [PubMed]
- Hong W, Wu Q, Zhang J, Zhou Y. Prognostic value of EGFR 19-del and 21-L858R mutations in patients with non-small cell lung cancer. Oncol Lett 2019;18:3887-95. [Crossref] [PubMed]
- Fukuoka M, Wu YL, Thongprasert S, Sunpaweravong P, Leong SS, Sriuranpong V, Chao TY, Nakagawa K, Chu DT, Saijo N, Duffield EL, Rukazenkov Y, Speake G, Jiang H, Armour AA, To KF, Yang JC, Mok TS. Biomarker analyses and final overall survival results from a phase III, randomized, open-label, first-line study of gefitinib versus carboplatin/paclitaxel in clinically selected patients with advanced non-small-cell lung cancer in Asia (IPASS). J Clin Oncol 2011;29:2866-74. [Crossref] [PubMed]
- Li S, Luo T, Ding C, Huang Q, Guan Z, Zhang H. Detailed identification of epidermal growth factor receptor mutations in lung adenocarcinoma: Combining radiomics with machine learning. Med Phys 2020;47:3458-66. [Crossref] [PubMed]
- Stewart EL, Tan SZ, Liu G, Tsao MS. Known and putative mechanisms of resistance to EGFR targeted therapies in NSCLC patients with EGFR mutations-a review. Transl Lung Cancer Res 2015;4:67-81. [PubMed]
- Ali A, Goffin JR, Arnold A, Ellis PM. Survival of patients with non-small-cell lung cancer after a diagnosis of brain metastases. Curr Oncol 2013;20:e300-6. [Crossref] [PubMed]
- Chen Z, Fillmore CM, Hammerman PS, Kim CF, Wong KK. Non-small-cell lung cancers: a heterogeneous set of diseases. Nat Rev Cancer 2014;14:535-46. [Crossref] [PubMed]
- Song J, Shi J, Dong D, Fang M, Zhong W, Wang K, Wu N, Huang Y, Liu Z, Cheng Y, Gan Y, Zhou Y, Zhou P, Chen B, Liang C, Liu Z, Li W, Tian J. A New Approach to Predict Progression-free Survival in Stage IV EGFR-mutant NSCLC Patients with EGFR-TKI Therapy. Clin Cancer Res 2018;24:3583-92. [Crossref] [PubMed]
- Cao R, Pang Z, Wang X, Du Z, Chen H, Liu J, Yue Z, Wang H, Luo Y, Jiang X. Radiomics evaluates the EGFR mutation status from the brain metastasis: a multi-center study. Phys Med Biol 2022; [Crossref] [PubMed]
- Gillies RJ, Kinahan PE, Hricak H. Radiomics: Images Are More than Pictures, They Are Data. Radiology 2016;278:563-77. [Crossref] [PubMed]
- Rogers W, Thulasi Seetha S, Refaee TAG, Lieverse RIY, Granzier RWY, Ibrahim A, Keek SA, Sanduleanu S, Primakov SP, Beuque MPL, Marcus D, van der Wiel AMA, Zerka F, Oberije CJG, van Timmeren JE, Woodruff HC, Lambin P. Radiomics: from qualitative to quantitative imaging. Br J Radiol 2020;93:20190948. [Crossref] [PubMed]
- Aerts HJ, Velazquez ER, Leijenaar RT, Parmar C, Grossmann P, Carvalho S, Bussink J, Monshouwer R, Haibe-Kains B, Rietveld D, Hoebers F, Rietbergen MM, Leemans CR, Dekker A, Quackenbush J, Gillies RJ, Lambin P. Decoding tumour phenotype by noninvasive imaging using a quantitative radiomics approach. Nat Commun 2014;5:4006. [Crossref] [PubMed]
- Avanzo M, Wei L, Stancanello J, Vallières M, Rao A, Morin O, Mattonen SA, El Naqa I. Machine and deep learning methods for radiomics. Med Phys 2020;47:e185-202. [Crossref] [PubMed]
