Prediction of the stage shift growth of early-stage lung adenocarcinomas by volume-doubling time
Introduction
Lung cancer is currently the leading cause of cancer mortality globally, especially among men who are heavy smokers and Asian non-smoking women with late-diagnosed lung cancer (1-4). Owing to the worldwide implementation of low-dose chest computed tomography (CT) for lung cancer screening, the early diagnosis of lung cancer is clinically feasible (5-8). Relevant evidence has shown that prolonged lung cancer screening in high-risk groups can reduce mortality (9,10). However, screening has some disadvantages. Overdiagnosis is inevitable during the lung screening process (11,12). Previous studies have shown the widespread implementation of lung cancer screening programs in clinical practice, and the proportion of precancerous lesions has increased, particularly in Asian communities where non-smoking-related lung cancer (13,14). Therefore, active preoperative monitoring of the rate of precancerous lesions and the growth trend of subsolid nodules (SSNs) to guide appropriate surgical time is clinically important. Previous studies have demonstrated the interval growth of SSNs based on the average or the longest lengths of these nodules (15-17). In addition, Wu et al. also has demonstrated that combined clinical-radiomic model improved prediction in SSN interval growth in term of stage shift growth. However, the NELSON trial used volume doubling time (VDT) as a quantitative imaging biomarker to evaluate interval changes in indeterminate pulmonary nodules during the follow-up period (6). This study has significant clinical value, as the application of VDT to monitor tracked lung nodules reduces the rate of false positives. Owing to the heterogeneity of growth patterns in the lung adenocarcinoma spectrum lesions, predictors of interval growth that affect prognostic outcomes regarding stage shift are crucial in SNN management guidance. Preoperative prediction of the interval growth of these SSNs provides an important guide for clinical decision-making. To the best of our knowledge, no study has investigated whether VDT is an independent factor for predicting SSN interval growth in term of stage shift or whether its predictive power is better than that of traditional semantic methods such as nodular diameter or type.
The correct diagnosis of SSN growth patterns can effectively improve patient outcomes and prevent overmanagement (18). We mainly aimed to explore the potential of VDT in predicting interval growth and prognostic outcomes, focusing on the stage shift of SSNs in patients with lung adenocarcinomas. We present this article in accordance with the TRIPOD reporting checklist (available at https://qims.amegroups.com/article/view/10.21037/qims-23-1759/rc).
Methods
Patients
This retrospective analysis received approval from the Institutional Review Board of Kaohsiung Veterans General Hospital under the reference number KSVGH21-CT2-12. The study was conducted in accordance with the Declaration of Helsinki (as revised in 2013). The requirement for written informed consent was waived owing to the retrospective nature of this study. We consecutively retrieved patients with persistent pulmonary nodules identified during two rounds of chest CT between February 2012 and December 2021. The criteria for inclusion were as follows: (I) persistent SSNs confirmed on a series of chest CT scans with pathologic proof, (II) SSNs with a diameter <30 mm in the initial CT image, (III) at least two or more unenhanced thin-section (<2.5 mm) chest CT scans using the standard protocol at different time points and a follow-up interval period >1 year, and (IV) pathological outcomes of resected SSNs confirmed with adenocarcinoma spectrum. The exclusion criteria included SSNs lacking histopathological confirmation, insufficient follow-up (<2 CT scans, <1 year), and non-adenocarcinoma spectrum lesions. Totally, 132 patients with SSNs were included in this study. Of the participants, 50 were males and 82 were females. Clinical characteristics and radiological features including patient age, sex, initial nodule size, initial solid part size, nodular type according to SSN classification, follow-up duration, VDT categories, and pathological reports were collected. The study flowchart is shown in Figure 1.
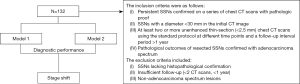
Qualitative and quantitative analysis of SSNs
CT scans were conducted using the following CT scanners: a 256-slice CT (Revolution CT, GE Healthcare, Milwaukee, USA), a 64-slice CT (Aquilion 64; Toshiba Medical Systems), and a 16-slice CT (Somatom Sensation, Siemens Healthcare, Germany). Scans spanning from the lung apex to the base were acquired at full inspiration without a contrast medium. Images were reconstructed with a slice thickness of 1–2.5 mm and displayed at a mediastinal window width of 350 Hounsfield units (HU) with a window level of 35 HU, lung window width of 1,600 HU, and window level of −600 HU. The characteristic CT features were retrospectively reviewed by an experienced thoracic radiologist (F.Z.W.) with 17 years of experience in chest radiology. The radiologist is blinded to the patients’ stage shift status. The imaging characteristics of each lesion confirmed by pathology were analyzed based on the following parameters: (I) size of the initial nodule along the long axis, (II) initial solid part (along the long axis) in the lung window, and (III) nodular type according to the Fleischer classification. Using a lung window setting, axial CT images were used to measure the maximum longest diameter of the primary nodular lesions and solid components.
