Magnetic resonance imaging-based prediction models for differentiating intraspinal schwannomas from meningiomas: classification and regression tree and random forest analysis
Introduction
Intradural extramedullary spinal meningiomas and schwannomas are frequently occurring tumors that are accompanied by progressive neurological symptoms. This well-documented fact is widely acknowledged in the medical community. These two growths comprise 55% of all initial neoplasms arising in the spinal region (1,2). In attempting to distinguish between the two lesions, healthcare professionals such as clinicians and radiologists have made use of imaging tools such as computed tomography (CT) and magnetic resonance imaging (MRI). These technologies are used to analyze the appearances of the lesions and identify any distinguishing features that can improve the accuracy of diagnosis. However, there are significant overlaps between features in traditional imaging and clinical settings in many cases, which can potentially lead to misdiagnosis (3). Hence, conventional imaging features should not be relied upon as sole means to differentiate spinal meningiomas from schwannomas.
Even though the majority of schwannomas and meningiomas are considered benign, it is crucial to have an accurate diagnosis prior to surgery since the surgical approach may differ depending on the type of the tumor (4,5). In the treatment of meningioma, the hard dura mater is partially removed along with the tumor to eradicate any remaining tumor cells, followed by a dural reconstruction surgery. Simultaneously, the arachnoid membrane can be conserved to avert the potential leakage of cerebrospinal fluid (CSF) post-surgery. This area must be conserved due to the presence of a meningioma that is located outside the subarachnoid space and beneath the dura mater. In contrast, schwannomas are located in the subarachnoid region, which calls for the resection of both the arachnoid membrane and dura mater in surgery. To prevent postoperative CSF leakage, it is necessary to suture the arachnoid membrane to the dura mater, creating an impermeable dural closure (6,7).
The decision tree (DT) is a classification algorithm known for its interpretability and applicability. Specifically, the classification and regression tree (CART) analysis utilizes binary recursive partitioning as a tree construction method (8). Being a non-parametric approach, CART offers greater flexibility compared to other statistical methods such as logistic regression (9). Consequently, the CART model is highly intuitive and straightforward, making it suitable for clinical settings where it can aid in radiological and clinical decision-making. Meanwhile, random forest (RF) is also an effective classifier, which can predict the class of input, and select the most important features by providing feature importance (10).
To the best of our knowledge, there has been no research conducted so far that explores the potential of a CART prediction model and an RF model in distinguishing between intraspinal schwannomas and meningiomas.
In order to address this problem, a retrospective study was conducted to establish a CART prediction model and an RF model that utilizes MRI features and simple clinical data to differentiate between the two neoplasms under consideration. In addition, we compared the predictive performance of two types of machine learning (ML) models to that of the radiologists. We present this article in accordance with the TRIPOD reporting checklist (available at https://qims.amegroups.com/article/view/10.21037/qims-23-1194/rc).
Methods
Patient data
The patient cohorts for this retrospective study were obtained from May 2012 to October 2022 at the First Affiliated Hospital of Jinan University. The study was conducted in accordance with the Declaration of Helsinki (as revised in 2013). The study was approved by the Ethics Committee of the First Affiliated Hospital of Jinan University, and individual consent for this retrospective analysis was waived. A meticulous screening was conducted on 160 patients who had undergone MRI examinations before the surgical resection. The study included two main criteria for patient selection: (I) patients with intraspinal spinal meningiomas or schwannomas that were confirmed through surgical resection and pathology. (II) patients who underwent multiparametric MR imaging, consisting of T1-weighted images (T1WI), T2-weighted images (T2WI), and contrast-enhanced T1WI sequences. A total of 21 patients were excluded from the study. The exclusion criteria were as follows: (I) patients with vertebral operation history before undergoing MRI examinations (n=4), (II) individuals with other types of intraspinal tumors such as neurofibromas, cavernous hemangiomas, or ependymomas (n=6), (III) patients with low MRI image quality that hindered accurate observations (n=6), and (IV) individuals who had incomplete MR scan sequences available (n=5).
