A new computed tomography score—based staging for melioidosis pneumonia to predict progression
Introduction
Melioidosis pneumonia is an infectious pneumonia caused by Burkholderia pseudomallei (1). Melioidosis has a fatality rate of 10–50% (2), with some models suggesting that 89,000 related deaths occur worldwide each year (3). Patients with acute melioidosis pneumonia are at a higher risk of septic shock, acute respiratory distress syndrome, and death (1,4).
The scientific staging of melioidosis pneumonia can provide guidance for prognosis, and the imaging manifestations are direct evidence of progression and play a central role in the diagnosis and management of pneumonia. The typical imaging features of melioidosis pneumonia are rapid lung disease progression with disseminated nodules and cavities, and patients with melioidosis pneumonia are at an increased risk of multiple organ dysfunction syndrome, which is a major factor leading to poorer clinical outcomes (5,6). Computed tomography (CT) is the most suitable technology for displaying and quantifying of the relevant details, such as early abscesses and cavities in the lungs, that are the main factors affecting severe or critical diseases (7,8). At present, the commonly used clinical staging of melioidosis pneumonia is based primarily on the clinical symptoms and signs of patients (9,10). However, this method lacks key parameters related to clinical outcomes, and providing reliable and valuable information for further diagnosis and treatment plans can be difficult under this clinical staging system. Therefore, there is an urgent need to develop a scientifically informed staging system for melioidosis pneumonia based on CT imaging and clinical outcomes.
In this study, we aimed to develop a new CT score as an important marker for predicting the progression of melioidosis pneumonia. Using multicenter melioidosis pneumonia data, we sought to evaluate a CT score—based staging system for predicting the progression of melioidosis pneumonia to severe or critical disease (Figure 1) and to provide a more accurate, fast, and reliable imaging assessment method for the clinical treatment and prognosis of melioidosis pneumonia. We present this article in accordance with the TRIPOD reporting checklist (available at https://qims.amegroups.com/article/view/10.21037/qims-23-1476/rc).
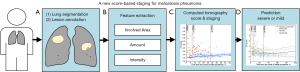
Methods
Dataset
The data used in this study comprised two parts. The first part was for lung segmentation and consisted of the open source novel coronavirus disease 2019 (COVID-19) dataset (11); this was combined with data from 10 participants with melioidosis pneumonia and annotated lung regions who were randomly drawn from the second part (the details of which are described below) as determined by expert radiologists (see Table 1). We randomly selected 120 scans (600 slices) of patients with COVID-19 and 7 scans (353 slices) of patients with melioidosis pneumonia for training. Meanwhile, 30 scans (150 slices) of patients with COVID-19 and 3 scans (121 slices) of patients with melioidosis pneumonia were used for testing (see Table 1). Finally, considering the unbalanced training data, we augmented the training images of patients with melioidosis pneumonia four times using left–right and up–down flipping. The second portion of data used for staging and predicting melioidosis pneumonia were collected from 11 hospitals in Hainan Province, China (see Appendix 1). This data set included 97 patients who had undergone continuous chest CT examinations between January 2002 and December 2021 throughout their treatment. The images were acquired with Siemens Healthineers, GE HealthCare, Toshiba, Philips and Neusoft CT machines. The patients whose CT images met the criteria for the radiologist’s requirements for disease diagnosis were included, with one senior physician assessing the image quality. Of these 97 patients, 46 (47.42%) had multiple CT scans (mild disease, 40.21%%; severe disease, 7.22%). We excluded patients without CT scans or with incomplete clinical data or in a severe condition not caused by melioidosis pneumonia (e.g., stroke). Scan sets containing any motion artifacts were excluded from the study. Patients with melioidosis pneumonia complicated by other pulmonary diseases (e.g., tumors or tuberculosis) or secondary melioidosis pneumonia (other systemic and visceral infections accompanying the initial symptoms and followed by pulmonary infection) were excluded (Figure 2). All patients were diagnosed based on culture. Standardized treatment of melioidosis pneumonia was considered to be targeted therapy for melioidosis conducted after a positive melioidosis test based on the drugs recommended according to drug sensitivity testing. The end point in the study was defined as severe or critical illness requiring admission to the intensive care unit (ICU) or respiratory support treatment (e.g., oxygen therapy via face mask, noninvasive techniques, full ventilatory support with endotracheal intubation) or death. In our melioidosis dataset, no patients died in the mild group, and there were 30 patients in the severe group, with a mortality rate of 20%. In this study, patients with this type of melioidosis pneumonia were uniformly classified into the severe group, while other patients were classified into the mild group. In the prediction, we randomly divided each dataset into two independent sets (training and testing sets) at a ratio of 7:3, with the scan levels for stage I (training: 15 scans of mild patients; testing: 6 scans of mild patients and 18 scans of severe patients), stage II (training: 40 scans of mild patients; testing: 20 scans of mild patients and 21 scans of severe patients), and stage III (training: 36 scans of mild patients for training; testing: 13 scans of mild patients and no scans of severe patients). It should be noted that none of the CT images overlapped between the training set and testing set (Figure 2).
