Prediction of histologic grade of hepatocellular carcinoma using dual-layer spectral-detector computed tomography (CT): comparison of two region of interest plotting methods
Introduction
Hepatocellular carcinoma (HCC) is one of the most common malignant tumors globally and the leading cause of death in patients with chronic liver disease (1). Due to the rapid disease progression and hidden lesions, most patients are diagnosed in the middle and advanced stages (2); the overall prognosis remains poor, with an international 5-year survival rate for liver cancer of only 14.1% (3). The pathological differentiation grade is one of the most important factors affecting the recurrence and prognosis of HCC (4). Therefore, accurate preoperative grading of HCC is crucial for predicting the prognosis (5).
Although histopathological or cytological examination is considered the gold standard for diagnosing liver cancer, the collection of biopsy samples is invasive and carries the risk of spreading liver tumors (6). Previous studies have demonstrated that non-invasive quantitative parameter models, such as the apparent diffusion coefficient (5,7), the ratio of enhancement (2), and the tumor stiffness (8), can be used to predict the pathological grade of HCC, but these studies have been based on magnetic resonance imaging. After iterative updates in computed tomography (CT) technology, multi-type post-processing imaging of spectral CT not only provides improved image contrast and reliable tissue enhancement measurement but also enhances lesion visibility and the rate of lesion detection (9). The multi-parameter imaging technology, which is based on spectral single-energy imaging, substance separation, and effective atomic number, is also helpful in predicting the pathological grade of tumors (10-14) or hepatic fibrosis grading (15). At the same time, researchers have shown that different methods of positioning region of interest (ROI) significantly affect the measurement parameters (5,8,16,17), thereby impacting the diagnostic performance of the study. As far as we know, in this field of energy CT studies for distinguishing liver cancer pathology, the planar sketching (PS) method is commonly used to plot and measure the lesions. However, few studies have analyzed the entire tumor, and even fewer have compared the 2 types of ROI plotting methods.
Therefore, the purpose of this study was to compare the impact of 2 different ROI plotting methods on data measurement and diagnosis, and to investigate functional quantitative parameters for distinguishing between poorly differentiated and non-poorly differentiated HCC based on dual-layer spectral-detector computed tomography (DLCT). We present this article in accordance with the STARD reporting checklist (available at https://qims.amegroups.com/article/view/10.21037/qims-23-1753/rc).
Methods
Patients
The study was conducted in accordance with the Declaration of Helsinki (as revised in 2013). This retrospective study was approved by the Institutional Review Board of Nanfang Hospital (No. NFEC-2023-521), and the requirement for written informed consent from patients was waived due to the retrospective nature of the study.
We reviewed 160 patients diagnosed with liver cancer using enhanced abdominal radiography with DLCT at our center from May 2022 to March 2023. Based on the time limit for continuous use of CT equipment and the research deadline of the Ethics Committee of our center, our research span was less than 1 year. The inclusion criteria were as follows: (I) use of DLCT for abdominal contrast-enhanced scanning at our center; (II) diagnosis of liver cancer through imaging examination or clinical diagnosis. Among them, 112 patients were excluded for the following reasons: (I) history of preoperative treatment including surgery, transhepatic arterial chemotherapy and embolization (TACE), ablation, immunotherapy, and radiotherapy (n=29); (II) patients with pathologically confirmed non-HCC lesions (n=16); (III) patients without pathologic confirmation of HCC from surgical resection or biopsy (n=63); (IV) absence or poor image quality that prevents identification of the lesions (n=4).
Dual-layer spectral-detector CT imaging protocol
CT was performed using DLCT (spectral CT 7500; Philips Healthcare, Best, Netherlands) with a non-enhanced and dual-phase contrast-enhanced scan in the craniocaudal direction and the supine position. These patients were intravenously injected with a non-ionic contrast agent (Ioversol Injection 350; Jiangsu Hengrui Pharmaceuticals Co., Ltd., Lianyungang, China) using a high-pressure injector at a rate of 2.5–3.0 mL/s, with a total dose of 60–80 mL (1 mL/kg of body weight). The total amount was calculated as 0.8–1 mL/kg body weight; 2.5–3.0 mL/s was injected into the elbow vein. The patients were scanned at 30 seconds and 65 seconds after the injection of the contrast agent in the arterial phase (AP) and portal venous phase (VP), respectively. The scanning parameters were as follows: tube voltage, 120 kVp; automated modulation with dose right index 20; pitch, 1.0; rotation time, 0.48 seconds, collimation, 128×0.625 mm. After scanning, the spectral-based image sequence was obtained by spectral reconstruction algorithm.
Image generation
The conventional images and quantitative spectral analysis were performed using the IntelliSpace Portal software (Version 12.1; Philips Healthcare). The spectral-based image sequences were post-processed to generate different types of images: (I) conventional CT images; (II) electron density relative to water (EDW) images; (III) effective atomic number (Zeff) images; (IV) iodine density (ID) images. All the images were reconstructed with 1 mm slice thickness and 1 mm interval.
Parameter measurement and quantitative analysis
All reconstructed images were measured by 2 radiologists (R1 and R2, with 3 and over 10 years of experience in reading CT images, respectively), who were blinded to the histopathology results.
