Quantitative susceptibility mapping of subcortical iron deposition in Parkinson disease and multiple system atrophy: clinical correlations and diagnostic implications
Introduction
Parkinson disease (PD) and multiple system atrophy (MSA) represent two prominent neurodegenerative parkinsonian disorders, with both being characterized by the aberrant accumulation of alpha-synuclein protein (1). PD, the more common of the two, predominantly manifests with motor symptoms such as bradykinesia, rigidity, and resting tremor. MSA, on the other hand, progresses more swiftly, characterized mainly by autonomic dysfunction and poor response to levodopa treatment (2). The clinical presentations of PD and MSA overlap to some degree, which significantly complicates their differential diagnosis.
From a pathological perspective, both PD and MSA involve disturbances in subcortical iron balance and oxidative stress, hinting at the potential involvement of iron dysregulation in their pathogenesis (3). The evolution of neuroimaging techniques, especially magnetic resonance imaging (MRI), has given rise to quantitative susceptibility mapping (QSM). QSM provides a noninvasive means to measuring brain iron deposition. Prior research has indicated increased iron accumulation in the substantia nigra in both patients with PD and those with MSA (4-6). Yet, the link between subcortical iron buildup and the various clinical features of these disorders is not fully understood.
Given this backdrop, our study aimed to achieve two main goals: first, to compare the iron levels in subcortical areas among patients with PD, patients with MSA, and healthy individuals; and second, to investigate how iron deposition correlates with the severity of both motor and nonmotor symptoms in PD and MSA. Based on existing literature and preliminary data, we hypothesized that the patient groups would display elevated iron concentrations in subcortical regions relative to controls. Furthermore, we anticipated there to be a correlation between clinical indices—both motor and nonmotor—and iron levels within specific anatomical networks.
In this context, we specifically targeted the bilateral substantia nigra pars compacta (SNc), substantia nigra pars reticulata (SNr), and globus pallidus internus (GPi) as our regions of interest (ROIs). This selection was predicated upon the hypothesis that the SNr and GPi, being areas implicated in later stages of PD progression (7,8), may exhibit differential involvement and extents of damage in MSA compared to PD. This could potentially explain the earlier impairment of motor circuits in MSA and its inconsistent response to Levodopa treatment, a key aspect of our investigation. We present this article in accordance with the STARD reporting checklist (available at https://qims.amegroups.com/article/view/10.21037/qims-24-168/rc).
Methods
Participants
This study included 26 patients with MSA [16 with parkinsonian type (MSA-P) and 10 with cerebellar type (MSA-C)], 40 patients with PD, and 35 healthy individuals, all of whom were from Xuanwu Hospital of Capital Medical University. Patients with MSA were diagnosed by expert neurologists according to the recognized “probable” MSA clinical criteria (9). Patients with PD had an established diagnosis according to the clinical diagnostic criteria of the Movement Disorder Society (MDS) (2). A minimum five-year clinical follow-up after report of initial motor symptoms was required for accurate diagnosis. Participants with MRI contraindications, neuropsychiatric comorbidities, or other neurodegenerative disorders or those unable to complete assessments were excluded. This study was conducted in accordance with the Declaration of Helsinki (as revised in 2013) and was approved by the ethics committee of Xuanwu Hospital of Capital Medical University (No. 2011-27). All participants provided written informed consent prior to participating in the study and undergoing MRI procedures.
Clinical assessments
Motor function was evaluated using the Unified Parkinson’s Disease Rating Scale Part III (UPDRS-III) (10). Nonmotor symptoms were assessed using the Non-Motor Symptoms Scale (NMSS) (11), the rapid-eye movement sleep behavior disorder questionnaire Hong Kong version (RBDQ-HK) (12), and the Hamilton Depression Scale (HAMD) (13). Higher scores indicated increased symptom severity. Cognitive abilities were assessed using the Montreal Cognitive Assessment (MoCA) test (14), in which lower scores denote cognitive decline.
