Pixelwise Gradient Model for Image Fusion (PGMIF): a multi-sequence magnetic resonance imaging (MRI) fusion model for tumor contrast enhancement of nasopharyngeal carcinoma
Introduction
The rapid development in imaging and radiation therapy technologies has allowed for a more accurate administration of a high ablative radiation dose to tumors (1-3). This increased precision has resulted in a better prognosis for patients with nasopharyngeal carcinoma (NPC) (4-7). Irrespective of the chosen radiation therapy method, accurate tumor demarcation is critical for the success of the treatment (8-10). Any misrepresentation of the tumor boundary is a significant error source, potentially resulting in missing the target during therapy. This mistake can drastically affect the dosage received by the tumor and nearby healthy tissue. Therefore, it is vital to accurately visualize the tumor and its borders within the normal tissue (8). Magnetic resonance imaging (MRI) is particularly effective for NPC cases because it provides a superior soft-tissue contrast. This offers a clearer view of tumor boundary compared to CT scans. Therefore, MRI is the preferred method for target delineation.
Nevertheless, the current MRI-based target delineation approach in radiation therapy has some drawbacks: (I) only one set of magnetic resonance (MR) sequences with a single weighting contrast can be examined at once, making the process of reviewing multiple MR image sets during target delineation quite time-consuming (11); (II) the tumor contrast can greatly vary between patients, leading to an increased uncertainty in target delineation (11). To address these drawbacks, significant advances have been made in the field of image fusion. Image fusion refers to the process of combining complementary information from multiple imaging sources to produce a single, composite image that is more informative and complete than any of the individual images alone. This technique has emerged as a vital tool in the process of tumor delineation in radiation therapy planning (12). Image fusion can blend the superior soft-tissue contrast of MRI images with the high-resolution anatomical information of computed tomography (CT) scans or the metabolic information of positron emission tomography (PET) scans (13). This fusion provides a comprehensive and precise representation of the tumor’s boundary, enhancing the accuracy of radiation therapy planning for NPC patients (14). Moreover, by synthesizing multiple sets of image data, fusion reduces the variation in tumor contrast between MR sequences. This feature allows for consistent and reliable target delineation, reducing uncertainties associated with single-modality images (15).
Image fusion can be used to assist tumor delineation (16). Tumor delineation plays an essential role in radiotherapy planning for NPC. Accurate delineation is crucial for determining the radiation dose to be delivered to the tumor while minimizing exposure to adjacent healthy tissues. Conventional techniques such as CT and MRI are commonly used for this purpose. However, these methods have limitations in terms of soft tissue contrast and may not provide adequate demarcation between the tumor and surrounding structures like the parapharyngeal space. By performing medical image fusion, it becomes possible to capture and amplify the image contrast in patients with NPC, thereby offering the potential for precise tumor delineation.
As imaging technology and computing capabilities continue to evolve, there is an increasing interest in using machine learning and deep learning techniques in the image fusion process (17). These advanced algorithms have the potential to automatically learn optimal fusion strategies from large datasets, which could lead to more reliable and efficient workflows. Current results from studies integrating machine learning in image fusion the use of image fusion in radiotherapy (12,18). Moreover, current image fusion methods are not without its potential risks and limitations. For example, the integration of images from multiple modalities can sometimes introduce artifacts or misregistration errors, which could lead to inaccurate tumor delineation. Hence, careful quality control measures are necessary, including rigorous clinical validation against known standards and regular audits of fusion processes and outcomes (12). Furthermore, the time and computational resources required for image fusion can be significant, which may pose practical constraints in busy clinical settings. Ongoing research aims to streamline this process, focusing on algorithm efficiency, automation, and integration into existing clinical workflows, aiming to make image fusion a routine and reliable component of modern radiation therapy planning (19).
The goal of this paper is to introduce a method, Pixelwise Gradient Model for Image Fusion (PGMIF), which is the best performed model compared to other fusion models introduced in later sections, to fuse images. PGMIF is based on the gradient method. The key idea of the gradient method is to use pixelwise gradient to capture the shape of the input images. Then by reproducing the input gradient with suitable amplification to the output fused images, the fused ones will capture the desired features of the input images. This model is an accurate and efficient method to produce fused images which can capture both the anatomical structures and tumor contrast clearly. It is expected that the fusion model PGMIF can be used to assist radiotherapy due to its enhancement of tumor contrast and may serve as the images for tumor segmentation.