Finally, a total of 139 patients (88 with schwannomas and 51 with meningiomas) met the inclusion criteria during the research period and were included as the cohort used to construct the CART model and RF model. Upon admission, the patients had an average age of 53 years, ranging from 19 to 86 years old. Histological examination of the surgical specimens in all cases revealed typical findings of intraspinal schwannomas and meningiomas.
MRI data
In total, 139 patients were examined using 1.5-Tesla MR scanners (Optima; GE Medical Systems, Milwaukee, WI, USA) or 3.0-Tesla MR scanners (Discovery MR750; GE Medical Systems, USA). The MRI scanning protocol includes T1-weighted fast spin echo (FSE) sagittal sequences [repetition time/echo time (TR/TE) =260/15.1 ms for 1.5T, TR/TE =598/8.9 ms for 3.0T, and number of excitation (NEX) =4] and FSE T2-weighted sequences (TR/TE =1,620/120 ms for 1.5T, TR/TE =2,000/102 ms for 3.0T). Following this, axial, sagittal, and coronal fat-suppressed T1WI were obtained for enhancement. The matrix range used for detection was from 288×192 to 384×224 pixels. The slice thickness for axial, sagittal, and coronal images was 4 mm with a 1 mm gap between slices. All individuals underwent intravenous administration of gadolinium-diethylenetriamine pentaacetic acid (Gd-DTPA), with a dosage of 0.1 mmol/kg of their respective body weight.
Two radiologists with three to 10 years of experience in spinal imaging diagnosis carefully examined all the images on the PACS workstation monitor. The two reviewers (radiologist 1: Y.L.W. and radiologist 2: X.R.C.) unanimously identified and provided detailed descriptions of the abnormal MRI findings. It is important to note that during the review process, both radiologists were unaware of any information regarding the patient’s age, sex, or histopathological results. Meanwhile, the two radiologists diagnosed every case based on the MRI images.
Clinical and MRI features
The identification of each intraspinal tumor in 139 patients was performed, and an evaluation was conducted on their location, maximum diameter, DTS, GLS, intervertebral foramen widening (IFW), signal intensity (SI) characteristics, and enhancement degree and pattern. The maximum diameter of each extramedullary tumor was measured on the contrast-enhanced images, and tumor location was classified as cervical region, thoracic region, or lumbar region.
The presence of the DTS was considered based on the following criteria: (I) the tail should be identified on two consecutive slices passing through the tumor, (II) the tail should smoothly taper away from the tumor, and (III) the tail must exhibit enhancement greater than the tumor itself (11).
The GLS was defined as follows: The appearance of the distorted cord and streak on enhanced axial MRI images bore a strong resemblance to that of a ginkgo leaf (12).
A comparison of SI between the lesion and the spinal cord was conducted, whereby the lesion was classified as either homogeneous or heterogeneous. The SI was further categorized based on the main part, accounting for over 80% of the lesion. On T1WI without enhancement, the SI of the tumor was divided into three groups, whereas on T2WI, it was divided into four groups. These groups included the following: (I) hypointense on T1WI, which is lower than that of the spinal cord but not equivalent to CSF. (II) Isointense on T1WI or T2WI, which is equivalent to that of the spinal cord. (III) Hyperintense on T2WI, which is higher than that of the spinal cord but not equivalent to CSF. (IV) Miscellaneous SI on T1WI or T2WI, which is representing different manifestations that do not belong to any of the above categories. (V) Fluid SI on T2WI, with SI equal to CSF, showing high intensity on T2WI (Figures 1,2).
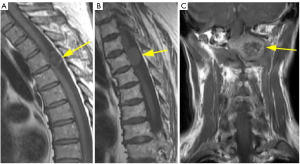
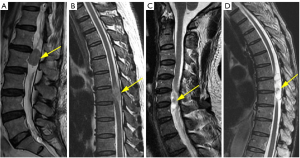
The degree of enhancement was defined as follows: strong, when the signal after the administration of Gd-DTPA is similar to that of subcutaneous fat (regardless of the enhancement pattern of the fat); moderate, when it is equivalent or lower to the signal of the vertebral body (Figure 3A,3B).
