Free-breathing motion corrected magnetic resonance elastography of the abdomen
Introduction
Magnetic resonance (MR) elastography (MRE) is a non-invasive imaging technique that uses an external vibration transducer, a motion-sensitive MR sequence, and an inversion algorithm to quantify soft tissue viscoelastic properties (1). These properties can reflect tissue microenvironment, such as tumor stroma in pancreatic ductal adenocarcinoma (PDAC) (2). MRE has been shown to be valuable in a number of abdominal organs, including the assessment of liver fibrosis (3). Renal MRE has shown promising results in healthy volunteers (4) and in patients as a biomarker for chronic kidney disease and for potential detection of Lupus Nephritis (5,6). Recently, the benefit of using MRE in determining tumor boundaries in PDAC has been investigated, as computed tomography (CT) often shows diffuse and ill-defined boundaries (7). Another study has shown the importance of using MRE to predict lymph node metastases in prostate cancer (8).
However, breathing motion can be detrimental for quantitative accuracy in abdominal MRE, particularly in small structures (9). In MRE data of the same structures is acquired multiple times, as several motion-encoding-gradient (MEG) directions and wave-offsets are required for a full dataset (10). During the acquisition breathing motion causes misalignment between these repeated measurements, therefore hampering accuracy. Breathing mitigation techniques, such as repeated breath-holding, are used to partially overcome this (11). However, this can introduce position-based errors across breath-holds and increases protocol time. Recent work has looked into the possibility of single breath hold acquisitions (12,13). However, the prolonged breath hold is uncomfortable for patients and limits data richness and precision. Moreover, moving to larger field of views (FOV) [e.g., whole liver coverage at adequate resolution (≤4 mm)] or increased spatial or temporal resolution drastically increases both number of breath-holds and their durations. Multi-frequency MRE adds another dimension and hence is often performed during free-breathing, allowing for a time-efficient acquisition and highly resolved stiffness maps. However, abdominal organs move substantially with respiration, potentially hampering accurate analysis due to misalignment over all dimensions (MEG directions, wave-offsets, and frequencies) of the MRE acquisition (14).
MRE is typically performed in an axial orientation to match anatomical scans. Approaches have been explored to correct respiratory motion in coronal single-shot spin-echo echo-planar-imaging (SE-EPI) MRE (9), however this work did not consider foot-head (FH) and anterior-posterior (AP) motion, both of which are present and substantial in the abdomen, as AP motion is not in-plane in a coronal acquisition. We hypothesize that a sagittal acquisition is more conducive to resolving these FH and AP motions using motion correction algorithms, as these motions are both in-plane in sagittal orientation. This study investigated the best motion correction strategies for sagittal multi-frequency free-breathing SE-EPI MRE acquisitions, in which we hypothesize that motion correction improved MRE and image quality to increase accuracy in estimation of viscoelastic properties of the abdomen without waiving patient comfort. This was first done through phantom experiments with simulated noise and motion. Next, all motion correction methods were compared using a ranking system in healthy volunteers. Upon which, the best motion correction was compared to no motion correction. Lastly, feasibility in patients was shown.
Methods
In silico experiments
Digital in silico simulations were conducted with an open-source agar-based phantom dataset, which can be accessed through BIOQIC-Downloads (https://bioqic-apps.charite.de/downloads) (15). The phantom comprises four parallel cylindrical inclusions consisting of different gel-water ratios, each possessing known shear wave speed (SWS) values of 3.2, 5.9, 2.2, 2.4 m/s, alongside a background material with a SWS of 3.8 m/s (16). The phantom data has a 1.5 mm3 isotropic voxel size and was acquired with mechanical frequencies ranging from 30 to 100 Hz with a 10 Hz increment and synchronized MEGs. In this specific application mechanical frequencies of 30, 40, 50, and 60 Hz, and inclusions exhibiting a stiffness less than 4 m/s, mirroring conditions typically encountered in malignancies in vivo, were chosen. The inclusion exhibiting higher stiffness was not taken into account as this could potentially influence outcomes.
To mimic in vivo breathing motion inaccuracies and explore the effect on MRE quality, simulated periodic breathing motion was introduced in the static phantom data both in the magnitude and phase signals, see Figure 1A. Three motion levels were used (6, 12, and 18 voxels of displacement) with manually defined translation matrices in AP. This simulated breathing motion was thereupon resolved using each motion correction method (see section motion-correction). In the supplementary information random noise was iteratively introduced in the static phantom dataset to explore the dependency of MRE on signal-to-noise (Appendix 1).
