Application of metal artifact reduction algorithm in reducing metal artifacts in post-surgery pediatric low radiation dose spine computed tomography (CT) images
Introduction
Computed tomography (CT) is a commonly used examination for spinal diseases (1-6), especially after posterior spinal fixation surgery with metal pedicle screws. The application of magnetic resonance (MR) is limited (7,8), and CT has become the most feasible tomographic imaging examination method. However, the display of structures around metals in CT images is often obstructed by the beam hardening artifacts caused by the high-density metals, affecting diagnosis. Therefore, reducing the beam hardening artifacts of pedicle screws can better evaluate the condition of the bone and soft tissue around the metal screws, helping orthopedic doctors and radiologists to make more accurate evaluations of the surgical effect and condition (9-11). At present, the commonly used methods for removing metal beam hardening artifacts often rely on the use of high energy photons with either high tube voltage or high energy virtual monoenergetic images in dual-energy CT (12-14), or the combination of metal artifact reduction (MAR) technology with reconstruction algorithms to further significantly reduce beam hardening artifacts and improve diagnostic effectiveness (15-18). However, in order to generate adequate signal when taking images of metals, radiation dose is usually relatively high. Given that children are more sensitive to ionizing radiation than adults, we would like to find a method that can eliminate metal artifacts under low radiation dose scanning conditions and maintain image quality.
The research on metal-induced beam hardening artifacts removal technology based on low-dose CT scanning in clinical practice is insufficient. There are many factors that affect the quality of CT images after spinal surgery in children, such as the selection of tube voltage, which can affect image contrast and the intensity of beam hardening artifacts (19,20), and the reconstruction algorithms, which can affect image noise (21-23). In order to better apply MAR technology in children, we evaluated the image quality of a group of low radiation dose pediatric spinal CT scans using different scanning voltages, radiation dose levels and image reconstruction algorithms. We evaluated the feasibility of reducing metal-induced beam hardening artifacts with MAR, and whether the scanning tube voltage, radiation dose and image reconstruction algorithm had an impact on the effectiveness of MAR and overall image quality. We present this article in accordance with the STROBE reporting checklist (available at https://qims.amegroups.com/article/view/10.21037/qims-23-1659/rc).
Methods
General information
The study was conducted in accordance with the Declaration of Helsinki (as revised in 2013). This study was a retrospective study and was approved by the Ethics Committee of Beijing Children’s Hospital (No. 2019-46). The study protocols were selected following the approved guidelines and regulations of Beijing Children’s Hospital. Informed consents were signed by the parents of pediatric patients. This retrospective study enrolled 77 children who underwent spinal CT performed on a 256 row CT scanner (Revolution CT, GE HealthCare, Waukesha, WI, USA), which was equipped with the MRA (Smart-MARTM, GE HealthCare, Waukesha, WI, USA) algorithm, after surgery to observe pedicle screw status from May 2021 to December 2022. The average age was 10.09±3.23 years old (3–15 years old), male to female ratio was 38:39 (Table 1), the time interval was 118.8±68.6 days (47–325 days) between the CT scan and surgery.
Table 1
Group | 2.6 mGy group | 1.4 mGy group | Total |
---|---|---|---|
Cases (number) | 52 | 25 | 77 |
140 kV | 29 | 18 | |
100 kV | 23 | 7 | |
Age (mean ± SD, years) | 11.87±2.18 | 6.40±1.78 | 10.09±3.23 |
140 kV | 12.00±2.09 | 6.44±1.75 | |
100 kV | 11.70±2.33 | 6.29±2.00 | |
Male:female (number) | 25:27 | 13:12 | 38:39 |
140 kV | 12:17 | 10:8 | |
100 kV | 13:10 | 3:4 | |
Screw (number) | 80 | 36 | 116 |
140 kV | 44 | 24 | |
100 kV | 36 | 12 |
SD, standard deviation.
CT imaging methodology
All scans used low-dose CT protocol with a tube voltage of either 140 or 100 kV randomly. The radiation dose was 1.40 mGy for children of the age of 3–8 years and 2.61 mGy for the age of 9–15, with fixed tube currents to achieve the desired radiation dose levels. The raw data was reconstructed with the 50% adaptive statistical iterative reconstruction-V (ASIR-V), 50% ASIR-V with MAR (AV-MAR), deep learning image reconstruction (DLIR) with high strength and high-strength DLIR with MAR (DL-MAR). All images had a thickness of 0.625 mm with a standard reconstruction kernel.