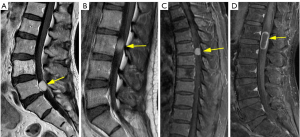
The pattern of enhancement was defined as follows: on the contrast-enhanced T1WI images, the contrast enhancement pattern is classified as diffuse when it appears homogeneous; it is classified as rim when there is non-uniform enhancement in the peripheral or central part (Figure 3C,3D).
Statistics and construction procedures of ML models
Categorical variables were presented as numbers and percentages, whereas continuous variables were described using median (interquartile range, IQR). The differences between the two groups of cases, schwannomas and meningiomas, for categorical variables, were analyzed using Pearson’s chi-squared test or Fisher’s exact test. For continuous variables, differences were analyzed using the non-parametric Wilcoxon rank sum test. Above statistics analysis was conducted by IBM SPSS Statistics 26.0 (IBM Corp., Armonk, NY, USA). A P value <0.05 was considered statistically significant. The data was randomly divided into a training set and a test set in a ratio of 6:4 in the CART and RF models, where the test set was used to examine the performance of the model. In the training group, the 5-fold stratified cross-validation was adopted for CART and RF model in order to more accurately estimate the generalization ability of the models.
This study utilized a comprehensive three-tiered approach to preprocess and sample the dataset in preparation for analysis. Firstly, feature mapping was carried out to ensure consistent representation across the dataset. Following this, z-score normalization was implemented to standardize the data, promoting comparability and analytical effectiveness. The final step involved the use of the synthetic minority over-sampling technique (SMOTE) to generate additional samples, addressing class imbalances in the dataset. Throughout this process, two kinds ML algorithms were employed: RF and CART algorithms, chosen for their robustness and proficiency in handling complex data structures, ultimately ensuring a thorough and reliable analysis.
The data analyses were conducted using Python version 3.7.12 (https://www.python.org/downloads/release/python-3712/) on the OnekeyAI platform version 3.1.8 (http://medai.icu/). The ML algorithms, including CART and RF, were implemented using scikit-learn version 1.0.2 (https://pypi.org/project/scikit-learn/1.0.2/). Scikit-learn uses an optimized version of the CART algorithm for the construction of the DT model. In this research, we aimed to explore the influence of hyperparameters on the CART and RF models. Our findings indicate that for CART, the most effective performance is achieved when the max_depth hyperparameter is set to 3. Meanwhile, for RF, optimal performance is attained when the n_estimators hyperparameter is set to 4 and the max_depth hyperparameter is set to 2. These results emphasize the significance of carefully selecting appropriate hyperparameters for ML models.
By utilizing CART analysis, a DT model was established in order to differentiate between intraspinal schwannomas and meningiomas. CART analysis was employed to identify the most optimal predictive variables and divide the data into two nodes with the highest level of purity. This iterative process was repeated for each child node until reaching the minimum size for terminal nodes or until further splitting no longer enhanced the purity of the terminal nodes (9). Response events for both CART and RF models were configured as “schwannoma”.
The predictive classification performance of the CART model and the RF model was assessed through the use of receiver operating characteristic (ROC) curve. The confusion matrix was adopted to describe the classification results of the CART and RF models. Furthermore, the accuracy, the area under the curve (AUC) and its 95% confidence interval (CI), sensitivity, specificity, positive predictive value (PPV), and negative predictive value (NPV) of the CART model, RF model, and two radiologists were also determined. Delong test was employed to compare the differences in diagnostic performance among the CART model, RF model, and two radiologists.
Results
Patient characteristics
The presented information in Table 1 provides a comprehensive overview of the patient demographics and MRI characteristics exhibited by both schwannomas and meningiomas.
