Agreement of ejection fraction measured by coronary computed tomography (CT) and cardiac ultrasound in evaluating patients with chronic heart failure: an observational comparative study
Introduction
The human heart, nestled between the lungs above the diaphragm, pumps vital nutrients and oxygen to the body through four chambers: the left and right atria and ventricles, which connect to major arteries and veins (1). With the improvement of people’s living standards, the incidence of heart diseases such as coronary heart disease, cardiomyopathy and arrhythmia is increasing yearly (2). Therefore, evaluating the cardiac function, especially left ventricular function, is becoming increasingly important. Function indicators of the left ventricle (LV) include cardiac output, cardiac index, and ejection fraction (EF) (3). EF refers to the percentage of the stroke output in the left ventricular end-diastolic volume. To ascertain a dependable measurement of the left ventricular function, it is imperative to precisely ascertain the volume of the LV across various phases, with particular emphasis on the end-diastolic and end-systolic periods.
There are several methods available to measure the LV volume, including echocardiography, radionuclide angiography and left ventricular angiography by cardiac catheter, etc. (4) These methods have their advantages and disadvantages, among which echocardiography is the most convenient and widely used. Radionuclide angiography is of limited use because of its contamination, while cardiac catheterization is invasive. Echocardiography methods mainly include M-type and two-dimensional echocardiography (5). M-type echocardiography assumes the LV as a geometry of a certain shape. By measuring the inner diameter of each section, it is substituted into the corresponding formula to calculate the LV volume. However, in practical application, the shape of the LV is changeable. It is difficult to be represented by a single-shape geometry, so this method is simple to operate, but its accuracy is unreliable. The commonly used algorithms of two-dimensional echocardiography include the Simpson method and the area-length method. The most commonly used method in clinical practice is the area-length method, which calculates the left ventricular volume by marking the endocardium and measuring its area and inner diameter, which is greatly affected by the geometric shape and image quality of the LV.
Recent years have seen rapid developments in artificial intelligence (AI) in cardiac medical imaging, making it possible to automate methods for segmenting the LV based on deep learning (6). Current AI-based LV-EF studies are mainly based on echocardiography and digital subtraction angiography (DSA), with relatively few based on computed tomography (CT) (7,8). There are even fewer comparative studies of EF calculation based on AI for echocardiography and CT (9). Furthermore, there is almost no agreement analysis research involving AI software, which has largely hindered the advancement of CT-based automated heart function analysis research. Accurate left ventricle (AccuLV, ArteryFlow Technology, Hangzhou, China) software is a fully automatic left ventricular functional analysis system based on cardiac CT images. In this study, the agreement of the AI-based AccuLV-measured EF for clinical diagnosis was evaluated, and the accuracy and reliability of the clinical use of AccuLV were further assessed. We present this article in accordance with the STARD reporting checklist (available at https://qims.amegroups.com/article/view/10.21037/qims-23-1864/rc).
Methods
Study design
In this retrospective study, we estimated the agreement of the left ventricular ejection fraction derived from coronary CT (EF-CT) according to a gold standard defined by cardiac ultrasound (EF) (10). There were 321 cases who underwent cardiac CT and ultrasound examination within one month for suspected symptoms of heart failure screened and enrolled for further analysis. Cardiac insufficiency was determined if the EF measured by ultrasound was below 50%, and the diagnostic performance of cardiac insufficiency between EF-CT and EF was evaluated. The study was conducted in accordance with the Declaration of Helsinki (as revised in 2013). The study was approved by the Medical Ethics Committee of the Second Affiliated Hospital of Zhejiang University School of Medicine (No. I2021001277). As a retrospective study based on historical patient images, no contact with patients was required, so the requirement of informed consent for this retrospective analysis was waived.
Participants
The patient inclusion criteria were clinical symptoms of heart failure, such as chest tightness and shortness of breath after exertion. The coronary CT examination was completed and the time was within three months from the ultrasound. All CT scans were performed on a CT scanner (SOMATON Force, Siemens, Munich, Germany), the scanning parameters were as follows: slice thickness ≤1 mm, image matrix 512×512. low-dose scanning or standard-dose scanning (combination of tube voltage 70–140 kV and tube current 84–2,300 mA). The patient exclusion criteria were complicated with chronic diseases (such as hypertension, diabetes, etc.) and life expectancy of ≤12 months or the conditions that researchers thought it difficult to follow up (such as due to travel, speech or mental disorders, etc.) or the patient who was participating in an interventional clinical study or with poor CT imaging quality. The time interval for all enrolled patients was from January 2019 to April 2020. A flow diagram of patient inclusion and exclusion is shown in Figure 1.