Methods
In this section, PGMIF and several models, including Gradient Model with Maximum Comparison among Images (GMMCI), Deep Learning Model with Weighted Loss (DLMWL), Pixelwise Weighted Average (PWA) and Maximum of Images (MoI), for image fusion are introduced.
PGMIF
In this method, we train the model so that the output images capture the shape and image contrast of the input images using the pixelwise gradient method and the generative adversarial network (GAN). The architecture is shown in Figure 1. The gradient method is originated in the studies of Haber et al. 2006, Rühaak et al. 2013 and König et al. 2014 (20-22) in the context of image registration. We observe that in the edge of the NPC tumor, if the tumor contrast is enhanced, there will be large gradient change in pixel intensity in this region. We adapt this technique to the fusion task as follows: to learn the shape of the input images, we first take the gradient of an image
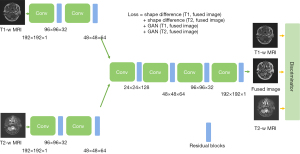
where represents the pixel of image. Then consider the normalized gradients , where is a small constant to avoid division of 0. If the output images capture the same shape as the input images, the gradient will point to the same or opposite directions as the gradient of pixel intensity is a geometric quantity that depends only on the anatomical structure instead of any particular image modality. Square of dot product between the input and output normalized gradient measure how much the gradient aligned with each other. Therefore, it is used for the loss function. This dot product encourages the output to learn the shape of the input.
where , are the input and output images, respectively and and are the normalized gradients of the input and output images, respectively.
To learn the image contrast of the input images, GAN terms between the input images and output images are used. This term is to ensure the output images have similar image contrast as the input, where the definition of GAN follows the paper (23).
Model architecture and training protocol
The PGMIF model consists of encoders and decoders, which contains 3 convolutional layers followed by batch normalization and rectified linear unit (ReLU) activation layers. The final layer is designed to generate fused images with preserved shapes and enhanced image contrast. We utilize Adam optimizer with a learning rate of 0.0002 and a batch size of 32. The model is trained for 50 epochs. Since the contrast of the tumor in T2-weighted (T2-w) MR images is not so sharp, more weighting is added to the loss term of the T2 gradient term. A ratio of 1:8 is taken for gradient loss of T1-weighted (T1-w) MR images to and T2-w MR images to illustrate the performance.
The model is implemented using Python and Pytorch. It is run in a computer with CPU 11900K and GPU RTX-3090. We performed 14,000 iterations for training.
Comparison fusion models
GMMCI
We use methods similar to previous PGMIF, which also involves pixelwise gradients and GAN term. We would like to capture the image contrast at the pixels where there are large gradient change, so this method just, at each pixel, captures the gradient change of the input image with largest gradient change. The pixelwise gradient term could be formulated as:
where and are the normalized gradient of the image with maximum gradient change and the output images, respectively, is the gradient of the maximum gradient change.
In case if that part of the anatomical structure, which is the entity indicating the structure of the brain, is not well captured in that image contrast, the gradient change of that part of this image contrast will be less than that of other image contrasts, so the maximum algorithm will pick other images’ anatomical information for the fused image.
DLMWL
In this method, CNN are applied to the T1-w and T2-w images. Figure 2 shows the architecture of the model. We would like to train a deep learning model that capture the shapes of input T1-w and T2-w MR images. So mean square error (MSE) between the fused image and the input images are considered respectively. Observe that if the intensity of the images with highest image intensity is captured at each pixel, the fused image would have clear anatomical structure. Therefore, we would like the fused image to incline to the image with larger image intensity. A term is multiplied to increase the weight with image with larger image contrast. A natural choice would be the image contrast itself.
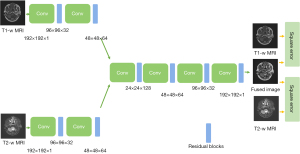
where and are the intensity of the T1-w and T2-w images, represents the square error at pixel i of the two images and the product is a scalar product.
In order to ensure the image intensity lies between 0 and 1, GAN terms between the fused images and T1-w images and T2-w images are added. We utilize Adam optimizer with a learning rate of 0.0001 and a batch size of 32. The model is trained for 50 epochs. The ratio between the MSE and the GAN terms is set to be 1:3, which is found to be optimal by error and trial.