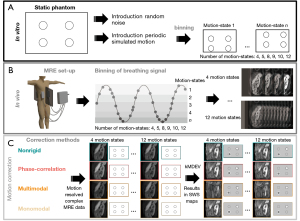
MRI protocol
The study was conducted in accordance with the Declaration of Helsinki (as revised in 2013). The study was approved by institutional ethics committee of the Amsterdam University Medical Centers (UMC), location Amsterdam Medical Center (AMC) (No. NL73810.018.20). All participants were 18 years or older and gave written informed consent. Participants were screened for general MRI contradictions. A total of eight healthy volunteers (♀ =5, ♂ =3, age =29±3 years) were enrolled with no known history of renal, hepatic, or pancreatic diseases. Subsequently, five patients diagnosed with pathologically proven PDAC (♀ =1, ♂ =4, mean age =69±11 years) were included. To show feasibility of MRE in other abdominal organs a patient with known metabolic dysfunction-associated steatotic liver disease (MASLD) was included (♂, age =42 years).
All in vivo MRE data was acquired with a 3T MR scanner (Ingenia, Philips, Best, The Netherlands) using a multi-slice, multi-frequency SE-EPI MRE sequence (7). Healthy volunteers underwent two consecutive sagittal MRE acquisitions for repeatability assessment. Healthy volunteers were repositioned between consecutive MRE acquisitions. Patients underwent one sagittal MRE scan. Mechanical vibrations were introduced using four pneumatic drivers placed on the lower thoracic cage (two anterolateral, two posterolateral; see Figure 1A) and held in their place using an elastic strap. The MR scanner respiratory belt (Philips, Best, The Netherlands) was placed on the lower abdomen to record the respiratory signal throughout each scan. Sagittal MRE data were acquired with 3 mm3 isotropic resolution, with an in-plane FOV of 336 mm (FH) × 255 mm (AP) and through-plane FOV of 45–120 mm (right-left, 15–40 slices). The echo time (TE) was 55 ms, the repetition time (TR) was 2,400 ms, and sensitivity encoding (SENSE) factor was 2.5. Four mechanical frequencies (30, 40, 50, and 60 Hz) were acquired at eight wave phase offsets and three motion encoding directions (17). The protocol for all participants consisted of T2-weighted turbo spin echo (T2w-TSE) images in sagittal and axial orientation for anatomical reference.
Motion correction
In total four registration methods were investigated combined with a different number of motion states. First, a stand-alone non-rigid registration was used. Moreover, three intensity-based registration methods were explored in all datasets: monomodal, multimodal, and phase-correlation, using a regular step-gradient descent optimizer, one-plus-one revolutionary optimizer, and windowing in the frequency domain, respectively. In short, monomodal registration uses a mean square error metric to register images. Multimodal makes use of the Mattes mutual information metric, which is an algorithm that uses a joint probability distribution of a single set of pixel locations from two images (18). Phase correlation makes use of the Fourier shift theorem to detect translations, rotations and scaling in the frequency domain (19). Pre-processing normalization and similarity registration were applied for all three methods. When applying motion correction in the pancreas the total FOV was reduced to minimize the influence of the static spine. The automated process involved selecting the center half in the AP and FH direction.
Each image slice of a single wave phase offset and encoding direction was binned into a respiratory motion state as determined from the height of the respiratory belt signal during acquisition. For in silico experiments the periodic motion was used for binning in motion states. The effect of bin size was explored by varying the number of motion states (4, 5, 8, 9, 10, and 12) for each motion correction method. All motion states were registered to the end-expiration state using the motion correction methods applied in the magnitude images, after which the geometric translations were imposed on the real and imaginary parts of the complex data. This resulted in 24 different motion resolved complex MRE datasets per subject scan. A schematic overview of this can be found in Figure 1B,1C.
Post-processing
Biomechanical inversion of the motion-resolved and original complex MRE data (in silico and in vivo) was performed using the wavenumber-based multifrequency dual elastovisco (k-MDEV) and multifrequency dual elastovisco (MDEV) reconstruction algorithm resulting in spatial frequency-resolved SWS and phase angle (ϕ) maps, respectively (15,17). The open-source algorithm is available at https://bioqic-apps.charite.de/ (version 1.0). Mean magnitude MRE images are created for anatomical reference by taking the mean over all wave-phase offsets, motion encoding directions and frequencies for each slice.
In the in silico experiments regions-of-interest (ROI) were drawn over the inclusions and the background in the phantom mean magnitude MRE data. For each healthy volunteer, ROIs were drawn on the mean magnitude MRE data over the pancreas and kidney in the original free-breathing anatomical magnitude images to determine the best method in the ranking analysis. The ROI was eroded by approximately 2 voxels in Matlab (R2022a, Mathworks, Natick, MA, USA). This was done to encompass the center of the healthy pancreas and kidney ROIs without boundaries for further ranking analysis. Thereupon, the average MRE (quality) parameters were determined in each ROI.