We used 2 different ROI plotting methods in the measurement: (I) conventional PS: the layer with the highest enhancement of the lesion in the arterial and VP images were selected, and 3 consecutive ROIs were drawn in the uniformly strengthened area. The average was then calculated for analysis, while avoiding fat, necrosis, vessels, and calcification. The abdominal aorta and normal liver tissue were sketched at the same level as the lesion; (II) whole tumor volume rendering (WTVR): the smart segmentation tool was used to render the volume of tumor lesions in ID images or conventional images, and they were supplemented or modified at continuous levels to ensure that all tumor tissues (including vessels, necrosis, bleeding, and avoiding calcification) were included. Subsequently, volumetric analysis was conducted using spectral sub-segmentation, which recorded the tumor volume, conventional CT attenuation values, EDW, Zeff, and ID based on the delineated tumor section.
To avoid the impact of individualized differences, it is necessary to normalize the values of various reconstructed images based on the patient’s normal liver tissue and abdominal aorta (18). The normalized effective atomic number (NZeff) and normalized iodine density (NID) were calculated at the AP and VP, respectively, according to the following formulas:
Then, the difference was calculated between AP and VP of all spectral parameters of the tumor according to the following formula:
Pathologic analysis
All patients underwent pathological examination within 1 week after the DLCT examination. The pathological reports after surgery or biopsy were used as a standard of reference. Pathologic grade analysis was based on the World Health Organization (WHO) classification of digestive system tumors (19). When the evaluated HCC had more than 1 grade, the higher pathologic grade was recorded. The tumors were allocated to either a non-poorly differentiated group (including well differentiated and moderately differentiated) or a poorly differentiated group.
Statistical analysis
Statistical analyses were performed using the software SPSS 26.0 (IBM Corp., Armonk, NY, USA). All tests were 2-sided, and p-values lower than 0.05 were considered statistically significant. The continuous variables were described by mean ± standard deviation. The categorical variables were reported as percentages. First, the intraclass correlation coefficient (ICC) was used to assess the reliability between 2 independent radiologists for each parameter. ICC values greater than 0.90 indicated excellent reliability. The normal distribution of quantitative variables was assessed using the Shapiro-Wilk test. The average value was measured by 2 radiologists for the analysis of diagnostic performance. We used either 2-sample t-test or Mann-Whitney U test to compare the differences in parameters between the 2 methods, as well as the differences in parameters among different histologic grade groups. The classified variables were compared between groups using a chi-square test. Spearman correlation analysis was utilized to assess the correlation between quantitative parameters and the degree of pathological differentiation, a correlation coefficient rho (r) results were interpreted according to the following criteria: less than 0.25, poor relationship; 0.25–0.5, moderate relationship; 0.5–0.75, good relationship; and 0.75–1.00, excellent relationship. The receiver operating characteristic (ROC) curve analysis was performed to evaluate the diagnostic performance of each spectral quantitative parameter for HCC grading and to determine the optimal parameters.
Sample size estimation was performed using PASS 15.0 software (NCSS LLC, East Kaysville, UT, USA). This was a retrospective study, with the positive group comprising the poorly differentiated group, and the negative group comprising the non-poorly differentiated group. The observational index of this study was the spectral parameters of tumors. Based on the analysis of the ΔCT parameter in the pre-experimental results, when using the PS method, the mean ΔCT value of the positive group was 9.0±10.3, and the mean ΔCT value of the negative group was −3.2±19.7. Assuming α = 0.05 and β = 0.20 (2-tailed test), we collected positive and negative patients in a 1:3.3 ratio. Using PASS 15.0 software, the sample size of the positive group was calculated to be 13, and the sample size of the negative group was calculated to be 43. When using the WTVR method, the mean ΔCT value of the positive group was −5.0±6.0, and the mean ΔCT value of the negative group was −16.5±13.1. Calculated under the same assumptions, the sample size for the positive group was 6, and the sample size for the negative group was 20. Combining the differences between the 2 methods, at least 56 lesions were ultimately included.
Results
Patient characteristics
A total of 48 patients (including 44 males and 4 females; age 52.06±12.26 years; range, 22–75 years) with 56 pathologically confirmed HCC lesions were included. Among them, 21 patients underwent surgical resection and 27 patients underwent biopsies. According to the histopathological report, 40 patients with 43 lesions were assigned to the non-poorly differentiated group, whereas 8 patients with 13 lesions were assigned to the poorly differentiated group. Figure 1 provides a flowchart showing the patient selection process.
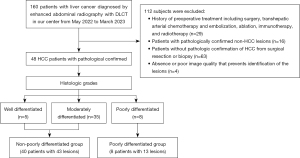
The clinical and pathological characteristics of the patients across different histologic grades are shown in Table 1. There were no significant differences in age, maximum tumor diameter, tumor volume, gender, cause, cirrhosis, baseline alpha-fetoprotein (AFP) level, and the Liver Imaging Reporting and Data System (LI-RADS) (20) classification between the poorly differentiated group and the non-poorly differentiated group (P>0.05). Patients with cirrhosis represented 62.50% of the poorly differentiated group compared with 52.50% of the non-differentiated group. There was no significant difference in liver cirrhosis between the 2 groups (P=0.90).