Definition of SSN growth in terms of stage shift
For the enrolled 132 patients with SSNs, all available series of chest CT scans were evaluated to define stage shift. Stage shift was defined as a specific stage shift growth of lung adenocarcinoma spectrum lesions diagnosed to detect notable shifts in different categories or stages according to the eighth edition of the lung cancer tumor-node-metastasis (TNM) staging system, which is based on clinical and pathological information at the presentation of consecutive CT scans proposed by Wu et al. (Figure 2) (19). The definition of a stage shift is based on subclassification changes in the TNM staging system when tumor growth reaches a certain point. As per the eighth edition of the American Joint Committee on Cancer Staging System, subclassification changes are closely associated with the prognosis of cancer survival; hence, termed prognostic stage shifts (represented by red solid arrows). Diagnoses were made according to changes in TNM. Regarding T, when the tumor size changed from T1a–T1b, it was considered a subclassification change. For N, when the lymph node changed from negative to N2, it was considered a subclassification change. For M, we identified SSNs with a tumor size of <3 cm, and all cases were T(<3 cm)N0M0 in their baseline CT clinical staging. Subclassification changes were also considered present whether bone metastases or other signs of metastases were detected on the final CT scans or surgical outcomes. Finally, a summary was made according to the TNM status. Herein, any evolving modification within a subclassification was designated as a stage shift. The converse was also true; should there be no changes in tumor size, no stage shift (represented by grey dashed arrows) was defined as persistent non-invasion of the lymph nodes and an absence of signs of distant metastasis. As per “The new IASLC/ATS/ERS lung adenocarcinoma classification from a clinical perspective: current concepts and future prospects”, the imaging features that define atypical adenomatous hyperplasia (AAH), adenocarcinoma in situ (AIS), and minimally invasive adenocarcinoma (MIA) serve as a reference guideline (20). Additionally, in the stage shift (+) group, four patients were diagnosed with AIS lesions based on their baseline CT scans; however, they were confirmed as MIA lesions in their final surgical results. One case was diagnosed as an AAH lesion based on a baseline CT scan but was confirmed as an AIS lesion in the final surgical results. In this study, we defined these five lesions as stage shifts (+) because such pathological tissue growth changes could still affect survival prognosis (21,22). This study included patients with SSNs measuring <3 cm. A previous study has reported a low incidence (3.7%) of lymph node metastasis in a cohort with SNNs <3 cm (23). For patient-based analysis, patients with stable SSNs were classified into the stage shift (−) group (Figure 3). Patients with any SSNs showing stage shift growth were classified into the stage shift (+) group (Figure 4).
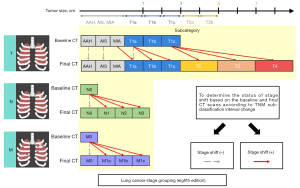
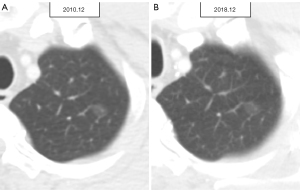
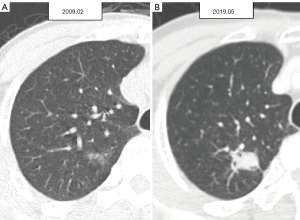
VDTs
For each SSN, two CT examinations were performed for the VDT analysis. The VDTs were calculated for SSNs with interval growth using a modified Schwartz formula (24). The volumes of the 132 SSNs were obtained using the LifeX package (LifeX freeware, version 5.10; Orsay, France, http://www.lifex soft.org) for nodular contour segmentation, with a volume of interest of ≥64 voxels for VDTs calculation (25). An experienced thoracic radiologist with 17 years of expertise manually outlined the contours of SSN. The regions of interest (ROIs) were manually delineated around the nodule boundary for each thin-slide CT section. Measurements were performed on non-contrast CT images using standard lung window settings (width, 1,600 HU; level, −600 HU). Once manual ROI segmentation of the pulmonary SSNs was completed, the VDT for the volume change of the lung nodules was calculated according to the following equation:
Where X2 and X1 denote the final and initial SSN volumes, respectively. ∆T (“delta T”) corresponds to the time (in d) between the two scans, and ln is the natural logarithm. The term “In” represents the natural logarithm (base “e”), while “In2” typically denotes the square of the natural logarithm. The VDT classification spans five levels, progressing from slow to rapid growth: VDT >1,600 d, VDT from 801–1,600 d, VDT from 401–800 d, VDT from 101–400 d, and VDT ≤100 d, respectively.
The utilization of a VDT cutoff value of 400 days for Kaplan-Meier analysis was based on findings from the NELSON study, which indicated that nodules with a VDT of ≥400 days at 3 months were classified as non-growing tumors {de Koning, 2020 #6}.
