Impact of nitroglycerin on machine-learning fractional flow reserve in coronary computed tomography (CT)-angiography
Introduction
Computed tomography (CT) derived fractional flow reserve (FFR, CT-FFR) is a non-invasive technique utilized for evaluating hemodynamic alterations in coronary artery disease (CAD) (1,2). Previous clinical trials have confirmed the efficacy of CT-FFR in identifying ischemia (3), and recent guidelines recommend its use in patients with acute chest pain who have coronary artery stenosis of 40% to 90% based on coronary CT-angiography (CCTA) (4). One of the most critical steps in calculating CT-FFR is to construct an accurate coronary artery anatomy model based on high-quality CCTA images, especially to accurately identify luminal boundaries and stenoses (5). Previous evidence shows that sublingual nitroglycerin improves the image quality and stenosis assessment of CCTA (6). And routine nitroglycerin administration before CCTA scan has been recommended in the 2016 guidelines (7).
Theoretically, nitroglycerin-induced vasodilation may affect CT-FFR values and diagnostic performance (8). Previous studies have reported that CT-FFR values increased in both post-lesion and distal vessel after nitroglycerin administration (9), and CT-FFR diagnostic specificity increased (10). However, it has not been systematically proven. Further clarification regarding nitroglycerin’s impact on CT-FFR is essential for its future clinical translation and widespread use. Therefore, we designed a single-center retrospective study to investigate the effect of preprocedural nitroglycerin administration on the calculation and diagnostic performance of machine learning CT-FFR, with invasive FFR as the reference standard. We present this article in accordance with the STARD reporting checklist (available at https://qims.amegroups.com/article/view/10.21037/qims-23-1212/rc).
Methods
Study design and participants
From January 2019 to December 2019, we enrolled 107 consecutive patients with suspected CAD from Fuwai Hospital, who underwent CCTA with nitroglycerin administration priorly. We retrospectively matched 107 patients undergoing CCTA without nitroglycerin administration from the electronic medical database between January 2016 and December 2016 from Fuwai Hospital. Inclusion and exclusion criteria were the same for both groups. Inclusion criteria were (I) aged ≥18 years; and (II) at least one coronary artery lesion with 50−90% diameter stenosis according to CCTA; and (III) perform invasive coronary angiography (ICA) and FFR within one month. Exclusion criteria were as follows: (I) previous coronary revascularization; (II) previous myocardial infarction; (III) implanted cardiac devices; (IV) complex congenital heart disease; and (V) cardiomyopathies and valvular heart disease. Matching accounted for age, and body mass index (match tolerance =1.05). A total of 214 patients with 237 target lesion vessels, which contained coronary artery lesions with 50% to 90% diameter stenosis, were finally included in the study.
The study was conducted in accordance with the Declaration of Helsinki (as revised in 2013). The study was approved by the Ethics Committee of Fuwai Hospital, Chinese Academy of Medical Sciences & Peking Union Medical College/National Center for Cardiovascular Diseases (No. 2018-0074). 107 consecutive participants provided written informed consent, and for the retrospectively matched participants, written informed consent was waived.
CCTA acquisition
CCTA examination was performed by second- or third-generation dual-source CT scanners (Somatom Definition Flash/Force, Siemens Healthcare, Forchheim, Germany), and the images were collected according to the cardiovascular CT protocol (7). Nitroglycerin 0.5 mg (11) (0.5 mg per press; Nitroglycerin Aerosol, Bencao Pharmacy, China) sublingually has been administered 5 minutes before the scan since January 2017. Before that, nitroglycerin was not routinely used for patient in our clinical center. Beta-blockers were administered if a patient had a heart rate ≥75 beats/min. Electrocardiogram-triggered prospective step-and-shoot acquisition was performed at 35–75% of R-R interval. The scan parameters were as follows: detector collimation, 64×2×0.6/96×2×0.6 mm; gantry rotation time, 280/250 ms; tube voltage, 100 kV (<60 kg) or 120 kV (>60 kg); tube current, automatic tube current modulation. All image data sets were reconstructed with a slice thickness of 0.75 mm and an increment of 0.5 mm. Reconstructions were performed according to dual-source CT scanner specifications. Iterative reconstruction (SAFIRE® with a strength level of 3) and medium smooth kernel (I26f) were used for Definition Flash data sets. Iterative reconstruction (Admire® with a strength level of 3) and medium soft convolution kernel (Bv40) were used for Force data sets. A triple phase contrast protocol was used as follows: the first injection of 60–65 mL iodinated contrast agent (Ultravist 370 mgI/mL, Bayer Schering Pharma; Germany) with flow rate 4–6 mL/s, followed by 30 mL contrast agent/saline mixture (mixing ratio 3:7) (4–6 mL/s), followed by injection of 30 mL saline (4 mL/s).
CCTA image assessments and analysis
All data were sent to a dedicated workstation (Syngo.Via, Frontier, Siemens, Germany). Image quality was assessed by two independent observers with five-year experience in a double-blind manner.