Table 1
Variables | All | Non-poorly differentiated | Poorly differentiated | P value |
---|---|---|---|---|
Age (years) | 52.06±12.26 | 53.40±12.39 | 45.38±9.64 | 0.09 |
Maximum tumor diameter (mm) | 67.13±42.16 | 65.67±40.54 | 71.95±48.59 | 0.85 |
Tumor volume (cm3) | 200.99±264.17 | 186.10±256.97 | 250.23±292.09 | 0.66 |
Gender | >0.99 | |||
Male | 44 (91.67) | 36 (90.00) | 8 (100.00) | |
Female | 4 (8.33) | 4 (10.00) | 0 (0.00) | |
Cause | >0.99 | |||
HBV | 39 (81.25) | 32 (80.00) | 7 (87.50) | |
No-HBV | 9 (18.75) | 8 (20.00) | 1 (12.50) | |
Cirrhosis | 0.90 | |||
Present | 26 (54.17) | 21 (52.50) | 5 (62.50) | |
Absent | 22 (45.83) | 19 (47.50) | 3 (37.50) | |
Baseline AFP level (ng/mL) | 0.66 | |||
>7 | 36 (75.00) | 29 (72.50) | 7 (87.50) | |
≤7 | 12 (25.00) | 11 (27.50) | 1 (12.50) | |
LI-RADS | 0.73 | |||
LR-4 | 6 (10.71) | 5 (11.63) | 1 (7.69) | |
LR-5 | 36 (64.29) | 28 (65.12) | 8 (61.54) | |
LR-M | 2 (3.57) | 1 (2.32) | 1 (7.69) | |
LR-TIV | 12 (21.43) | 9 (20.93) | 3 (23.08) |
Data are presented as the mean ± standard deviation or numbers (percentages). A total of 48 patients with 56 lesions were included: the non-poorly differentiated group comprised 40 patients with 43 lesions, and the poorly differentiated group comprised 8 patients with 13 lesions. HCC, hepatocellular carcinoma; HBV, hepatitis B virus; AFP, alpha-fetoprotein; LI-RADS, The Liver Imaging Reporting and Data System; LR-M, The Liver Imaging-probably or definitely malignant; LR-TIV, The Liver Imaging-tumor in vein.
Interobserver reproducibility
The interobserver reliability of DLCT parameters measurement of tumors was excellent (the range of ICC was 0.926 to 0.991). Except for the IDlive in AP with an ICC value of 0.725, which shows moderate reliability, the interobserver reliability of other DLCT parameter measurement of normal liver tissue and abdominal aorta were excellent (the range of ICC was 0.924 to 0.998). The detailed results are shown in Tables S1,S2.
Comparison of two different ROI plotting methods in parameter measurement
In certain parameters in AP (CT, NZeff, NID) and some difference parameters (∆CT, ∆EDW, ∆NZeff), the average values of the measured data plotted in the WTVR method were lower than those in the PS method (Figures 2,3) and the difference was statistically significant (R1: P<0.05, R2: P<0.05). However, for EDW in AP, the statistical results between the 2 sketching methods were inconsistent between the 2 observers (R1: P<0.05; R2: P>0.05). In addition, for all parameters in VP (CT, EDW, NZeff, NID) and ∆NID, the average values of the measured data plotted in the WTVR method were lower than those in the PS method, but the 2 methods did not show a statistically significant difference (R1: P>0.05, R2: P>0.05). The detailed results are shown in Table 2.
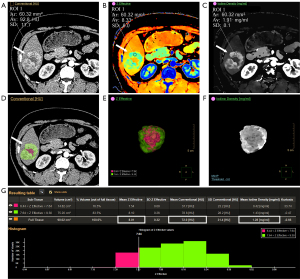
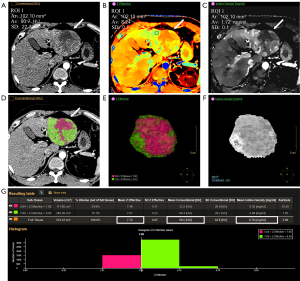
Table 2
Parameter | R1 | R2 | |||||
---|---|---|---|---|---|---|---|
PS (n=56) | WTVR (n=56) | P value | PS (n=56) | WTVR (n=56) | P value | ||
AP | |||||||
CT (HU) | 94.235±26.406 | 70.780±18.486 | <0.001 | 82.532±23.895 | 69.996±19.350 | 0.003 | |
EDW (%) | 104.590±0.788 | 104.155±0.635 | <0.001 | 104.333±0.766 | 104.109±0.684 | 0.106* | |
NZeff | 0.062±0.032 | 0.032±0.021 | <0.001 | 0.052±0.029 | 0.032±0.023 | <0.001 | |
NID | 0.134±0.081 | 0.064±0.046 | <0.001 | 0.104±0.074 | 0.061±0.054 | 0.001 | |
VP | |||||||
CT (HU) | 90.174±17.078 | 84.548±15.001 | 0.061 | 87.286±16.779 | 83.925±16.353 | 0.285* | |
EDW (%) | 104.398±0.705 | 104.257±0.618 | 0.220 | 104.315±0.682 | 104.220±0.693 | 0.528 | |
NZeff | −0.003±0.025 | −0.010±0.021 | 0.170 | −0.005±0.023 | −0.011±0.022 | 0.181* | |
NID | −0.023±0.129 | −0.057±0.105 | 0.254 | −0.032±0.121 | −0.058±0.109 | 0.244* | |
AP-VP | |||||||
∆CT (HU) | 4.061±20.282 | −13.768±12.490 | <0.001 | −4.755±17.971 | −13.929±13.292 | 0.002 | |
∆EDW (%) | 0.192±0.471 | −0.102±0.214 | <0.001 | 0.017±0.380 | −0.111±0.248 | 0.011 | |
∆NZeff | 0.065±0.026 | 0.042±0.021 | <0.001 | 0.057±0.028 | 0.043±0.024 | 0.005* | |
∆NID | 0.156±0.100 | 0.121±0.091 | 0.067 | 0.136±0.101 | 0.118±0.100 | 0.355* |
Data are presented as the mean ± standard deviation. *, P value for 2-sample t-test. ROI, region of interest; R1, radiologist 1; R2, radiologist 2; PS, planar sketching; WTVR, whole tumor volume rendering; AP, arterial phase; CT, attenuation value of conventional computed tomography; HU, Hounsfield unit; EDW, electron density relative to water; NZeff, normalized effective atomic number; NID, normalized iodine density; VP, venous phase; AP-VP, the difference between the arterial phase and venous phase; ; ; ; .