MRI acquisition
Imaging was performed on a 3T Skyra scanner (Siemens Healthineers, Erlangen, Germany) with a 20-channel head–neck coil. Three-dimensional (3D) T1-weighted (T1w) magnetization prepared rapid gradient echo (MPRAGE) images were acquired under the following parameters: repetition time (TR) =2,530 ms, echo time (TE) =2.98 ms, inversion time (TI) =1,100 ms, flip angle =7°, field of view (FoV) = 256×224 mm2, voxel size =1×1×1 mm3, and scanning time =5 min and 13 s. QSM data were obtained using a 3D gradient echo (GRE) sequence under the following parameters: TR =25 ms, TE =17.5 ms, flip angle =15˚, FoV =256×192 mm2, voxel size =0.667×0.667×1.5 mm3, and scanning time =5 min and 6 s.
QSM processing
QSM maps were reconstructed from multiecho phase data using MATLAB 2014a (Mathworks, Natick, MA, USA) based on STI Suite software (STI Suite: https://people.eecs.berkeley.edu/~chunlei.liu/software.html). The multiple-phase images were unwrapped by a Laplacian-based algorithm method and used to remove the background field via the V-sophisticated harmonic artifact reduction for phase data (V-SHARP) method. The magnetic susceptibility was measured using streaking artifact reduction for QSM (STAR-QSM). The bilateral SNc, SNr, and GPi were defined as ROIs based on the California Institute of Technology’s 168 atlas of subcortical nuclei in Montreal Neurological Institute (MNI) 152 space. Image registration was performed using FMRIB Software Library (FSL) v. 6.0 (https://fsl.fmrib.ox.ac.uk/fsl/fslwiki/). Individual 3D T1w imaging was first skull stripped and registered to the GRE magnitude image using the FMRIB’s linear image registration tool (FLIRT) tool to acquire the first warping field. Subsequently, the 3D-T1w image was registered to standard MNI 152 space using the FLIRT and FMRIB’s nonlinear image registration tool (FNIRT), and the warping field from individual T1w image to standard space was inverted to acquire the third warping field. The first and third warping fields were combined to obtain the fourth warping field for converting MNI 152 space to an individual susceptibility map. Finally, the ROIs in the MNI 152 space were registered to individual susceptibility maps and used to calculate QSM values (Figure 1).
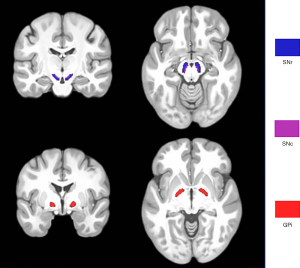
Statistical analysis
For the analysis of group differences in QSM values, three specific ROIs—the SNc, SNr, and GPi—were scrutinized. We first performed intergroup comparisons between the two MSA subtypes, cerebellar (C-type) and parkinsonian (P-type), using nonparametric Mann-Whitney tests. If differences were found, further analyses would be conducted by comparing each subtype separately with the PD and healthy control (HC) groups. If no differences were found, the subtypes would be combined into an MSA group for analysis. One-way analysis of variance (ANOVA) was performed to assess intergroup differences among the MSA, PD, and HC groups. If the ANOVA indicated significant differences, post hoc tests, including Tukey or Bonferroni, would be employed to identify the specific groups that differed. Multiple comparison corrections were applied to adjust the P values. To clarify the associations between QSM values and clinical scores, Spearman rank-order correlation and Pearson correlation coefficient were implemented. The clinical evaluation scales considered in the study included the HAMD, MoCA, NMSS, RBDQ-HK, and UPDRS-III. Analyses were conducted separately for the MSA and PD cohorts. P<0.05 was considered statistically significant.
An extended analysis of covariance (ANCOVA) was employed to assess the impact of specific covariates—age, gender, duration of the disease, and educational level—on QSM values within the MSA and PD groups. The level of significance for each covariate was set to P<0.05.
In our analysis, the discriminative capacity of the individual and combined nuclei values (SNc, SNr, and GPi) for distinguishing MSA from HC, MSA from PD, and PD from HC was evaluated. Initially, receiver operating characteristic (ROC) curves were generated based on individual nuclei values, with their performance being quantified using the area under the curve (AUC). Subsequently, logistic regression models were employed, with these nuclei combinations being the input features. By splitting the data into training and test sets, we assessed the model’s performance on unseen data. These models yielded probability scores, from which ROC curves were drawn and AUC values computed, offering insights into the optimal nuclei combinations for patient differentiation.