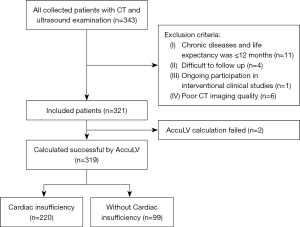
Working principle of AccuLV and EF-CT calculation
AccuLV is an image processing software based on AI for cardiac CT image analysis of patients running on Ubuntu. The software provides a semi-automated analysis process, mainly including LV segmentation and calculation of functional parameters of the LV.
An 8-layer U-Net segmentation network was applied to the LV segmentation module (9,11). Cardiac CT images were input into the U-Net model, including an encoder for semantic condensation and a decoder for image size restoration. The encoder networks consisted of nine encoding blocks, each containing two 3×3 convolutional layers. Each convolutional layer was followed by a batch normalization layer and a rectified linear unit (ReLU) activation layer, and the encoding blocks were followed by a 2×2 max pooling layer used for downsampling. The decoder networks included eight decoding blocks; each decoding block contained two 3×3 convolutional layers, each convolutional layer was followed by a batch normalization layer and a ReLU activation layer, and 2×2 deconvolutional layers preceded each decoding block for upsampling. The feature maps of the corresponding blocks in the encoder and the decoder were skip-connected according to the channel dimension. In each block of the U-Net model networks, a residual structure was incorporated, and in the decoder, an auxiliary path was added to the first seven upsampling operations (12). Based on the 8-layer U-Net segmentation network structure described above, the left ventricular region can be segmented accurately and quickly.
After the deep learning automatic segmentation of the cardiac CT image, the LV segmentation results were superimposed according to the three-dimensional coordinates. While obtaining the three-dimensional model of the LV, the mass and volume of the LV were automatically calculated. Then according to the LV volume at the end of systole and end of diastole, automatic calculation of left ventricular functional parameters such as cardiac output, cardiac index, and EF were completed. EF-CT values were calculated by AccuLV in all cases and analyzed in comparison with EF values from cardiac ultrasound. Figure 2 shows a three-dimensional (3D) rendering of the left ventricular segmentation, left ventricular wall and cavity of the AccuLV.
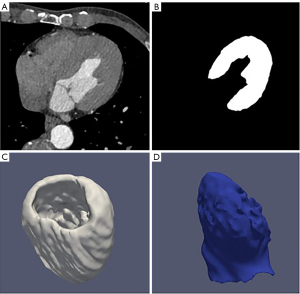
Statistical analysis
The primary endpoint analysis was to assess the diagnostic specificity of EF-CT. Estimation of sample size calculation was based on the non-inferior test for one proportion using PASS 15 software (NCSS, LLC., Utah, USA), the expected proportion was evaluated as 0.4, and the target value for EF-CT was set as 0.3, with a power of 0.9, one-sided alpha of 0.025 (13). With a 20% loss rate, approximately 321 cases would be required.
The diagnostic metrics mainly included the sensitivity, specificity, positive predictive value (PPV) and negative predictive value (NPV) of EF-CT compared to EF. Regression analysis between proportions of patients with an EF value <50% and reduced patient-level EF-CT value was conducted using weighted linear regression with robust estimation. We used correlation analysis and Bland-Altman plot to perform agreement analysis of EF and EF-CT. A P value <0.05 (two-tailed) was considered statistically significant for Kendall’s tau-b trend test. All the above statistical analyses were performed using statsmodels package in Python 3.9.0.
Results
Clinical characteristics
Basic characteristics of the study cohort are shown in Table 1. The mean age was 68.43 years, 223 (69.5%) were men and 98 (30.5%) were women, 64 (19.9%) had diabetes, 169 (52.6%) had hypertension, 102 (31.8%) smoked, 158 (49.2%) had cerebrovascular disease (CVD), 26 (8.1%) had peripheral vascular disease, and 36 (11.2%) had pulmonary heart disease. Of those with a previous medical history, 14 (4.4%) had a stroke, 9 (2.8%) had a myocardial infarction, 14 (4.4%) had pacemaker implantation, and 6 (1.9%) had a heart valve replacement.