Statistical analysis
Statistical analyses were performed using SPSS software (version 22.0; SPSS Inc., Chicago, IL, USA) and Stata 13 (Version 13.1; StataCorp, College Station, TX, USA). Continuous variables are expressed as mean ± standard deviation, while categorical variables were summarized using frequencies and percentages and compared utilizing either the chi-square or Fisher’s exact test. Multivariate logistic regression was used to establish better diagnostic models for stage shift predictions in SSNs with and without VDT parameters. The results are expressed as odds ratios (ORs) with 95% confidence intervals (CIs). One nomogram was established to predict stage shifts with and without VDT. To compare the diagnostic performance of these prediction models, we constructed receiver operating characteristic (ROC) curves and calculated the areas under the curves (AUC). Additionally, positive predictive value, negative predictive value, sensitivity, specificity, positive likelihood ratio (LR+), and negative LR (LR−) were calculated to measure the overall diagnostic accuracy of these prediction models for stage shift (26). The Kaplan-Meier method and log-rank test were used to analyze the two groups according to the VDT class for stage shift status (27,28). Statistical techniques are available to analyze differences in AUC values obtained from ROC curves. DeLong’s test is employed for comparing AUC values derived from different models or predictors, facilitating rigorous assessment of performance variations. The statistical significance threshold for all tail-tailed tests was set at P<0.05. By summing the scores assigned on the point scale for each parameter, we could plot a straight line to determine the approximate individual probability score for stage shift status.
Results
Clinical characteristics and CT semantic features in SSNs
The clinical features and CT semantic parameters of 132 lung cancer patients with 132 SSNs at baseline CT are as follows: the initial mean nodule size was 1.21±0.953 cm, with a solid part size of 0.44±0.820 cm. Among these patients, 80 (60.61%) exhibited ground-glass nodules (GGNs) and 52 (39.39%) showed part-solid nodules (PSNs). After an average follow-up duration of 3.629 years, 43 (32.58%) individuals had GGNs and 89 (67.42%) had PSNs. Among the 44 patients experiencing a positive stage shift in the final evaluation, one manifested mediastinal lymph node metastasis, four showed distant organ metastases, and six displayed mediastinal lymph node and distant organ metastases.
For general characteristics and CT semantic features, no significant differences in age, sex ratio, initial nodule size, initial solid part size, and VDT category subclassification between the two groups were noted according to stage shift progression status. The stage shift progression (−) and progression (+) groups comprised 88 and 44 patients, respectively. The general characteristics and CT semantic features of the patients with lung cancer in the two groups are summarized in Table 1. A significant difference in the distribution of invasive pulmonary adenocarcinoma (IPA) lesions was observed between the two groups. Regarding the spectrum of lung adenocarcinoma lesions, IPA was significantly more prevalent in patients in the stage shift progression (+) group than that in the progression (−) group (88.6% vs. 52.3%; P=0.001). For SSN classification, the stage shift progression (+) group had a significantly greater proportion of GGNs than the (−) group (75% vs. 53.4%; P=0.017). The follow-up period in the stage shift progression (+) group was significantly longer than that in the (−) group (5.63±3.630 vs. 2.64±2.513 years, P<0.001).
Table 1
Characteristics | Stage shift progression (−) (n=88) | Stage shift progression (+) (n=44) | P value |
---|---|---|---|
Age (years) | 58.56±9.271 | 59.95±10.005 | 0.43 |
Gender (male) | 29 (33) | 21 (47.7) | 0.09 |
Initial nodule size (cm) | 1.12±0.794 | 1.34±1.188 | 0.27 |
Initial solid part size (cm) | 0.41±0.685 | 0.51±1.053 | 0.57 |
SSN classification | 0.02 | ||
GGN nodule | 47 (53.4) | 33 (75) | |
Part-solid nodule | 41 (46.6) | 11 (25) | |
Follow up period (years)* | 2.64±2.513 | 5.63±3.630 | <0.001 |
VDT classification (days)* | 0.07 | ||
>1,600 | 39 (44.3) | 10 (23.8) | |
801–1,600 | 14 (16.3) | 12 (28.6) | |
401–800 | 15 (17.4) | 13 (31) | |
101–400 | 15 (17.4) | 5 (11.9) | |
≤100 | 3 (3.5) | 2 (4.8) | |
132 SSNs with surgical proof of lung adenocarcinoma spectrum lesions | 0.001 | ||
AAH | 4 (4.5) | 0 (0) | |
AIS | 17 (19.3) | 1 (2.3) | |
MIA | 21 (23.9) | 4 (9.1) | |
IPA | 46 (52.3) | 39 (88.6) |
Data are expressed as n (%) or mean ± standard deviation. *, two cases in the stage shift progression (+) group were unable to undergo VDT measurement. CT, computed tomography; SSN, subsolid nodule; GGN, ground-glass nodule; VDT, volume doubling time; AAH, atypical adenomatous hyperplasia; AIS, adenocarcinoma in situ; MIA, minimally invasive adenocarcinoma; IPA, invasive pulmonary adenocarcinoma.