Table 1
Data | Training (participant/scan/slice) | Testing (participant/scan/slice) |
---|---|---|
Melioidosis pneumonia | 7/7/353 | 3/3/121 |
COVID-19 (11) | 120/120/600 | 30/30/150 |
COVID-19, coronavirus disease 2019.
This study (registration No. ChiCTR2200060613) was conducted in accordance with the Declaration of Helsinki (as revised in 2013) and approved by the institutional review boards of two medical centers: West China Hospital of Sichuan University (No. 844) and Central South University Xiangya School of Medicine Affiliated Haikou Hospital of Biomedical Ethics Committee (No. SC20210192). The requirement for written informed consent was waived due to the retrospective nature of the study.
Lung segmentation and lesion annotation
To evaluate the performance of the automatic segmentations, we compared the spatial- and channel-wise coarse-to-fine attention network (SCOAT-Net) (12) segmentation with three other deep learning (DL)—based methods, including U-Net (13), U-Net++ (14), and fully convolution network (FCN) (15). SCOAT-Net, which showed the best performance, was employed for lung segmentation. We used Medical Imaging Interaction Tool (MITK) software to label the CT images of melioidosis pneumonia, which were manually labeled and segmented by four attending physicians (Long Fan, Yi Lin, Lifeng Li and Zidong Lv) according to the three signs (consolidation, nodules, and cavitation) (10) and identified with red, yellow, and blue indicators. The physicians were blinded to the clinical metadata according to the diagnostic criteria of Thoracic Imaging Pulmonary and Cardiovascular Radiology (16). A senior physician (Y.Z.) reviewed the types of signs and lesion boundaries via manual segmentation and finally reached an agreement with the attending physicians (Figure S1). We annotated 791 consolidation lesions, 3,919 nodular lesions, and 357 cavity lesions from all 169 CT scans.
In addition, there were two phases completed in CT image preprocessing: resolution regularization and Hounsfield unit (HU) value normalization (see Appendix 1).
Feature extraction and importance of CT features
Based on the annotated lesion labels and DL-based lung segmentation of the series of two-dimensional (2D) CT images, we could easily obtain the features of lung and lesions in three-dimensional (3D) space after postprocessing (for the details and distributions of some features, see Figure S2). Each feature value was derived from one CT scan of one participant. To extract the features of melioidosis pneumonia, we devised a feature extraction algorithm (see Appendix 1), the features of which included the involved area (volume), amount (number), and intensity (HU) of each of the three lesions (consolidation, nodules, and cavity). If the calculation of the change rate at a given time involved only one CT examination, and thus the CT lesion value at the initial onset time would be set to zero. Hence, data on a single CT and the timing of symptom onset were adequate for predicting the severity of the illness.
Furthermore, we included a time feature sourced from the contemporary medical history of the patient’s electronic medical record that represented the time elapsed since the onset of symptoms. To analyze which features are more important in the prediction of severe versus critical illness, a random forest (RF) machine learning algorithm consisting of 50 trees was applied (17). We also calculated the out-of-bag error. We stratified the dataset and randomly split the dataset into a training set using 70% of the data (118 scans; 93 mild; 25 severe) and tuning set using 30% of the data (51 scans; 37 mild; 14 severe). We obtained the average importance ranking for feature selection.