Coronary segments were evaluated using an 18-segment coronary model (12). For semiquantitative image quality assessment, we used a four-scale: 4 = excellent, no visible artifacts; 3 = good, artifacts exist in less than 20% of coronary segments; 2 = acceptable, artifacts exist in 20–40% of segments; and 1 = poor, artifacts exist in more than 40% of coronary segments. When two observers disagreed, they decided through mutual consultation. In order to obtain quantitative image quality assessment, two circular regions of interest with ≥1.5 cm2 were placed within the aortic root and subcutaneous adipose tissue of the chest to calculate the signal-to-noise ratio (SNR) and contrast-to-noise ratio (CNR). Image noise was measured using the standard deviation of pixel values. Averaged values measured by two observers were used to minimize bias. The SNR and CNR were calculated using the following formulas: SNR = mean aortic root attenuation value/image noise, CNR = (mean aortic root attenuation value – mean subcutaneous adipose tissue attenuation value)/image noise.
The diameter of coronary artery was measured using the centerline-derived multiplanar reconstruction images. The luminal diameters of the left main artery (LM), left anterior descending coronary artery (LAD), left circumflex coronary artery (LCX), and right coronary artery (RCA) were measured within 1 cm from the ostia while avoiding plaques. The average measurement values of the two observers were also obtained to minimize bias. Coronary artery calcification (CAC) scores were assessed by one experienced radiologist with 3 years of clinical experience using the Agatston method in non-enhanced scanning (13).
CT-FFR analysis
CT-FFR was performed on dedicated software cFFR 3.2.2 (Syngo.Via, Frontier, Siemens, Germany) (not for clinical use) by a radiologist with five-year experience who was blinded to FFR values. CT-FFR values were calculated based on CCTA images as follows: (I) three-dimensional anatomical model construction of coronary trees; (II) centerline definition; (III) boundary condition; (IV) stenosis definition; and (V) CT-FFR calculation (14). The CT-FFR value of the target lesion vessel was obtained at 1–2 cm distal to the stenosis. CT-FFR ≤0.80 was considered lesion-specific ischemia. The number of evaluable coronary artery arteries including branches extracted for anatomical model construction during the CT-FFR calculation procedure was recorded.
ICA and FFR measurements
ICA was performed and FFR was determined according to standard practice (15) by senior cardiovascular physicians. Target coronary stenosis severity was categorized into 0 (0%), 1 (1–24%), 2 (25–49%), 3 (50–69%), or 4 (70–99%).
FFR was performed on target lesion vessels deemed clinically indicated for evaluation except the lesions with diameter stenosis >90%. A pressure-sensor-tipped guidewire (St Jude Medical, Minneapolis, Minn, USA) was advanced 1–2 cm distal to the stenosis. Intravenous adenosine (140–180 µg/kg/min) was administered to obtain maximal coronary hyperemia. Then slowly retract the guide wire and record the FFR value during the whole process. FFR ≤0.80 was considered hemodynamically significant. And the difference between CT-FFR and FFR was calculated as follows: ∆FFR=CT-FFR value – FFR value.
Statistical analysis
MedCalc version 18.2 (MedCalc Software) and SPSS version 22.0 (IBM SPSS Statistics, USA) were used for statistical analysis. Quantitative variables were expressed as mean ± standard deviation (SD) and compared using one-way analysis of variance (ANOVA) test if normally distributed (tested by Kolmogorov-Smirnov method), otherwise the median and interquartile range were used and compared by Kruskal-Wallis H test. Categorical variables were represented by numbers and percentages. The χ2 test or Fisher exact test, as appropriate, were used for comparison of categorical variables and rates. Spearman correlation and Bland-Altman analysis were conducted for the correlation and consistency of CT-FFR and FFR. The correlation coefficient values were interpreted as follows: 0.00 to 0.09 considered negligible, 0.10 to 0.39 considered weak, 0.40 to 0.69 considered moderate, 0.70 to 0.89 considered strong, and 0.90 to 1.00 considered very strong (16). The accuracy and performance characteristics [sensitivity, specificity, positive predictive value (PPV), negative predictive value (NPV), and area under the curve (AUC)] of CT-FFR in identifying ischemia on per-vessel and per-patient levels were assessed in nitroglycerin and non-nitroglycerin groups, with FFR as the reference standard. Receiver operating characteristics curves of CT-FFR in the two groups were performed and compared by DeLong method (17). Statistical significance was assumed at a P<0.05 (two-tailed).
Results
Baseline characteristic
Initially, 197 consecutive patients with clinically suspected CAD in 2019 were screened. Seventy-two patients with coronary stenosis <50% (n=64) or >90% (n=8) based on CCTA were excluded, not suitable for FFR. Eighteen patients were further excluded because of failure of FFR operation (n=18). Meanwhile, 224 patients undergoing CCTA with 50–90% stenosis from January to December 2016 were retrospectively collected from electronic database. Forty-six patients were excluded due to the history of previous coronary intervention or coronary bypass surgery (n=12), previous myocardial infarction (n=10), implanted cardiac devices (n=5), cardiomyopathies and valvular heart disease (n=11), or missed FFR measurements (n=8).
After patient matching, a total of 214 patients with 237 target lesion vessels were enrolled in this study, including 120 vessels in nitroglycerin group and 117 vessels in non-nitroglycerin groups (Flow chart displays in Figure 1). Of these patients, 155 (72.4%) were male and 59 (27.6%) were female, with an average age of (56.1±8.9) years. There was no statistically significant difference in the age, sex ratio, high-risk factors of CAD between nitroglycerin and non-nitroglycerin groups. Additionally, no significant differences were found in dual-source CT specifications and their heart rates during CCTA scans between two groups (Table 1).