- Wang G, Wang B, Wang Z, Li W, Xiu J, Liu Z, Han M. Radiomics signature of brain metastasis: prediction of EGFR mutation status. Eur Radiol 2021;31:4538-47. [Crossref] [PubMed]
- Zheng X, Yao Z, Huang Y, Yu Y, Wang Y, Liu Y, Mao R, Li F, Xiao Y, Wang Y, Hu Y, Yu J, Zhou J. Deep learning radiomics can predict axillary lymph node status in early-stage breast cancer. Nat Commun 2020;11:1236. [Crossref] [PubMed]
- Hosny A, Parmar C, Coroller TP, Grossmann P, Zeleznik R, Kumar A, Bussink J, Gillies RJ, Mak RH, Aerts HJWL. Deep learning for lung cancer prognostication: A retrospective multi-cohort radiomics study. PLoS Med 2018;15:e1002711. [Crossref] [PubMed]
- Nardelli P, Jimenez-Carretero D, Bermejo-Pelaez D, Washko GR, Rahaghi FN, Ledesma-Carbayo MJ, San Jose Estepar R. Pulmonary Artery-Vein Classification in CT Images Using Deep Learning. IEEE Trans Med Imaging 2018;37:2428-40. [Crossref] [PubMed]
- Xu Y, Hosny A, Zeleznik R, Parmar C, Coroller T, Franco I, Mak RH, Aerts HJWL. Deep Learning Predicts Lung Cancer Treatment Response from Serial Medical Imaging. Clin Cancer Res 2019;25:3266-75. [Crossref] [PubMed]
- Levine AB, Schlosser C, Grewal J, Coope R, Jones SJM, Yip S. Rise of the Machines: Advances in Deep Learning for Cancer Diagnosis. Trends Cancer 2019;5:157-69. [Crossref] [PubMed]
- Chaudhary K, Poirion OB, Lu L, Garmire LX. Deep Learning-Based Multi-Omics Integration Robustly Predicts Survival in Liver Cancer. Clin Cancer Res 2018;24:1248-59. [Crossref] [PubMed]
- Tong C, Liang B, Su Q, Yu M, Zheng Z. Pulmonary nodule classification based on heterogeneous features learning. IEEE J Sel Areas Commun 2021;39:574-81. [Crossref]
- Hu S, Yu W, Chen Z, Wang S. Medical Image Reconstruction Using Generative Adversarial Network for Alzheimer Disease Assessment with Class-Imbalance Problem. 2020 IEEE 6th International Conference on Computer and Communications (ICCC), Chengdu, China, 2020:1323-7.
- Hu S, Shen Y, Wang S, Lei B. Brain MR to PET Synthesis via Bidirectional Generative Adversarial Network. In: Martel AL, Abolmaesumi P, Stoyanov D, Mateus D, Zuluaga MA, Zhou SK, Racoceanu D, Joskowicz L. Medical Image Computing and Computer Assisted Intervention – MICCAI 2020. Lecture Notes in Computer Science(), vol 12262. Springer, 2020:698-707.
- Lei B, Liang E, Yang M, Yang P, Zhou F, Tan EL, Lei Y, Liu CM, Wang T, Xiao X, Wang S. Predicting clinical scores for Alzheimer’s disease based on joint and deep learning. Expert Systems with Applications 2022;187:115966. [Crossref]
- Wang S, Shi J, Ye Z, Dong D, Yu D, Zhou M, Liu Y, Gevaert O, Wang K, Zhu Y, Zhou H, Liu Z, Tian J. Predicting EGFR mutation status in lung adenocarcinoma on computed tomography image using deep learning. Eur Respir J 2019;53:1800986. [Crossref] [PubMed]
- Yin G, Wang Z, Song Y, Li X, Chen Y, Zhu L, Su Q, Dai D, Xu W. Prediction of EGFR Mutation Status Based on 18F-FDG PET/CT Imaging Using Deep Learning-Based Model in Lung Adenocarcinoma. Front Oncol 2021;11:709137. [Crossref] [PubMed]