Risk model and nomogram establishment for stage shift prediction
Multivariate analyses of the characteristic parameters affecting stage shift prediction are summarized in Table 2. Model 1 included the clinical characteristics and CT semantic features for model development. Additionally, model 2 contained the clinical characteristics, CT semantic features, and parameters of the VDT classification for model development. In model 1, SSN classification (OR =0.046, 95% CI: 0.008–0.273) and the follow-up period (OR =1.4, 95% CI: 1.193–1.642) were significant predictors of a stage shift. In model 2, initial nodule size (OR =4.074, 95% CI: 1.368–12.135; P=0.012), SSN classification (OR =0.042; 95% CI: 0.006–0.288; P=0.001), follow-up period (OR =1.692, 95% CI: 1.337–2.140; P<0.001), and VDT classification (OR =2.327, 95% CI: 1.368–3.958; P=0.002) were significant predictors of stage shift. Table 3 shows the diagnostic AUC, sensitivity, and specificity values of the two models at the optimal probability score threshold for the stage shift prediction. The sensitivity of model 1 was 79.55%, and specificity was 75% for stage shift prediction. The sensitivity of model 2 was 76.19% and specificity was 87.21% for stage shift prediction. Among the two models, model 2 was the most specific for stage shift prediction. The areas under the ROC curves for stage shift prediction were 0.833 (95% CI: 0.757–0.893) for model 1 and 0.877 (95% CI: 0.807–0.928) for model 2. Compared with model 1, model 2 had the better predictive performance for stage shift prediction (AUC model 1: 0.833 versus AUC model 2: 0.877, P=0.047) after adding the parameter of VDT classification (Figure 5). The probability of stage shift status was used for nomogram development. A nomogram was generated to predict the stage shift status based on the multivariable logistic regression shown in Figure 6.
Table 2
Characteristics | Coefficient | OR | 95% CI | P value |
---|---|---|---|---|
Model 1 | ||||
Age (years) | 0.021 | 1.022 | 0.969–1.077 | 0.43 |
Gender (male) | 0.105 | 1.111 | 0.396–3.114 | 0.84 |
Initial nodule size (cm) | 0.868 | 2.383 | 0.923–6.150 | 0.07 |
Initial solid part size (cm) | 0.679 | 1.972 | 0.653–5.956 | 0.23 |
SSN classification | −3.071 | 0.046 | 0.008–0.273 | 0.001 |
Follow up period (years) | 0.336 | 1.4 | 1.193–1.642 | <0.001 |
Model 2 | ||||
Age (years) | 0.017 | 1.017 | 0.961–1.076 | 0.57 |
Gender (male) | 0.78 | 2.182 | 0.683–6.970 | 0.19 |
Initial nodule size (cm) | 1.405 | 4.074 | 1.368–12.135 | 0.012 |
Initial solid part size (cm) | 0.038 | 1.038 | 0.319–3.374 | 0.95 |
SSN classification | −3.169 | 0.042 | 0.006–0.288 | 0.001 |
Follow up period (years) | 0.526 | 1.692 | 1.337–2.140 | <0.001 |
VDT classification | 0.845 | 2.327 | 1.368–3.958 | 0.002 |
Model 1: clinical characteristics and CT semantic feature; model 2: clinical characteristics, CT semantic feature, and VDT classification. OR, odds ratio; CI, confidence interval; CT, computed tomography; VDT, volume doubling time; SSN, subsolid nodule.
Table 3
Prediction model | AUC (95% CI) | Cut-point | Sensitivity (95% CI) |
Specificity (95% CI) |
P value | Difference between areas | SE | 95% CI |
---|---|---|---|---|---|---|---|---|
Model 1 | 0.833 (0.757–0.893) |
>0.3169 | 79.55% (64.7–90.2%) |
75% (64.6–83.6%) |
<0.001 | – | – | – |
Model 2 | 0.877 (0.807–0.928) |
>0.4198 | 76.19% (60.5–87.9%) |
87.21% (78.3–93.4%) |
<0.001 | – | – | – |
Model 1 vs. model 2 | – | – | – | – | 0.047 | 0.0437 | 0.022 | 0.000632–0.0869 |
Model 1: clinical characteristics and CT semantic feature; model 2: clinical characteristics, CT semantic feature, and VDT classification. ROC, receiver operating characteristic; AUC, area under the curve; CI, confidence interval; SE, standard error; CT, computed tomography; VDT, volume doubling time.
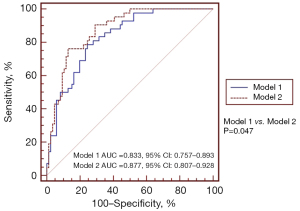
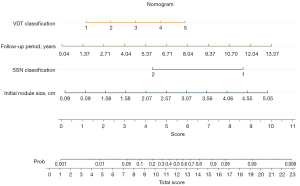
Influence of VDT on survival analysis for stage shift
Figure 7 depicts the Kaplan-Meier curves for stage shift in this cohort, based on the VDT (>400 d) and (≤400 d) groups. The 1-year stage shift rate of the VDT (≤400 d) group patients was 5%, which was significantly worse than that of the VDT (>400 d) group (0%). The 6-year stage shift rate of the VDT (≤400 d) group was 71.85%, which was significantly lower than that of the VDT (>400 d) group (35.49%). For the time estimate of stage shift, the mean progression time for the VDT (>400 d) group was 7.595 years and the median progression time was 7.430 years, whereas the VDT (≤400 d) group had a mean progression time of 3.250 years, and a median of 2.030 years. The log-rank test of these two groups revealed significant differences (P<0.0001; Figure 7). The distribution of VDTs according to the histologic subtypes of lung adenocarcinoma spectrum lesions is shown in Figure 8. The high proportion (100%) of patients with long VDT (d) seems to be particularly large among those with indolent AAH lesions. However, the proportion of patients with a short VDT dramatically increased for lung adenocarcinoma spectrum lesions, with a corresponding increase in the degree of invasion.