Ranking analysis of all methods was based on different quality parameters in MRE (in silico and in vivo). Quality parameters were defined as: displacement signal-to-noise (displacement-SNR) for both the pancreas and kidney (16), Laplacian-SNR: the variance (σ) of the Laplacian (Δ) of the MRE wave data over the whole image (9), octahedral-shear-strain-SNR (OSS-SNR) within the pancreas and kidney (20,21), the sharpness of the SWS maps (sharpness) calculated by iteratively smoothing the SWS map five times and determining the gradient of the resulting smoothened image (22), and lastly, a sum of the relative SWS difference with the ground truth per inclusion and background for the simulated breathing resolved data of the phantom (phantom accuracy).
Each quality parameter was assigned a relative rank score per method using mean-normalized values across methods. Lastly, the sum of all ranks resulted in the overall ranking of the best motion correction method for sagittal MRE.
This best motion correction method was then applied in the two consecutive sagittal MRE acquisitions in healthy volunteers and the single MRE acquisition in patients. ROIs were drawn on the corrected and original free-breathing MRE data in the pancreas and kidney for all healthy volunteers. Subsequently, ROIs were drawn in the lesion or affected liver for all patients. Average SWS and ϕ were calculated for all phantom datasets, healthy volunteer datasets, and patient data.
Statistical analysis
All healthy volunteer data were tested on normality using the Shapiro-Wilk test. SWS from the best motion correction method and non-motion-corrected data were compared using repeated measures analysis of variance (ANOVA) or, depending on normality, the Friedman test and, where applicable, pairwise comparison with Bonferroni correction. A significance threshold was set at a P value of <0.05. Repeatability was assessed in healthy volunteers using Bland-Altman analysis, the intraclass correlation coefficient (ICC), and repeatability coefficient (RC) (23). Image registration and analysis, delineation, and statistical analysis were performed in Matlab (R2022a, Mathworks, Natick, MA, USA), ITK-snap (v3.8.0; http://www.itksnap.org/), and SPSS (version 28; IBM Corp., Armonk, NY, USA), respectively.
Results
In silico experiments
Magnitude images and SWS maps of the phantom before and after breathing motion simulation are shown in Figure 2. The median SWS of the static phantom, after the introduction of motion and after motion correction using monomodal registration are shown in Figure 3. Magnitude images and corresponding SWS maps with added random noise can be seen in the supplementary material Figure S1. The median SWS for each iteration of added random noise as a function of displacement-, OSS- and Laplacian-SNR are shown in Figure S2.
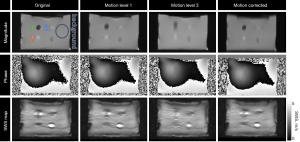
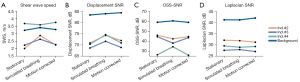
Healthy volunteers
Twenty-five different motion correction strategies and original non-motion-corrected data were ranked for all quality measures, see Table 1. The method with the best overall quality measures in the pancreas was monomodal registration in nine motion states, whilst the method that showed the lowest score in quality measures was Original. The mean magnitude images and corresponding SWS maps are shown in Figure 4 for the best motion correction method and no motion correction of a representative healthy volunteer. In the supplementary material Figure S3 an example of different motion states are shown.
Table 1
Index ranking | Method | Number of bins | Displacement SNR pancreas (dB) | Displacement SNR kidney (dB) | OSS-SNR pancreas (dB) | OSS-SNR kidney (dB) | Laplacian SNR (dB) | Sharpness in SWS map | Phantom accuracy |
---|---|---|---|---|---|---|---|---|---|
1 | Monomodal | 9 | 39.02 | 42.59 | 12.84 | 17.21 | 11.44 | 0.000176 | 0.77 |