Table 1
Characteristic | Training group (N=86) | Test group (N=53) | |||||||
---|---|---|---|---|---|---|---|---|---|
Overall (N=86) | Schwannoma (N=56) | Meningioma (N=30) | P value | Overall (N=53) | Schwannoma (N=32) | Meningioma (N=21) | P value | ||
Agea | 54 (42, 64) | 53 (38, 61) | 57 (47, 75) | 0.02 | 52 (42, 65) | 49 (39, 62) | 55 (44, 70) | 0.12 | |
Sexb | <0.001 | 0.002 | |||||||
Male | 41 (48) | 34 (61) | 7 (23) | 21 (40) | 18 (56) | 3 (14) | |||
Female | 45 (52) | 22 (39) | 23 (77) | 32 (60) | 14 (44) | 18 (86) | |||
Tumor location (C/T/L)b | 0.002 | <0.001 | |||||||
Cervical | 21 (24) | 14 (25) | 7 (23) | 13 (25) | 9 (28) | 4 (19) | |||
Thoracic | 38 (44) | 18 (32) | 20 (67) | 26 (49) | 9 (28) | 17 (81) | |||
Lumbar | 27 (31) | 24 (43) | 3 (10) | 14 (26) | 14 (44) | 0 (0) | |||
Max diameter (cm)a | 2.00 (1.53, 2.58) | 2.20 (1.60, 3.03) | 1.90 (1.50, 2.00) | 0.009 | 2.00 (1.60, 2.50) | 2.20 (1.60, 2.60) | 1. 80 (1.50, 2.00) | 0.12 | |
T1WI signal intensityc | 0.01 | 0.06 | |||||||
Isointense | 62 (72) | 35 (63) | 27 (90) | 36 (68) | 18 (56) | 18 (86) | |||
Hypointense | 23 (27) | 20 (36) | 3 (10) | 15 (28) | 12 (38) | 3 (14) | |||
Miscellaneous | 1 (1) | 1 (2) | 0 (0) | 2 (4) | 2 (6) | 0 (0) | |||
T2WI signal intensityb | <0.001 | 0.002 | |||||||
Isointense | 31 (36) | 12 (21) | 19 (63) | 18 (34) | 7 (22) | 11 (52) | |||
Hyperintense | 25 (29) | 16 (29) | 9 (30) | 21 (40) | 11 (34) | 10 (48) | |||
Miscellaneous | 8 (9) | 6 (11) | 2 (7) | 8 (15) | 8 (25) | 0 (0) | |||
Fluid signal | 22 (26) | 22 (39) | 0 (0) | 6 (11) | 6 (19) | 0 (0) | |||
Enhancement degreeb | 0.76 | >0.99 | |||||||
Strong | 73 (85) | 48 (86) | 25 (83) | 48 (91) | 29 (91) | 19 (90) | |||
Moderate | 13 (15) | 8 (14) | 5 (17) | 5 (9) | 3 (9) | 2 (10) | |||
Enhancement patternb | <0.001 | <0.001 | |||||||
Diffuse | 56 (65) | 28 (50) | 28 (93) | 36 (68) | 16 (50) | 20 (95) | |||
Rim | 30 (35) | 28 (50) | 2 (7) | 17 (32) | 16 (50) | 1 (5) | |||
Dural tail signb | <0.001 | 0.004 | |||||||
Yes | 12 (14) | 2 (4) | 10 (33) | 11 (21) | 2 (6) | 9 (43) | |||
No | 74 (86) | 54 (96) | 20 (67) | 42 (79) | 30 (94) | 12 (57) | |||
Ginkgo leaf signc | 0.04 | 0.39 | |||||||
Yes | 3 (3) | 0 (0) | 3 (10) | 1 (2) | 0 (0) | 1 (5) | |||
No | 83 (97) | 56 (100) | 27 (90) | 52 (98) | 32 (100) | 20 (95) | |||
Intervertebral foramen wideningb | 0.007 | 0.07 | |||||||
Yes | 12 (14) | 12 (21) | 0 (0) | 9 (17) | 8 (25) | 1 (5) | |||
No | 74 (86) | 44 (79) | 30 (100) | 44 (83) | 24 (75) | 20 (95) |
Data are expressed as n (%) or median (25th, 75th percentiles). a, Wilcoxon rank sum test; b, Pearson’s Chi-squared test; c, Fisher’s exact test. MRI, magnetic resonance imaging; T1WI, T1-weighted images; T2WI, T2-weighted images; C/T/L, cervical vertebra/thoracic vertebra/lumbar vertebra.