In this study, the analysts of the QSM data were blinded to the clinical information of participants, and similarly, the assessors of the reference standard were not informed of the index test results. This dual-blinding approach was adopted to prevent bias and ensure objective data interpretation and a robust and accurate evaluation, focused solely on imaging findings. We addressed indeterminate results from both the index test and the reference standard systematically. Any ambiguous or unclear results were reviewed by a panel of experts to reach a consensus. Missing data in the index test or reference standard were managed by the imputation of average values, with a focus on maintaining the consistency and completeness of the dataset.
Results
Demographics
The cohort consisted of 26 patients diagnosed with MSA, of whom 16 had the parkinsonian subtype (MSA-P) and 10 had the cerebellar subtype (MSA-C). Additionally, 40 patients with PD and 35 HC were included for comparison. No statistically significant differences were observed among the two types of MSA in terms of age (P=0.847), gender (P=0.422), or QSM value of the three ROIs (SNc: P=0.257; SNr: P=0.356; GPi: P=0.772). The subtypes would be combined into an MSA group for analysis.
No significant differences were found among the three groups concerning age, gender distribution, or educational level (al P values >0.05). The median duration of illness was longer in patients with PD [3 years, interquartile range (IQR) 3 years] than in patients with MSA (2 years, IQR 2.3 years), with a P value of 0.027 indicating statistical significance (Table 1).
Table 1
Parameter/characteristic | MSA (n=26) | PD (n=40) | HC (n=35) | P value |
---|---|---|---|---|
Age (years) | 57.5±10.5 | 60±10.6 | 61±10 | 0.308 |
Gender (M/F), n | 12/14 | 21/19 | 16/19 | 0.810 |
Years of education | 10.5±6 | 10.5±5.8 | 11±3 | 0.975 |
Duration (years) | 2±2.3 | 3±3 | – | 0.027 |
MoCA | 24.5±7 | 24.5±5 | 25±5 | 0.139 |
UPDRS-III | 40±20 | 26±19 | – | 0.001 |
RBDQ-HK | 25.5±12 | 15±17 | 8±9 | 0.000 |
NMSS | 55±20 | 19.5±24 | 8±14 | 0.000 |
HAMD | 9±4 | 4.5±5 | 2±4 | 0.000 |
Data are presented as the median ± interquartile range. MSA, multiple system atrophy; PD, Parkinson disease; HC, heathy control; M/F, male/female; MoCA, Montreal Cognitive Assessment; UPDRS-III, Unified Parkinson’s Disease Rating Scale Part III; RBDQ-HK, rapid-eye movement sleep behavior disorder questionnaire-Hong Kong; NMSS, Non-Motor Symptoms Scale; HAMD, Hamilton Depression Rating Scale.
QSM analysis
In the assessment of QSM measurements across the SNc, SNr, and GPi regions, significant group differences were noted. Although the SNc region did not demonstrate any significant difference between MSA and PD (U=588; P=0.376), the SNr region did (U=693; P=0.024). The GPi region exhibited a difference, but this was not significant (U=669; P=0.051). Both the SNc (U=759; P<0.00001) and SNr (U=823; P<0.00001) regions showed highly significant differences between the MSA and HC groups, while the GPi region showed a significant difference (U=714; P<0.0001). Finally the PD and HC groups were significantly different in the SNc (U=1,149; P<0.00001), SNr (U=1,076; P<0.0001), and GPi (U=984; P=0.0026) regions (Figure 2).
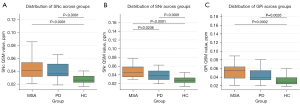
Correlations
For MSA, a significantly positive correlation was identified between iron content in SNc and NMSS scores (r=0.4; P=0.043). However, no such associations were found with the UPDRS-III, HAMD, or RBDQ-HK scores (all P values >0.05) (Figure 3A).