Table 1
Baseline character | Outcomes (N=321), N (%) |
---|---|
Age (years) (mean ± SD) | 68.43±38.57 |
Female | 98 (30.5) |
Smoke | 102 (31.8) |
Hypertension | 169 (52.6) |
Diabetes | 64 (19.9) |
CVD | 158 (49.2) |
PVD | 26 (8.1) |
Pulmonary heart disease | 36 (11.2) |
Previous | |
Stroke | 14 (4.4) |
Myocardial infarction | 9 (2.8) |
Pacemaker implantation | 14 (4.4) |
Valve replacement | 6 (1.9) |
SD, standard deviation; CVD, cerebrovascular disease; PVD, peripheral vascular disease.
Median [interquartile range (IQR)] time delay between coronary CTA and cardiac ultrasound was 2 (1 to 7) days. Selected characteristics of treatment of the study population are presented in Table 2. There were 125 (38.9%) cases of selective coronary angiography, 101 (31.5%) cases of percutaneous coronary intervention, 33 (10.3%) with pacemaker implantation, 15 (4.7%) with heart valve replacement, 198 (61.7%) with platelet inhibitors, 146 (45.5%) with Beta-blockers, 160 (49.8%) with angiotensin-converting enzyme inhibitors, 32 (10.0%) with the application of sacubitril-valsartan, 125 (38.9%) of diuretics, 180 (56.1%) of statins, 52 (16.2%) of calcium antagonists, and 42 (13.1%) of nitrates.
Table 2
Treatments | Value (N=321), n (%) |
---|---|
SCA | 125 (38.9) |
PCI | 101 (31.5) |
Pacemaker implantation | 33 (10.3) |
Valve replacement | 15 (4.7) |
Drugs | |
Platelet inhibitors | 198 (61.7) |
Beta-blockers | 146 (45.5) |
ACEI | 160 (49.8) |
Sacubitril/valsartan | 32 (10.0) |
Diuretics | 125 (38.9) |
Statins | 180 (56.1) |
Calcium antagonists | 52 (16.2) |
Nitrates | 42 (13.1) |
SCA, selective coronary angiography; PCI, percutaneous coronary intervention; ACEI, angiotensin-converting enzyme inhibitors.
EF-CT for evaluating the clinical diagnostics
EF-CT analysis was performed successfully on 319 patients (99%). Patients were classified as having significant cardiac dysfunction if the EF-CT value measured by echocardiography was <50%. Our results showed that the sensitivity and specificity of EF measured by cardiac CT reached a high level of more than 90%, and the positive diagnostic value was up to 96% (Table 3).
Table 3
Metrics | Values, % (95% CI) |
---|---|
Sensitivity | 91 (86–94) |
Specificity | 92 (86–96) |
Positive predictive value | 96 (92–98) |
Negative predictive value | 82 (73–88) |
EF-CT, ejection fraction-computed tomography; EF, ejection fraction; CI, confidence intervals.
Upon stratifying patients based on their EF-CT values, a pronounced upward trend in the proportion of true positive cases was observed as the interval value diminished. This trend not only underscores the precision of CT in gauging the left ventricular EF but also highlights its clinical relevance (Table 4). Analysis of the data revealed that when the EF-CT value fell below 50%, the prevalence of true positives exceeded 80%, affirming the efficacy of this diagnostic criterion. High consistency was also shown when EF-CT was greater than 60%. Figure 3 shows a good correlation and regression results between EF-CT and EF values in all cases (R square =0.704, r=0.837), and Figure 4 shows the Bland-Altman analysis between EF and EF-CT (mean bias 6.6%, mean percentage error 27.5%, 95% limit of agreement −17% to 30%). The overall distribution of patient-level EF-CT values is shown in Table 4.
Table 4
EF-CT range, % | Patients, n | EF <50%, n (%) |
---|---|---|
≥60 | 77 | 4 (5.2) |
50–59.9 | 34 | 16 (47.1) |
40–49.9 | 31 | 25 (80.6) |
30–39.9 | 40 | 39 (97.5) |
20–29.9 | 51 | 51 (100) |
<20 | 86 | 85 (98.8) |
Kendall’s tau-b test for trend (P<0.05). EF-CT, ejection fraction-computed tomography; EF, ejection fraction.