CT score
We computed a new CT score from the important features selected by the RF. First, to eliminate the extreme difference caused by the difference in measurement units between the different features, the histogram (frequency) distribution of each feature was calculated, and all the features were binned into scores of 1 to 10 (Eq. [1]). Second, the feature vectors of the prediction results were sorted via the RF importance (Eq. [2]). According to the different contribution values of the different feature vectors to the prediction results, the weights were assigned to make the scores more separable for prediction of severe versus critical illness. The CT score is defined in Eq. [3]:
where is the binned features belonging to an integer value between 1 and 10; is the binning; is the weight of the importance from RF of the feature, ; is the normalized importance of the feature; is the CT score; and is the number of features.
Server prediction with Gaussian process regression (GPR)
We further used the CT scores to predict severe versus critical illness at different stages using GPR (18). GPR is a nonparametric algorithm that assigns a probability to describe sequence data and can be used to predict progression (19). First, we standardized the CT score as follows:
where E is the mean value, and δ is standard deviation. Therefore, we assumed a probabilistic function with independent and identically normally distributed noise . We trained a GPR model to approximate the following:
where is the mean function, and is the covariance function with hyperparameters . Finally, in the GPR training performed by SciKitLearn (20), the Gaussian kernel was selected as the kernel function, and limited-memory Broyden-Fletcher-Goldfarb-Shanno algorithm (L-BFGS) (21) was selected as the hyperparameter optimization function, with the noise figure being set to 0.75.
Statistical analysis and evaluation metrics
Statistical analyses were performed by using Origin 2018C software (OriginLab Corporation, Northampton, MA, USA). All quantitative data are presented as raw values unless otherwise specified. The comparisons of quantitative data were evaluated by using the Kolmogorov-Smirnov test. P<0.05 was indicative of a statistically significant difference. Moreover, we applied six metrics to evaluate the segmentation and prediction performance. Dice coefficient and pixel accuracy were used to evaluate the lung field segmentation performance. The accuracy, sensitivity, specificity, and area under the curve (AUC) were used to evaluate the performance of the classifiers.
To protect patient privacy, patient-related data are not publicly accessible, but all data are available upon reasonable request emailed to the corresponding author. All the codes are available online (https://github.com/Rsolut1on/MP_CTscore).
Results
Patients
A total of 97 patients (84 men and 13 women) were included in the study. The mean patient age was 54±20 years for the severe group and 50±12 years for the mild group (Table 2). A portion of patients had diabetes (severe patients: 12/30, 40.0%; mild patients: 42/67, 62.7%), and the majority did not undergo standardized treatment (severe patients: 4/12, 33.3%; mild patients: 21/42, 50.0%). Fewer than half of the patients had a history of smoking and alcohol drinking. The relevant clinical critical periods are also listed in Table 2. The incidence of melioidosis pneumonia was higher in the rainy season (April to September) than in the other seasons (80 vs. 17; P<0.05). The severe group was admitted earlier and therefore received standardized treatment earlier compared to the mild group (severe: 7±4 days; mild: 14±16 days; P<0.05).
Table 2
Parameter | Severe (n=30) | Mild (n=67) | P value |
---|---|---|---|
Sex | >0.05 | ||
Male | 25 [83] | 59 [88] | |
Female | 5 [17] | 8 [12] | |
Age (y) | 54±20 | 50±12 | >0.05 |
Time between initial symptoms and admission (d) | 7±4 | 14±16 | <0.05 |
Season (spring:summer:fall:winter) | 3:16:9:2 | 4:24:31:8 | >0.05 |
Time between admission and diagnosis (d) | 6±4 | 7±6 | >0.05 |
Hospitalization period (d) | 21±16 | 23±12 | >0.05 |
Smoking (y) | 13 (43.3) | 26 (38.8) | >0.05 |
Alcohol drinking (y) | 10 (33.3) | 12 (17.9) | >0.05 |
Diagnosed with diabetes | 12 (40.0) | 42 (62.7) | >0.05 |
Duration of diabetes (y) | 3±5 | 2±3 | >0.05 |
Diabetes treatment not standardized | 4 (33.3) | 21 (50.0) | >0.05 |
Except where indicated, data are the numbers of patients, with percentages in parentheses. Data are presented as the mean ± SD where applicable. y, years; d, days; SD, standard deviation.