Diagnostic performance of spectral parameters in differentiating HCC histologic grade
In discriminating poorly from non-poorly differentiated HCC, only certain parameters showed statistical significance. The NZeffVP and NIDVP values of the poorly differentiated group were significantly lower than those of the non-poorly differentiated group (all P<0.05). The ∆CT, ∆EDW, ∆NZeff, and ∆NID values of the poorly differentiated group were significantly higher than those of the non-poorly differentiated group (all P<0.05) (Table 3). Other quantitative parameters show no statistical difference in identification, so they cannot be used to evaluate HCC grade.
Table 3
Parameter | Method | Non-poorly differentiated (n=43) | Poorly differentiated (n=13) | P value |
---|---|---|---|---|
VP | ||||
NZeff | PS | −0.001±0.023 | −0.015±0.018 | 0.045 |
WTVR | −0.007±0.021 | −0.023±0.018 | 0.015 | |
NID | PS | −0.011±0.122 | −0.082±0.096 | 0.035* |
WTVR | −0.040±0.104 | −0.115±0.090 | 0.022 | |
AP-VP | ||||
∆CT (HU) | PS | −3.158±19.662 | 8.951±10.300 | 0.043* |
WTVR | −16.510±13.059 | −5.042±5.998 | <0.001 | |
∆EDW (%) | PS | 0.037±0.362 | 0.328±0.434 | 0.019 |
WTVR | −0.169±0.171 | 0.100±0.237 | 0.002 | |
∆NZeff | PS | 0.054±0.024 | 0.085±0.017 | <0.001 |
WTVR | 0.036±0.020 | 0.065±0.012 | <0.001* | |
∆NID | PS | 0.123±0.091 | 0.223±0.060 | <0.001 |
WTVR | 0.096±0.090 | 0.197±0.051 | <0.001 |
Data are presented as the mean ± standard deviation. *, P value for Mann-Whitney U test. HCC, hepatocellular carcinoma; VP, venous phase; NZeff, normalized effective atomic number; NID, normalized iodine density; PS, planar sketching; WTVR, whole tumor volume rendering; AP-VP, the difference between the arterial phase and venous phase; CT, attenuation value of conventional computed tomography; HU, Hounsfield unit; EDW, electron density relative to water; ; ; ; .
According to ROC curve analysis (Figure 4), the detailed results of the area under the curve (AUC), 95% confidence interval (CI), cut-off value, sensitivity, specificity, and Youden index are shown in Table 4. The ∆NZeff parameters exhibited the best performance for predicting poorly differentiated HCC. The ∆NZeff in the PS method, the values for AUC, sensitivity, specificity, accuracy, and Youden index were 0.853, 0.840, 0.760, 0.786, and 0.614, respectively. In the WTVR method, the values for AUC, sensitivity, specificity, accuracy, and Youden index were 0.918, 1.000, 0.810, 0.857, and 0.814, respectively. The optimal cut-off value for ΔNZeff in the WTVR method was 0.054, and in the PS method was 0.071.