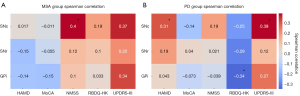
For PD, there was a positive correlation of SNc iron levels with UPDRS-III (r=0.395; P=0.012) and HAMD score (r=0.313; P=0.049). Moreover, iron content in the GPi negatively correlated with RBDQ-HK score (r=−0.342; P=0.031), but there were no significant correlation with NMSS score (P>0.05) (Figure 3B).
The ANCOVA results indicated that none of the considered covariates (age, gender, duration, education) had a significant association on the SNc, SNr, and GPi measures in either the MSA or PD groups.
Sensitivity and specificity analysis
In distinguishing MSA from PD, SNr had the highest AUC of 0.67, indicating good discriminative power, which was followed by GPi (AUC =0.64) and SNc (AUC =0.57) (Figure 4A). In differentiating MSA from HC, SNr showcased had the highest AUC of 0.90, followed by SNc at 0.83 and GPi at 0.78 (Figure 4B). In differentiating between patients with PD and HCs, SNc and SNr exhibited AUCs of 0.82 and 0.77 respectively, suggesting potential utility, whereas GPi (AUC =0.70) appeared less promising (Figure 4C). Both the paired and triplet nuclei combinations yielded AUC values less than 0.8. Moreover, their discriminative capacities were inferior to that of the individual nuclei, suggesting a more pronounced differentiation potential when nuclei were assessed independently.
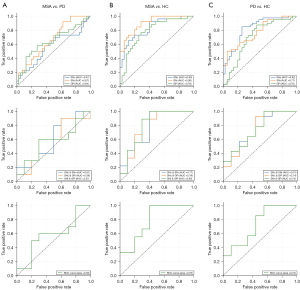
Discussion
In this study, no significant differences in age, gender, or QSM measurements from the SNc, SNr, and GPi regions were observed between the MSA subtypes. A comparative analysis between MSA and PD revealed notable disparities in the SNr but not in the SNc. In MSA, a clear correlation emerged between iron levels in the SNc and NMSS scores. For PD participants, SNc iron concentrations positively correlated with UPDRS-III and HAMD scores, whereas an inverse relationship was noted between GPi iron levels and RBDQ-HK scores. ANCOVA results suggested a minimal influence of evaluated covariates on QSM readings in both the MSA and PD groups. In differentiating MSA from PD, the SNr emerged as the most discriminative region, followed by the GPi and SNc.
Previous studies have demonstrated links between abnormal iron regulation, ferroptosis, and PD pathogenesis. Iron accumulation and dysregulation of iron metabolism proteins such as transferrin receptor 2 (TfR2), divalent metal transporter 1 (DMT1), and ferroportin (FPN-1) are observed in the SN of patients with PD (15-17). Iron buildup may promote ferroptosis through reactive oxygen species (ROS) generation via Fenton reactions (18). Ferroptosis inhibitors such as ferrostatin-1 have shown promise in mitigating dopaminergic neuronal death in PD models (19,20). Additional evidence indicates that α-synuclein aggregation and dopamine depletion in PD may increase susceptibility to iron-dependent ferroptotic cell death (21,22). Moreover, abnormal iron deposition, which are significant iron stores in the brain, have been observed in oligodendrocytes (23), and it has been suggested that iron may play a critical role in alpha-synuclein aggregation (24). Dysregulation of iron homeostasis can also markedly impact oxidative stress responses in oligodendrocytes, which may ultimately precipitate neurodegenerative changes (25).
Previous studies have reported increased magnetic susceptibility in the SN of patients with MSA compared to that of controls (26-29) but no significant changes in the GP (28). Our study aligns with these findings, suggesting iron accumulation, α-synuclein deposition, and glial dysfunction in MSA. Interestingly, our results also revealed increased GPi susceptibility in patients with MSA compared to controls, possibly reflecting a more advanced disease stage.
In most studies on PD, including ours, increased SNc susceptibility has been observed (7,30,31) although some discrepancies exist (32), and SNr and GPi findings vary across studies (7,8,33). Guan et al. (8) noted early-stage PD affecting iron deposition in the SNc, with late-stage PD impacting the SNr and GPi.