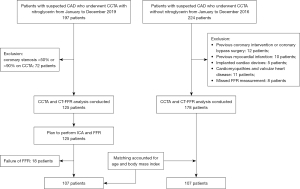
Table 1
Parameters | Full | Nitroglycerin | Non-nitroglycerin | P |
---|---|---|---|---|
No. of patients | 214 | 107 | 107 | |
Age (years) | 56.1±8.9 | 56.1±9.5 | 56.0±8.4 | 0.91 |
Male | 155 (72.4) | 83 (77.6) | 72 (67.3) | 0.18 |
Body mass index (kg/m2) | 25.7±3.2 | 26.0±3.2 | 25.4±3.2 | 0.08 |
Hypertension | 125 (58.4) | 65 (60.7) | 60 (56.1) | 0.57 |
Hyperlipidemia | 184 (86.0) | 92 (86.0) | 92 (86.0) | >0.99 |
Diabetes | 68 (31.8) | 39 (36.4) | 29 (27.1) | 0.18 |
Smoker | 110 (51.4) | 53 (49.5) | 57 (53.3) | 0.68 |
Heart rate (beats/min) | 66.7±11.4 | 67.8±11.6 | 65.8±11.2 | 0.12 |
CACs | 48.3 (0, 188.5) | 69.0 (10.5, 277.7) | 38.0 (0, 138.0) | 0.24 |
DSCT | 0.48 | |||
Somatom definition flash | 81 (37.9) | 38 (35.5) | 43 (40.2) | |
Somatom force | 133 (62.1) | 69 (64.5) | 64 (59.8) | |
No. of vessels | 237 | 120 | 117 | |
Target lesion vessel | 0.20 | |||
Left anterior descending artery | 173 (73.0) | 87 (72.5) | 86 (73.5) | |
Left circumflex artery | 30 (12.7) | 19 (15.8) | 11 (9.4) | |
Right coronary artery | 34 (14.3) | 14 (11.7) | 20 (17.1) | |
Stenosis grade by ICA | 0.71 | |||
0 (0%) | 4 (1.7) | 1 (0.8) | 3 (2.6) | |
1 (1–24%) | 7 (2.9) | 3 (2.5) | 4 (3.4) | |
2 (25–49%) | 0 (0) | 0 (0) | 0 (0) | |
3 (50–69%) | 59 (24.9) | 31 (25.9) | 28 (23.9) | |
4 (70–99%) | 167 (70.5) | 85 (70.8) | 82 (70.1) | |
FFR | 0.26 | |||
<0.75 | 45 (19.0) | 24 (20.0) | 21 (17.9) | |
0.75–0.8 | 45 (19.0) | 20 (16.7) | 25 (21.4) | |
>0.8 | 147 (62.0) | 76 (63.3) | 71 (60.7) |
The continuous variables are expressed as mean ± standard deviation or median (interquartile range), and the categorical variables are expressed as number (percentage). CACs, coronary artery calcium score; DSCT, dual source computed tomography; ICA, invasive coronary angiography; FFR, fractional flow reserve.
Most (95.4%, 226/237) of the target lesion vessels had stenosis degree ≥50%, and particularly (38.0%, 90/237) of them was flow-limiting (FFR ≤0.8). The Baseline characteristics of the patients and target lesion vessels are listed in Table 1. Figure 2 list two patients in nitroglycerin and non-nitroglycerin groups.
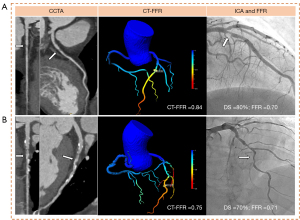
The correlation and difference of CT-FFR and FFR in nitroglycerin and non-nitroglycerin groups
The Spearman correlation between CT-FFR and FFR was moderate (r=0.606, P<0.001) in all target vessels. Subgroup analysis showed that the nitroglycerin group had a higher correlation coefficient (r=0.664, P<0.001) compared to the non-nitroglycerin group (r=0.548, P<0.001) (Figure 3).
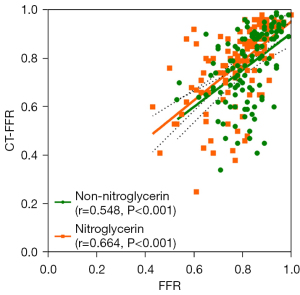
Bland-Altman plots was used for the agreement of CT-FFR and FFR values (Figure 4). The biases of CT-FFR and FFR were as follows: nitroglycerin group [mean difference =−0.01, 95% limits of agreement (LOA): −0.25 to 0.24], and non-nitroglycerin group (mean difference =−0.05, 95% LoA: −0.32 to 0.21). And accordingly, significantly larger bias of CT-FFR was observed in non-nitroglycerin group (−0.05±0.13) than nitroglycerin group (−0.01±0.12) (P=0.007). It was also noticed the underestimation of CT-FFR compared to FFR in the two groups.
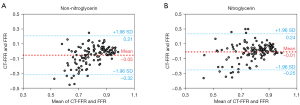
The effect of nitroglycerin on CT-FFR diagnostic performance
In total, the diagnostic sensitivity, specificity, PPV, NPV, accuracy, and AUC of CT-FFR identifying lesion-specific ischemia were 78.6%, 70.9%, 67.5%, 81.2%, 74.3%, 0.78 on a per-vessel level and 80.8%, 71.3%, 70.8%, 81.2%, 75.7%, 0.77 on a per-patient level, respectively.