Objective image quality evaluation
Images were transmitted to a post-processing advanced workstation (AW4.7, GE HealthCare, USA) for analysis. Two radiologists (with 15 and 8 years of pediatric radiology experience) made objective evaluations of the images, including the measurement of the length of beam hardening artifact (LHA) (Figure 1) and calculation of the artifact index (AI). Considering that the severity of beam hardening artifacts is related to the direction of the screw, this study selected screws that were parallel to the imaging plane for evaluation (Figure 2). A total of 558 pedicle screws were scanned, with 4–17 screws per patient, and a total of 116 screws met the evaluation criteria (Table 1). LHA was calculated using ImageJ software in reference to other relevant studies (24,25). As demonstrated in Figure 1, the specific method was to display the axial image of the maximum length of the screw as the evaluation layer, set a measurement baseline at the centerline of the screw’s long axis, and use the software to capture the CT values of all points on this baseline. The LHA was calculated one-sided from the tip of the screw outward. The point (point 1) where the CT value near the screw tip is less than 3,000 Hounsfield unit (HU) was used as the starting point for calculating LHA. The CT value would turn into negative values due to metal/beam hardening artifacts, the next point (point 2) where the CT value turns positive was used as the endpoint for LHA calculation. The length between the two points was calculated as LHA. The profiles through the metal for calculating LHA were the same for the four sets of images of the same patient with the same screw, which were jointly set by the two observers. The AI was calculated based on Lin et al.’s paper (26): set region of interest (ROI) at the evaluation level to measure the CT and standard deviation values of the most hypodense streak (sd1) and normal homogeneous tissue (sd0) without metal artifact in the same slice (Figure 2B) and calculate AI value using the equation . Sd1 and sd0 were also compared to evaluating the influence of metal artifacts on normal structures.
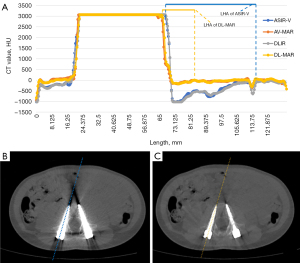
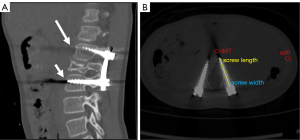
The length and diameter of the screws were measured on multi-planner reconstruction images (Figure 2B) with a window level of 3,000 and window width of 8,000, then compared with the actual size recorded after the surgery with a paired-t analysis.
Subjective and subjective image quality evaluation
The same two radiologists independently applied a 4-point scale to subjectively evaluate the degree of impact of metal beam hardening artifacts on images in all cases (77 cases) (4 = the minor streaks only at the thick ports of the metal implant, 3 = some streaks, could identify the surrounding structures, 2 = pronounced streaks impacting the evaluation of surrounding structures, 1 = significant artifacts). The degree of beam hardening artifacts was evaluated on the image slice with the most obvious beam hardening artifacts. The overall subjective image quality was also evaluated using the 4-point scale (4 = little image noise, clear structures, excellent image quality; 3 = some image noise, mostly clear structures, acceptable image quality; 2 = high image noise, questionable structures, unacceptable image quality; 1= severe image noise, hard to detect organ margin, undiagnosable).
Statistical analysis
The subjective results and objective results were listed as mean ± standard deviation, analysis of variance was used for comparison between groups, a followed post hoc analysis (Bonferroni correction test) was performed to determine differences. If the data did not follow a normal distribution, then the Friedman test was used to analysis. The kappa test was performed to analysis the consistency of the subjective scores given by two radiologists. All statistical analyses procedure were performing on SPSS software (version 17.0; IBM Corp., Armonk, NY, USA), and differences with P<0.05 were considered statistically significant.
Results
The subjective scores and objective measurements of different reconstruction algorithms under different radiation doses and scanning voltages are shown in Table 2 and Table 3.