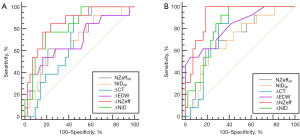
Table 4
Parameter | Method | AUC | 95% CI | Cut-off | Sensitivity | Specificity | Accuracy | Youden index |
---|---|---|---|---|---|---|---|---|
VP | ||||||||
NZeff | PS | 0.692 | 0.529–0.856 | −0.017 | 0.615 (8/13) | 0.791 (34/43) | 0.750 | 0.406 |
WTVR | 0.719 | 0.564–0.875 | −0.023 | 0.615 (8/13) | 0.814 (35/43) | 0.768 | 0.429 | |
NID | PS | 0.694 | 0.531–0.857 | −0.103 | 0.538 (7/13) | 0.814 (35/43) | 0.750 | 0.352 |
WTVR | 0.703 | 0.548–0.858 | −0.133 | 0.615 (8/13) | 0.791 (31/43) | 0.696 | 0.406 | |
AP-VP | ||||||||
∆CT (HU) | PS | 0.687 | 0.549–0.825 | −0.300 | 0.923 (12/13) | 0.558 (24/43) | 0.643 | 0.481 |
WTVR | 0.776 | 0.659–0.894 | −13.45 | 1.000 (13/13) | 0.605 (26/43) | 0.696 | 0.605 | |
∆EDW (%) | PS | 0.694 | 0.518–0.870 | 0.375 | 0.538 (7/13) | 0.837 (36/43) | 0.768 | 0.376 |
WTVR | 0.814 | 0.677–0.951 | 0.125 | 0.538 (7/13) | 0.953 (41/43) | 0.857 | 0.492 | |
∆NZeff | PS | 0.853 | 0.743–0.963 | 0.071 | 0.840 (11/13) | 0.760 (33/43) | 0.786 | 0.614 |
WTVR | 0.918 | 0.847–0.988 | 0.054 | 1.000 (13/13) | 0.810 (35/43) | 0.857 | 0.814 | |
∆NID | PS | 0.825 | 0.707–0.943 | 0.198 | 0.760 (10/13) | 0.814 (35/43) | 0.804 | 0.583 |
WTVR | 0.818 | 0.709–0.926 | 0.124 | 1.000 (13/13) | 0.600 (26/43) | 0.696 | 0.605 |
Data are presented as the values or values (numbers/total number of lesions). HCC, hepatocellular carcinoma; AUC, area under the curve; CI, confidence interval; VP, venous phase; NZeff, normalized effective atomic number; NID, normalized iodine density; PS, planar sketching; WTVR, whole tumor volume rendering; AP-VP, the difference between the arterial phase and venous phase; CT, attenuation value of conventional computed tomography; HU, Hounsfield unit; EDW, electron density relative to water; ; ; ; .
Correlation analysis between spectral parameters and pathological differentiation degree
The Spearman correlation analysis between the histologic grade of HCC (well-differentiated, moderately differentiated, and poorly differentiated) and the spectral parameters showed that some VP parameters (NZeff and NID) were moderately negatively correlated with the histologic grade, whereas some parameters (∆CT, ∆EDW, and ∆NID) were moderately positively correlated. Detailed information such as correlation coefficients is shown in Table 5. It is worth noting that ∆NZeff had a good correlation (PS: r=0.517, P<0.05; WTVR: r=0.611, P<0.05). The lower the degree of differentiation of HCC, the higher the difference in blood supply.
Table 5
Parameters | PS | WTVR | |||
---|---|---|---|---|---|
r | P value | r | P value | ||
VP | |||||
NZeff | −0.281 | 0.036 | −0.321 | 0.016 | |
NID | −0.284 | 0.034 | −0.297 | 0.026 | |
AP-VP | |||||
∆CT (HU) | 0.273 | 0.041 | 0.404 | 0.002 | |
∆EDW (%) | 0.284 | 0.034 | 0.460 | <0.001 | |
∆NZeff | 0.517 | <0.001 | 0.611 | <0.001 | |
∆NID | 0.475 | <0.001 | 0.464 | <0.001 |
HCC, hepatocellular carcinoma; PS, planar sketching; WTVR, whole tumor volume rendering; r, correlation coefficient rho; VP, venous phase; NZeff, normalized effective atomic number; NID, normalized iodine density; AP-VP, the difference between the arterial phase and venous phase; CT, attenuation value of conventional computed tomography; HU, Hounsfield unit; EDW, electron density relative to water; ; ; ; .
Discussion
We found that different ROI plotting methods substantially influence the measurement of spectral CT quantitative parameters. In this study, the average values of all quantitative parameters measured by the WTVR method are lower than those measured by the PS method, which is similar to the results of Zhong et al. (17). In his comparative study of spectral CT volume rendering and planar rendering methods, the CT values and effective atomic number were lower in volumetric spectral analysis than in conventional planar spectral analysis.
In our study, 2 parameters (NZeff, NID) in VP were statistically significant for distinguishing between poorly differentiated and non-poorly differentiated HCC. A comparison study of enhancement patterns and histologic differentiation of HCC under contrast-enhanced ultrasonic showed that well-differentiated nodules tend to wash out late or not at all, whereas poorly differentiated nodules tend to wash out rapidly (21). This is consistent with the results of our study. The NZeff and NID in VP of poorly differentiated HCC are significantly lower than those of non-poorly differentiated HCC. This can be explained that tumor stain washout becomes faster as the histological differentiation of HCC advances.
We initially mentioned the difference between arterial phase and venous phase (AP-VP) parameters after normalization. These parameters utilize the difference between the 2 phases to indicate the relative extent of arterial hypervascularity and portal vein phase washout. Li et al. (22) used the difference in iodine density (∆ID) between AP and VP to distinguish HCC from hepatic hemangioma and focal nodular hyperplasia, and ∆ID had higher diagnostic performance than conventional ID. Combined with previous studies, it has been shown that enhanced scanning based on the characteristics of angiogenesis and blood supply changes in HCC tumors has a high diagnostic value for HCC (23). With an increasing level of malignancy, the blood supply to the portal vein and hepatic artery decreases, whereas that to the abnormal artery gradually increases (24,25). The combined application of AP hyperenhancement and washout can improve the diagnostic specificity of HCC (26). This can also explain why AP-VP parameters (∆CT, ∆EDW, ∆NZeff, ∆NID) show higher diagnostic performance in evaluating poorly differentiated and non-poorly differentiated of HCC than VP parameters.