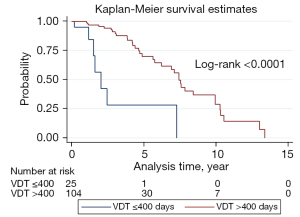
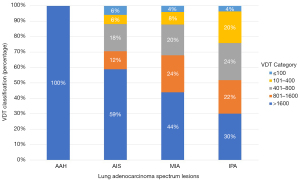
Discussion
In this study, a total of 132 SSNs underwent long-term follow-up chest CT examinations for an average duration of 3.629±3.231 years; the added value of VDT and semantic features part in predicting the stage shift of lung adenocarcinoma spectrum lesions were analyzed. Finally, we established one combined clinical-semantic-VDT model (model 2) that could better predict the status of stage-shift growth in lung adenocarcinoma spectrum lesions. Most prior studies have examined the factors that contribute to the risk of the interval growth pattern of GGNs or PSNs by tumor doubling time or an increase in diameter ≥2 mm to determine the aggressive behavior of pulmonary SSNs (29-32). This predictive nomogram, utilizing VDT parameters, represents the pioneering attempt to assess stage-shift growth in lung adenocarcinoma spectrum lesions initially appearing as SSNs within an Asian population. This study has three major findings. First, we created an innovative and valuable VDT-based nomogram prediction model that incorporated clinical characteristics, CT semantic features, and VDT parameters. This model effectively predicted stage shifts in lung adenocarcinoma spectrum lesions, demonstrating a strong performance in an Asian cohort. Second, compared with model 1, the innovative VDT-based model demonstrated a notably improved discriminatory capacity. Third, it has been demonstrated that VDT ≤400 d played an important role in aggressive growth behavior with stage shift status in SSNs in the follow-up period of 3.629±3.231 years, independent of clinical characteristics and CT semantic features.
Previous studies have shown that the VDT value is a crucial volumetric parameter primarily utilized in lung cancer screening and subsequent follow-up in the NELSON trial (6,33). Therefore, aggressive tumor growth can be differentiated from indolent lesions using the VDT values. Recent studies also reported that VDT varies significantly according to the pathological subtype of lung adenocarcinoma (34,35). Park et al. identified the VDT of <400 d as an independent risk factor for predicting poor disease-free survival in patients with lung adenocarcinoma (34). This explanation is consistent with previous research, indicating that VDT has a greater impact on tumor growth and prognostic outcomes in lung adenocarcinomas. Our study provides additional information for developing a better nomogram to predict the long-term natural course of SSN growth regarding stage shifts based on VDT parameters. Furthermore, VDT showed additional value for predicting stage shifts in SSNs when added to model 1. Thus, we believe that this novel nomogram/prediction model could be used to guide management and follow-up strategies for SSNs during extended follow-up periods when two series of thin-slice (≤2.5 mm) chest CT scans are available. Therefore, it is clinically important to detect SSNs with rapid growth patterns. In the future, personalized risk prediction models based on the clinical characteristics, the CT semantic features, VDT parameters, and radiomic features in high-risk Asian populations are clinically warranted and need to be externally validated (36).
Furthermore, the VDT <400 d group showed a notable association with a high rate of stage shift in SSNs over approximately 3.6 years. SSNs generally require a median follow-up of 7.430 and 2.030 years to grow in terms of stage shift in the VDT (>400 d) and VDT (≤400 d) groups, respectively. Therefore, these findings suggest a heterogeneous growth behavior for SSNs. Additionally, it is theoretically inaccurate to predict future growth trends based only on the baseline characteristics of SSNs. Furthermore, it is critical to monitor the interval growth of SSNs with prognostic outcomes in terms of stage shift more accurately, guided by a series of CT scan-derived VDT parameters. Previous systematic reviews have demonstrated that CT attenuation may be useful in predicting the natural growth of SSNs by defining growth as an increase in diameter of ≥2 mm (37). Few studies have addressed using texture or CT semantic characteristics to evaluate volumetric changes in the SSNs (38-40).
Therefore, this study is the first to demonstrate that a VDT of ≤400 d is a useful prognostic factor for stage shift prediction in the natural course of early lung adenocarcinomas.
As VDT is considered an important prognostic factor reflecting a stage shift, effective follow-up strategies according to the threshold of VDT are mandatory in personalized medicine. Considering these results, a 7-year follow-up period with annual or biennial intervals was necessary for the VDT group (>400 d). Prompt tissue proofing through surgical resection is indicated when SSNs exhibit obvious stage shifts. Regarding the VDT (≤400 d) group, most SSNs will eventually grow in terms of stage shift within 3 years. Based on these findings, it is recommended to conduct follow-up examinations for SSNs at 6-month intervals over a 3-year period. Therefore, this study is believed to have the greatest clinical value in demonstrating that using VDT to detect traced lung nodules can reduce false positives and overdiagnosis. The indications for watchful waiting with imaging or surgical management can be tailored based on risk stratification using VDT parameters (16,17,41). Treatment strategies should be individualized based on each patient’s clinical information, shared decision plans, and predicted probability (42-44).