PWA
This method is not a deep learning method. In order to construct fused images that capture the desired features of the input, averages on the pixel intensity is taken. To encourage the fused image to incline to input image with large image intensity, more weight is given to that input images and the weight is pixelwise. A natural choice for the weight would be the image intensity itself. So the image will multiply the image contrast itself.
where and are the pixelwise image intensities at pixel i of two input images.
MoI
We would like the fused image to capture the part of the input images with largest image contrast. Therefore, this method considers the maximum of the all the input images.
at pixel , where and are the image intensities of input images
Data
The study was conducted in accordance with the Declaration of Helsinki (as revised in 2013). The study was approved by Research Ethics Committee in Hong Kong (Kowloon Central/Kowloon East, reference number: KC/KE-19-0085/ER-1) and individual consent for this retrospective analysis was waived. 80 NPC patient of stage I to IVb T1-w, T2-w MRI images are used retrospectively. The T1-w and T2-w images are scanned using a 3T-Siemens scanner with TR: 620 ms, TE: 9.8 ms; TR: 2,500 ms, TE: 74 ms, respectively. The average age of the selected patients is 57.6±8.6 years old. Forty-six of them are males, 34 are females. One three-dimensional (3D) image is acquired for each patient and we divide the 3D images to two-dimensional (2D) slices for training. We performed min-max normalization on each slice (min-max-each-slice) of the images to 0 and 1. Since image fusion required the images to be aligned, the 3D T1-w MR images are registered with T2-w MR images using 3dSlicer. The registration of 3D T1-w MR images to T2-w MR images was performed using 3dSlicer with a B-Spline transform and mutual information as the similarity metric. The quality of the registration of whether the anatomical structures are aligned is checked by visual inspection. Then different 2D slices, which are the 2D section of the 3D images, were extracted from the 3D images. We randomly select 70 patients for training and 10 for testing, resulting in 3,051 2D image slices for each of the T1-w images and T2-w images for training and 449 2D slices for each of the T1-w images and T2-w images for testing. After that, the 2D slices are resized to 192×192. This size was chosen because it was a multiple of 64, so this would not cause the dimension problem of the convolutional network.
Evaluation methods
We use two different metrics to quantify the performance of the fusion models. The first one is the tumor contrast-to-noise ratio (CNR), which is defined as
where and are the mean and standard deviation (SD) of the regional intensities, respectively. The CNR is used to check if the image contrast of the tumor to the noise is enhanced. The tumor and non-tumor represent the gross tumor volume (GTV) contoured by physicians and a nearby homogenous region, respectively. Then we compute the inter-patient (IP) mean, SD, and coefficient of variation (CV). The CV of CNR is defined as
where i =1 to 10 represents the 10 testing patients.
The second metric is to use Sobel Operator for edge detection to explore the intensity enhancement of the tumor site. We first crop the images with the tumor parts only. Then we performed Sobel operator to detect the edge by:
These Sx and Sy detect how much change in that pixel and is the image. After that we calculate .
In Petrović et al. 2005 (24), they consider
at pixel , where is the fused image and is the input image. is largest when of the fused image is the same as the original one. Since we would like to encourage contrast enhancement, we modify the definition as . The larger , the sharper image contrast enhanced. Since the perceptual loss of edge strength information is modeled by sigmoid nonlinearities. We define
and the Generalized Sobel Operator as the parameter to measure the sharpness of edge. It ranges from 0 to 1.
The Generalized Sobel Operator is applied to the GTV region.
P value of Mann-Whitney test of the null hypothesis of whether the tumor CNR ratio and the Generalized Sobel Operator of PGMIF is equal to that of other models are reported. A P value <0.05 is considered statistically significant for claiming the tumor CNR ratio and the Generalized Sobel Operator of PGMIF is greater than other models. The tumor CNR and Generalized Sobel Operator are calculated using MATLAB.
Results
Figure 3 shows the fused images using different fusion methods. It can be seen that the tumor contrast of PGMIF is the sharpest, while the tumor contrast of the other fusion methods is not so sharp. To illustrate the performance of the best model, PGMIF, Figure 4 shows more fused images using PGMIF.