Feature extraction and CT score
First, we trained SCOAT-Net (12) for lung segmentation. The CT images were segmented into two parts: lung and the other tissues (Figure 3). Second, using RF, we calculated the importance of all 24 features by labeling whether the patient with this scan would develop severe or critical disease. Combining clinical knowledge and the experimental results (2,10) (see Appendix 1), we selected 12 image features and 1 clinical feature, as shown in Table 3. The relative importance of the selected image features is shown in Figure 4. Third, we binned 12 features into scores of 1 to 10 using histograms (Figure S3). Finally, the CT score was obtained based on the 12 image features with importance (Figure 5A).
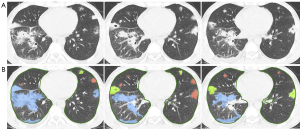
Table 3
Feature |
Time from onset of initial symptoms** |
Ratio of volume |
All lesions* |
Consolidation* |
Nodule* |
Cavity |
Ratio of volume of individual lesions |
Nodule (max*, mean, min, and median*) |
Cavity (max, mean*, min*, and median) |
Max Hounsfield unit |
Consolidation |
Nodule |
Min Hounsfield unit |
Consolidation |
Nodule |
Rate of volume change |
All lesions* |
Consolidation* |
Nodule* |
Cavity* |
Number of lesions |
Nodule |
Cavity* |
Ratio of air to cavity |
*, a selected image feature; **, a clinical feature.
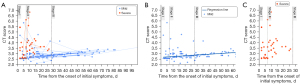
Staging
It was difficult to distinguish mild (blue dots) from severe (red dots) patients within approximately 7 days after the onset of symptoms, and the CT scores of both the severe and mild patients ranged from 2.5 to 6.5 (Figure 5A). In the patients who did not develop severe disease after 28 days (in our dataset), the CT score of the mild patients fluctuated approximately between 2.5 and 3.5 in a linear distribution (Figure 5B). Between these two time points, the main range of the CT scores for the severe patients was 3.0–7.0 (Figure 5C), and the range of the CT scores for the mild patients was 2.0–5.0 (Figure 5B). On the basis of the CT score distribution above from day 0 to day 100 after disease onset, three stages were identified from the onset of initial symptoms (Figure 5A): stage I (acute stage: 0–7 days, 39 scans), stage II (subacute stage: 8–28 days, 81 scans), and stage III (chronic stage: >28 days, 49 scans). For the CT scores, there were significant differences among the three stages (stage I vs. stage II: P<0.001; stage II vs. stage III: P<0.001; stage I vs. stage III: P<0.001).
There were different changes in the kind of lesions between the three stages. Because volume demonstrated the greatest variability among all 12 imaging features, we used the performance and changes of this feature in the three stages to further elaborate the clinical rationality of staging based on the CT score. Consolidation is the most common lesion type in melioidosis pneumonia, and the volume ratio of consolidation lesions decreased successively in the three stages in both the severe (91.80% to 83.84%) and mild cases (93.70% to 66.78%) (Figure S4A). As a result, the proportion of nodules and cavities increased from less than 20% (stage I) to approximately 40% (stage III) (Figure S4A). Figure S4B shows the volume ratio when compared to the lung volume for the three types of lesions in three stages. We can see that the three types of lesion volume ratios in the scans of the patients who would develop severe disease increased significantly from 10.28% to 21.54% between stages I and II. There was only a slight increase from 5.65% to 7.84% in stages I and II, and stage III dropped significantly to a level (2.91%) lower than that of stage I for the scans of mild disease (Figure S4B). In our data, no severe cases progressed to stage III, which may indicate improvement or a severe outcome in stage II.
The proportion of scans with nodules and cavities is shown in Figure S4C. Among the 169 CT scans, nodules were present in 40 (23.67%), consolidation was present in 160 (94.67%), and cavities were present in 71 (42.01%). The percentage of the severe scans with nodules decreased from 72.22% (13 of 18 scans) to 47.62% (10 of 21 scans) between stages I and II (P<0.05). Meanwhile, in the severe scans, the percentage of scans with cavities significantly increased from 16.67% to 57.14% (P<0.05). Furthermore, the percentages of scans with nodules and cavities in the mild group were both slightly increased between the first two stages (nodules: 76.19% to 85.00%; cavities: 52.38% to 65.00%). In stage III, only the mild scans were collected.