2 | Monomodal | 10 | 39.09 | 42.64 | 12.39 | 17.34 | 11.42 | 0.000172 | 0.79 |
3 | Monomodal | 8 | 38.96 | 42.68 | 12.75 | 17.34 | 11.37 | 0.000180 | 0.80 |
4 | Monomodal | 5 | 38.66 | 42.68 | 12.72 | 17.33 | 11.25 | 0.000179 | 0.81 |
5 | Phase correlation | 9 | 38.04 | 41.76 | 12.73 | 16.97 | 11.05 | 0.000178 | 0.82 |
6 | Nonrigid | 12 | 39.24 | 42.29 | 12.44 | 17.16 | 11.03 | 0.000185 | 0.80 |
7 | Phase correlation | 8 | 38.18 | 41.77 | 12.48 | 16.84 | 11.14 | 0.000183 | 0.79 |
8 | Monomodal | 12 | 38.41 | 42.70 | 12.26 | 17.10 | 11.24 | 0.000175 | 0.79 |
9 | Nonrigid | 10 | 39.24 | 41.90 | 12.78 | 17.03 | 10.90 | 0.000190 | 0.79 |
10 | Monomodal | 4 | 38.26 | 42.43 | 12.70 | 17.19 | 11.04 | 0.000173 | 0.80 |
11 | Phase correlation | 12 | 37.25 | 41.66 | 12.79 | 16.75 | 10.79 | 0.000171 | 0.80 |
12 | Nonrigid | 9 | 39.05 | 42.05 | 12.81 | 17.02 | 10.78 | 0.000174 | 0.80 |
13 | Nonrigid | 8 | 38.93 | 42.07 | 12.61 | 17.33 | 10.81 | 0.000179 | 0.71 |
14 | Phase correlation | 10 | 37.76 | 40.13 | 12.93 | 15.84 | 11.20 | 0.000175 | 0.75 |
15 | Phase correlation | 5 | 38.24 | 41.84 | 12.57 | 16.99 | 11.15 | 0.000179 | 0.85 |
16 | Nonrigid | 5 | 38.88 | 41.93 | 13.01 | 17.30 | 10.74 | 0.000173 | 0.86 |
17 | Phase correlation | 4 | 38.12 | 42.11 | 12.35 | 17.21 | 11.04 | 0.000172 | 0.88 |
18 | Multimodal | 5 | 37.93 | 40.77 | 13.15 | 15.89 | 10.97 | 0.000162 | 0.85 |
19 | Nonrigid | 4 | 38.79 | 42.01 | 12.82 | 17.25 | 10.71 | 0.000170 | 0.67 |
20 | Multimodal | 12 | 37.97 | 40.70 | 13.16 | 15.61 | 10.95 | 0.000174 | 0.71 |
21 | Multimodal | 8 | 38.04 | 40.67 | 12.91 | 15.97 | 10.88 | 0.000182 | 0.76 |
22 | Multimodal | 10 | 38.23 | 40.51 | 12.93 | 15.82 | 10.88 | 0.000168 | 0.78 |
23 | Multimodal | 9 | 38.14 | 40.55 | 12.81 | 15.78 | 10.90 | 0.000179 | 0.80 |
24 | Multimodal | 4 | 38.16 | 41.01 | 12.21 | 16.05 | 10.95 | 0.000178 | 0.82 |
25 | Original | 0 | 36.52 | 40.83 | 12.26 | 16.49 | 10.07 | 0.000167 | 0.79 |
SNR, signal-to-noise ratio; OSS, octahedral shear strain; SWS, shear wave speed.
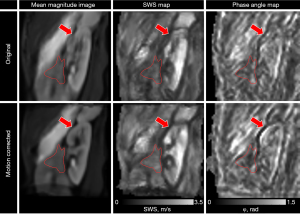
Original sagittal scans had a displacement SNR of 36.6 dB and an OSS-SNR of 12.2 dB in the pancreas, a displacement SNR of 40.8 dB and an OSS-SNR of 16.5 dB in the kidney, a Laplacian-SNR of 10.1 dB, and a sharpness of 0.000167 in the SWS map. The best motion correction method had a displacement SNR of 39.0 dB (P=0.01) and an OSS-SNR of 12.8 dB (P=0.39) in the pancreas, a displacement SNR of 42.6 dB (P=0.01) and an OSS-SNR of 17.2 dB (P=0.17) in the kidney, a Laplacian-SNR of 11.44 dB (P=0.002), and a sharpness of 0.000176 after five iterations of smoothing in the SWS map (P=0.29). In Figure 5, the SNR measures are shown per method and bin size for each healthy volunteer. The rain-plot shows density curves with colored lines representing the median and interquartile range. Each dot represents separate values for number of motion states per method and the SNR measures of the non-motion corrected scans are shown through a dashed line.
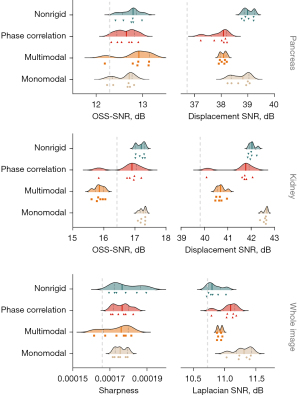
Healthy volunteer repeatability for Original scan without motion correction and Best motion correction method is shown in Figure 6A using Bland-Altman analysis. Limits of agreement were (−0.25, 0.18) m/s and (−0.18, 0.14), for Original and Best motion correction method in the pancreas, respectively. The limits of agreement for the kidney were (−0.13, 0.23) m/s and (−0.17, 0.14) m/s, for Original and Best motion correction method, respectively. Repeatability analysis showed an ICC =0.726 and RC =0.16 m/s for Original and ICC =0.903 and RC =0.16 m/s for Best motion correction method in the pancreas. In the kidney the ICC was 0.971 before motion correction, while after motion correction the ICC =0.972. Test for normality revealed no significant indication for a non-normal distribution. The average SWS and φ were compared before and after the best motion correction method and shown in Figure 6B.