Our study has a few limitations. First, this was a retrospective study designed at a single institution and included only surgical lung adenocarcinoma spectrum lesions. Second, the predictive model lacked validation data. Third, our study did not consider other potential factors that could influence tumor growth patterns and disease-free survival, such as molecular biomarkers. Future research incorporating these variables could provide a more comprehensive understanding of the prognostic factors in early lung adenocarcinoma. Hence, prospective studies are necessary to confirm the validity and reliability of our findings. A previous study demonstrated that a VDT of <400 d had a significant impact on disease-free survival in patients with lung cancer after complete resection (34). Our findings contribute additional value regarding tumor growth patterns, emphasizing the prognostic significance of stage shifts in early lung adenocarcinoma lesions. Fourth, a single radiologist conducted the measurements and ROI processes. However, this may have affected the consistency of the study and its external validation. In the future, the adoption of artificial intelligence for automated nodule analysis will optimize this process and reduce inconsistencies.
Conclusions
In summary, this study investigated the diagnostic utility of integrating CT features with VDT parameters in predicting stage shift of lung adenocarcinoma lesions. Our developed combined model offers improved predictive capability for assessing the growth rate of pulmonary SSNs, aiding in clinical decision-making.
Acknowledgments
The authors thank all radiologists at the hospitals for assisting with the collection of imaging data used in this study.
Funding: This study was supported by grants from
Footnote
Reporting Checklist: The authors have completed the TRIPOD reporting checklist. Available at https://qims.amegroups.com/article/view/10.21037/qims-23-1759/rc
Conflicts of Interest: All authors have completed the ICMJE uniform disclosure form (available at https://qims.amegroups.com/article/view/10.21037/qims-23-1759/coif). The authors have no conflicts of interest to declare.
Ethical Statement: The authors are accountable for all aspects of the work in ensuring that questions related to the accuracy or integrity of any part of the work are appropriately investigated and resolved. The study was conducted in accordance with the Declaration of Helsinki (as revised in 2013). This retrospective analysis received approval from the Institutional Review Board of Kaohsiung Veterans General Hospital under the reference number KSVGH21-CT2-12. The requirement for written informed consent was waived owing to the retrospective nature of this study.
Open Access Statement: This is an Open Access article distributed in accordance with the Creative Commons Attribution-NonCommercial-NoDerivs 4.0 International License (CC BY-NC-ND 4.0), which permits the non-commercial replication and distribution of the article with the strict proviso that no changes or edits are made and the original work is properly cited (including links to both the formal publication through the relevant DOI and the license). See: https://creativecommons.org/licenses/by-nc-nd/4.0/.
References
- Barbone F, Bovenzi M, Cavallieri F, Stanta G. Cigarette smoking and histologic type of lung cancer in men. Chest 1997;112:1474-9. [Crossref] [PubMed]
- Rahal Z, El Nemr S, Sinjab A, Chami H, Tfayli A, Kadara H. Smoking and Lung Cancer: A Geo-Regional Perspective. Front Oncol 2017;7:194. [Crossref] [PubMed]
- Siegel RL, Miller KD, Fuchs HE, Jemal A. Cancer statistics, 2022. CA Cancer J Clin 2022;72:7-33. [Crossref] [PubMed]
- Zhou F, Zhou C. Lung cancer in never smokers-the East Asian experience. Transl Lung Cancer Res 2018;7:450-63. [Crossref] [PubMed]
- Aberle DR, Adams AM, Berg CD, Black WC, Clapp JD, Fagerstrom RM, Gareen IF, Gatsonis C, Marcus PM, Sicks JD. Reduced lung-cancer mortality with low-dose computed tomographic screening. N Engl J Med 2011;365:395-409. [Crossref] [PubMed]
- de Koning HJ, van der Aalst CM, de Jong PA, Scholten ET, Nackaerts K, Heuvelmans MA, et al. Reduced Lung-Cancer Mortality with Volume CT Screening in a Randomized Trial. N Engl J Med 2020;382:503-13. [Crossref] [PubMed]