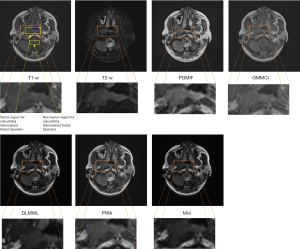
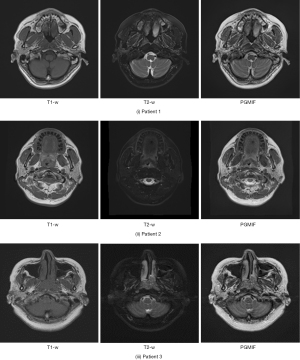
Tumor CNR
Figure 5A shows the comparison of the CNR between different fusion methods and original T1-w MR images and T2-w MR images, respectively. Firstly, it can be observed that the fused MR images using PGMIF achieved the highest CNR [median (mdn) =1.208, interquartile range (IQR) =1.175–1.381], leading to a statistically significant enhancement as compared to that of T1-w MR images (mdn =1.044, IQR =0.957–1.042, P<5.60×10−4) and T2-w MR images (mdn =1.111, IQR =1.023–1.182, P<2.40×10−3). These show that the fused images PGMIF enhances the tumor contrast or reduces the noise. Comparison of PGMIF with other fusion models are as follows: GMMCI (mdn =0.967, IQR =0.795–0.982, P<5.60×10−4), DLMWL (mdn =0.883, IQR =0.832–0.943, P<5.60×10−4), PWA (mdn =0.875, IQR =0.806–0.972, P<5.60×10−4) and MoI (mdn =0.863, IQR =0.823–0.991, P<5.60×10−4). These indicate that PGMIF outperforms other models in terms of tumor contrast enhancement. The IP CV of tumor CNR was the lowest in the PGMIF (31.4%), followed by GMMCI (36.8%), PWA (42.6%), T2-w MR images (43.9%), DLMWL (52.1%), T1-w MR images (58.6%) and MoI (62.3%).
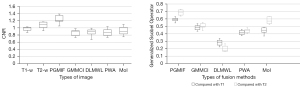
Generalized Sobel Operator Analysis
Figure 5B shows the comparison of the Generalized Sobel Operator between different fusion methods and original T1-w and T2-w MR images, respectively. It can be observed that the fused MR images using PGMIF achieved the highest Generalized Sobel Operator (mdn =0.594, IQR =0.579–0.607 for comparison with T1-w MR images and mdn =0.692, IQR =0.651–0.718 for comparison with T2-w MR images), leading to a statistically significant enhancement as compared to that of GMMCI (mdn =0.491, IQR =0.458–0.507, P<5.60×10−4, for comparison with T1-w MR images; mdn =0.495, IQR =0.487–0.533, P<5.60×10−4, for comparison with T2-w MR images), DLMWL (mdn =0.292, IQR =0.248–0.317, P<5.60×10−4, for comparison with T1-w MR images; mdn =0.191, IQR =0.179–0.243, P<5.60×10−4, for comparison with T2-w MR images), PWA (mdn =0.423, IQR =0.383–0.455, P<5.60×10−4, for comparison with T1-w MR images; mdn =0.448, IQR =0.414–0.463, P<5.60×10−4, for comparison with T2-w MR images) and MoI (mdn =0.437, IQR =0.406–0.479, P<5.60×10−4, for comparison with T1-w MR images; mdn =0.540, IQR =0.521–0.636, for comparison with T2-w MR images).
Discussion
By amplifying and reproducing these gradients into the fused output images, our proposed PGMIF method of fusion has the potential to enhance both the tumor contrast captured in T2-w images and the anatomical structure captured by T1-w images. Our method capitalizes on the pixelwise gradient analysis to delineate the intricate structures within the input images. This integration results in images with significantly improved clarity and information content, addressing the identified uncertainties associated with MRI-based target delineation.
This study also underscores the potential of image fusion, and specifically the PGMIF method, in adaptive radiation therapy (ART). As NPC patients often experience anatomical changes during their treatment course due to various factors including weight loss and tumor shrinkage, the ability to continually adapt the treatment plan is crucial. Image fusion, via the PGMIF method, allows for this flexibility, providing precise images that can inform real-time adjustments to treatment plans. This adaptability ensures that the radiation doses remain optimally focused on the tumor while minimizing exposure to surrounding healthy tissues, thus promising better treatment outcomes and reduced side effects for patient.
The time needed to run PGMIF is also within reasonable time in clinical setting. The time needed to run a pair of T1-w and T2-w images is within 0.1 s while time needed to train the model is around 2 hours for 120,000 iterations.