It is noteworthy that the ∆NZeff parameters exhibit the best diagnostic performance in distinguishing poorly differentiated from non-poorly differentiated cases. In the spectral parameters, materials composed of different elements may have the same CT value, making the differentiation and classification of various types of tissues extremely challenging (27). ID values can directly reflect the iodine contrast concentration in the tissues, providing information on perfusion and vascularity. ID and Zeff changes could be used to indicate the change in microcirculation (28), but ID cannot evaluate tissue heterogeneity within ROI. The effective atomic number describes the nature of interactions of materials or compounds with radiation (10), providing a more accurate representation of material characteristics compared to attenuation in HU (29). In early HCC, tumor cells are small and organized into thin beams. In late-stage HCC, there is poor differentiation, irregular cell shape, and a loose arrangement. Chen et al. (10) showed that in invasive colorectal adenocarcinoma, higher Zeff may be attributed to increased tumor heterogeneity, where the tumor tissue exhibited nuclear pleomorphism and abnormal elemental composition. In contrast, regions with lower Zeff imply the likelihood of less densely populated cellular structures or elements with lower atomic numbers (30). We believe that the standardized effective atomic number can better reflect the heterogeneity between HCC and may provide new markers for assessing poorly differentiated HCC.
For the ∆NZeff parameter, the AUC and Youden index were 0.853 and 0.614, respectively, in the PS method, and the AUC and Youden index were 0.918 and 0.814, respectively, in the WTVR method. Compared to the PS method, the WTVR method had a higher AUC and Youden index for distinguishing different differentiated HCC groups. Zhong et al. (17) showed that volumetric quantitative analysis had a significant advantage in the whole lesion observation range. Liu et al. (8) also showed that the measurement of magnetic resonance elastography hardness obtained by the whole tumor volumetric analysis is superior to PS ROI-based methods for predicting the grade of HCC. All these are consistent with our results. Among all the spectral parameters with differential diagnosis, the WTVR method was superior to the PS method in terms of AUC value and Youden index. This is because the WTV method involves the sampling of the whole tumor which can effectively reduce the bias caused by sampling errors. Furthermore, the intratumor heterogeneity can be better captured by using the whole tumor volumetric analysis and is better for determining the dominant grade of tumor tissue (5). The WTVR method outlines the non-enhanced part of the tumor except the enhanced part of the tumor, and tumor enhancement with non-enhanced areas is a valuable finding on contrast CT in the prediction of poorly differentiated HCC (31). These findings suggest that volumetric measurement may be a better indicator of tumor heterogeneity, so it may be more suitable to evaluate tumor pathological differentiation, but these findings need to be confirmed in larger studies in the future.
There are some limitations of our study. First, the sample size of this study was small. The combination of well-differentiated and moderately differentiated HCC was considered as the non-poorly differentiation group, whereas the sample size of the poorly differentiated group was limited, which resulted in less and uneven data. Second, this was a single-center study, and the repeatability of the study needs to be verified. Meanwhile, due to sampling errors in biopsies, we were unable to determine the degree of HCC differentiation in the biopsy results for some patients, resulting in their exclusion, which is also a limitation of biopsy. Given that this article solely focuses on the relationship between the degree of differentiation of HCC and spectral parameters, without delving into other pathological findings, further research will be conducted with sufficient patient data in the future. Finally, the measurement parameters in this study were limited to a single DLCT, so further verification is needed to determine the applicability of the same type of parameter values measured by different manufacturers and different dual-energy CT parameter calculation methods, as well as the normalized parameter results.
Conclusions
Volumetric quantitative analysis of DLCT is a valuable non-invasive technique for distinguishing the histological grading of HCC and deserves clinical recommendation. Compared to planar analysis, volumetric analysis yields lower spectral parameter measurements, but it enhances the performance of quantitative parameters in distinguishing histological grades of HCC. Therefore, when quantitatively evaluating the pathological grade of HCC using spectral parameters, the ROI should include as much of the tumor as possible to enhance discrimination efficiency. Additionally, the ∆NZeff plotted in the WTVR method has the highest diagnostic performance in distinguishing between poorly differentiated and non-poorly differentiated HCC.
Acknowledgments
The authors thank the Department of Medical Imaging Center, Nanfang Hospital for providing CT images and clinical histopathology reports of HCCs for this study. The authors also thank the Department of Clinical and Technical Support, Philips Healthcare Inc. for guiding the data measurement on the post-processing workstation.
Funding: This work was supported by
Footnote
Reporting Checklist: The authors have completed the STARD reporting checklist. Available at https://qims.amegroups.com/article/view/10.21037/qims-23-1753/rc
Conflicts of Interest: All authors have completed the ICMJE uniform disclosure form (available at https://qims.amegroups.com/article/view/10.21037/qims-23-1753/coif). X.L. is employed by Philips HealthCare China, Inc. The other authors have no conflicts of interest to declare.
Ethical Statement: The authors are accountable for all aspects of the work in ensuring that questions related to the accuracy or integrity of any part of the work are appropriately investigated and resolved. The study was conducted in accordance with the Declaration of Helsinki (as revised in 2013). The study was approved by the institutional ethics board of Nanfang Hospital (No. NFEC-2023-521) and the requirement for individual consent for this retrospective analysis was waived.
Open Access Statement: This is an Open Access article distributed in accordance with the Creative Commons Attribution-NonCommercial-NoDerivs 4.0 International License (CC BY-NC-ND 4.0), which permits the non-commercial replication and distribution of the article with the strict proviso that no changes or edits are made and the original work is properly cited (including links to both the formal publication through the relevant DOI and the license). See: https://creativecommons.org/licenses/by-nc-nd/4.0/.