The vessel-based and patient-based diagnostic performance of CT-FFR identifying ischemia in nitroglycerin and non-nitroglycerin groups is displayed in Table 2 and Figure 5. On a per-vessel level, the accuracy of CT-FFR in nitroglycerin group [80.0% (71.7–86.7%), 96/120] was significantly higher than non-nitroglycerin group [68.4% (59.1–76.7%), 80/117] (P=0.041). While no major difference was found in the sensitivity [80.7% (68.1–90.0%) vs. 76.1% (61.2–87.4%), P=0.37], specificity [79.4% (67.3–88.5%) vs. 63.3% (51.1–74.5%), P=0.38], PPV [78.0% (68.2–85.4%) vs. 57.4% (48.8–65.6%), P=0.68], NPV [82.0% (72.5–88.7%) vs. 80.4% (70.3–87.6%), P=0.22], and AUC [0.82 (0.74–0.89) vs. 0.74 (0.65–0.83), P=0.13] of CT-FFR between the two groups. On a per-patient basis, nitroglycerin administration before CCTA significantly elevated the accuracy [83.2% (74.7–89.7%) vs. 68.2% (58.5–76.9%), P=0.01], specificity [82.7% (69.7–91.8%) vs. 61.9% (48.8–73.9%), P=0.01], PPV [83.6% (73.6–90.4%) vs. 58.6% (50.0–66.9%), P=0.004], and AUC [0.83 (0.75–0.89) vs. 0.71 (0.61–0.79), P=0.03] of CT-FFR. And the two groups possessed similar sensitivity [83.6% (71.2–92.2%) vs. 77.3% (62.2–88.5%), P=0.60] and NPV [82.7% (72.2–89.8%) vs. 79.6% (68.6–87.4%), P=0.85].
Table 2
Analysis basis | Results | Diagnostic parameters | ||||||||||||||||||||
---|---|---|---|---|---|---|---|---|---|---|---|---|---|---|---|---|---|---|---|---|---|---|
Sensitivity | Specificity | PPV | NPV | Accuracy | AUC | |||||||||||||||||
TP | TN | FP | FN | % (95% CI) | P | % (95% CI) | P | % (95% CI) | P | % (95% CI) | P | % (95% CI) | P | % (95% CI) | P | |||||||
Per-vessel level | 0.37 | 0.38 | 0.68 | 0.22 | 0.041* | 0.13 | ||||||||||||||||
All | 81 | 95 | 39 | 22 | 78.6 (69.5–86.1) | 70.9 (62.4–78.4) | 67.5 (61.0–73.4) | 81.2 (74.6–86.4) | 74.3 (68.2–79.7) | 0.78 (0.73–0.84) | ||||||||||||
Non-nitroglycerin | 35 | 45 | 26 | 11 | 76.1 (61.2–87.4) | 63.3 (51.1–74.5) | 57.4 (48.8–65.6) | 80.4 (70.3–87.6) | 68.4 (59.1–76.7) | 0.74 (0.65–0.83) | ||||||||||||
Nitroglycerin | 46 | 50 | 13 | 11 | 80.7 (68.1–90.0) | 79.4 (67.3–88.5) | 78.0 (68.2–85.4) | 82.0 (72.5–88.7) | 80.0 (71.7–86.7) | 0.82 (0.74–0.89) | ||||||||||||
Per-patient level | 0.60 | 0.01* | 0.004* | 0.85 | 0.01* | 0.03* | ||||||||||||||||
All | 80 | 82 | 33 | 19 | 80.8 (71.7–88.0) | 71.3 (62.1–79.4) | 70.8 (64.1–76.7) | 81.2 (73.9–86.8) | 75.7 (69.4–81.3) | 0.77 (0.70–0.82) | ||||||||||||
Non-nitroglycerin | 34 | 39 | 24 | 10 | 77.3 (62.2–88.5) | 61.9 (48.8–73.9) | 58.6 (50.0–66.9) | 79.6 (68.6–87.4) | 68.2 (58.5–76.9) | 0.71 (0.61–0.79) | ||||||||||||
Nitroglycerin | 46 | 43 | 9 | 9 | 83.6 (71.2–92.2) | 82.7 (69.7–91.8) | 83.6 (73.6–90.4) | 82.7 (72.2–89.8) | 83.2 (74.7–89.7) | 0.83 (0.75–0.89) |
Data are presented as percentage (95% CI) or value (95% CI). *, statistical significance. CT-FFR, computed tomography derived fractional flow reserve; TP, true positive; TN, true negative; FP, false positive; FN, false negative; PPV, positive predictive value; NPV, negative predictive value; AUC, area under the curve; CI, confidence interval.
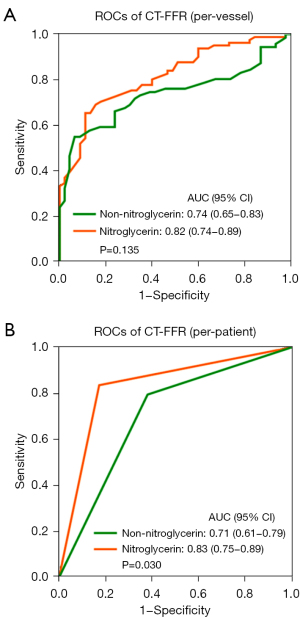
Assessments of image quality and coronary evaluability in nitroglycerin and non-nitroglycerin groups
The evaluation of image quality and coronary artery were conducted and compared in nitroglycerin and non-nitroglycerin groups (Table 3). The semiquantitative image quality assessment was mainly focused on the artifacts causing by various factors. Semiquantitative image quality comparison showed a higher score in the nitroglycerin group than non-nitroglycerin group (3.7±0.5 vs. 3.5±0.7, P=0.02). However, there was no significant difference found in quantitative assessment parameters (SNR: 27.6±22.0 vs. 28.9±30.5, P=0.72; CNR: 34.0±22.4 vs. 35.4±37.1, P=0.73) of CCTA image quality between the two groups.