Table 2
Subject | Group | ASIR-V | AV-MAR | DLIR | DL-MAR | F value | P |
---|---|---|---|---|---|---|---|
Metal beam hardening artifacts | 2.6 mGy 140 kV | 1.73±0.45 | 3.30±0.46 | 1.68±0.47 | 3.34±0.48 | 172.36 | <0.001 |
2.6 mGy 100 kV | 1.67±0.48 | 3.13±0.34 | 1.79±0.41 | 3.54±0.51 | 109.85 | <0.001 | |
1.4 mGy 140 kV | 1.89±0.32 | 3.64±0.49 | 1.94±0.23 | 3.61±0.49 | 219.95 | <0.001 | |
1.4 mGy 100 kV | 1.42±0.51 | 3.33±0.49 | 1.42±0.51 | 3.42±0.51 | 59.18 | <0.001 | |
Mean | 1.73±0.44 | 3.37±0.49 | 1.76±0.43 | 3.47±0.50 | 381.00 | <0.001 | |
Overall subjective image quality | 2.6 mGy 140 kV | 2.93±0.50 | 3.07±0.25 | 3.34±0.78 | 3.52±0.51 | 20.38 | <0.001 |
2.6 mGy 100 kV | 2.79±0.41 | 3.00±0.00 | 2.92±0.83 | 3.67±0.48 | 13.46 | <0.001 | |
1.4 mGy 140 kV | 2.81±0.47 | 3.00±0.00 | 3.11±0.75 | 3.42±0.50 | 9.16 | <0.001 | |
1.4 mGy 100 kV | 2.67±0.49 | 3.00±0.00 | 3.00±0.74 | 3.50±0.52 | 5.34 | 0.003 | |
Mean | 2.84±0.47 | 3.03±0.16 | 3.15±0.78 | 3.52±0.50 | 160.30 | <0.001 |
SD, standard deviation; ASIR-V, 50% adaptive statistical iterative reconstruction-V; AV-MAR, 50% ASIR-V with MAR; DLIR, deep learning image reconstruction; DL-MAR, DLIR with MAR; MAR, metal artifact reduction.
Table 3
Subject | Group | ASIR-V | AV-MAR | DLIR | DL-MAR | F value | P |
---|---|---|---|---|---|---|---|
Sd1 (HU) | 2.6 mGy 140 kV | 117.06±29.85 | 57.42±15.89 | 113.67±32.40 | 54.35±16.21 | 37.68 | <0.001 |
2.6 mGy 100 kV | 300.51±94.51 | 104.89±46.37 | 300.89±98.94 | 100.43±47.53 | 22.55 | <0.001 | |
1.4 mGy 140 kV | 173.36±83.23 | 55.19±17.16 | 171.04±79.62 | 51.39±16.10 | 38.80 | <0.001 | |
1.4 mGy 100 kV | 181.01±55.11 | 59.22±15.42 | 164.96±56.17 | 54.83±13.09 | 21.42 | <0.001 | |
Mean | 179.10±94.87 | 66.74±31.82 | 175.52±96.24 | 63.01±31.73 | 95.67 | <0.001 | |
Sd0 (HU) | 2.6 mGy 140 kV | 23.90±6.01 | 20.12±5.25 | 14.21±4.36 | 11.89±2.94 | 8.22 | <0.001 |
2.6 mGy 100 kV | 18.81±3.01 | 17.66±2.98 | 12.71±2.14 | 11.36±2.21 | 3.85 | <0.001 | |
1.4 mGy 140 kV | 25.51±4.86 | 21.89±5.02 | 16.43±4.47 | 14.65±2.94 | 13.52 | <0.001 | |
1.4 mGy 100 kV | 26.28±9.15 | 21.31±5.83 | 23.23±10.28 | 16.67±6.58 | 13.96 | <0.001 | |
Mean | 23.59±6.07 | 20. 28±5.01 | 15.52±5.72 | 13.24±3.87 | 91.21 | <0.001 | |
AI | 2.6 mGy 140 kV | 114.29±30.44 | 53.11±17.29 | 112.61±32.69 | 52.82±116.66 | 83.50 | <0.001 |
2.6 mGy 100 kV | 299.83±94.79 | 102.85±47.53 | 300.57±99.08 | 99.51±48.07 | 54.24 | <0.001 | |
1.4 mGy 140 kV | 170.75±84.01 | 49.80±18.92 | 169.85±80.38 | 48.71±17.46 | 49.24 | <0.001 | |
1.4 mGy 100 kV | 178.34±56.95 | 54.35±17.52 | 162.44±57.98 | 51.09±14.85 | 31.35 | <0.001 | |
Mean | 176.82±96.01 | 62.50±33.51 | 174.42±96.82 | 61.03±32.61 | 95.69 | <0.001 | |
LHA (mm) | 2.6 mGy 140 kV | 35.77±19.41 | 15.14±10.58 | 35.62±19.02 | 15.11±10.94 | 20.58 | <0.001 |
2.6 mGy 100 kV | 35.86±20.29 | 17.65±8.92 | 35.80±20.00 | 17.31±9.31 | 11.02 | <0.001 | |
1.4 mGy 140 kV | 40.48±24.31 | 15.29±11.53 | 40.25±24.04 | 15.47±12.01 | 20.73 | <0.001 | |