For clinical applications, regardless of the selected radiotherapy method (be it intensity-modulated radiotherapy, stereotactic body radiotherapy, or others), accurately outlining the tumor is critical for a successful radiotherapy outcome. Mistakes in defining the tumor boundaries can lead to a missed target during treatment, affecting the dosage to both the tumor and surrounding healthy tissues. Proper visualization of the tumor and its borders among the normal tissues is essential for accurate delineation.
Nevertheless, there are certain shortcomings in the prevailing MRI-based target identification process in radiotherapy. First, only a single MR sequence with one contrast weighting can be assessed at once, which prolongs the time required to scrutinize various MR image sets during the delineation process. Secondly, the contrast of the tumor may differ significantly among patients, introducing variations and, consequently, uncertainties in defining the target. The image fusion method, as demonstrated in our study, can amplify MRI tumor contrast and its uniformity among patients. This method offers a potential solution to the previously mentioned MRI-based target definition challenges for NPC.
The two original MR image sets (T1-w, T2-w MR images) were used as input for the fusion method in this study. These images are commonly used in NPC radiotherapy treatment planning and are typically included in routine MR imaging protocol. It is noted that the current study is not limited to T1-w and T2-w MR images, more image modalities, like CT and contrast enhanced T1 (T1C), could also be included. It is expected that the performance of the model will be improved because additional image modalities provide more information on the tumor and anatomical structure. Further studies on fusing more image modalities will be left for future research direction.
From the quantitative analysis, it is found that the performance of PGMIF is the best based on Tumor CNR Analysis and Generalized Sobel Operator Analysis. Using this method, both the anatomical contrast of T1-w MR images and tumor contrast of T2-w MR images are captured. The disadvantage of DLMWL is tuning the hyperparameters might require an extensive search or even a trial-and-error approach. The PWA generates a less sharp fused image as the averaging process inherently smoothens the image (Figure 3). This method does not take into consideration the spatial information and differences in image contrast between the input images. As a result, this method fails to optimally combine the anatomical structure and tumor contrast in the fused image. By considering the pixel intensities in the weighting process, PWA method aims to preserve the image contrast from the input images. However, this method may suffer from artifacts and noise amplification due to intensity-based weighting (Figure 3). The MoI method shows promising results in preserving the anatomical and tumor contrast from both input images. However, this method can introduce abrupt transitions in the fused image, especially in regions where the intensities of the input images vary significantly (Figure 3). This might result in a fused image that does not accurately represent the underlying anatomical structure and tumor contrast.
In summary, PGMIF outperforms the other proposed methods in capturing the anatomical structure and tumor contrast in the fused image without the requirement of error-and-trial. The other methods, although they provide some benefits, exhibit limitations in preserving the essential information from both input images or require extensive parameter tuning. However, the current gradient-based method has a limitation. Since the fused images are based on the gradient of the input ones, the ripples of the input images were amplified in the fused ones. If the output fused images are enlarged, it is seen that the bright regions from T1-w MR images have some noise coming from T2-w MR images. Moreover, the model generalizability of our model has not been tested at current stage. Future research direction may to test the fusion performance of multi-hospital images.
Conclusions
In conclusion, this study introduces and thoroughly evaluates the PGMIF as a novel method for multi-modal image fusion in the context of radiation therapy planning for NPC. As depicted in the results section, PGMIF outperforms other state-of-the-art fusion algorithms, as validated by both qualitative and quantitative analysis. Compared with studies like Cheung et al. 2022 (25), our method does not require error and trial and in principle produce sharp image contrast. However, it should be noted that the study is limited to one dataset from one institution and no external testing was done to evaluate generalizability beyond the given dataset. For future research directions, it would be interesting to compare the result of the presented fusion result with other tumor contrast enhancement methods using deep learning methods, like virtual contrast enhanced T1 images Li et al. 2022, 2023 (26,27). One possible comparison method would be using segmentation of the tumor region to evaluate the methods. This would be a method to test if the tumor contrast of the fused images is enhanced.