References
- Bray F, Ferlay J, Soerjomataram I, Siegel RL, Torre LA, Jemal A. Global cancer statistics 2018: GLOBOCAN estimates of incidence and mortality worldwide for 36 cancers in 185 countries. CA Cancer J Clin 2018;68:394-424. [Crossref] [PubMed]
- Rong D, Liu W, Kuang S, Xie S, Chen Z, Chen F, Xie Q, Luo Q, He B, Zhang Y, Deng Y, Yang H, Chen S, Wang J. Preoperative prediction of pathologic grade of HCC on gadobenate dimeglumine-enhanced dynamic MRI. Eur Radiol 2021;31:7584-93. [Crossref] [PubMed]
- Allemani C, Matsuda T, Di Carlo V, Harewood R, Matz M, Nikšić M, et al. Global surveillance of trends in cancer survival 2000-14 (CONCORD-3): analysis of individual records for 37 513 025 patients diagnosed with one of 18 cancers from 322 population-based registries in 71 countries. Lancet 2018;391:1023-75. [Crossref] [PubMed]
- Court CM, Harlander-Locke MP, Markovic D, French SW, Naini BV, Lu DS, Raman SS, Kaldas FM, Zarrinpar A, Farmer DG, Finn RS, Sadeghi S, Tomlinson JS, Busuttil RW, Agopian VG. Determination of hepatocellular carcinoma grade by needle biopsy is unreliable for liver transplant candidate selection. Liver Transpl 2017;23:1123-32. [Crossref] [PubMed]
- Wei Y, Gao F, Wang M, Huang Z, Tang H, Li J, Wang Y, Zhang T, Wei X, Zheng D, Song B. Intravoxel incoherent motion diffusion-weighted imaging for assessment of histologic grade of hepatocellular carcinoma: comparison of three methods for positioning region of interest. Eur Radiol 2019;29:535-44. [Crossref] [PubMed]
- Stigliano R, Marelli L, Yu D, Davies N, Patch D, Burroughs AK. Seeding following percutaneous diagnostic and therapeutic approaches for hepatocellular carcinoma. What is the risk and the outcome? Seeding risk for percutaneous approach of HCC. Cancer Treat Rev 2007;33:437-47. [Crossref] [PubMed]
- Xu YS, Liu HF, Xi DL, Li JK, Liu Z, Yan RF, Lei JQ. Whole-lesion histogram analysis metrics of the apparent diffusion coefficient: a correlation study with histological grade of hepatocellular carcinoma. Abdom Radiol (NY) 2019;44:3089-98. [Crossref] [PubMed]
- Liu W, Rong D, Zhu J, Xiao Y, Zhang L, Deng Y, Chen J, Yin M, Venkatesh SK, Ehman RL, Wang J. Diagnostic accuracy of 3D magnetic resonance elastography for assessing histologic grade of hepatocellular carcinoma: comparison of three methods for positioning region of interest. Abdom Radiol (NY) 2021;46:4601-9. [Crossref] [PubMed]
- Kulkarni NM, Fung A, Kambadakone AR, Yeh BM. Computed Tomography Techniques, Protocols, Advancements, and Future Directions in Liver Diseases. Magn Reson Imaging Clin N Am 2021;29:305-20. [Crossref] [PubMed]
- Chen W, Ye Y, Zhang D, Mao L, Guo L, Zhang H, Du X, Deng W, Liu B, Liu X. Utility of dual-layer spectral-detector CT imaging for predicting pathological tumor stages and histologic grades of colorectal adenocarcinoma. Front Oncol 2022;12:1002592. [Crossref] [PubMed]
- He Y, Qi X, Luo X, Wang W, Yang H, Xu M, Wu X, Fan W. The clinical value of dual-energy CT imaging in preoperative evaluation of pathological types of gastric cancer. Technol Health Care 2023;31:1799-808. [Crossref] [PubMed]
- Lin LY, Zhang Y, Suo ST, Zhang F, Cheng JJ, Wu HW. Correlation between dual-energy spectral CT imaging parameters and pathological grades of non-small cell lung cancer. Clin Radiol 2018;73:412.e1-7. [Crossref] [PubMed]
- Wang Y, Tian W, Tian S, He L, Xia J, Zhang J. Spectral CT - a new supplementary method for preoperative assessment of pathological grades of esophageal squamous cell carcinoma. BMC Med Imaging 2023;23:110. [Crossref] [PubMed]
- Zhang X, Zhang G, Xu L, Bai X, Zhang J, Chen L, Lu X, Yu S, Jin Z, Sun H. Prediction of World Health Organization /International Society of Urological Pathology (WHO/ISUP) Pathological Grading of Clear Cell Renal Cell Carcinoma by Dual-Layer Spectral CT. Acad Radiol 2023;30:2321-8. [Crossref] [PubMed]
- Yoon JH, Lee JM, Kim JH, Lee KB, Kim H, Hong SK, Yi NJ, Lee KW, Suh KS. Hepatic fibrosis grading with extracellular volume fraction from iodine mapping in spectral liver CT. Eur J Radiol 2021;137:109604. [Crossref] [PubMed]