- Zhao W, Yang J, Ni B, Bi D, Sun Y, Xu M, Zhu X, Li C, Jin L, Gao P, Wang P, Hua Y, Li M. Toward automatic prediction of EGFR mutation status in pulmonary adenocarcinoma with 3D deep learning. Cancer Med 2019;8:3532-43. [Crossref] [PubMed]
- Coudray N, Ocampo PS, Sakellaropoulos T, Narula N, Snuderl M, Fenyö D, Moreira AL, Razavian N, Tsirigos A. Classification and mutation prediction from non-small cell lung cancer histopathology images using deep learning. Nat Med 2018;24:1559-67. [Crossref] [PubMed]
- Liao CY, Lee CC, Yang HC, Chen CJ, Chung WY, Wu HM, Guo WY, Liu RS, Lu CF. Predicting survival after radiosurgery in patients with lung cancer brain metastases using deep learning of radiomics and EGFR status. Phys Eng Sci Med 2023;46:585-96. [Crossref] [PubMed]
- Chen S, Han X, Tian G, Cao Y, Zheng X, Li X, Li Y. Using stacked deep learning models based on PET/CT images and clinical data to predict EGFR mutations in lung cancer. Front Med (Lausanne) 2022;9:1041034. [Crossref] [PubMed]
- Zhang B, Qi S, Pan X, Li C, Yao Y, Qian W, Guan Y. Deep CNN Model Using CT Radiomics Feature Mapping Recognizes EGFR Gene Mutation Status of Lung Adenocarcinoma. Front Oncol 2021;10:598721. [Crossref] [PubMed]
- Jiang X, Ren M, Shuang X, Yang H, Shi D, Lai Q, Dong Y. Multiparametric MRI-Based Radiomics Approaches for Preoperative Prediction of EGFR Mutation Status in Spinal Bone Metastases in Patients with Lung Adenocarcinoma. J Magn Reson Imaging 2021;54:497-507. [Crossref] [PubMed]
- Fan Y, Dong Y, Wang H, Wang H, Sun X, Wang X, Zhao P, Luo Y, Jiang X. Development and externally validate MRI-based nomogram to assess EGFR and T790M mutations in patients with metastatic lung adenocarcinoma. Eur Radiol 2022;32:6739-51. [Crossref] [PubMed]
- Cao R, Dong Y, Wang X, Ren M, Wang X, Zhao N, Yu T, Zhang L, Luo Y, Cui EN, Jiang X. MRI-Based Radiomics Nomogram as a Potential Biomarker to Predict the EGFR Mutations in Exon 19 and 21 Based on Thoracic Spinal Metastases in Lung Adenocarcinoma. Acad Radiol 2022;29:e9-e17. [Crossref] [PubMed]
- He K, Zhang X, Ren S, Sun J. Deep residual learning for image recognition. Proceedings of the IEEE Conference on Computer Vision and Pattern Recognition (CVPR), 2016:770-8.
- Woo S, Park J, Lee JY, Kweon IS. CBAM: Convolutional Block Attention Module. Proceedings of the European Conference on Computer Vision (ECCV), 2018:3-19.
- Lin M, Chen Q, Yan S. Network in Network. 2nd International Conference on Learning Representations, ICLR 2014, Banff, AB, 2014.
- van Griethuysen JJM, Fedorov A, Parmar C, Hosny A, Aucoin N, Narayan V, Beets-Tan RGH, Fillion-Robin JC, Pieper S, Aerts HJWL. Computational Radiomics System to Decode the Radiographic Phenotype. Cancer Res 2017;77:e104-7. [Crossref] [PubMed]
- Li Z, Duan H, Zhao K, Zhao K, Ding Y. Stability of MRI Radiomics Features of Hippocampus: An Integrated Analysis of Test-Retest and Inter-Observer Variability. IEEE Access 2019;7:97106-16.