Acknowledgments
Funding: This research was partly supported by research grants of
Footnote
Conflicts of Interest: All authors have completed the ICMJE uniform disclosure form (available at https://qims.amegroups.com/article/view/10.21037/qims-23-1559/coif). J.C. reports that this research was partly supported by research grants of Shenzhen Basic Research Program (No. JCYJ20210324130209023) of Shenzhen Science and Technology Innovation Committee, Project of Strategic Importance Fund (No. P0035421) and Projects of RISA (No. P0043001) from The Hong Kong Polytechnic University of The Hong Kong Polytechnic University, Mainland-Hong Kong Joint Funding Scheme (MHKJFS) (No. MHP/005/20), and Health and Medical Research Fund (No. HMRF 09200576), the Health Bureau, The Government of the Hong Kong Special Administrative Region. The other authors have no conflicts of interest to declare.
Ethical Statement: The authors are accountable for all aspects of the work in ensuring that questions related to the accuracy or integrity of any part of the work are appropriately investigated and resolved. The study was conducted in accordance with the Declaration of Helsinki (as revised in 2013). The study was approved by Research Ethics Committee in Hong Kong (Kowloon Central/Kowloon East, reference number: KC/KE-19-0085/ER-1) and individual consent for this retrospective analysis was waived.
Open Access Statement: This is an Open Access article distributed in accordance with the Creative Commons Attribution-NonCommercial-NoDerivs 4.0 International License (CC BY-NC-ND 4.0), which permits the non-commercial replication and distribution of the article with the strict proviso that no changes or edits are made and the original work is properly cited (including links to both the formal publication through the relevant DOI and the license). See: https://creativecommons.org/licenses/by-nc-nd/4.0/.
References
- Gao Y, Liu R, Chang CW, Charyyev S, Zhou J, Bradley JD, Liu T, Yang X. A potential revolution in cancer treatment: A topical review of FLASH radiotherapy. J Appl Clin Med Phys 2022;23:e13790. [Crossref] [PubMed]
- Zhang Z, Liu X, Chen D, Yu J. Radiotherapy combined with immunotherapy: the dawn of cancer treatment. Signal Transduct Target Ther 2022;7:258. [Crossref] [PubMed]
- Hamdy FC, Donovan JL, Lane JA, Metcalfe C, Davis M, Turner EL, et al. Fifteen-Year Outcomes after Monitoring, Surgery, or Radiotherapy for Prostate Cancer. N Engl J Med 2023;388:1547-58. [Crossref] [PubMed]
- Ng WT, Chow JCH, Beitler JJ, Corry J, Mendenhall W, Lee AWM, Robbins KT, Nuyts S, Saba NF, Smee R, Stokes WA, Strojan P, Ferlito A. Current Radiotherapy Considerations for Nasopharyngeal Carcinoma. Cancers (Basel) 2022;14:5773. [Crossref] [PubMed]
- Chen D, Cai SB, Soon YY, Cheo T, Vellayappan B, Tan CW, Ho F. Dosimetric comparison between Intensity Modulated Radiation Therapy (IMRT) vs dual arc Volumetric Arc Therapy (VMAT) for nasopharyngeal cancer (NPC): Systematic review and meta-analysis. J Med Imaging Radiat Sci 2023;54:167-77. [Crossref] [PubMed]
- Milazzotto R, Lancellotta V, Posa A, Fionda B, Massaccesi M, Cornacchione P, Spatola C, Kovács G, Morganti AG, Bussu F, Valentini V, Iezzi R, Tagliaferri L. The role of interventional radiotherapy (brachytherapy) in nasopharynx tumors: A systematic review. J Contemp Brachytherapy 2023;15:383-90. [Crossref] [PubMed]
- Blanchard P, Lee A, Marguet S, Leclercq J, Ng WT, Ma J, et al. Chemotherapy and radiotherapy in nasopharyngeal carcinoma: an update of the MAC-NPC meta-analysis. Lancet Oncol 2015;16:645-55. [Crossref] [PubMed]
- Bortfeld T, Jeraj R. The physical basis and future of radiation therapy. Br J Radiol 2011;84:485-98. [Crossref] [PubMed]
- van Herk M. Errors and margins in radiotherapy. Semin Radiat Oncol 2004;14:52-64. [Crossref] [PubMed]