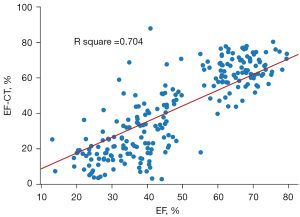
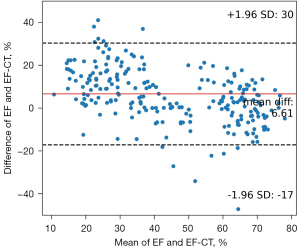
Discussion
In this study, cardiac CT-based measurements of left ventricular EF and its diagnostic performance with heart failure were compared with cardiac ultrasound. The results demonstrated good agreement between EF-CT and EF for evaluating cardiac insufficiency.
The agreement between the EF-CT and EF can be interpreted in two ways. The first is a direct comparison of quantitative results of EF values. Regression analysis based on ordinary least squares (OLS) and Bland-Altman analysis showed excellent agreement between EF-CT and EF (Figures 3,4), confirming the high consistency and reliability of EF values calculated from AccuLV based on CT images and EF values calculated from ultrasound images. The second aspect is based on the qualitative assessment of the diagnostic performance of heart failure, mainly involving the evaluation of diagnostic consistency. In this regard, EF-CT achieved a sensitivity and specificity of 0.9 or more for the diagnosis of heart failure (Table 3), and post-stratification patient statistics also showed that the difference between them was not significant, especially for EF-CT values in the lower intervals (Table 4). Nearly half of heart failure cases among patients are attributed to a diminished left ventricular EF, increasingly emerging as a critical and conspicuous issue within public health. Conversely, while cardiac ultrasound stands as the most expedient and swift method for assessing cardiac function at present, it frequently encounters challenges such as the poor reproducibility of outcomes and a significant dependency on the operator’s practical expertise. Consequently, from a standpoint of consistency, EF-CT emerges as a viable alternative diagnostic criterion for evaluating cardiac functionality.
Recent advances in deep learning for medical imaging have led to the emergence of an AI-based method for automatically segmenting the contour of the LV and calculating EF using convolutional neural networks (14-17). These approaches are not limited to any specific imaging modality, providing strong adaptability to different types of images. Compared to traditional methods, the AI-based approach is faster and more accurate and can continuously improve its performance with increasing data, making it a promising alternative for EF calculation (18-20). Although most of the algorithms have achieved good performance, most of these studies have been limited to the thesis research stage. Very few have successfully transitioned to software tools that can be used in medical imaging-assisted diagnosis. In contrast, AccuLV is a left ventricular function assessment software that employs deep learning algorithms for clinical practice. The research presented in this paper demonstrates that AccuLV has high accuracy and reliability, making it a suitable tool for clinical use.
There are some limitations to our study. Firstly, this is a single-center study, which makes the generalizability of the findings questionable. In fact, some of the results in Table 4 also show that EF-CT still has some deviation with EF, especially in the range of 50–59.9%, demonstrating significant inconsistency. We will extend this study to multiple centers in the future to further validate our findings. Secondly, we used the EF calculated based on cardiac ultrasound as the standard value for EF-CT rather than cardiac magnetic resonance (CMR), which is recognized as the gold standard for judging the structural and functional assessment of the heart (21-25). However, in cases where CMR examinations are uncommon, there is nothing particularly inappropriate about using the more convenient and widely accepted cardiac ultrasound as the reference standard. Finally, the AccuLV automated coronary CT analysis software developed based on the deep learning U-Net network may be biased due to factors such as inadequate model training samples and poor generalization of the segmentation algorithm. However, this is only a potential shortcoming, and the results of our validation show that AccuLV’s calculations have excellent accuracy and reliability. We will follow up with further validation of AccuLV on data from multiple centers.
Conclusions
CT-based calculations yielded EF with excellent correlation and agreement with ultrasound-based EF, especially using the AI model-driven AccuLV-based software. As for the diagnostic performance of EF-CT, further validation in a multicenter prospective study is needed.