Age and sex
An analysis of age revealed that median ages differed slightly between the two groups, with schwannoma patients having a median age of 53 and 49 in the training and test groups, respectively, whereas meningioma patients had a median age of 57 and 55 in the two groups, respectively. Sex distribution showed statistically significant differences in the training and test groups (P<0.001 and P=0.002, respectively), with a higher importance of males in the Schwannoma group (61% and 56%) compared to females (39% and 44%), while the opposite was observed in the Meningioma group.
Tumor location and size
In terms of tumor location, the analysis revealed that meningiomas were predominantly found in the thoracic region both in the training and test groups (67% and 81% respectively), whereas schwannomas were more evenly distributed across cervical (25% and 28%) and lumbar (43% and 44%) regions. When considering tumor size, the median maximum diameter of the lesions was observed to be 1.90 and 1.80 cm for meningiomas in the training and test groups, respectively, and 2.20 cm in both the training and test groups for schwannomas. The analysis of size distribution revealed a significant difference between the two tumors in the training groups, with a P value of 0.009. However, the size of two tumors was not statistically significant in the test group.
T1WI and T2WI SI
Examination of the imaging features revealed that isointense signal was more common in meningiomas (90% and 86% in the training and test groups, respectively) compared to schwannomas (63% and 56%, respectively) based on T1WI, whereas hypointense signal was less common in meningiomas (10% and 14%, respectively) compared to schwannomas (36% and 38%, respectively). Similar trends were observed in T2WI, with meningiomas displaying a higher prevalence of isointense signal (63% and 52%, respectively) compared to schwannomas (21% and 22%, respectively).
Enhancement degree and pattern
Strong enhancement degree was the most common characteristic, observed in 73 (85%) and 48 (91%) of overall cases in the training and test cohorts, respectively, 86% and 91% of schwannoma cases, along with 83% and 90% of meningioma cases. Moderate enhancement degree was observed in 15% and 9% of overall cases among the training and test cohorts, respectively, 14% and 9% of Schwannoma cases, as well as 17% and 10% of meningioma cases. In our research, we found no significant statistical difference in the enhancement degree between schwannomas and meningiomas. However, enhancement pattern showed a significant difference between two tumors, with diffuse enhancement observed in 65% and 68% of overall cases in the training and test groups, respectively, 50% of schwannoma cases, but 93% and 95% of meningioma cases. In contrast, rim enhancement was seen in 35% and 32% of overall cases in the training and test groups, respectively, 50% of schwannoma cases, but only 7% and 5% of meningioma cases. The P value for the difference in enhancement pattern was found to be less than 0.001.
DTS and IFW and GLS
Notably, the DTS was more frequently observed in meningiomas (33% and 43% in the training and test groups, respectively) compared to schwannomas (4% and 6%, respectively) (Figure 4A), whereas the IFW was more prevalent in schwannomas (21% and 25% in the training and test cohorts, respectively) than meningiomas (only one case in the test group) (Figure 4B). The GLS was found in 3% and 2% of overall cases in the training and test groups, with no cases observed in the Schwannoma group but present in 10% and 5% of the Meningioma group respectively. The difference in the prevalence of the GLS between the Meningioma and Schwannoma groups was statistically significant (P=0.04) in the training group, whereas the GLS of two tumors was not statistically significant in the test group (P=0.39) (Figure 4C). These findings provide valuable insights into the baseline characteristics and imaging features of patients with schwannoma and meningioma, highlighting important differences between the two tumor types.