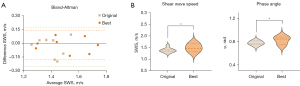
The pancreas had an average SWS of 1.39±0.10 and 1.50±0.17 m/s for no motion correction and the best motion correction method, respectively (P=0.036). The pancreas had an average φ of 0.78±0.04 and 0.82±0.07 rad for no motion correction and the best motion correction method, respectively (P=0.039). The kidney showed no significant difference between no motion correction (SWS =2.27±0.25 and φ =0.83±0.08 rad) and best motion correction (SWS =2.36±0.20 and φ =0.83±0.07 rad) (SWS: P=0.08 and φ: P=0.35).
Outlook of patient data
In Figure 7 the mean magnitude images, corresponding SWS maps for a patient with PDAC and a participant with a simple renal cyst are shown for the best motion correction method and no motion correction. The PDAC patients had an average SWS of 1.42±0.25 m/s before motion correction and 1.56±0.27 m/s after motion correction using monomodal registration in nine motion states (P=0.01). The average φ for PDAC patients were 0.93±0.13 rad and 0.98±0.14 rad (P=0.10) before and after motion correction, respectively. Motion correction on sagittal liver MRE resulted in an average SWS of 2.07±0.20 m/s, while in free-breathing this was 2.12±0.18 m/s. Figure 8 displays magnitude images in sagittal orientation and resolved for the coronal and axial orientation before and after motion correction. Additionally, the corresponding SWS map resolved in the axial orientation is presented.
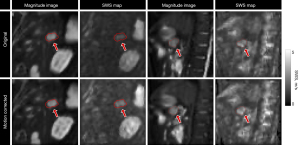
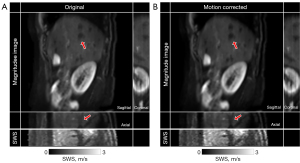
Discussion
This study investigated motion correction strategies on sagittal multi-frequency free-breathing SE-EPI MRE acquisitions to resolve all breathing motions without hampering patient comfort. The implementation of post-processing motion correction yielded improved quality metrics, including displacement-, OSS-, Laplacian-SNR, and SWS map sharpness compared to no motion correction with all data showing an OSS-SNR above 3 dB, indicating stable and reproducible results (20). Moreover, breathing motion was simulated through in silico experiments in a phantom dataset that has inclusions with predefined stiffness values. We showed that motion correction could (partially) resolve the original SWS map, thereby underscoring the potential in enhancing MRE quality under dynamic physiological conditions.
Ranking the quality metrics revealed that monomodal registration in nine motion states is the best motion correction method out of the methods tested in this paper for abdominal MRE in sagittal orientation. When comparing the different quality metrics for each method, monomodal registration consistently has the highest values, while multimodal registration showed the lowest quality values. The optimal number of motion states will vary when the acquisition parameters change; for shorter scans (e.g., with fewer wave offsets) it may not be feasible to have nine motion states.
Both monomodal and multimodal image registration methods were tested. While in principle each repeated slice acquisition (for wave phase offset and encoding direction) should have the same signal intensity for SE-EPI, we observed occasional differences in intensity. These are likely due to characteristic EPI artifacts at 3T and were overcome through normalization during registration using monomodal and phase correlation methods. Multimodal registration is a method that potentially could register MR images with different contrasts and would hypothetically not need normalization. However, this method poses issues with registration of tissues with different contrast, such as the kidneys, which was reflected in the lower quality metrics compared to monomodal.
The introduction of simulated motion in the phantom data showed an increase of SWS for inclusions that have a lower stiffness than the surrounding background and a decrease of SWS for the stiffest inclusion that has a higher stiffness than the surrounding background; motion of two nearby tissues with different stiffness’s blurs their apparent SWS closer to the mean, increasing inaccuracy. This corroborates the hypothesis that the apparent SWS in free-breathing is affected by interference with the soft surrounding tissue in the pancreas.
While not thoroughly studied here, it is noteworthy that the respiratory bin size has a substantial influence on MRE quality. It can be seen that a relatively high (~12) or low (~4) number of motion states both show lesser rankings compared to the same registration method at ~8 motion states. A large number of respiratory bins does not benefit from slice-averaging across the MRE acquisition, potentially lowering image quality for motion correction. Conversely, a smaller number of bins could potentially cause blurring due to unresolved motion present in each motion state.