Acknowledgments
Funding: The study was funded by
Footnote
Reporting Checklist: The authors have completed the STARD reporting checklist. Available at https://qims.amegroups.com/article/view/10.21037/qims-23-1864/rc
Conflicts of Interest: All authors have completed the ICMJE uniform disclosure form (available at https://qims.amegroups.com/article/view/10.21037/qims-23-1864/coif). W.L., X.L. and J.X. are employees of ArteryFlow Technology company. The other authors have no conflicts of interest to declare.
Ethical Statement: The authors are accountable for all aspects of the work in ensuring that questions related to the accuracy or integrity of any part of the work are appropriately investigated and resolved. The study was conducted in accordance with the Declaration of Helsinki (as revised in 2013). The study was approved by the Medical Ethics Committee of the Second Affiliated Hospital of Zhejiang University School of Medicine (No. I2021001277). As a retrospective study based on historical patient images, no contact with patients was required, so the requirement of informed consent for this retrospective analysis was waived.
Open Access Statement: This is an Open Access article distributed in accordance with the Creative Commons Attribution-NonCommercial-NoDerivs 4.0 International License (CC BY-NC-ND 4.0), which permits the non-commercial replication and distribution of the article with the strict proviso that no changes or edits are made and the original work is properly cited (including links to both the formal publication through the relevant DOI and the license). See: https://creativecommons.org/licenses/by-nc-nd/4.0/.
References
- Maeder MT, Kaye DM. Heart failure with normal left ventricular ejection fraction. J Am Coll Cardiol 2009;53:905-18. [Crossref] [PubMed]
- Khan MA, Hashim MJ, Mustafa H, Baniyas MY, Al Suwaidi SKBM, AlKatheeri R, Alblooshi FMK, Almatrooshi MEAH, Alzaabi MEH, Al Darmaki RS, Lootah SNAH. Global Epidemiology of Ischemic Heart Disease: Results from the Global Burden of Disease Study. Cureus 2020;12:e9349. [Crossref] [PubMed]
- Grand J, Kjaergaard J, Bro-Jeppesen J, Wanscher M, Nielsen N, Lindholm MG, Thomsen JH, Boesgaard S, Hassager C. Cardiac output, heart rate and stroke volume during targeted temperature management after out-of-hospital cardiac arrest: Association with mortality and cause of death. Resuscitation 2019;142:136-43. [Crossref] [PubMed]
- Romero-Farina G, Aguadé-Bruix S. Equilibrium radionuclide angiography: Present and future. J Nucl Cardiol 2021;28:1315-22. [Crossref] [PubMed]
- Vieillard-Baron A, Millington SJ, Sanfilippo F, Chew M, Diaz-Gomez J, McLean A, Pinsky MR, Pulido J, Mayo P, Fletcher N. A decade of progress in critical care echocardiography: a narrative review. Intensive Care Med 2019;45:770-88. [Crossref] [PubMed]
- LeCun Y, Bengio Y, Hinton G. Deep learning. Nature 2015;521:436-44. [Crossref] [PubMed]
- Asch FM, Mor-Avi V, Rubenson D, Goldstein S, Saric M, Mikati I, Surette S, Chaudhry A, Poilvert N, Hong H, Horowitz R, Park D, Diaz-Gomez JL, Boesch B, Nikravan S, Liu RB, Philips C, Thomas JD, Martin RP, Lang RM. Deep Learning-Based Automated Echocardiographic Quantification of Left Ventricular Ejection Fraction: A Point-of-Care Solution. Circ Cardiovasc Imaging 2021;14:e012293. [Crossref] [PubMed]
- Rostami B, Fetterly K, Attia Z, Challa A, Lopez-Jimenez F, Thaden J, Asirvatham S, Friedman P, Gulati R, Alkhouli M. Deep learning to estimate left ventricular ejection fraction from routine coronary angiographic images. JACC Adv 2023;2:100632.
- Zhang J, Yang L, Hu Y, Leng X, Huang W, Liu Y, Liu X, Wang L, Zhang J, Li D, Tang L, Xiang J, Du C. Calculation of left ventricular ejection fraction using an 8-layer residual U-Net with deep supervision based on cardiac CT angiography images versus echocardiography: a comparative study. Quant Imaging Med Surg 2023;13:5852-62. [Crossref] [PubMed]
- Thomas JD, Popović ZB. Assessment of left ventricular function by cardiac ultrasound. J Am Coll Cardiol 2006;48:2012-25. [Crossref] [PubMed]
- Ronneberger O, Fischer P, Brox T. U-Net: Convolutional networks for biomedical image segmentation. International Conference on Medical image computing and computer-assisted intervention. In Proceedings of 18th International Conference on Medical Image Computing and Computer-Assisted Intervention, 2015;234-41.