Table 3
Parameters | Full (n=214) | Nitroglycerin (n=107) | Non-nitroglycerin (n=107) | P |
---|---|---|---|---|
Semiquantitative image quality | 3.6±0.7 | 3.7±0.5 | 3.5±0.7 | 0.02* |
Quantitative image quality | ||||
Attenuation of aortic root (HU) | 406.5±76.5 | 400.0±67.5 | 413.9±84.6 | 0.59 |
SNR | 28.2±26.4 | 27.6±22.0 | 28.9±30.5 | 0.72 |
CNR | 34.7±30.4 | 34.0±22.4 | 35.4±37.1 | 0.73 |
SD | 16.6±4.2 | 16.3±3.3 | 16.8±4.9 | 0.33 |
Coronary artery diameter (mm) | ||||
Left main artery | 4.1±0.9 | 4.3±0.8 | 3.8±0.8 | <0.001* |
Left anterior descending artery | 3.0±0.6 | 3.1±0.6 | 2.9±0.6 | 0.001* |
Left circumflex artery | 2.8±0.7 | 2.9±0.7 | 2.7±0.6 | 0.01* |
Right coronary artery | 3.5±0.7 | 3.7±0.7 | 3.4±0.7 | 0.001* |
Number of evaluable coronary arteries | 10.0 (8.0, 12.0) | 11.0 (9.0, 14.0) | 8.0 (7.0, 10.0) | <0.001* |
Data are presented as mean ± standard deviation or median (interquartile range). *, statistical significance. HU, Hounsfield unit; SNR, signal-to-noise ratio; CNR, contrast-to-noise ratio; SD, standard deviation.
For coronary artery evaluation, it included the assessment about the vessel diameter and the number of evaluable vessels including branches extracted for anatomical model construction during the CT-FFR calculation procedure. The vessel diameters of four main coronary arteries in nitroglycerin group were higher than the non-nitroglycerin group (LMs: 4.3±0.8 vs. 3.8±0.8 mm, P<0.001; LADs: 3.1±0.6 vs. 2.9±0.6 mm, P=0.001; LCXs: 2.9±0.7 vs. 2.7±0.6 mm, P=0.012; RCAs: 3.7±0.7 vs. 3.4±0.7 mm, P=0.001). Meanwhile, the number of evaluable coronary arteries was also significantly larger in nitroglycerin group than the non-nitroglycerin group [median:11.0 (interquartile range: 9.0,14.0) vs. 8.0 (7.0, 10.0), P<0.001].
In addition, there was a weak correlation between CT-FFR and vessel diameter in LMs (r=0.203, P=0.003), LADs (r=0.274, P<0.001), LCXs (r=0.210, P=0.002), and RCAs (r=0.187, P=0.006) (as detailed in Appendix 1, Figure S1). However, no significant association was found between the semiquantitative image quality and CT-FFR (P=0.19) (as detailed in Appendix 1, Figure S2).
Discussion
In this comparative, retrospective study, we investigated the impact of nitroglycerin administration on machine-learning based CT-FFR for identifying ischemia, with invasive FFR as the reference standard. The major findings of this study can be summarized as follows: (I) sublingual nitroglycerin administration before the CCTA scan improved diagnostic performance and reduced bias of CT-FFR. (II) A higher semiquantitative image score was obtained with nitroglycerin administration. (III) A larger vessel diameter and a higher number of evaluable coronary arteries were observed in the nitroglycerin group.
Currently, there have been limited studies investigating the effect of nitroglycerin on CT-FFR (9,10,18-20). Gao et al. (9) found the use of sublingual nitroglycerin was associated with increased post-stenosis and vessel distal CT-FFR values. Interestingly, Holmes et al. (19) found that different dosages of nitroglycerin (0.4 vs. 0.8 mg) did not alter CT-FFR values. However, none of the above studies validated the diagnostic accuracy of CT-FFR compared with the gold standard invasive FFR. Leipsic et al. (10) got a preliminary result that the diagnostic specificity of CT-FFR increased when using nitroglycerin, while there remained some interference effects of confounding factors such as patients’ baseline characteristics on the results. In this study, we included two clusters of patients with similar baseline characteristics, so that the interference of potential baseline confounding factors could be excluded when exploring the effect of nitroglycerin. In addition, due to CT-FFR diagnostic performance varies for different degrees of stenosis (21), especially inadequate diagnostic accuracy within the “gray zone” range of 0.7–0.8 (3,22). Consequently, it is crucial to ensure that there are no disparities in the vessel stenosis grade and hemodynamic condition as determined by FFR between the two groups, thereby avoiding potential interference from inherent lesion characteristics. In addition to excluding potential bias in patient selection, differences in CT specifications and scan parameters may also affect CT-FFR calculations (23). In this study, there were no significant differences between the two groups in dual-source CT specifications and scan parameters.