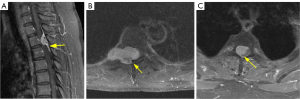
The performance of the CART model, RF model, and two radiologists
In the CART model, the feature importance plot showed that the most crucial predictor variable was enhancement pattern. The contribution of the top predictor variable is approximately 48.01%. The following list describes the second to fifth most important variables in this CART model (Figure 5A): The importance of IFW was approximately 23.99%. The importance of tumor location was approximately 16.35%. The importance of maximum diameter was approximately 8.91%. The importance of T2WI SI was approximately 2.74%. The remaining variables do not possess the same level of importance as the aforementioned five variables.
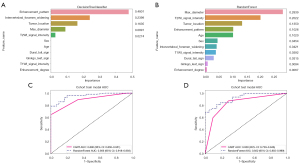
In the RF model, the top predictor variable was maximum diameter and its contribution was approximately 28.39%. The following list shows the second to 11th most important variables in this RF model (Figure 5B): the importance of T2WI SI was approximately 20.22%; that of tumor location was 13.50%; that of enhancement pattern was approximately 10.26%; that of age was approximately 10.20%; that of sex was 4.54%; that of IFW was approximately 4.21%; that of T1WI SI was approximately 3.92%; that of DTS was 3.15 %; that of GLS was approximately 0.94%; and that of enhancement degree was approximately 0.67%.
The AUC of the CART model, RF model, radiologist 1, and radiologist 2 was calculated to be 0.890, 0.956, 0.681, and 0.723 in the training group, and 0.838, 0.922, 0.580, and 0.659 in the test group, respectively. The corresponding CI of each AUC are displayed in Figure 5C,5D, and Table 2).
Table 2
Group | Signature | Accuracy | AUC | 95% CI | Sensitivity | Specificity | PPV | NPV |
---|---|---|---|---|---|---|---|---|
Training | CART | 0.814 | 0.89 | 0.8299–0.9510 | 0.893 | 0.667 | 0.833 | 0.769 |
Random forest | 0.86 | 0.956 | 0.9185–0.9934 | 0.839 | 0.9 | 0.94 | 0.75 | |
Radiologist 1 | 0.756 | 0.681 | 0.5846–0.7773 | 0.929 | 0.433 | 0.754 | 0.765 | |
Radiologist 2 | 0.791 | 0.723 | 0.6275–0.8189 | 0.946 | 0.5 | 0.779 | 0.833 | |
Test | CART | 0.811 | 0.838 | 0.7297–0.9459 | 0.875 | 0.714 | 0.824 | 0.789 |
Random forest | 0.811 | 0.922 | 0.8551–0.9887 | 0.812 | 0.81 | 0.867 | 0.739 | |
Radiologist 1 | 0.642 | 0.58 | 0.4655–0.6952 | 0.875 | 0.286 | 0.651 | 0.6 | |
Radiologist 2 | 0.717 | 0.659 | 0.5446–0.7739 | 0.937 | 0.381 | 0.698 | 0.8 |
CART, classification and regression tree; RF, random forest; AUC, area under the curve; CI, confidence interval; PPV, positive predictive value; NPV, negative predictive value.
Table 2 shows the diagnostic performance of the ML models and radiologists: (I) CART model (accuracy: 0.814, sensitivity: 0.893, specificity: 0.667, PPV: 0.833, NPV: 0.769), (II) RF model (accuracy: 0.860, sensitivity: 0.839, specificity: 0.900, PPV: 0.940, NPV: 0.750), (III) Radiologist 1 (accuracy: 0.756, sensitivity: 0.929, specificity: 0.433, PPV: 0.754, NPV: 0.765), (IV) Radiologist 2 (accuracy: 0.791, sensitivity: 0.946, specificity: 0.500, PPV: 0.779, NPV: 0.833), and (V) CART model (accuracy: 0.811, sensitivity: 0.875, specificity: 0.714, PPV: 0.824, NPV: 0.789), (VI) RF model (accuracy: 0.811, sensitivity: 0.812, specificity: 0.810, PPV: 0.867, NPV: 0.739), (VII) Radiologist 1 (accuracy: 0.642, sensitivity: 0.875, specificity: 0.286, PPV: 0.651, NPV: 0.600), and (VIII) Radiologist 2 (accuracy: 0.717, sensitivity: 0.937, specificity: 0.381, PPV: 0.698, NPV: 0.800). (I–IV: training group, V–VIII: test group).