- Wu FZ, Huang YL, Wu CC, Tang EK, Chen CS, Mar GY, Yen Y, Wu MT. Assessment of Selection Criteria for Low-Dose Lung Screening CT Among Asian Ethnic Groups in Taiwan: From Mass Screening to Specific Risk-Based Screening for Non-Smoker Lung Cancer. Clin Lung Cancer 2016;17:e45-56. [Crossref] [PubMed]
- Nawa T, Nakagawa T, Mizoue T, Kusano S, Chonan T, Fukai S, Endo K. Long-term prognosis of patients with lung cancer detected on low-dose chest computed tomography screening. Lung Cancer 2012;75:197-202. [Crossref] [PubMed]
- Rota M, Pizzato M, La Vecchia C, Boffetta P. Efficacy of lung cancer screening appears to increase with prolonged intervention: results from the MILD trial and a meta-analysis. Ann Oncol 2019;30:1040-3. [Crossref] [PubMed]
- Pastorino U, Silva M, Sestini S, Sabia F, Boeri M, Cantarutti A, Sverzellati N, Sozzi G, Corrao G, Marchianò A. Prolonged lung cancer screening reduced 10-year mortality in the MILD trial: new confirmation of lung cancer screening efficacy. Ann Oncol 2019;30:1162-9. [Crossref] [PubMed]
- Detterbeck FC. Overdiagnosis during lung cancer screening: is it an overemphasised, underappreciated, or tangential issue? Thorax 2014;69:407-8. [Crossref] [PubMed]
- Yankelevitz DF, Henschke CI. Overdiagnosis in lung cancer screening. Transl Lung Cancer Res 2021;10:1136-40. [Crossref] [PubMed]
- Hung YC, Tang EK, Wu YJ, Chang CJ, Wu FZ. Impact of low-dose computed tomography for lung cancer screening on lung cancer surgical volume: The urgent need in health workforce education and training. Medicine (Baltimore) 2021;100:e26901. [Crossref] [PubMed]
- Kim HY, Jung KW, Lim KY, Lee SH, Jun JK, Kim J, Hwangbo B, Lee JS. Lung Cancer Screening with Low-Dose CT in Female Never Smokers: Retrospective Cohort Study with Long-term National Data Follow-up. Cancer Res Treat 2018;50:748-56. [Crossref] [PubMed]
- Hiramatsu M, Inagaki T, Inagaki T, Matsui Y, Satoh Y, Okumura S, Ishikawa Y, Miyaoka E, Nakagawa K. Pulmonary ground-glass opacity (GGO) lesions-large size and a history of lung cancer are risk factors for growth. J Thorac Oncol 2008;3:1245-50. [Crossref] [PubMed]
- Kakinuma R, Noguchi M, Ashizawa K, Kuriyama K, Maeshima AM, Koizumi N, Kondo T, Matsuguma H, Nitta N, Ohmatsu H, Okami J, Suehisa H, Yamaji T, Kodama K, Mori K, Yamada K, Matsuno Y, Murayama S, Murata K. Natural History of Pulmonary Subsolid Nodules: A Prospective Multicenter Study. J Thorac Oncol 2016;11:1012-28. [Crossref] [PubMed]
- Tang EK, Chen CS, Wu CC, Wu MT, Yang TL, Liang HL, Hsu HT, Wu FZ. Natural History of Persistent Pulmonary Subsolid Nodules: Long-Term Observation of Different Interval Growth. Heart Lung Circ 2019;28:1747-54. [Crossref] [PubMed]
- Ricciardi S, Booton R, Petersen RH, Infante M, Scarci M, Veronesi G, Cardillo G. Managing of screening-detected sub-solid nodules-a European perspective. Transl Lung Cancer Res 2021;10:2368-77. [Crossref] [PubMed]
- Wu FZ, Wu YJ, Chen CS, Tang EK. Prediction of Interval Growth of Lung Adenocarcinomas Manifesting as Persistent Subsolid Nodules ≤3 cm Based on Radiomic Features. Acad Radiol 2023;30:2856-69. [Crossref] [PubMed]
- Zugazagoitia J, Enguita AB, Nuñez JA, Iglesias L, Ponce S. The new IASLC/ATS/ERS lung adenocarcinoma classification from a clinical perspective: current concepts and future prospects. J Thorac Dis 2014;6:S526-36. [Crossref] [PubMed]
- Detterbeck FC. The eighth edition TNM stage classification for lung cancer: What does it mean on main street? J Thorac Cardiovasc Surg 2018;155:356-9.
- Lim W, Ridge CA, Nicholson AG, Mirsadraee S. The 8(th) lung cancer TNM classification and clinical staging system: review of the changes and clinical implications. Quant Imaging Med Surg 2018;8:709-18. [Crossref] [PubMed]
- Cho JY, Leem CS, Kim Y, Kim ES, Lee SH, Lee YJ, Park JS, Cho YJ, Lee JH, Lee CT, Yoon HI. Solid part size is an important predictor of nodal metastasis in lung cancer with a subsolid tumor. BMC Pulm Med 2018;18:151. [Crossref] [PubMed]
- SCHWARTZ M.. A biomathematical approach to clinical tumor growth. Cancer 1961;14:1272-94. [Crossref] [PubMed]
- Nioche C, Orlhac F, Boughdad S, Reuzé S, Goya-Outi J, Robert C, Pellot-Barakat C, Soussan M, Frouin F, Buvat I. LIFEx: A Freeware for Radiomic Feature Calculation in Multimodality Imaging to Accelerate Advances in the Characterization of Tumor Heterogeneity. Cancer Res 2018;78:4786-9. [Crossref] [PubMed]
- DeLong ER, DeLong DM, Clarke-Pearson DL. Comparing the areas under two or more correlated receiver operating characteristic curves: a nonparametric approach. Biometrics 1988;44:837-45.