Our study manifested that sublingual nitroglycerin administration was related to smaller bias of CT-FFR values than non-sublingual nitroglycerin group, in relation to the gold standard FFR. Higher diagnostic performance of CT-FFR was observed in nitroglycerin group on both per-vessel and per-patient levels, especially the improved specificity. Diagnosis of ischemia by CT-FFR is a dichotomous judgment with a single cut-off value of ≤0.8 (3,24). When the FFR value is around 0.8, a small measurement deviation of CT-FFR may lead to a change in diagnosis. It is also one of the reasons for the poor diagnostic accuracy of CT-FFR in “gray zone” (22,25). That is theoretically inevitable. But from a technical point of view, based on our study, the nitroglycerin administration may improve the diagnostic performance of CT-FFR in “gray zone” lesions by improving the accurate measurement. Further systematic study is necessary to confirm it. In addition, all the participants in this study came from real clinical practice, and CT-FFR showed 74.5% (68.8–79.7%) diagnostic accuracy. To some extent, it reflects the performance of CT-FFR in real world, which is consistent with a recent meta-analysis (3).
Precise CT-FFR measurement relies on accurate coronary artery model derived from high-quality CCTA image. There are many factors influencing CCTA image quality, including preprocedural preparation and patients’ condition, which may influence the CT-FFR calculation (10,20,26). It’s essential to understand the impact of nitroglycerin administration on CCTA image quality and coronary artery evaluability before interpreting the result above. Previous studies have reached different conclusions on the effects of nitroglycerin on subject and objective CCTA image quality (6,27). This study showed that nitroglycerin administration resulted in higher semiquantitative score (subjective quality), which meant less artifacts and more evaluable coronary segments. Besides, the lumen attenuation is also the basis of segmentation and quantization of CT technology (28). Xu et al. (20) indicated that intracoronary enhancement degree of 300–400 HU could be beneficial to accurate CT-FFR analysis. While no significant correlation was observed between the objective quantitative parameters of image quality and nitroglycerin in this study.
Additionally, improved coronary evaluability by nitroglycerin was also revealed in this study. Sublingual nitroglycerin administration resulted in significant coronary artery dilation and increased numbers of evaluable coronary arteries, consistent with previous studies (29-31). Klass et al. (31), and Okada et al. (29) even found a larger vessel diameter increase in the distal and peripheral segments compared to the proximal ones. The nitroglycerin-induced vasodilation and improved coronary evaluability including distal segments and branches, greatly promote CT-FFR to simulate the maximum hyperemia flow of coronary (10). And CT-FFR measurement is closer to the real FFR value. In summary, nitroglycerin administration improved image quality and coronary evaluability, resulting in more accurate CT-FFR calculations. Sublingual nitroglycerin administration should be suggested in routine CT-FFR practice. Our study adds original evidence to standardize CT-FFR operation.
This study still has some limitations. Firstly, this is a retrospective study. Although there were no differences in baseline characteristics or scan parameters between the two groups, there may be some unknown confounding factors also present. Secondly, the study was conducted in a single clinic center and focused on the machine-learning CT-FFR. It’s still unknown whether the results can be generalized to the real world and FFR with computational fluid hemodynamics. A multicenter prospective randomized study is necessary to verify the results, involving different CT scanner, scanning protocol, CT-FFR algorithms, and hospitals with different medical levels.
Conclusions
In conclusion, this study reveals that the sublingual nitroglycerin administration prior to CCTA has a positive effect on the diagnostic performance of CT-FFR, due to significant vessel dilation and improved evaluability of coronary arteries.
Acknowledgments
The authors wish to thank all participants dedicated to contributing to this study.
Funding: This work was supported by
Footnote
Reporting Checklist: The authors have completed the STARD reporting checklist. Available at https://qims.amegroups.com/article/view/10.21037/qims-23-1212/rc
Conflicts of Interest: All authors have completed the ICMJE uniform disclosure form (available at https://qims.amegroups.com/article/view/10.21037/qims-23-1212/coif). The authors have no conflicts of interest to declare.
Ethical Statement: The authors are accountable for all aspects of the work in ensuring that questions related to the accuracy or integrity of any part of the work are appropriately investigated and resolved. The study was conducted in accordance with the Declaration of Helsinki (as revised in 2013). The study was approved by the Ethics Committee of Fuwai Hospital, Chinese Academy of Medical Sciences & Peking Union Medical College/National Center for Cardiovascular Diseases (No. 2018-0074). 107 consecutive participants provided written informed consent, and for the retrospectively matched participants, written informed consent was waived.
Open Access Statement: This is an Open Access article distributed in accordance with the Creative Commons Attribution-NonCommercial-NoDerivs 4.0 International License (CC BY-NC-ND 4.0), which permits the non-commercial replication and distribution of the article with the strict proviso that no changes or edits are made and the original work is properly cited (including links to both the formal publication through the relevant DOI and the license). See: https://creativecommons.org/licenses/by-nc-nd/4.0/.