According to the Delong test, the comparison of AUCs between the RF model and the two radiologists showed significant differences in the training and test groups (Delong test, all P<0.001). There were also significant differences in the comparison of AUCs between CART model and the two radiologists in the training and test groups (Delong test, training group: P<0.001, CART vs. Radiologist 1; P=0.004, CART vs. Radiologist 2; test group: P=0.006, CART vs. Radiologist 1; P=0.02, CART vs. Radiologist 2). The comparison of AUCs between the RF model and CART model showed significant differences in the training group (Delong test, P=0.002). There were no statistically significant differences in the comparison of AUCs between the CART model and RF model in the test group (Delong test, P=0.09). There was also no significant difference in the diagnostic performance between the two radiologists in the training and test groups (Delong test, P=0.46 and P=0.29, respectively) (Figure 6A,6B).
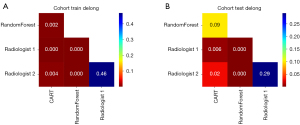
Figure 7 depicts examples of correctly predicted and incorrectly predicted outcomes by the CART model, RF model, and radiologists. The confusion matrix illustrates the classification results of the CART and RF models (Figure S1).
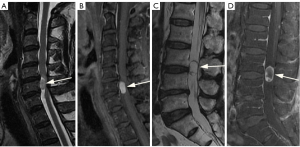
The result of the 5-fold stratified cross-validation in the training group is shown in the Figure S2.
Discussion
The differentiation between preoperative schwannomas and meningiomas is of utmost importance in determining the appropriate surgical treatment approach. Our study developed and validated a novel CART prediction model and RF model for differentiating schwannomas and meningiomas. To the best of our knowledge, these two models have never been used to distinguish between these two types of tumors. Having sufficient clinical and imaging information is crucial for accurately differentiating between schwannomas and meningiomas. The statistical importance of MRI findings and clinical information was investigated using the CART and RF models. The CART model was developed by combining five variables, whereas the RF model was constructed by integrating all 11 variables. These features were found to be informative for differential diagnosis in the two ML models. The CART model demonstrated a predictive ability of 0.890 in the training group and 0.838 in the test group, whereas the RF model showed a higher discriminative ability with AUCs of 0.956 and 0.922 in the training and test groups, respectively. Meanwhile, the Delong test indicated that the RF and CART models both performed better than the two radiologists, suggesting that the RF and CART models could be used in this diagnostic analysis, and the RF model can be regarded as a more reliable and effective classifier for distinguishing between the two types of entities.
Previous studies have suggested that more than 95% of meningiomas are benign tumors and are most frequently observed in elderly women (4). Furthermore, the advantage of women in terms of intraspinal meningioma occurrence is even greater than the advantage observed specifically for intracranial meningiomas. This phenomenon is believed to be influenced by estrogen (13,14). Our research findings are partially consistent with previous studies. The median age of patients diagnosed with spinal meningiomas was higher compared to that of those with schwannomas. Additionally, it is worth noting that a majority importance (77% and 86% in the training and test groups, respectively) of patients diagnosed with intraspinal meningiomas are female.
Regarding the location of two types of tumors, most spinal meningiomas are found in the region of thoracic vertebra, whereas a smaller portion occur in the region of cervical and lumbar vertebrae (5). On the contrary, spinal schwannomas are solitary, well-defined, and encapsulated tumors that typically originate from the posterior sensory roots of the region of cervical and lumbar vertebra. There are fewer cases involving the thoracic vertebra, and these tumors rarely occur in the sacral region (13,15). The results of our research align with prior studies, demonstrating that a significant importance of spinal meningiomas (67% and 81% in the training and test groups, severally) are localized in the thoracic region, whereas the majority of schwannomas (43% and 44%, respectively) manifest in the lumbar region. It is reported that the size of schwannomas was larger than that of meningiomas, and significant differences were observed between the two groups (2). Our research produced similar results in the training group, whereas the results of the test group were not statistically significant, probably due to the limited sample size. Based on the DT model, tumor location and maximum diameter (representing size) held the third and fourth positions. As a result, these two variables contributed significantly to the model. For the RF model, maximum diameter was the top predictor variable, and tumor location was the third most important variable in the model. The results indicate that the maximum diameter and tumor location are both important predictors in differentiating the intraspinal schwannomas and meningiomas.