1.4 mGy 100 kV | 32.01±16.16 | 15.64±5.89 | 32.37±16.41 | 14.94±5.86 | 7.64 | <0.001 | |
Mean | 36.86±20.86 | 15.76±10.12 | 36.76±20.58 | 15.66±10.49 | 36.18 | <0.001 |
SD, standard deviation; ASIR-V, 50% adaptive statistical iterative reconstruction-V; AV-MAR, 50% ASIR-V with MAR; DLIR, deep learning image reconstruction; DL-MAR, DLIR with MAR; MAR, metal artifact reduction; sd1, standard deviation values of the most hyperdense streak; sd0, normal homogeneous tissue without metal artifact in same slice; HU, Hounsfield unit; AI, artifact index; LHA, length of hardening artifact.
The LHA values were 36.86±20.86, 15.76±10.12, 36.76±20.58, and 15.66±10.49 mm, and the AI values were 176.82±96.01, 62.50±33.51, 174.42±96.82, 61.03±32.61, with ASIR-V, AV-MAR, DLIR and DL-MAR, respectively. After using MAR, the beam hardening artifact length was reduced by about 57% (range, 51.14–62.23%, all P<0.001) across different tube voltages, radiation dose levels and reconstruction algorithms.
Both the LHA and AI of images with MAR were lower than those of without MAR, and there was no statistically significant difference between ASIR-V images and DLIR images (Figure 3). The artifact degree scores were 1.73±0.44, 3.37±0.49, 1.76±0.43, and 3.47±0.50, respectively, indicating that MAR subjectively reduced the influence of beam hardening artifacts. Further sub-group analysis indicated (Table 2) that the objective measurements and subjective scores were improved for both the 100 and 140 kV scanning voltages after applying MAR and was true for both ASIR-V and DLIR algorithms.
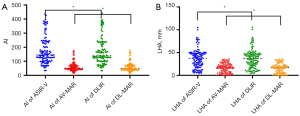
The diameter measurements of the screws in the four image groups were 4.30±0.98, 3.49±0.78, 4.34±0.93, and 3.47±0.77 mm, respectively. There was no statistically significant difference in the diameter between the measured and true values for the without-MAR images (Table 4), while the measured values in MAR images were smaller than those in the without-MAR images and the true diameters, with statistically significant differences. The lengths of screw were 32.34±4.90, 31.74±5.16, 32.37±5.05, and 31.93±5.19 mm with the ASIR-V, AV-MAR, DLIR and DL-MAR images (Table 4), respectively. There was no statistical difference among the four image groups and between the measurement in each group and the actual length of metal nails.
Table 4
Subject | ASIR-V | AV-MAR | DLIR | DL-MAR | True value | F value | P |
---|---|---|---|---|---|---|---|
Screw width (mm) | 4.30±0.98 | 3.49±0.78* | 4.34±0.93 | 3.47±0.77# | 4.21±0.83*# | 30.20 | <0.001 |
Screw length (mm) | 32.34±4.90 | 31.74±5.16 | 32.37±5.05 | 31.93±5.19 | 32.20±4.95 | 0.25 | 0.86 |
*, there was a statistical difference between AV-MAR and the screw true size; #, there was a statistical difference between DL-MAR and the screw true size. SD, standard deviation; ASIR-V, 50% adaptive statistical iterative reconstruction-V; AV-MAR, 50% ASIR-V with MAR; DLIR, deep learning image reconstruction; DL-MAR, DLIR with MAR; MAR, metal artifact reduction.