Prediction of severe disease
To predict whether the patients in different stages would develop severe disease, we projected the standardized image features to the Z score through the confidence interval (CI) of the GPR. Overall, we found that points above the 95% CI were associated with a higher probability of severe disease [Figure 6A: posterior mean and 95% (±1.96) (dashed lines) and 99.7% (±3) credible intervals (dotted lines) estimated from the training data (gray)]. Additionally, to predict severity, we projected the standardized CT score to the Z score using the GPR credible interval for prediction (Figure 6B).
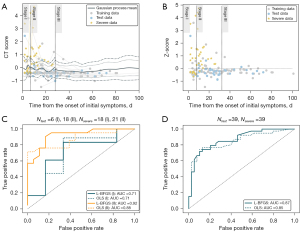
The result of the receiver operator characteristic evaluation for quantifying the predictive potential of the proposed GPR model is shown in Figure 6C,6D. Importantly, because there were no severe patients in stage III, the AUCs of the first stage and the second stage were calculated. The results showed that the GPR optimization function with L-BFGS had an AUC of 0.71 and 0.92 for stage I and stage II, respectively, which was superior to the AUCs of 0.71 and 0.88, respectively, yielded with ordinary least squares (OLS). In addition, the AUC for the all stages was calculated, yielding an AUC of 0.87 for L-BFGS and an AUC of 0.85 for OLS.
Discussion
In this study, we found that the CT score based on different stages of melioidosis pneumonia can be used as a quantitative indicator to improve clinicians’ ability to predict severe or critical disease. The patients in this study are similar to those reported internationally in terms of sex, age, season of onset, and underlying diseases (22), which suggests that the results of this study can be generalized.
Traditional CT quantitative estimates are mainly performed by radiologists and are therefore affected by inter- and intraobserver consistency. In recent years, quantitative analysis methods (23,24) of CT images have often incorporated first-order statistical features, texture features, and wavelet features in radiomics, but these are too numerous for the physicians evaluating them (25). We manually annotated the lesions and conducted targeted feature extraction, which included calculating changes between each image feature and previous features and determining the proportion of lesion volume relative to the entire lung volume. Finally, we incorporated medical expertise to perform feature selection (2,10), which not only could reflect the disease development process but also reduce the staging instability caused by individual differences. The machine learning method was used to obtain CT scores by weighting according to the contribution of reliable and quantitative CT feature parameters (26). In the process, we found that the rate of volume change, which reflected progression, contributed the most to the prediction. However, in real clinical work, due to economic, radiation, and other reasons, it is often difficult to obtain multiple CT examinations in a short period of time. Most patients had only one CT examination before they progressed to severe disease. The calculation of the change rate with this number will set the CT lesion value at the initial onset time to zero, so it does not necessarily reflect the actual changes in the lesions. In contrast, the ratio of the lesion volume to the entire lung volume may be the most objective and robust indicator (27,28). The CT score in this study included capturing both the static range and the dynamic change rate of the disease, and the combination of the two side parameters may be able to truly reflect the severity of disease progression. In addition, we proposed a Gaussian model for staging melioidosis pneumonia according to the prognostic status because the relationship between the severity of pneumonia and the risk of adverse outcomes is unlikely to be linear. Gaussian models are a better solution for these types temporal classification problems (18).