Direct comparisons of QSM values between MSA and PD are scarce. Mazzuchelli et al. found that the susceptibility of the SN is higher in those with MSA versus those with PD. However, we did not find significant differences, possibly since disease stage in MSA may not only be associated with the UPDRS but also Unified Multiple System Atrophy Rating Scale (UMSARS), nonmotor symptoms, and disease duration. The younger age and shorter disease duration of patients with MSA in this study may explain the lack of differences in QSM in the SN of patients with MSA compared to patients with PD (28). Previously collected evidence also suggests greater iron accumulation in the putamen of patients with MSA-P compared to those with PD (28), warranting further study in this area. However, some studies have reported there to be differences in the GP between patients with MSA and those with PD (27,28), which is consistent with our results, as the GP is typically affected in the later stage of both diseases. Different stages and subtypes of the disease may exhibit different QSM values in the GP. Conversely, a significant difference being observed in the SNr region underscores its potential as a distinguishing factor in the pathology of MSA compared to PD, possibly reflecting the distinct progression mechanisms and the later-stage involvement in PD. This observation is particularly intriguing, as it supports our initial hypothesis that the SNr, alongside the GPi—despite its nonsignificant P value—may exhibit unique patterns of involvement in MSA that could underlie the distinct clinical manifestations and poorer levodopa response observed in this condition.
These links discovered between iron levels and clinical scores can potentially connect neuroimaging findings with clinical symptoms. In MSA cases, the positive correlation between SNc iron content and NMSS scores ties nigral iron accumulation to the worsening of autonomic dysfunction, a key characteristic of MSA (34). In contrast, for PD, the relationship between SNc iron levels and both UPDRS-III and HAMD scores suggests iron’s role in influencing motor functions and mood disorders (26). Interestingly, a previous study noted that in PD, QSM values for various brain regions, including the SNc, SNr, and GP, did not linearly correlate with the The Korean version of the NMS scale (K-NMSS) K-NMSS total score (32), a finding echoed in our research. In evaluating the association between MoCA scores and susceptibility values in neurodegenerative disorders, our study specifically investigated the SNc, SNr, and GPi nuclei in PD and patients with MSA. Unlike prior studies indicating negative correlations between cognitive scores and susceptibility in regions such as the caudate head and cuneus (35,36), our study did not observe significant correlations within these nuclei. This suggests a nuanced role of iron deposition in cognitive impairment across different brain regions and underscores the heterogeneity of cognitive decline mechanisms in neurodegenerative diseases. Our findings prompt further research for elucidating these relationships that may potentially influence future therapeutic strategies.
Our ROC curve analysis highlights the potential of QSM values as biomarkers in neurodegenerative diseases. The SNc’s ability to differentiate MSA from HC positions it as a promising biomarker. In contrast, the GPi’s lower AUC values in distinguishing PD from HC suggest its limited effectiveness in this role. The utility of QSM values from individual nuclei in differentiating MSA, PD, and HC appears limited. An integrated approach, combining QSM data with other clinical parameters or multimodal MRI sequences, might enhance diagnostic precision.
Although our findings are insightful, we recognize certain limitations to our study. The sample size, albeit sufficient, could be larger in future studies to confirm our conclusions. Longitudinal research is necessary to monitor the progression of iron accumulation and its association with clinical symptoms over time. Moreover, our study lacked pathological verification of the imaging findings across various disease stages, and we did not consider the impact of medications on clinical metrics. Future research should include larger, multisite studies with autopsy correlations to build upon these initial observations.
In summary, our study highlights QSM’s potential as a diagnostic tool for distinguishing between MSA and PD. The correlations we observed between iron content and clinical scores offer a pathway for future investigations to examine the mechanism underlying the relationship between iron dysregulation and the progression of neurodegenerative disorders.
Acknowledgments
We would like to express our deepest gratitude to all the participants and their families, who generously contributed their time and effort to this study despite the challenges posed by Parkinson’s Disease and Multiple System Atrophy. Our sincere thanks also go to the dedicated team of neurologists and radiologists for their invaluable expertise and commitment to the meticulous execution of this study.
Funding: This work was supported by
Footnote
Reporting Checklist: The authors have completed the STARD reporting checklist. Available at https://qims.amegroups.com/article/view/10.21037/qims-24-168/rc
Conflicts of Interest: All authors have completed the ICMJE uniform disclosure form (available at https://qims.amegroups.com/article/view/10.21037/qims-24-168/coif). The authors have no conflicts of interest to declare.