The overall subjective image quality scores were 2.84±0.47, 3.03±0.16, 3.15±0.78 and 3.52±0.50 with ASIR-V, AV-MAR, DLIR, and DL-MAR, respectively. DL-MAR further improved image quality compared to AV-MAR with reduced image noise (P<0.05). The two observers had excellent agreement with a kappa value of 0.87 (P<0.05).
Discussion
In our study we evaluated the effectiveness of MAR and the impact of combining MAR with the newly introduced DLIR on the overall image quality in pediatric patients with metal screws. Our results showed that MAR could significantly reduce the LHA and AI of the images. LHA mainly evaluates the extend of metal-induced beam hardening artifacts, and the most obvious appearance of beam hardening artifacts is the low-density streaks along the long axis of the metal screws. The structures in this low-density area cannot be observed and reducing the beam hardening artifacts length would allow us to evaluate the situation of bone and soft tissue in front of the screws more accurately. So, when defining LHA, we mainly evaluated the parts with CT values below 0 HU to reflect the length of beam hardening artifacts more accurately. Unlike LHA, AI mainly reflects the degree of difference in density changes around screws (26-28). The higher the AI value, the less accurate in observing surrounding tissues. This indicator has also been used in multiple studies. Combining AI to determine the degree of artifacts and LHA to determine the length of beam hardening artifacts in our study provided a more accurate and objective evaluation of beam hardening artifacts. The AI results showed that after using MAR, the beam hardening artifact severity in the ASIR-V and DLIR images decreased by 46.31% and 47.54% (all P<0.001), respectively. Our results also showed that the degree of reduction in AI by MAR was true across different tube voltages, radiation dose levels and reconstruction algorithms.
The subjective scoring results of metal-induced beam hardening artifacts showed that the subjective scores with MAR were 3.37–3.47, and there was no statistically significant difference between the AV-MAR and DL-MAR. These scores were both statistically higher than the 1.73–1.76 of the images without MAR.
During the evaluation process, we found that MAR technology could reduce beam hardening artifacts for the surrounding structures while causing certain distortion on screw itself, which is consistent with previous research results (12,27,29). Some scholars believed that this distortion of MAR could affect the judgment of screws and recommended to use a 140 keV monochromatic images in dual energy CT without the use of MAR when evaluating the metal screws. We measured the diameter and length of the screws on the MAR images, and the diameter measurement results on the MAR images were 9.62–18.02% smaller than the actual diameters, with the differences statistically significant. The measurement results of the screws’ length showed no significant difference between the MAR images and the actual length. However, postoperative CT scans of the spine mainly observe the integrity of screws and the presence of abnormalities in the surrounding structures (2-9). Due to the high density of screws, although they were affected by metal beam hardening artifacts, the integrity of screws could still be understood by adjusting the window width and position. The distortion of the metal screws in MAR images did not affect the evaluation of screw integrity. The role of MAR is mainly reflected in the evaluation of the surrounding structures of screws (2,6,9,30) (Figure 4), The bone and soft tissue conditions around the screw are the key and difficult points of postoperative observation. By using MAR technology, the length of beam hardening artifacts (reduction of LHA length) and the intensity of beam hardening artifacts (improvement of AI) were reduced, which could improve image quality, enhance the observation range and clarity of surrounding tissues to achieve a comprehensive evaluation of postoperative conditions using low-dose CT.
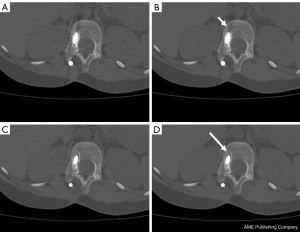
Our study indicated that by combining MAR with the newly developed DLIR algorithm, image noise was reduced compared with ASIR-V resulted in higher subjective score of 3.52±0.5 than AV-MAR (3.03±0.16). In reducing image noise and maintaining image texture, we were able to obtain quieter and clearer images (Figure 5). The subjective score of ASIR-V was the lowest (2.84±0.47) due to higher metal beam hardening artifacts and higher image noise.