Contrary to recent work, which performed motion correction of coronal abdominal MRE at 1.5T using a two-dimensional rigid-body image registration method, this work showed increased apparent SWS after motion correction in the pancreas both in healthy volunteers and pancreatic lesions in PDAC patients (9). The cause of this could be due to the increased accuracy and reflect the ground truth more accurately as motion correction removes errors caused by misalignment and increases MRE SNR values. However, motion correction could artificially increase the SWS through altering the phase. Meyer et al. looked into the relation of OSS-SNR with underestimation of stiffness values as there is an dispersion-by-inversion bias for long and noisy waves, which are predominantly present in abdominal images (15). The noise of the waves could potentially be partially reduced by using motion correction, which leads to a milder underestimation of the SWS.
Feasibility of motion correction was shown in patients and results hinted at better defined boundaries of the lesions and anatomy in the liver. This could be crucial for clinical implementation of MRE to increase accuracy in characterization of pancreatic tumors, application as a predictive biomarker, and for future implementation as a surgical guidance for PDAC boundaries (7,24). There was an increase in SWS seen after motion correction in the tumor. It is hypothesized that this could be attributed to motion blurring at the tumor boundaries. Motion of the pancreatic tumor mostly affects the tumor boundaries, decreasing apparent SWS due to blurring with the surrounding tissue. This is substantiated by the phantom data, which evidenced heightened SWS in inclusions characterized by lower stiffness in comparison to the surrounding background, while registering a marginal reduction in SWS for inclusions possessing greater stiffness than the surrounding background. Nonetheless, this increase in SWS should be considered when applying motion correction. Similarly, as in pancreatic MRE, clinical application of renal MRE remains challenging due to the intricate and small structures, such as the outer- and inner-cortex and medulla, that show different viscoelastic properties (6,25). Teasing out the slight differences in viscoelastic properties that happen in these relatively small structures within the kidney could be improved through the use of motion correction strategies like the one presented here.
Motion corrected MRE on a patient with clinical liver fibrosis showed a comparable SWS with current literature, with SWS values indicating severe fibrosis (F4) (26). However, one-to-one comparison with literature values is not possible as different methodologies employ disparate transducer frequencies (3,26). While MRE is already a useful tool for assessing liver stiffness (27), post-acquisition motion correction of liver MRE may further enhance measurement accuracy. This increased accuracy would be useful in the probing of fibrotic heterogeneity (28) or the evaluation of hepatocellular carcinoma in which small tumors (less than 1 cm) are often unable to be measured (29).
Bland-Altman analysis showed an improved repeatability after using the best performing motion correction method, whilst no motion correction showed wider limits-of-agreement. Increased repeatability increases the robustness of this technique, which is critical in assessing MRE parameters across patient populations, in repeat examinations to assess treatment efficacy, and in multi-centre trials. This may have further implications on future clinical findings—particularly in smaller organs such as the pancreas for improved quantification of the heterogeneous tumor microenvironment.
This study has some limitations. The small subset of healthy volunteers and patients hampers firm conclusions on improved quality and accuracy. Secondly, while a sagittal oriented scan allows for the resolving and correction of both head-foot and AP motion, this orientation limits the slice coverage in the left-right direction. This may not be optimal when coverage of larger organs, such as the liver, is necessary. Third, the incorporation of simulated motion in a static phantom dataset fails to completely reflect the dynamic in vivo conditions of motion that occurs during acquisition. Motion occurring during acquisition elicits more than the induced misalignment between consecutive wave-phase offsets in the described phantom dataset. Notably, tissues will exhibit disparities in phase accumulation when positioned at different locations throughout the acquisition. Furthermore, the shear wave does not reach a steady state during free breathing in the tissue of interest. Lastly, simulated motion was periodic and in a single direction, which does not reflect in vivo conditions. Therefore, the observation of correlation of SWS with SNR needs further analysis. In this work, the phantom data was used explorative to show feasibility of using motion correction in MRE. In future research the effects of motion correction in MRE can be further investigated by the introduction of an in vivo breathing signal in a static phantom to simulate in vivo conditions more accurately. To determine effects of breathing on the shear wave steady state a moving phantom with known stiffness inclusions that shows dynamic motion during acquisition can be used to eliminate causation by motion correction on the shear stiffness estimation.
Conclusions
To conclude, ranking analysis revealed that monomodal registration with nine motion states shows the best MRE quality parameters. Motion correction in sagittal free-breathing SE-EPI MRE is promising, with improved data quality, inversion precision and repeatability compared to no correction.
Acknowledgments
Funding: This research was supported by
Footnote
Conflicts of Interest: All authors have completed the ICMJE uniform disclosure form (available at https://qims.amegroups.com/article/view/10.21037/qims-23-1727/coif). The authors have no conflicts of interest to declare.
Ethical Statement: The authors are accountable for all aspects of the work in ensuring that questions related to the accuracy or integrity of any part of the work are appropriately investigated and resolved. The study was conducted in accordance with the Declaration of Helsinki (as revised in 2013). The study was approved by institutional ethics committee of the Amsterdam University Medical Centers (UMC), location Amsterdam Medical Center (AMC) (No. NL73810.018.20). All participants were 18 years or older and gave written informed consent.