In this study, (I) stage I was consistent with the clinical stage reported by Dhiensiri et al. (10), and pulmonary lesions in the early stage of the disease are characterized by capillary vasculitis and accompanying alveolar hemorrhage and destruction (2). Compared to those of mild patients, the volume ratio of consolidation and the proportion of scans with nodules in severe patients were not significantly different, which suggests that it is difficult to predict the disease by imaging at the early stage of the disease. However, the possibility of developing severe disease should be closely monitored, and the change rate of lesions might be a more sensitive indicator for predicting severe disease. (II) Stage II referred to Dhiensiri et al.’s (10) and Currie et al.’s (9,29) clinical staging of melioidosis pneumonia (<2 months, equal to 8 weeks) and the characteristics of this group of data (almost no severely ill patients after 28 days). Staging in this study being conducted on a weekly basis (28 days, equal to 4 weeks). In the severe group, the volume ratio of all lesions increased (10.28% to 21.54%; P<0.05), the percentage of nodules decreased (72.22% to 47.62%; P<0.05), and the percentage of cavities significantly increased (16.67% to 57.14%; P<0.05), which suggests that as the disease progresses, the lesions become granuloma-like, small abscesses appear, and then cavities form (10,30). (III) None of the severe patients were stage III, indicating that the subacute stage may be a critical period for disease outcomes. The proportion of nodules and cavities is increased, which is similar to the radiographic findings of small nodules and cavities in the chronic stage reported in the literature (31). Our findings thus suggest the superiority of an prognosis staging based on the imaging of patients, and the staging may be more accurate and more in line with the clinical needs.
There are some limitations in this study that should be addressed. First, the results of this study are only applicable to patients with melioidosis pneumonia whose first symptom is a pulmonary infection, as determining the onset time of secondary melioidosis pneumonia is difficult. Second, while the rate of volume change may be used as an important indicator for prognostic prediction, it is often challenging to obtain clinically. Third, the number of cases was relatively small; however, the data were obtained from multiple centers, and thus the results of this study maintain some representativeness.
Conclusions
For patients with melioidosis pneumonia, it is reasonable to categorize the disease progression into three stages, with stage II serving as a potential pivotal point in determining progression. The evaluation of the patient’s risk of severe progression based on CT scores can assist in treatment planning.
Acknowledgments
We would like to thank Kang Zhang et al. for providing the public COVID-19 dataset and Long Fan (Department of Radiology, The Second Affiliated Hospital of Hainan Medical University), Yi Lin (Hainan Women and Children’s Medical Center), Lifeng Li (The Affiliated Changsha Central Hospital, Department of Radiology, Hengyang Medical School, University of South China), and Zidong Lv (Hainan Women and Children’s Medical Center) for labeling the images. We also express our gratitude to Xiaohua Li (Department of Radiology, The First Affiliated Hospital of Hainan Medical University), Long Fan (Department of Radiology, The Second Affiliated Hospital of Hainan Medical University), Shengshi Mai (Department of Radiology, Sanya People’s Hospital), Jingxiong Li (Department of Radiology, Sanya Central Hospital), Xiaohua Fu (Department of Radiology, Central South University Xiangya School of Medicine Affiliated Haikou Hospital), Zewei Wang (Department of Radiology, Hainan Hospital of Traditional Chinese Medicine), Hao Yun (Department of Radiology, Wenchang People’s Hospital), and Hanwen Chen (Department of Radiology, LeDong Second People’s Hospital) for providing part of melioidosis pneumonia dataset.
Funding: This work was supported by
Footnote
Reporting Checklist: The authors have completed the TRIPOD reporting checklist. Available at https://qims.amegroups.com/article/view/10.21037/qims-23-1476/rc
Conflicts of Interest: All authors have completed the ICMJE uniform disclosure form (available at https://qims.amegroups.com/article/view/10.21037/qims-23-1476/coif). The authors have no conflicts of interest to declare.
Ethical Statement: The authors are accountable for all aspects of the work in ensuring that questions related to the accuracy or integrity of any part of the work are appropriately investigated and resolved. The study was conducted in accordance with the Declaration of Helsinki (as revised in 2013) and was approved by the institutional review boards of two medical centers: West China Hospital of Sichuan University (No. 844) and Central South University Xiangya School of Medicine Affiliated Haikou Hospital of Biomedical Ethics Committee (No. SC20210192). The requirement for written informed consent was waived due to the retrospective nature of the study.
Open Access Statement: This is an Open Access article distributed in accordance with the Creative Commons Attribution-NonCommercial-NoDerivs 4.0 International License (CC BY-NC-ND 4.0), which permits the non-commercial replication and distribution of the article with the strict proviso that no changes or edits are made and the original work is properly cited (including links to both the formal publication through the relevant DOI and the license). See: https://creativecommons.org/licenses/by-nc-nd/4.0/.