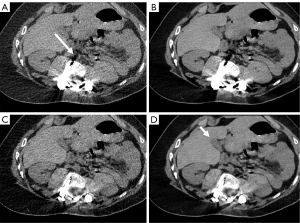
There are some limitations in this study: first, this study was a retrospective study with a small sample size and uneven distribution of cases and limited number of spinal surgeries performed on children in the younger age group. Therefore, the number of cases in the younger age group was small, more studies are needed with larger sample sizes to further validate the conclusion. In terms of tube voltage selection, although it is recommended to use 100 kV scanning in children, which can improve image contrast under the premise of low dose scanning, it is believed that higher voltage can suppress metal beam hardening artifacts more in post spinal surgery scanning (27). To achieve higher image quality, in practical work, we do not explicitly specify the scanning voltage of CT after pediatric spinal surgery. Radiologists could use either 100 or 140 kV based on their own experience. Therefore, the distribution of 100 and 140 kV groups in the cases we reviewed was not uniform. Second, in our study, we did not have sufficient cases with loosening of screws, so we could not assess the effectiveness with MAR technology. Third, there was no control group which uses dual energy CT imaging mode. In the next step, we should observe the difference in image quality between conventional CT MAR and dual energy CT MAR. Fourth, the images were reconstructed with standard kernel, because the current version DLIR cannot be combined with bone kernel.
In conclusion, by using MAR technology in routine low-dose pediatric post-surgery CT scans, the metal-induced beam hardening artifacts can be reduced, and the effectiveness of MAR in reducing such beam hardening artifacts is true across different tube voltages, radiation dose levels and reconstruction algorithms. Combining MAR with advanced DLIR algorithm further significantly reduces image noise and improves image quality to realize the possibility of CT imaging in low radiation dose conditions.
Acknowledgments
Funding: This work was supported by
Footnote
Reporting Checklist: The authors have completed the STROBE reporting checklist. Available at https://qims.amegroups.com/article/view/10.21037/qims-23-1659/rc
Conflicts of Interest: All authors have completed the ICMJE uniform disclosure form (available at https://qims.amegroups.com/article/view/10.21037/qims-23-1659/coif). The authors have no conflicts of interest to declare.
Ethical Statement: The authors are accountable for all aspects of the work in ensuring that questions related to the accuracy or integrity of any part of the work are appropriately investigated and resolved. The study was conducted in accordance with the Declaration of Helsinki (as revised in 2013). This study was a retrospective study and was approved by the Ethics Committee of Beijing Children’s Hospital (No. 2019-46). The study protocols were performed in accordance with the approved guidelines and regulations of Beijing Children’s Hospital. Informed consents were signed by the parents of pediatric patients.
Open Access Statement: This is an Open Access article distributed in accordance with the Creative Commons Attribution-NonCommercial-NoDerivs 4.0 International License (CC BY-NC-ND 4.0), which permits the non-commercial replication and distribution of the article with the strict proviso that no changes or edits are made and the original work is properly cited (including links to both the formal publication through the relevant DOI and the license). See: https://creativecommons.org/licenses/by-nc-nd/4.0/.