Open Access Statement: This is an Open Access article distributed in accordance with the Creative Commons Attribution-NonCommercial-NoDerivs 4.0 International License (CC BY-NC-ND 4.0), which permits the non-commercial replication and distribution of the article with the strict proviso that no changes or edits are made and the original work is properly cited (including links to both the formal publication through the relevant DOI and the license). See: https://creativecommons.org/licenses/by-nc-nd/4.0/.
References
- Venkatesh SK, Ehman RL. Magnetic Resonance Elastography. Springer, 2014.
- Wassenaar NPM, van Schelt AS, Schrauben EM, Kop MPM, Nio CY, Wilmink JW, Besselink MGH, van Laarhoven HWM, Stoker J, Nederveen AJ, Runge JH. MR Elastography of the Pancreas: Bowel Preparation and Repeatability Assessment in Pancreatic Cancer Patients and Healthy Controls. J Magn Reson Imaging 2024;59:1582-92. [Crossref] [PubMed]
- Troelstra MA, Witjes JJ, van Dijk AM, Mak AL, Gurney-Champion O, Runge JH, Zwirs D, Stols-Gonçalves D, Zwinderman AH, Ten Wolde M, Monajemi H, Ramsoekh S, Sinkus R, van Delden OM, Beuers UH, Verheij J, Nieuwdorp M, Nederveen AJ, Holleboom AG. Assessment of Imaging Modalities Against Liver Biopsy in Nonalcoholic Fatty Liver Disease: The Amsterdam NAFLD-NASH Cohort. J Magn Reson Imaging 2021;54:1937-49. [Crossref] [PubMed]
- Morrell GR, Zhang JL, Lee VS. Magnetic Resonance Imaging of the Fibrotic Kidney. J Am Soc Nephrol 2017;28:2564-70. [Crossref] [PubMed]
- Marticorena Garcia SR, Grossmann M, Bruns A, Dürr M, Tzschätzsch H, Hamm B, Braun J, Sack I, Guo J. Tomoelastography Paired With T2* Magnetic Resonance Imaging Detects Lupus Nephritis With Normal Renal Function. Invest Radiol 2019;54:89-97. [Crossref] [PubMed]
- Marticorena Garcia SR, Grossmann M, Lang ST, Tzschätzsch H, Dittmann F, Hamm B, Braun J, Guo J, Sack I. Tomoelastography of the native kidney: Regional variation and physiological effects on in vivo renal stiffness. Magn Reson Med 2018;79:2126-34. [Crossref] [PubMed]
- Marticorena Garcia SR, Zhu L, Gültekin E, Schmuck R, Burkhardt C, Bahra M, Geisel D, Shahryari M, Braun J, Hamm B, Jin ZY, Sack I, Guo J. Tomoelastography for Measurement of Tumor Volume Related to Tissue Stiffness in Pancreatic Ductal Adenocarcinomas. Invest Radiol 2020;55:769-74. [Crossref] [PubMed]
- Hu B, Deng Y, Chen J, Kuang S, Tang W, He B, Zhang L, Xiao Y, Chen J, Rossman P, Arani A, Yin Z, Glaser KJ, Yin M, Venkatesh SK, Ehman RL, Wang J. Evaluation of MR elastography for prediction of lymph node metastasis in prostate cancer. Abdom Radiol (NY) 2021;46:3387-400. [Crossref] [PubMed]
- Shahryari M, Meyer T, Warmuth C, Herthum H, Bertalan G, Tzschätzsch H, Stencel L, Lukas S, Lilaj L, Braun J, Sack I. Reduction of breathing artifacts in multifrequency magnetic resonance elastography of the abdomen. Magn Reson Med 2021;85:1962-73. [Crossref] [PubMed]
- Manduca A, Bayly PJ, Ehman RL, Kolipaka A, Royston TJ, Sack I, Sinkus R, Van Beers BE. MR elastography: Principles, guidelines, and terminology. Magn Reson Med 2021;85:2377-90. [Crossref] [PubMed]
- Keenan KE, Biller JR, Delfino JG, Boss MA, Does MD, Evelhoch JL, et al. Recommendations towards standards for quantitative MRI (qMRI) and outstanding needs. J Magn Reson Imaging 2019;49:e26-39. [Crossref] [PubMed]
- van Schelt AS, Gottwald LM, Wassenaar NPM, Runge JH, Sinkus R, Stoker J, Nederveen AJ, Schrauben EM. Single Breath-Hold MR Elastography for Fast Biomechanical Probing of Pancreatic Stiffness. J Magn Reson Imaging 2024;59:688-98. [Crossref] [PubMed]