References
- Meumann EM, Cheng AC, Ward L, Currie BJ. Clinical features and epidemiology of melioidosis pneumonia: results from a 21-year study and review of the literature. Clin Infect Dis 2012;54:362-9. [Crossref] [PubMed]
- Gassiep I, Armstrong M, Norton R. Human Melioidosis. Clin Microbiol Rev 2020;33:e00006-19. [Crossref] [PubMed]
- Limmathurotsakul D, Golding N, Dance DA, Messina JP, Pigott DM, Moyes CL, Rolim DB, Bertherat E, Day NP, Peacock SJ, Hay SI. Predicted global distribution of Burkholderia pseudomallei and burden of melioidosis. Nat Microbiol 2016;1:15008. [Crossref]
- Chan KP, Low JG, Raghuram J, Fook-Chong SM, Kurup A. Clinical characteristics and outcome of severe melioidosis requiring intensive care. Chest 2005;128:3674-8. [Crossref] [PubMed]
- Wiersinga WJ, Virk HS, Torres AG, Currie BJ, Peacock SJ, Dance DAB, Limmathurotsakul D. Melioidosis. Nat Rev Dis Primers 2018;4:17107. [Crossref] [PubMed]
- Gelissen H, de Grooth HJ, Smulders Y, Wils EJ, de Ruijter W, Vink R, Smit B, Röttgering J, Atmowihardjo L, Girbes A, Elbers P, Tuinman PR, Oudemans-van Straaten H, de Man A. Effect of Low-Normal vs High-Normal Oxygenation Targets on Organ Dysfunction in Critically Ill Patients: A Randomized Clinical Trial. JAMA 2021;326:940-8. [Crossref] [PubMed]
- Claessens YE, Debray MP, Tubach F, Brun AL, Rammaert B, Hausfater P, Naccache JM, Ray P, Choquet C, Carette MF, Mayaud C, Leport C, Duval X. Early Chest Computed Tomography Scan to Assist Diagnosis and Guide Treatment Decision for Suspected Community-acquired Pneumonia. Am J Respir Crit Care Med 2015;192:974-82. [Crossref] [PubMed]
- Aliberti S, Dela Cruz CS, Amati F, Sotgiu G, Restrepo MI. Community-acquired pneumonia. Lancet 2021;398:906-19. [Crossref] [PubMed]
- Currie BJ, Mayo M, Ward LM, Kaestli M, Meumann EM, Webb JR, Woerle C, Baird RW, Price RN, Marshall CS, Ralph AP, Spencer E, Davies J, Huffam SE, Janson S, Lynar S, Markey P, Krause VL, Anstey NM. The Darwin Prospective Melioidosis Study: a 30-year prospective, observational investigation. Lancet Infect Dis 2021;21:1737-46. [Crossref] [PubMed]
- Dhiensiri T, Puapairoj S, Susaengrat W. Pulmonary melioidosis: clinical-radiologic correlation in 183 cases in northeastern Thailand. Radiology 1988;166:711-5. [Crossref] [PubMed]
- Zhang K, Liu X, Shen J, Li Z, Sang Y, Wu X, et al. Clinically Applicable AI System for Accurate Diagnosis, Quantitative Measurements, and Prognosis of COVID-19 Pneumonia Using Computed Tomography. Cell 2020;181:1423-1433.e11. [Crossref] [PubMed]
- Zhao S, Li Z, Chen Y, Zhao W, Xie X, Liu J, Zhao D, Li Y. SCOAT-Net: A novel network for segmenting COVID-19 lung opacification from CT images. Pattern Recognit 2021;119:108109. [Crossref] [PubMed]
- Ronneberger O, Fischer P, Brox T. U-net: Convolutional networks for biomedical image segmentation. In: Navab N, Hornegger J, Wells W, Frangi A. editors. Medical Image Computing and Computer-Assisted Intervention – MICCAI 2015. MICCAI 2015. Lecture Notes in Computer Science, Springer, Cham, 2015:9351:234-41.