Ethical Statement: The authors are accountable for all aspects of the work in ensuring that questions related to the accuracy or integrity of any part of the work are appropriately investigated and resolved. This study was conducted in accordance with the Declaration of Helsinki (as revised in 2013) and was approved by the ethics committee of Xuanwu Hospital of Capital Medical University (No. 2011-27). All participants provided written informed consent prior to participating in the study and undergoing MRI procedures.
Open Access Statement: This is an Open Access article distributed in accordance with the Creative Commons Attribution-NonCommercial-NoDerivs 4.0 International License (CC BY-NC-ND 4.0), which permits the non-commercial replication and distribution of the article with the strict proviso that no changes or edits are made and the original work is properly cited (including links to both the formal publication through the relevant DOI and the license). See: https://creativecommons.org/licenses/by-nc-nd/4.0/.
References
- Hughes AJ, Lees AJ, Stern GM. Apomorphine test to predict dopaminergic responsiveness in parkinsonian syndromes. Lancet 1990;336:32-4. [Crossref] [PubMed]
- Postuma RB, Berg D, Stern M, Poewe W, Olanow CW, Oertel W, Obeso J, Marek K, Litvan I, Lang AE, Halliday G, Goetz CG, Gasser T, Dubois B, Chan P, Bloem BR, Adler CH, Deuschl G. MDS clinical diagnostic criteria for Parkinson's disease. Mov Disord 2015;30:1591-601. [Crossref] [PubMed]
- Ward RJ, Zucca FA, Duyn JH, Crichton RR, Zecca L. The role of iron in brain ageing and neurodegenerative disorders. Lancet Neurol 2014;13:1045-60. [Crossref] [PubMed]
- Horowitz MP, Greenamyre JT. Mitochondrial iron metabolism and its role in neurodegeneration. J Alzheimers Dis 2010;20:S551-68. [Crossref] [PubMed]
- Ostrerova-Golts N, Petrucelli L, Hardy J, Lee JM, Farer M, Wolozin B. The A53T alpha-synuclein mutation increases iron-dependent aggregation and toxicity. J Neurosci 2000;20:6048-54. [Crossref] [PubMed]
- Yan Y, Wang Z, Wei W, Yang Z, Guo L, Wang Z, Wei X. Correlation of brain iron deposition and freezing of gait in Parkinson's disease: a cross-sectional study. Quant Imaging Med Surg 2023;13:7961-72. [Crossref] [PubMed]
- Chen Q, Chen Y, Zhang Y, Wang F, Yu H, Zhang C, Jiang Z, Luo W. Iron deposition in Parkinson's disease by quantitative susceptibility mapping. BMC Neurosci 2019;20:23. [Crossref] [PubMed]
- Guan X, Xuan M, Gu Q, Huang P, Liu C, Wang N, Xu X, Luo W, Zhang M. Regionally progressive accumulation of iron in Parkinson's disease as measured by quantitative susceptibility mapping. NMR Biomed 2017;30: [Crossref] [PubMed]
- Wenning GK, Stankovic I, Vignatelli L, Fanciulli A, Calandra-Buonaura G, Seppi K, et al. The Movement Disorder Society Criteria for the Diagnosis of Multiple System Atrophy. Mov Disord 2022;37:1131-48. [Crossref] [PubMed]
- Goetz CG, Tilley BC, Shaftman SR, Stebbins GT, Fahn S, Martinez-Martin P, et al. Movement Disorder Society-sponsored revision of the Unified Parkinson's Disease Rating Scale (MDS-UPDRS): scale presentation and clinimetric testing results. Mov Disord 2008;23:2129-70. [Crossref] [PubMed]