References
- Ruiz Santiago F, Láinez Ramos-Bossini AJ, Wáng YXJ, Martínez Barbero JP, García Espinosa J, Martínez Martínez A. The value of magnetic resonance imaging and computed tomography in the study of spinal disorders. Quant Imaging Med Surg 2022;12:3947-86. [Crossref] [PubMed]
- Oba H, Uehara M, Ikegami S, Hatakenaka T, Kamanaka T, Miyaoka Y, Kurogouchi D, Fukuzawa T, Mimura T, Tanikawa Y, Koseki M, Ohba T, Takahashi J. Tips and pitfalls to improve accuracy and reduce radiation exposure in intraoperative CT navigation for pediatric scoliosis: a systematic review. Spine J 2023;23:183-96. [Crossref] [PubMed]
- Lenz M, Oikonomidis S, Harland A, Fürnstahl P, Farshad M, Bredow J, Eysel P, Scheyerer MJ. Scoliosis and Prognosis-a systematic review regarding patient-specific and radiological predictive factors for curve progression. Eur Spine J 2021;30:1813-22. [Crossref] [PubMed]
- Calloni SF, Huisman TA, Poretti A, Soares BP. Back pain and scoliosis in children: When to image, what to consider. Neuroradiol J 2017;30:393-404. [Crossref] [PubMed]
- Kwan MK, Loh KW, Chung WH, Chiu CK, Hasan MS, Chan CYW. Perioperative outcome and complications following single-staged Posterior Spinal Fusion (PSF) using pedicle screw instrumentation in Adolescent Idiopathic Scoliosis (AIS): a review of 1057 cases from a single centre. BMC Musculoskelet Disord 2021;22:413. [Crossref] [PubMed]
- Qu B, Cao J, Qian C, Wu J, Lin J, Wang L, Ou-Yang L, Chen Y, Yan L, Hong Q, Zheng G, Qu X. Current development and prospects of deep learning in spine image analysis: a literature review. Quant Imaging Med Surg 2022;12:3454-79. [Crossref] [PubMed]
- Heinrich A, Reinhold M, Güttler FV, Matziolis G, Teichgräber UK, Zippelius T, Strube P. MRI following scoliosis surgery? An analysis of implant heating, displacement, torque, and susceptibility artifacts. Eur Radiol 2021;31:4298-307. [Crossref] [PubMed]
- Wang S, Qiu Y, Liu W, Shi B, Wang B, Yu Y, Zhu Z, Qian B, Zhu F, Sun X. The potential risk of spinal cord injury from pedicle screw at the apex of adolescent idiopathic thoracic scoliosis: magnetic resonance imaging evaluation. BMC Musculoskelet Disord 2015;16:310. [Crossref] [PubMed]
- Xu F, Zou D, Li W, Sun Z, Jiang S, Zhou S, Li Z. Hounsfield units of the vertebral body and pedicle as predictors of pedicle screw loosening after degenerative lumbar spine surgery. Neurosurg Focus 2020;49:E10. [Crossref] [PubMed]
- Wang T, Ishihara T, Kono A, Yoshida N, Akasaka H, Mukumoto N, Yada R, Ejima Y, Yoshida K, Miyawaki D, Kakutani K, Nishida K, Negi N, Minami T, Aoyama Y, Takahashi S, Sasaki R. Application of dual-energy CT to suppression of metal artefact caused by pedicle screw fixation in radiotherapy: a feasibility study using original phantom. Phys Med Biol 2017;62:6226-45. [Crossref] [PubMed]
- Zhao H, Wang YJ, Wang RG, Liu D, Duan YQ, Liu YJ, Zeng YH, Zhao QP, Zhang ZP. Three-Dimensional Hounsfield Units Measurement of Pedicle Screw Trajectory for Predicating Screw Loosening in Lumbar Fusion Surgery. Clin Interv Aging 2023;18:485-93. [Crossref] [PubMed]
- Pettersson E, Bäck A, Thilander-Klang A. COMPARISON OF METAL ARTEFACTS FOR DIFFERENT DUAL ENERGY CT TECHNIQUES. Radiat Prot Dosimetry 2021;195:232-45. [Crossref] [PubMed]
- Cheraya G, Sharma S, Chhabra A. Dual energy CT in musculoskeletal applications beyond crystal imaging: bone marrow maps and metal artifact reduction. Skeletal Radiol 2022;51:1521-34. [Crossref] [PubMed]
- Lee KYG, Cheng HMJ, Chu CY, Tam CWA, Kan WK. Metal artifact reduction by monoenergetic extrapolation of dual-energy CT in patients with metallic implants. J Orthop Surg (Hong Kong) 2019;27:2309499019851176. [Crossref] [PubMed]