- Altman DG, Bland JM. Time to event (survival) data. BMJ 1998;317:468-9. [Crossref] [PubMed]
- Bland JM, Altman DG. Survival probabilities (the Kaplan-Meier method). BMJ 1998;317:1572. [Crossref] [PubMed]
- Matsuguma H, Mori K, Nakahara R, Suzuki H, Kasai T, Kamiyama Y, Igarashi S, Kodama T, Yokoi K. Characteristics of subsolid pulmonary nodules showing growth during follow-up with CT scanning. Chest 2013;143:436-43. [Crossref] [PubMed]
- Kobayashi Y, Sakao Y, Deshpande GA, Fukui T, Mizuno T, Kuroda H, Sakakura N, Usami N, Yatabe Y, Mitsudomi T. The association between baseline clinical-radiological characteristics and growth of pulmonary nodules with ground-glass opacity. Lung Cancer 2014;83:61-6. [Crossref] [PubMed]
- Bak SH, Lee HY, Kim JH, Um SW, Kwon OJ, Han J, Kim HK, Kim J, Lee KS, Quantitative CT. Scanning Analysis of Pure Ground-Glass Opacity Nodules Predicts Further CT Scanning Change. Chest 2016;149:180-91. [Crossref] [PubMed]
- Borghesi A, Farina D, Michelini S, Ferrari M, Benetti D, Fisogni S, Tironi A, Maroldi R. Pulmonary adenocarcinomas presenting as ground-glass opacities on multidetector CT: three-dimensional computer-assisted analysis of growth pattern and doubling time. Diagn Interv Radiol 2016;22:525-33. [Crossref] [PubMed]
- Heuvelmans MA, Vliegenthart R, de Koning HJ, Groen HJM, van Putten MJAM, Yousaf-Khan U, Weenink C, Nackaerts K, de Jong PA, Oudkerk M. Quantification of growth patterns of screen-detected lung cancers: The NELSON study. Lung Cancer 2017;108:48-54. [Crossref] [PubMed]
- Park S, Lee SM, Kim S, Lee JG, Choi S, Do KH, Seo JB. Volume Doubling Times of Lung Adenocarcinomas: Correlation with Predominant Histologic Subtypes and Prognosis. Radiology 2020;295:703-12. [Crossref] [PubMed]
- Hong JH, Park S, Kim H, Goo JM, Park IK, Kang CH, Kim YT, Yoon SH. Volume and Mass Doubling Time of Lung Adenocarcinoma according to WHO Histologic Classification. Korean J Radiol 2021;22:464-75. [Crossref] [PubMed]
- Huang CY, Huang CC, Huang WM, Liang CH, Wu FZ. Letter to the Editor Regarding "Long-Term Follow-Up of Ground-Glass Nodules After 5 Years of Stability." by Lee et al., J Thorac Oncol 2019;14:1370-7. Heart Lung Circ 2020;29:e254-7. [Crossref] [PubMed]
- Gao C, Li J, Wu L, Kong D, Xu M, Zhou C. The Natural Growth of Subsolid Nodules Predicted by Quantitative Initial CT Features: A Systematic Review. Front Oncol 2020;10:318. [Crossref] [PubMed]
- Qi LL, Wu BT, Tang W, Zhou LN, Huang Y, Zhao SJ, Liu L, Li M, Zhang L, Feng SC, Hou DH, Zhou Z, Li XL, Wang YZ, Wu N, Wang JW. Long-term follow-up of persistent pulmonary pure ground-glass nodules with deep learning-assisted nodule segmentation. Eur Radiol 2020;30:744-55. [Crossref] [PubMed]
- Yoon HJ, Park H, Lee HY, Sohn I, Ahn J, Lee SH. Prediction of tumor doubling time of lung adenocarcinoma using radiomic margin characteristics. Thorac Cancer 2020;11:2600-9. [Crossref] [PubMed]
- Tan M, Ma W, Sun Y, Gao P, Huang X, Lu J, Chen W, Wu Y, Jin L, Tang L, Kuang K, Li M. Prediction of the Growth Rate of Early-Stage Lung Adenocarcinoma by Radiomics. Front Oncol 2021;11:658138. [Crossref] [PubMed]
- MacMahon H, Naidich DP, Goo JM, Lee KS, Leung ANC, Mayo JR, Mehta AC, Ohno Y, Powell CA, Prokop M, Rubin GD, Schaefer-Prokop CM, Travis WD, Van Schil PE, Bankier AA. Guidelines for Management of Incidental Pulmonary Nodules Detected on CT Images: From the Fleischner Society 2017. Radiology 2017;284:228-43. [Crossref] [PubMed]
- Wu FZ, Huang YL, Wu YJ, Tang EK, Wu MT, Chen CS, Lin YP. Prognostic effect of implementation of the mass low-dose computed tomography lung cancer screening program: a hospital-based cohort study. Eur J Cancer Prev 2020;29:445-51. [Crossref] [PubMed]
- Lowenstein LM, Godoy MCB, Erasmus JJ, Zirari Z, Bennett A, Leal VB, Housten AJ, Volk RJ. Implementing Decision Coaching for Lung Cancer Screening in the Low-Dose Computed Tomography Setting. JCO Oncol Pract 2020;16:e703-25. [Crossref] [PubMed]
- Wu YJ, Wu FZ, Yang SC, Tang EK, Liang CH. Radiomics in Early Lung Cancer Diagnosis: From Diagnosis to Clinical Decision Support and Education. Diagnostics (Basel) 2022;12:1064. [Crossref] [PubMed]