- Sauerbier A, Jitkritsadakul O, Titova N, Klingelhoefer L, Tsuboi Y, Carr H, Kumar H, Banerjee R, Erro R, Bhidayasiri R, Schrag A, Zis P, Lim SY, Al-Hashel JY, Kamel WA, Martinez-Martin P, Ray Chaudhuri K. Non-Motor Symptoms Assessed by Non-Motor Symptoms Questionnaire and Non-Motor Symptoms Scale in Parkinson's Disease in Selected Asian Populations. Neuroepidemiology 2017;49:1-17. [Crossref] [PubMed]
- Li SX, Wing YK, Lam SP, Zhang J, Yu MW, Ho CK, Tsoh J, Mok V. Validation of a new REM sleep behavior disorder questionnaire (RBDQ-HK). Sleep Med 2010;11:43-8. [Crossref] [PubMed]
- HAMILTON M.. A rating scale for depression. J Neurol Neurosurg Psychiatry 1960;23:56-62. [Crossref] [PubMed]
- Lu J, Li D, Li F, Zhou A, Wang F, Zuo X, Jia XF, Song H, Jia J. Montreal cognitive assessment in detecting cognitive impairment in Chinese elderly individuals: a population-based study. J Geriatr Psychiatry Neurol 2011;24:184-90. [Crossref] [PubMed]
- Mastroberardino PG, Hoffman EK, Horowitz MP, Betarbet R, Taylor G, Cheng D, Na HM, Gutekunst CA, Gearing M, Trojanowski JQ, Anderson M, Chu CT, Peng J, Greenamyre JT. A novel transferrin/TfR2-mediated mitochondrial iron transport system is disrupted in Parkinson's disease. Neurobiol Dis 2009;34:417-31. [Crossref] [PubMed]
- Rhodes SL, Buchanan DD, Ahmed I, Taylor KD, Loriot MA, Sinsheimer JS, Bronstein JM, Elbaz A, Mellick GD, Rotter JI, Ritz B. Pooled analysis of iron-related genes in Parkinson's disease: association with transferrin. Neurobiol Dis 2014;62:172-8. [Crossref] [PubMed]
- Yanatori I, Kishi F. DMT1 and iron transport. Free Radic Biol Med 2019;133:55-63. [Crossref] [PubMed]
- Ferreira A, Neves P, Gozzelino R. Multilevel Impacts of Iron in the Brain: The Cross Talk between Neurophysiological Mechanisms, Cognition, and Social Behavior. Pharmaceuticals (Basel) 2019;12:126. [Crossref] [PubMed]
- Kabiraj P, Valenzuela CA, Marin JE, Ramirez DA, Mendez L, Hwang MS, Varela-Ramirez A, Fenelon K, Narayan M, Skouta R. The neuroprotective role of ferrostatin-1 under rotenone-induced oxidative stress in dopaminergic neuroblastoma cells. Protein J 2015;34:349-58. [Crossref] [PubMed]
- Sun Y, He L, Wang T, Hua W, Qin H, Wang J, Wang L, Gu W, Li T, Li N, Liu X, Chen F, Tang L. Activation of p62-Keap1-Nrf2 Pathway Protects 6-Hydroxydopamine-Induced Ferroptosis in Dopaminergic Cells. Mol Neurobiol 2020;57:4628-41. [Crossref] [PubMed]
- Deas E, Cremades N, Angelova PR, Ludtmann MH, Yao Z, Chen S, Horrocks MH, Banushi B, Little D, Devine MJ, Gissen P, Klenerman D, Dobson CM, Wood NW, Gandhi S, Abramov AY. Alpha-Synuclein Oligomers Interact with Metal Ions to Induce Oxidative Stress and Neuronal Death in Parkinson's Disease. Antioxid Redox Signal 2016;24:376-91. [Crossref] [PubMed]
- Wang D, Peng Y, Xie Y, Zhou B, Sun X, Kang R, Tang D. Antiferroptotic activity of non-oxidative dopamine. Biochem Biophys Res Commun 2016;480:602-7. [Crossref] [PubMed]
- Dexter DT, Jenner P, Schapira AH, Marsden CD. Alterations in levels of iron, ferritin, and other trace metals in neurodegenerative diseases affecting the basal ganglia. The Royal Kings and Queens Parkinson's Disease Research Group. Ann Neurol 1992;32:S94-100. [Crossref] [PubMed]