References
- Yan H, Zhao N, Geng W, Yu X, Gao Y, Lu B. Identification of ischemia-causing lesions using coronary plaque quantification and changes in fractional flow reserve derived from computed tomography across the lesion. Quant Imaging Med Surg 2023;13:3630-43. [Crossref] [PubMed]
- Dai X, Lu Z, Yu Y, Yu L, Xu H, Zhang J. The use of lesion-specific calcium morphology to guide the appropriate use of dynamic CT myocardial perfusion imaging and CT fractional flow reserve. Quant Imaging Med Surg 2022;12:1257-69. [Crossref] [PubMed]
- Cook CM, Petraco R, Shun-Shin MJ, Ahmad Y, Nijjer S, Al-Lamee R, Kikuta Y, Shiono Y, Mayet J, Francis DP, Sen S, Davies JE. Diagnostic Accuracy of Computed Tomography-Derived Fractional Flow Reserve: A Systematic Review. JAMA Cardiol 2017;2:803-10. [Crossref] [PubMed]
- Gulati M, Levy PD, Mukherjee D, Amsterdam E, Bhatt DL, et al. 2021 AHA/ACC/ASE/CHEST/SAEM/SCCT/SCMR Guideline for the Evaluation and Diagnosis of Chest Pain: Executive Summary: A Report of the American College of Cardiology/American Heart Association Joint Committee on Clinical Practice Guidelines. J Am Coll Cardiol 2021;78:2218-61. [Crossref] [PubMed]
- Taylor CA, Fonte TA, Min JK. Computational fluid dynamics applied to cardiac computed tomography for noninvasive quantification of fractional flow reserve: scientific basis. J Am Coll Cardiol 2013;61:2233-41. [Crossref] [PubMed]
- Takx RA, Suchá D, Park J, Leiner T, Hoffmann U. Sublingual Nitroglycerin Administration in Coronary Computed Tomography Angiography: a Systematic Review. Eur Radiol 2015;25:3536-42. [Crossref] [PubMed]
- Abbara S, Blanke P, Maroules CD, Cheezum M, Choi AD, Han BK, Marwan M, Naoum C, Norgaard BL, Rubinshtein R, Schoenhagen P, Villines T, Leipsic J. SCCT guidelines for the performance and acquisition of coronary computed tomographic angiography: A report of the society of Cardiovascular Computed Tomography Guidelines Committee: Endorsed by the North American Society for Cardiovascular Imaging (NASCI). J Cardiovasc Comput Tomogr 2016;10:435-49. [Crossref] [PubMed]
- Ignarro LJ, Napoli C, Loscalzo J. Nitric oxide donors and cardiovascular agents modulating the bioactivity of nitric oxide: an overview. Circ Res 2002;90:21-8. [Crossref] [PubMed]
- Gao Y, Wang W, Wang H, Zhou Z, Xu P, Jiang M, Yang L, Wang H, Wen H, Sun Z, Leipsic JA, Zhang L, Xu L. Impact of Sublingual Nitroglycerin on the Assessment of Computed Tomography-derived Fractional Flow Reserve: An Intraindividual Comparison Study. J Comput Assist Tomogr 2022;46:23-8. [Crossref] [PubMed]
- Leipsic J, Yang TH, Thompson A, Koo BK, Mancini GB, Taylor C, Budoff MJ, Park HB, Berman DS, Min JK. CT angiography (CTA) and diagnostic performance of noninvasive fractional flow reserve: results from the Determination of Fractional Flow Reserve by Anatomic CTA (DeFACTO) study. AJR Am J Roentgenol 2014;202:989-94. [Crossref] [PubMed]
- Kim C, Ha M, Kim W, Park SJ, Hwang SH, Yong HS, Oh YW, Kang EY, Cha J, Lee SH, Lee KY. Nitrates administered by spray versus tablet: comparison of coronary vasodilation on CT angiography. Eur Radiol 2021;31:515-24. [Crossref] [PubMed]
- Raff GL, Abidov A, Achenbach S, Berman DS, Boxt LM, Budoff MJ, Cheng V, DeFrance T, Hellinger JC, Karlsberg RPSociety of Cardiovascular Computed Tomography. SCCT guidelines for the interpretation and reporting of coronary computed tomographic angiography. J Cardiovasc Comput Tomogr 2009;3:122-36. [Crossref] [PubMed]
- Agatston AS, Janowitz WR, Hildner FJ, Zusmer NR, Viamonte M Jr, Detrano R. Quantification of coronary artery calcium using ultrafast computed tomography. J Am Coll Cardiol 1990;15:827-32. [Crossref] [PubMed]
- Itu L, Rapaka S, Passerini T, Georgescu B, Schwemmer C, Schoebinger M, Flohr T, Sharma P, Comaniciu D. A machine-learning approach for computation of fractional flow reserve from coronary computed tomography. J Appl Physiol (1985) 2016;121:42-52. [Crossref] [PubMed]
- Bashore TM, Balter S, Barac A, Byrne JG, Cavendish JJ, Chambers CE, Hermiller JB Jr, Kinlay S, Landzberg JS, Laskey WK, McKay CR, Miller JM, Moliterno DJ, Moore JW, Oliver-McNeil SM, Popma JJ, Tommaso CLACCF Task Force Members. 2012 American College of Cardiology Foundation/Society for Cardiovascular Angiography and Interventions expert consensus document on cardiac catheterization laboratory standards update: A report of the American College of Cardiology Foundation Task Force on Expert Consensus documents developed in collaboration with the Society of Thoracic Surgeons and Society for Vascular Medicine. J Am Coll Cardiol 2012;59:2221-305. [Crossref] [PubMed]