- He K, Zhang X, Ren S, Sun J. Deep residual learning for image recognition. Proceedings of the IEEE Conference on Computer Vision and Pattern Recognition (CVPR), 2016:770-8.
- Sayers A, Crowther MJ, Judge A, Whitehouse MR, Blom AW. Determining the sample size required to establish whether a medical device is non-inferior to an external benchmark. BMJ Open 2017;7:e015397. [Crossref] [PubMed]
- Moradi S, Oghli MG, Alizadehasl A, Shiri I, Oveisi N, Oveisi M, Maleki M, Dhooge J. MFP-Unet: A novel deep learning based approach for left ventricle segmentation in echocardiography. Phys Med 2019;67:58-69. [Crossref] [PubMed]
- Ge R, Yang G, Chen Y, Luo L, Feng C, Ma H, Ren J, Li S. K-Net: Integrate Left Ventricle Segmentation and Direct Quantification of Paired Echo Sequence. IEEE Trans Med Imaging 2020;39:1690-702. [Crossref] [PubMed]
- Li C, Song X, Zhao H, Feng L, Hu T, Zhang Y, Jiang J, Wang J, Xiang J, Sun Y. An 8-layer residual U-Net with deep supervision for segmentation of the left ventricle in cardiac CT angiography. Comput Methods Programs Biomed 2021;200:105876. [Crossref] [PubMed]
- Koo HJ, Lee JG, Ko JY, Lee G, Kang JW, Kim YH, Yang DH. Automated Segmentation of Left Ventricular Myocardium on Cardiac Computed Tomography Using Deep Learning. Korean J Radiol 2020;21:660-9. [Crossref] [PubMed]
- Yuan N, Jain I, Rattehalli N, He B, Pollick C, Liang D, Heidenreich P, Zou J, Cheng S, Ouyang D. Systematic Quantification of Sources of Variation in Ejection Fraction Calculation Using Deep Learning. JACC Cardiovasc Imaging 2021;14:2260-2. [Crossref] [PubMed]
- Li H, Wang Y, Qu M, Cao P, Feng C, Yang J. EchoEFNet: Multi-task deep learning network for automatic calculation of left ventricular ejection fraction in 2D echocardiography. Comput Biol Med 2023;156:106705. [Crossref] [PubMed]
- Kulkarni P, Madathil D. Fully automatic segmentation of LV from echocardiography images and calculation of ejection fraction using deep learning. Int J Biomed Eng Technol 2022;40:241-61.
- Alfakih K, Reid S, Jones T, Sivananthan M. Assessment of ventricular function and mass by cardiac magnetic resonance imaging. Eur Radiol 2004;14:1813-22. [Crossref] [PubMed]
- Lau C, Elshibly MMM, Kanagala P, Khoo JP, Arnold JR, Hothi SS. The role of cardiac magnetic resonance imaging in the assessment of heart failure with preserved ejection fraction. Front Cardiovasc Med 2022;9:922398. [Crossref] [PubMed]
- Papanastasiou CA, Bazmpani MA, Kokkinidis DG, Zegkos T, Efthimiadis G, Tsapas A, Karvounis H, Ziakas A, Kalogeropoulos AP, Kramer CM, Karamitsos TD. The prognostic value of right ventricular ejection fraction by cardiovascular magnetic resonance in heart failure: A systematic review and meta-analysis. Int J Cardiol 2022;368:94-103. [Crossref] [PubMed]
- Barison A, Aimo A, Todiere G, Grigoratos C, Aquaro GD, Emdin M. Cardiovascular magnetic resonance for the diagnosis and management of heart failure with preserved ejection fraction. Heart Fail Rev 2022;27:191-205. [Crossref] [PubMed]
- Li H, Zheng Y, Peng X, Liu H, Li Y, Tian Z, Hou Y, Jin S, Huo H, Liu T. Heart failure with preserved ejection fraction in post myocardial infarction patients: a myocardial magnetic resonance (MR) tissue tracking study. Quant Imaging Med Surg 2023;13:1723-39. [Crossref] [PubMed]