- Levin J, Högen T, Hillmer AS, Bader B, Schmidt F, Kamp F, Kretzschmar HA, Bötzel K, Giese A. Generation of ferric iron links oxidative stress to α-synuclein oligomer formation. J Parkinsons Dis 2011;1:205-16. [Crossref] [PubMed]
- Stefanova N, Wenning GK. Multiple system atrophy: at the crossroads of cellular, molecular and genetic mechanisms. Nat Rev Neurosci 2023;24:334-46. [Crossref] [PubMed]
- Sjöström H, Granberg T, Westman E, Svenningsson P. Quantitative susceptibility mapping differentiates between parkinsonian disorders. Parkinsonism Relat Disord 2017;44:51-7. [Crossref] [PubMed]
- Ito K, Ohtsuka C, Yoshioka K, Kameda H, Yokosawa S, Sato R, Terayama Y, Sasaki M. Differential diagnosis of parkinsonism by a combined use of diffusion kurtosis imaging and quantitative susceptibility mapping. Neuroradiology 2017;59:759-69. [Crossref] [PubMed]
- Mazzucchi S, Frosini D, Costagli M, Del Prete E, Donatelli G, Cecchi P, Migaleddu G, Bonuccelli U, Ceravolo R, Cosottini M. Quantitative susceptibility mapping in atypical Parkinsonisms. Neuroimage Clin 2019;24:101999. [Crossref] [PubMed]
- Sugiyama A, Sato N, Kimura Y, Fujii H, Maikusa N, Shigemoto Y, Suzuki F, Morimoto E, Koide K, Takahashi Y, Matsuda H, Kuwabara S. Quantifying iron deposition in the cerebellar subtype of multiple system atrophy and spinocerebellar ataxia type 6 by quantitative susceptibility mapping. J Neurol Sci 2019;407:116525. [Crossref] [PubMed]
- Guan X, Zhang Y, Wei H, Guo T, Zeng Q, Zhou C, Wang J, Gao T, Xuan M, Gu Q, Xu X, Huang P, Pu J, Zhang B, Liu C, Zhang M. Iron-related nigral degeneration influences functional topology mediated by striatal dysfunction in Parkinson's disease. Neurobiol Aging 2019;75:83-97. [Crossref] [PubMed]
- Ahmadi SA, Bötzel K, Levin J, Maiostre J, Klein T, Wein W, Rozanski V, Dietrich O, Ertl-Wagner B, Navab N, Plate A. Analyzing the co-localization of substantia nigra hyper-echogenicities and iron accumulation in Parkinson's disease: A multi-modal atlas study with transcranial ultrasound and MRI. Neuroimage Clin 2020;26:102185. [Crossref] [PubMed]
- Shin C, Lee S, Lee JY, Rhim JH, Park SW. Non-Motor Symptom Burdens Are Not Associated with Iron Accumulation in Early Parkinson's Disease: a Quantitative Susceptibility Mapping Study. J Korean Med Sci 2018;33:e96. [Crossref] [PubMed]
- Du G, Lewis MM, Sica C, He L, Connor JR, Kong L, Mailman RB, Huang X. Distinct progression pattern of susceptibility MRI in the substantia nigra of Parkinson's patients. Mov Disord 2018;33:1423-31. [Crossref] [PubMed]
- An H, Zeng X, Niu T, Li G, Yang J, Zheng L, Zhou W, Liu H, Zhang M, Huang D, Li J. Quantifying iron deposition within the substantia nigra of Parkinson's disease by quantitative susceptibility mapping. J Neurol Sci 2018;386:46-52. [Crossref] [PubMed]
- Uchida Y, Kan H, Sakurai K, Inui S, Kobayashi S, Akagawa Y, Shibuya K, Ueki Y, Matsukawa N. Magnetic Susceptibility Associates With Dopaminergic Deficits and Cognition in Parkinson's Disease. Mov Disord 2020;35:1396-405. [Crossref] [PubMed]
- Uchida Y, Kan H, Sakurai K, Arai N, Kato D, Kawashima S, Ueki Y, Matsukawa N. Voxel-based quantitative susceptibility mapping in Parkinson's disease with mild cognitive impairment. Mov Disord 2019;34:1164-73. [Crossref] [PubMed]