- Zhou Z, Siddiquee MMR, Tajbakhsh N, Liang J. UNet++: A Nested U-Net Architecture for Medical Image Segmentation. Deep Learn Med Image Anal Multimodal Learn Clin Decis Support (2018) 2018;11045:3-11. [Crossref] [PubMed]
- Shelhamer E, Long J, Darrell T. Fully Convolutional Networks for Semantic Segmentation. IEEE Trans Pattern Anal Mach Intell 2017;39:640-51. [Crossref] [PubMed]
- Webb WR, Higgins CB. Thoracic imaging: pulmonary and cardiovascular radiology. Lippincott Williams & Wilkins, 2010.
- Breiman L. Random forests. Mach Learn 2001;45:5-32. [Crossref]
- Pietsch M, Ho A, Bardanzellu A, Zeidan AMA, Chappell LC, Hajnal JV, Rutherford M, Hutter J. APPLAUSE: Automatic Prediction of PLAcental health via U-net Segmentation and statistical Evaluation. Med Image Anal 2021;72:102145. [Crossref] [PubMed]
- Cole JH, Franke K. Predicting Age Using Neuroimaging: Innovative Brain Ageing Biomarkers. Trends Neurosci 2017;40:681-90. [Crossref] [PubMed]
- Pedregosa F, Varoquaux G, Gramfort A, Michel V, Thirion B, Grisel O, Blondel M, Prettenhofer P, Weis R, Dubourg V. Scikit-learn: Machine learning in Python. J Mach Learn Res 2011;12:2825-30.
- Liu DC, Nocedal J. On the limited memory BFGS method for large scale optimization. Math Program 1989;45:503-28. [Crossref]
- Birnie E, Virk HS, Savelkoel J, Spijker R, Bertherat E, Dance DAB, Limmathurotsakul D, Devleesschauwer B, Haagsma JA, Wiersinga WJ. Global burden of melioidosis in 2015: a systematic review and data synthesis. Lancet Infect Dis 2019;19:892-902. [Crossref] [PubMed]
- Saeki Y, Kitazawa S, Kobayashi N, Kikuchi S, Goto Y, Sato Y. Prediction of invasiveness in lung adenocarcinoma using machine learning algorithm based on 3D-CT imaging. Ann Oncol 2019;30:ix152. [Crossref]
- Cho JL, Villacreses R, Nagpal P, Guo J, Pezzulo AA, Thurman AL, Hamzeh NY, Blount RJ, Fortis S, Hoffman EA, Zabner J, Comellas AP. Quantitative Chest CT Assessment of Small Airways Disease in Post-Acute SARS-CoV-2 Infection. Radiology 2022;304:185-92. [Crossref] [PubMed]
- Mackin D, Fave X, Zhang L, Fried D, Yang J, Taylor B, Rodriguez-Rivera E, Dodge C, Jones AK, Court L. Measuring CT scanner variability of radiomics features. Invest Radiol 2015;50:757-65. [Crossref] [PubMed]
- Araiza A, Duran M, Patiño C, Marik PE, Varon J. The Ichikado CT score as a prognostic tool for coronavirus disease 2019 pneumonia: a retrospective cohort study. J Intensive Care 2021;9:51. [Crossref] [PubMed]
- Pan F, Ye T, Sun P, Gui S, Liang B, Li L, Zheng D, Wang J, Hesketh RL, Yang L, Zheng C. Time Course of Lung Changes at Chest CT during Recovery from Coronavirus Disease 2019 (COVID-19). Radiology 2020;295:715-21. [Crossref] [PubMed]
- Wang Y, Dong C, Hu Y, Li C, Ren Q, Zhang X, Shi H, Zhou M. Temporal Changes of CT Findings in 90 Patients with COVID-19 Pneumonia: A Longitudinal Study. Radiology 2020;296:E55-64. [Crossref] [PubMed]
- Currie BJ, Fisher DA, Anstey NM, Jacups SP. Melioidosis: acute and chronic disease, relapse and re-activation. Trans R Soc Trop Med Hyg 2000;94:301-4. [Crossref] [PubMed]
- Flemma RJ, DiVincenti FC, Dotin LN, Pruitt BA Jr. Pulmonary melioidosis; a diagnostic dilemma and increasing threat. Ann Thorac Surg 1969;7:491-9. [Crossref] [PubMed]
- Burivong W, Wu X, Saenkote W, Stern EJ. Thoracic radiologic manifestations of melioidosis. Curr Probl Diagn Radiol 2012;41:199-209. [Crossref] [PubMed]