- Rasch C, Steenbakkers R, van Herk M. Target definition in prostate, head, and neck. Semin Radiat Oncol 2005;15:136-45. [Crossref] [PubMed]
- Jena R, Price SJ, Baker C, Jefferies SJ, Pickard JD, Gillard JH, Burnet NG. Diffusion tensor imaging: possible implications for radiotherapy treatment planning of patients with high-grade glioma. Clin Oncol (R Coll Radiol) 2005;17:581-90. [Crossref] [PubMed]
- Zhang H, Xu H, Tian X, Jiang J, Ma J. Image fusion meets deep learning: A survey and perspective. Inf Fusion 2021;76:323-36. [Crossref]
- Jaffray DA, Siewerdsen JH. Cone-beam computed tomography with a flat-panel imager: initial performance characterization. Med Phys 2000;27:1311-23. [Crossref] [PubMed]
- Wang H, Dong L, Lii MF, Lee AL, de Crevoisier R, Mohan R, Cox JD, Kuban DA, Cheung R. Implementation and validation of a three-dimensional deformable registration algorithm for targeted prostate cancer radiotherapy. Int J Radiat Oncol Biol Phys 2005;61:725-35. [Crossref] [PubMed]
- Jaffray DA, Drake DG, Moreau M, Martinez AA, Wong JW. A radiographic and tomographic imaging system integrated into a medical linear accelerator for localization of bone and soft-tissue targets. Int J Radiat Oncol Biol Phys 1999;45:773-89. [Crossref] [PubMed]
- Seo H, Yu L, Ren H, Li X, Shen L, Xing L. Deep Neural Network With Consistency Regularization of Multi-Output Channels for Improved Tumor Detection and Delineation. IEEE Trans Med Imaging 2021;40:3369-78. [Crossref] [PubMed]
- Rivaz H, Collins DL. Deformable registration of preoperative MR, pre-resection ultrasound, and post-resection ultrasound images of neurosurgery. Int J Comput Assist Radiol Surg 2015;10:1017-28. [Crossref] [PubMed]
- Li Y, Zhao J, Lv Z, Li J. Medical image fusion method by deep learning. International Journal of Cognitive Computing in Engineering 2021;2:21-9. [Crossref]
- Liu Y, Chen X, Wang Z, Wang ZJ, Ward RK, Wang X. Deep learning for pixel-level image fusion: Recent advances and future prospects. Inf Fusion 2018;42:158-73. [Crossref]
- Haber E, Modersitzki J. Intensity gradient based registration and fusion of multi-modal images. Med Image Comput Comput Assist Interv 2006;9:726-33.
- Rühaak J, König L, Hallmann M, Papenberg N, Heldmann S, Schumacher H, Fischer B. A fully parallel algorithm for multimodal image registration using normalized gradient fields. 2013 IEEE 10th International Symposium on Biomedical Imaging, San Francisco, CA, USA, 2013:572-5.
- König L, Rühaak J. A fast and accurate parallel algorithm for non-linear image registration using normalized gradient fields. 2014 IEEE 11th International Symposium on Biomedical Imaging (ISBI), Beijing, China, 2014:580-3.
- Goodfellow I, Pouget-Abadie J, Mirza M, Xu B, Warde-Farley D, Ozair S, Courville A, Bengio Y. Proceedings of the 27th International Conference on Neural Information Processing Systems 2014;27:2672-80.
- Petrović V, Xydeas C. Objective evaluation of signal-level image fusion performance. Optical Engineering 2005;44:087003. [Crossref]
- Cheung AL, Zhang L, Liu C, Li T, Cheung AH, Leung C, Leung AK, Lam SK, Lee VH, Cai J. Evaluation of Multisource Adaptive MRI Fusion for Gross Tumor Volume Delineation of Hepatocellular Carcinoma. Front Oncol 2022;12:816678. [Crossref] [PubMed]
- Li W, Xiao H, Li T, Ren G, Lam S, Teng X, Liu C, Zhang J, Kar-Ho Lee F, Au KH, Ho-Fun Lee V, Chang ATY, Cai J. Virtual Contrast-Enhanced Magnetic Resonance Images Synthesis for Patients With Nasopharyngeal Carcinoma Using Multimodality-Guided Synergistic Neural Network. Int J Radiat Oncol Biol Phys 2022;112:1033-44. [Crossref] [PubMed]
- Li W, Lam S, Wang Y, Liu C, Li T, Kleesiek J, Cheung AL, Sun Y, Lee FK, Au KH, Lee VH, Cai J. Model Generalizability Investigation for GFCE-MRI Synthesis in NPC Radiotherapy Using Multi-Institutional Patient-Based Data Normalization. IEEE J Biomed Health Inform 2024;28:100-9. [Crossref] [PubMed]