- Pessis E, Sverzut JM, Campagna R, Guerini H, Feydy A, Drapé JL. Reduction of Metal Artifact with Dual-Energy CT: Virtual Monospectral Imaging with Fast Kilovoltage Switching and Metal Artifact Reduction Software. Semin Musculoskelet Radiol 2015;19:446-55. [Crossref] [PubMed]
- Wellenberg RHH, Hakvoort ET, Slump CH, Boomsma MF, Maas M, Streekstra GJ. Metal artifact reduction techniques in musculoskeletal CT-imaging. Eur J Radiol 2018;107:60-9. [Crossref] [PubMed]
- Zhu Y, Zhao H, Wang T, Deng L, Yang Y, Jiang Y, Li N, Chan Y, Dai J, Zhang C, Li Y, Xie Y, Liang X. Sinogram domain metal artifact correction of CT via deep learning. Comput Biol Med 2023;155:106710. [Crossref] [PubMed]
- Byl A, Klein L, Sawall S, Heinze S, Schlemmer HP, Kachelrieß M. Photon-counting normalized metal artifact reduction (NMAR) in diagnostic CT. Med Phys 2021;48:3572-82. [Crossref] [PubMed]
- Sun J, Zhang Q, Zhou Z, Jia C, Yang W, Li H, Peng Y. Optimal tube voltage for abdominal enhanced CT in children: a self-controlled study. Acta Radiol 2020;61:101-9. [Crossref] [PubMed]
- Tian X, Chang Z, Dilixiati S, Haimiti Y, Wang S, Sun J. Optimizing image quality and minimizing radiation dose in pediatric abdominal multiphase contrast-enhanced computed tomography: a study on CARE kV and CARE Dose 4D. Quant Imaging Med Surg 2024;14:1985-93. [Crossref] [PubMed]
- Sun J, Li H, Gao J, Li J, Li M, Zhou Z, Peng Y. Performance evaluation of a deep learning image reconstruction (DLIR) algorithm in "double low" chest CTA in children: a feasibility study. Radiol Med 2021;126:1181-8. [Crossref] [PubMed]
- Jiang B, Li N, Shi X, Zhang S, Li J, de Bock GH, Vliegenthart R, Xie X. Deep Learning Reconstruction Shows Better Lung Nodule Detection for Ultra-Low-Dose Chest CT. Radiology 2022;303:202-12. [Crossref] [PubMed]
- Han WK, Na JC, Park SY. Low-dose CT angiography using ASiR-V for potential living renal donors: a prospective analysis of image quality and diagnostic accuracy. Eur Radiol 2020;30:798-805. [Crossref] [PubMed]
- Tatsugami F, Higaki T, Sakane H, Fukumoto W, Kaichi Y, Iida M, Baba Y, Kiguchi M, Kihara Y, Tsushima S, Awai K. Coronary Artery Stent Evaluation with Model-based Iterative Reconstruction at Coronary CT Angiography. Acad Radiol 2017;24:975-81. [Crossref] [PubMed]
- Kitahara H, Nagatani Y, Otani H, Nakayama R, Kida Y, Sonoda A, Watanabe Y. A novel strategy to develop deep learning for image super-resolution using original ultra-high-resolution computed tomography images of lung as training dataset. Jpn J Radiol 2022;40:38-47. [Crossref] [PubMed]
- Lin XZ, Miao F, Li JY, Dong HP, Shen Y, Chen KM, High-definition CT. Gemstone spectral imaging of the brain: initial results of selecting optimal monochromatic image for beam-hardening artifacts and image noise reduction. J Comput Assist Tomogr 2011;35:294-7. [Crossref] [PubMed]
- Wang Y, Qian B, Li B, Qin G, Zhou Z, Qiu Y, Sun X, Zhu B. Metal artifacts reduction using monochromatic images from spectral CT: evaluation of pedicle screws in patients with scoliosis. Eur J Radiol 2013;82:e360-6. [Crossref] [PubMed]
- Jabas A, Abello Mercado MA, Altmann S, Ringel F, Booz C, Kronfeld A, Sanner AP, Brockmann MA, Othman AE. Single-Energy Metal Artifact Reduction (SEMAR) in Ultra-High-Resolution CT Angiography of Patients with Intracranial Implants. Diagnostics (Basel) 2023;13:620. [Crossref] [PubMed]
- Puvanasunthararajah S, Fontanarosa D, Wille ML, Camps SM. The application of metal artifact reduction methods on computed tomography scans for radiotherapy applications: A literature review. J Appl Clin Med Phys 2021;22:198-223. [Crossref] [PubMed]
- Chen Z, Lei F, Ye F, Zhang H, Yuan H, Li S, Feng D. Prediction of Pedicle Screw Loosening Using an MRI-Based Vertebral Bone Quality Score in Patients with Lumbar Degenerative Disease. World Neurosurg 2023;171:e760-7. [Crossref] [PubMed]