- Darwish OI, Gharib AM, Jeljeli S, Metwalli NS, Feeley J, Rotman Y, Brown RJ, Ouwerkerk R, Kleiner DE, Stäb D, Speier P, Sinkus R, Neji R. Single Breath-Hold 3-Dimensional Magnetic Resonance Elastography Depicts Liver Fibrosis and Inflammation in Obese Patients. Invest Radiol 2023;58:413-9. [Crossref] [PubMed]
- Murphy IG, Graves MJ, Reid S, Patterson AJ, Patterson I, Priest AN, Lomas DJ. Comparison of breath-hold, respiratory navigated and free-breathing MR elastography of the liver. Magn Reson Imaging 2017;37:46-50. [Crossref] [PubMed]
- Meyer T, Marticorena Garcia S, Tzschätzsch H, Herthum H, Shahryari M, Stencel L, Braun J, Kalra P, Kolipaka A, Sack I. Comparison of inversion methods in MR elastography: An open-access pipeline for processing multifrequency shear-wave data and demonstration in a phantom, human kidneys, and brain. Magn Reson Med 2022;88:1840-50. [Crossref] [PubMed]
- Bertalan G, Guo J, Tzschätzsch H, Klein C, Barnhill E, Sack I, Braun J. Fast tomoelastography of the mouse brain by multifrequency single-shot MR elastography. Magn Reson Med 2019;81:2676-87. [Crossref] [PubMed]
- Tzschätzsch H, Guo J, Dittmann F, Hirsch S, Barnhill E, Jöhrens K, Braun J, Sack I. Tomoelastography by multifrequency wave number recovery from time-harmonic propagating shear waves. Med Image Anal 2016;30:1-10. [Crossref] [PubMed]
- Mattes D, Haynor DR, Vesselle H, Lewellyn TK, Eubank W. Non-rigid multimodality image registration. Medical Imaging: SPIE Publications, San Diego, CA, United States, 2001:1609-20.
- Reddy BS, Chatterji BN. An FFT-based technique for translation, rotation, and scale-invariant image registration. IEEE Trans Image Process 1996;5:1266-71. [Crossref] [PubMed]
- McGarry MD, Van Houten EE, Perriñez PR, Pattison AJ, Weaver JB, Paulsen KD. An octahedral shear strain-based measure of SNR for 3D MR elastography. Phys Med Biol 2011;56:N153-64.
- Donoho DL, Johnstone IM, Kerkyacharian G, Picard D. Wavelet Shrinkage: Asymptopia? Journal of the Royal Statistical Society: Series B (Methodological) 1995;57:301-37. [Crossref]
- Birdal T. Sharpness Estimation From Image Gradients. MATLAB Central File Exchange. 2023. Available online: https://www.mathworks.com/matlabcentral/fileexchange/32397-sharpness-estimation-from-image-gradients. Accessed October 2 2023.
- Shukla-Dave A, Obuchowski NA, Chenevert TL, Jambawalikar S, Schwartz LH, Malyarenko D, Huang W, Noworolski SM, Young RJ, Shiroishi MS, Kim H, Coolens C, Laue H, Chung C, Rosen M, Boss M, Jackson EF. Quantitative imaging biomarkers alliance (QIBA) recommendations for improved precision of DWI and DCE-MRI derived biomarkers in multicenter oncology trials. J Magn Reson Imaging 2019;49:e101-21. [Crossref] [PubMed]
- Patel K, Sebastiani G. Limitations of non-invasive tests for assessment of liver fibrosis. JHEP Rep 2020;2:100067. [Crossref] [PubMed]
- Serai SD, Yin M. MR Elastography of the Abdomen: Basic Concepts. Methods Mol Biol 2021;2216:301-23. [Crossref] [PubMed]
- Reiter R, Tzschätzsch H, Schwahofer F, Haas M, Bayerl C, Muche M, Klatt D, Majumdar S, Uyanik M, Hamm B, Braun J, Sack I, Asbach P. Diagnostic performance of tomoelastography of the liver and spleen for staging hepatic fibrosis. Eur Radiol 2020;30:1719-29. [Crossref] [PubMed]
- Hoodeshenas S, Yin M, Venkatesh SK. Magnetic Resonance Elastography of Liver: Current Update. Top Magn Reson Imaging 2018;27:319-33. [Crossref] [PubMed]
- Venkatesh SK, Yin M, Ehman RL. Magnetic resonance elastography of liver: technique, analysis, and clinical applications. J Magn Reson Imaging 2013;37:544-55. [Crossref] [PubMed]
- Liang J, Ampuero J, Castell J, Zhang Q, Zhang S, Chen Y, Romero-Gómez M. Clinical application of Magnetic resonance elastography in hepatocellular carcinoma: from diagnosis to prognosis. Ann Hepatol 2023;28:100889. [Crossref] [PubMed]