- Schober P, Boer C, Schwarte LA. Correlation Coefficients: Appropriate Use and Interpretation. Anesth Analg 2018;126:1763-8. [Crossref] [PubMed]
- DeLong ER, DeLong DM, Clarke-Pearson DL. Comparing the areas under two or more correlated receiver operating characteristic curves: a nonparametric approach. Biometrics 1988;44:837-45. [Crossref] [PubMed]
- Andreini D, Belmonte M, Penicka M, Van Hoe L, Mileva N, Paolisso P, Nagumo S, Nørgaard BL, Ko B, Otake H, Koo BK, Jensen JM, Mizukami T, Munhoz D, Updegrove A, Taylor C, Leipsic J, Sonck J, De Bruyne B, Collet C. Impact of coronary CT image quality on the accuracy of the FFR(CT) Planner. Eur Radiol 2024;34:2677-88. [Crossref] [PubMed]
- Holmes KR, Fonte TA, Weir-McCall J, Anastasius M, Blanke P, Payne GW, Ellis J, Murphy DT, Taylor C, Leipsic JA, Sellers SL. Impact of sublingual nitroglycerin dosage on FFR(CT) assessment and coronary luminal volume-to-myocardial mass ratio. Eur Radiol 2019;29:6829-36. [Crossref] [PubMed]
- Xu PP, Li JH, Zhou F, Jiang MD, Zhou CS, Lu MJ, et al. The influence of image quality on diagnostic performance of a machine learning-based fractional flow reserve derived from coronary CT angiography. Eur Radiol 2020;30:2525-34. [Crossref] [PubMed]
- Koo HJ, Kang JW, Kang SJ, Kweon J, Lee JG, Ahn JM, Park DW, Lee SW, Lee CW, Park SW, Park SJ, Kim YH, Yang DH. Impact of coronary calcium score and lesion characteristics on the diagnostic performance of machine-learning-based computed tomography-derived fractional flow reserve. Eur Heart J Cardiovasc Imaging 2021;22:998-1006. [Crossref] [PubMed]
- Matsumura-Nakano Y, Kawaji T, Shiomi H, Kawai-Miyake K, Kataoka M, Koizumi K, Matsuda A, Kitano K, Yoshida M, Watanabe H, Tazaki J, Kato T, Saito N, Shizuta S, Ono K, Togashi K, Morimoto T, Kimura T. Optimal Cutoff Value of Fractional Flow Reserve Derived From Coronary Computed Tomography Angiography for Predicting Hemodynamically Significant Coronary Artery Disease. Circ Cardiovasc Imaging 2019;12:e008905. [Crossref] [PubMed]
- Ammon F, Moshage M, Smolka S, Goeller M, Bittner DO, Achenbach S, Marwan M. Influence of reconstruction kernels on the accuracy of CT-derived fractional flow reserve. Eur Radiol 2022;32:2604-10. [Crossref] [PubMed]
- Min JK, Leipsic J, Pencina MJ, Berman DS, Koo BK, van Mieghem C, Erglis A, Lin FY, Dunning AM, Apruzzese P, Budoff MJ, Cole JH, Jaffer FA, Leon MB, Malpeso J, Mancini GB, Park SJ, Schwartz RS, Shaw LJ, Mauri L. Diagnostic accuracy of fractional flow reserve from anatomic CT angiography. JAMA 2012;308:1237-45. [Crossref] [PubMed]
- Gaur S, Bezerra HG, Lassen JF, Christiansen EH, Tanaka K, Jensen JM, Oldroyd KG, Leipsic J, Achenbach S, Kaltoft AK, Bøtker HE, Nørgaard BL. Fractional flow reserve derived from coronary CT angiography: variation of repeated analyses. J Cardiovasc Comput Tomogr 2014;8:307-14. [Crossref] [PubMed]
- Coenen A, Lubbers MM, Kurata A, Kono A, Dedic A, Chelu RG, Dijkshoorn ML, van Geuns RJ, Schoebinger M, Itu L, Sharma P, Nieman K. Coronary CT angiography derived fractional flow reserve: Methodology and evaluation of a point of care algorithm. J Cardiovasc Comput Tomogr 2016;10:105-13. [Crossref] [PubMed]
- Patrick DJ, Hakim M, Ahmed F, Hakim DE, Labbe R, Rubimbura V, Hacquin G, Gaux JC, Auguste M, Mansour H, Pernes JM. Effect of sublingual nitro-glycerine premedication on image analysis of using 256 multidetector computed tomography coronary angiography. OMICS J Radiol 2014;3:173. [Crossref]
- De Geer J, Coenen A, Kim YH, Kruk M, Tesche C, Schoepf UJ, Kepka C, Yang DH, Nieman K, Persson A. Effect of Tube Voltage on Diagnostic Performance of Fractional Flow Reserve Derived From Coronary CT Angiography With Machine Learning: Results From the MACHINE Registry. AJR Am J Roentgenol 2019;213:325-31. [Crossref] [PubMed]
- Okada M, Nakashima Y, Nomura T, Miura T, Nao T, Yoshimura M, Sano Y, Matsunaga N. Coronary vasodilation by the use of sublingual nitroglycerin using 64-slice dual-source coronary computed tomography angiography. J Cardiol 2015;65:230-6. [Crossref] [PubMed]
- Lee CM, Wang HJ, Kung CH, Lin YH, Leung TK, Huang CY, Shih CM, Hsiao WT, Chen YY. Evaluation of nitroglycerin premedication on the arterial luminal diameter and branches of coronary arteries on 64-multidetector computed tomography angiography. J Exp Clin Med 2011;3:85-8. [Crossref]
- Klass O, Mutlu S, Hohl K, Feuerlein S, Jeltsch M, Brambs HJ, Hoffmann MH. Multidetector computed tomography coronary angiography: sublingual nitroglycerine improves image quality significantly because of peripheral coronary vasodilatation. J Comput Assist Tomogr 2009;33:199-203. [Crossref] [PubMed]