- Yin P, Mao N, Zhao C, Wu J, Sun C, Chen L, Hong N. Comparison of radiomics machine-learning classifiers and feature selection for differentiation of sacral chordoma and sacral giant cell tumour based on 3D computed tomography features. Eur Radiol 2019;29:1841-7. [Crossref] [PubMed]
- Ruopp MD, Perkins NJ, Whitcomb BW, Schisterman EF. Youden Index and optimal cut-point estimated from observations affected by a lower limit of detection. Biom J 2008;50:419-30. [Crossref] [PubMed]
- Selvaraju RR, Cogswell M, Das A, Parikh D, Batra D. Grad-CAM: visual explanations from deep networks via gradient-based localization. Int J Comput Vis 2020;128:336-59. [Crossref]
- Wang S, Yu H, Gan Y, Wu Z, Li E, Li X, et al. Mining whole-lung information by artificial intelligence for predicting EGFR genotype and targeted therapy response in lung cancer: a multicohort study. Lancet Digit Health 2022;4:e309-19. [Crossref] [PubMed]
- Zhao W, Chen W, Li G, Lei D, Yang J, Chen Y, Jiang Y, Wu J, Ni B, Sun Y, Wang S, Sun Y, Li M, Liu J. GMILT: A Novel Transformer Network That Can Noninvasively Predict EGFR Mutation Status. IEEE Trans Neural Netw Learn Syst 2024;35:7324-38. [Crossref] [PubMed]
- Wang C, Ma J, Shao J, Zhang S, Liu Z, Yu Y, Li W. Predicting EGFR and PD-L1 Status in NSCLC Patients Using Multitask AI System Based on CT Images. Front Immunol 2022;13:813072. [Crossref] [PubMed]
- Wang C, Xu X, Shao J, Zhou K, Zhao K, He Y, Li J, Guo J, Yi Z, Li W. Deep Learning to Predict EGFR Mutation and PD-L1 Expression Status in Non-Small-Cell Lung Cancer on Computed Tomography Images. J Oncol 2021;2021:5499385. [Crossref] [PubMed]
- Gui D, Song Q, Song B, Li H, Wang M, Min X, Li A. AIR-Net: A novel multi-task learning method with auxiliary image reconstruction for predicting EGFR mutation status on CT images of NSCLC patients. Comput Biol Med 2022;141:105157. [Crossref] [PubMed]
- Tan X, Li Y, Wang S, Xia H, Meng R, Xu J, Duan Y, Li Y, Yang G, Ma Y, Jin Y. Predicting EGFR mutation, ALK rearrangement, and uncommon EGFR mutation in NSCLC patients by driverless artificial intelligence: a cohort study. Respir Res 2022;23:132. [Crossref] [PubMed]
- Shi J, Zhao Z, Jiang T, Ai H, Liu J, Chen X, Luo Y, Fan H, Jiang X. A deep learning approach with subregion partition in MRI image analysis for metastatic brain tumor. Front Neuroinform 2022;16:973698. [Crossref] [PubMed]
- Haim O, Abramov S, Shofty B, Fanizzi C, DiMeco F, Avisdris N, Ram Z, Artzi M, Grossman R. Predicting EGFR mutation status by a deep learning approach in patients with non-small cell lung cancer brain metastases. J Neurooncol 2022;157:63-9. [Crossref] [PubMed]
- Jiang T, Sun XY, Dong Y, Guo W, Wang HB, Yue ZB, Luo YH, Jiang XR. Deep learning for preoperative prediction of the EGFR mutation and subtypes based on the MRI image of spinal metastasis from primary NSCLC. Biomedical Signal Processing and Control 2023;79:104084. [Crossref]
- Kong T, Yao AB, Chen YR, Sun F. Hypernet: Towards accurate region proposal generation and joint object detection. Proceedings of the IEEE Conference on Computer Vision and Pattern Recognition (CVPR), 2016:845-53.
- Vaswani A, Shazeer N, Parmar N, Uszkoreit J, Jones L, Gomez AN, Kaiser Ł, Polosukhin I. Attention is all you need. Advances in Neural Information Processing Systems 30 (NIPS 2017) 2017. doi:
10.48550/arXiv.1706.03762 .10.48550/arXiv.1706.03762 - Hu J, Shen L, Albanie S, Sun G, Vedaldi A. Gather-Excite: Exploiting Feature Con-text in Convolutional Neural Networks. Advances in Neural Information Processing Systems 31 (NeurIPS 2018) 2018.
- Hu J, Shen L, Albanie S, Sun G, Wu E. Squeeze-and-Excitation Networks. IEEE Trans Pattern Anal Mach Intell 2020;42:2011-23. [Crossref] [PubMed]
- Song J, Ding C, Huang Q, Luo T, Xu X, Chen Z, Li S. Deep learning predicts epidermal growth factor receptor mutation subtypes in lung adenocarcinoma. Med Phys 2021;48:7891-9. [Crossref] [PubMed]
- Zhang Y, Hong D, McClement D, Oladosu O, Pridham G, Slaney G. Grad-CAM helps interpret the deep learning models trained to classify multiple sclerosis types using clinical brain magnetic resonance imaging. J Neurosci Methods 2021;353:109098. [Crossref] [PubMed]