- Priola AM, Priola SM, Parlatano D, Gned D, Giraudo MT, Giardino R, Ferrero B, Ardissone F, Veltri A. Apparent diffusion coefficient measurements in diffusion-weighted magnetic resonance imaging of the anterior mediastinum: inter-observer reproducibility of five different methods of region-of-interest positioning. Eur Radiol 2017;27:1386-94. [Crossref] [PubMed]
- Zhong LJ, Yu N, Zhou XJ, Fu LZ, Zhou DQ, Wang Y, Yan M. Differentiating between pulmonary adenocarcinoma and squamous cell carcinoma by spectral CT volumetric quantitative analysis: a comparative study with conventional spectral analysis. J Thorac Dis 2023;15:679-89. [Crossref] [PubMed]
- Liu YI, Shin LK, Jeffrey RB, Kamaya A. Quantitatively defining washout in hepatocellular carcinoma. AJR Am J Roentgenol 2013;200:84-9. [Crossref] [PubMed]
- Nagtegaal ID, Odze RD, Klimstra D, Paradis V, Rugge M, Schirmacher P, Washington KM, Carneiro F, Cree IA. The 2019 WHO classification of tumours of the digestive system. Histopathology 2020;76:182-8. [Crossref] [PubMed]
- Chernyak V, Fowler KJ, Kamaya A, Kielar AZ, Elsayes KM, Bashir MR, Kono Y, Do RK, Mitchell DG, Singal AG, Tang A, Sirlin CB. Liver Imaging Reporting and Data System (LI-RADS) Version 2018: Imaging of Hepatocellular Carcinoma in At-Risk Patients. Radiology 2018;289:816-30. [Crossref] [PubMed]
- Jang HJ, Kim TK, Burns PN, Wilson SR. Enhancement patterns of hepatocellular carcinoma at contrast-enhanced US: comparison with histologic differentiation. Radiology 2007;244:898-906. [Crossref] [PubMed]
- Li W, Li R, Zhao X, Lin X, Yu Y, Zhang J, Chen K, Chai W, Yan F. Differentiation of Hepatocellular Carcinoma from Hepatic Hemangioma and Focal Nodular Hyperplasia using Computed Tomographic Spectral Imaging. J Clin Transl Hepatol 2021;9:315-23. [Crossref] [PubMed]
- Tang A, Bashir MR, Corwin MT, Cruite I, Dietrich CF, Do RKG, Ehman EC, Fowler KJ, Hussain HK, Jha RC, Karam AR, Mamidipalli A, Marks RM, Mitchell DG, Morgan TA, Ohliger MA, Shah A, Vu KN, Sirlin CB. Evidence Supporting LI-RADS Major Features for CT- and MR Imaging-based Diagnosis of Hepatocellular Carcinoma: A Systematic Review. Radiology 2018;286:29-48. [Crossref] [PubMed]
- Liao Z, Tang C, Luo R, Gu X, Zhou J, Gao J. Current Concepts of Precancerous Lesions of Hepatocellular Carcinoma: Recent Progress in Diagnosis. Diagnostics (Basel) 2023;13:1211. [Crossref] [PubMed]
- Matsui O. Imaging of multistep human hepatocarcinogenesis by CT during intra-arterial contrast injection. Intervirology 2004;47:271-6. [Crossref] [PubMed]
- Rimola J, Forner A, Tremosini S, Reig M, Vilana R, Bianchi L, Rodríguez-Lope C, Solé M, Ayuso C, Bruix J. Non-invasive diagnosis of hepatocellular carcinoma ≤ 2 cm in cirrhosis. Diagnostic accuracy assessing fat, capsule and signal intensity at dynamic MRI. J Hepatol 2012;56:1317-23. [Crossref] [PubMed]
- McCollough CH, Leng S, Yu L, Fletcher JG. Dual- and Multi-Energy CT: Principles, Technical Approaches, and Clinical Applications. Radiology 2015;276:637-53. [Crossref] [PubMed]
- Zhu Y, Feng B, Cai W, Wang B, Meng X, Wang S, Ma X, Zhao X. Prediction of Microvascular Invasion in Solitary AFP-Negative Hepatocellular Carcinoma ≤ 5 cm Using a Combination of Imaging Features and Quantitative Dual-Layer Spectral-Detector CT Parameters. Acad Radiol 2023;30:S104-16. [Crossref] [PubMed]
- Hua CH, Shapira N, Merchant TE, Klahr P, Yagil Y. Accuracy of electron density, effective atomic number, and iodine concentration determination with a dual-layer dual-energy computed tomography system. Med Phys 2018;45:2486-97. [Crossref] [PubMed]
- Piacentino F, Fontana F, Zorzetto G, Saccomanno A, Gatta T, Recaldini C, et al. Dual-Layer Spectral CT as Innovative Imaging Guidance in Lung Biopsies: Could Color-Coded Z-Effective Images Allow More Diagnostic Samplings and Biomarkers Information? J Clin Med 2023;12:7426. [Crossref] [PubMed]
- Nakachi K, Tamai H, Mori Y, Shingaki N, Moribata K, Deguchi H, Ueda K, Inoue I, Maekita T, Iguchi M, Kato J, Ichinose M. Prediction of poorly differentiated hepatocellular carcinoma using contrast computed tomography. Cancer Imaging 2014;14:7. [Crossref] [PubMed]