Friedman et al. (16) have documented that schwannomas exhibit comparable or diminished SI relative to the spinal cord in T1WI images. Conversely, T2WI images generally demonstrate a spectrum of SI ranging from slight to significantly high intensity signals. Areas displaying hyperintensity on T2WI images commonly correspond to cystic regions, whereas lower intensity may suggest the presence of collagen deposition, hemorrhage, or densely cellularity (17). In terms of the degree of tumor enhancement, studies conducted by Zhai et al. and Schroth et al. have suggested that there is no significant statistical difference in contrast enhancement degree between intraspinal schwannomas and meningiomas (2,18). Our findings support the above viewpoints, as we observed no statistically significant difference in the degree of enhancement (P=0.76 and P>0.99 in the training and test cohorts, severally). However, the utilization of the enhancement pattern in our study yielded significant differences between meningiomas and schwannomas, thereby offering potential assistance in the differentiation of diagnoses (P<0.001). The feature importance plot in the CART model suggests that the T2WI SI variable holds a importance at 2.74%, whereas the T1WI variable is not included in the model. It is worth noting that the feature importance plot suggests that the enhancement degree is not incorporated in the model; however, the enhancement pattern is the most important variable in the construction of the model, with an importance of 48.01%. For the RF model, the T2WI SI and enhancement pattern accounted for 20.22% and 10.26%, respectively, whereas the T1WI SI and enhancement degree were of low importance in the model. The results demonstrate that the T2WI SI and enhancement pattern were two important variables in distinguishing the two tumors.
In a previous study conducted in 1989, researchers initially described a phenomenon referred to as the “dural tail sign”. This sign was exclusively described on contrast-enhanced MRI images in individuals with cranial meningiomas (19). Initially, Alorainy et al. (11) held the belief that the DTS exhibited high specificity towards meningiomas. However, Guermazi et al. (20-22) currently posit that this phenomenon merely suggests the presence of meningiomas, lacking specificity exclusively towards them. Previous studies have reported that various conditions such as sarcoidosis, lymphoma, loculated leptomeningeal metastasis with adjacent meningeal enhancement, and other diseases could mimic meningiomas, presenting DTS. This similar imaging feature can also be observed in cases of any type of histiocytic or lymphocytic infiltration as well as granulomatosis (23). De Verdelhan et al. (24) found that a DTS was present in 67% of meningeal tumors, rare extradural meningiomas, and recurrent meningeal tumors. Similarly, Alorainy et al. demonstrated that the DTS is equally common in spinal meningiomas and cranial meningiomas. In our current study, we observed a DTS in 10 out of 30 meningiomas in the training group (P<0.001), and nine out of 21 in the test group (P=0.004), whereas only four in all schwannoma cases. However, this finding is not entirely consistent with the study conducted by Zhai et al. (2), as they reported a DTS in 60.4% of meningioma cases. We speculate that this difference may be attributed to the limited sample size of spinal meningiomas in our study. Due to the fact that DTS can only be observed in the sagittal or coronal enhanced scans of T1WI, and its importance in relation to other variables is relatively low, it makes little contribution to the CART and RF model. Therefore, the CART model excluded the DTS as a predictor, and the importance of DTS is also of low importance in the RF model.
When discussing the IFW, Iwanami et al. (25) found that the presence of lesions accompanied by IFW or a dumbbell shape is highly useful for differential diagnosis. In our study, we encountered 12 cases (21%) of schwannomas with IFW in the training cohort (P=0.007), whereas the results of test group were not statistically significant (P=0.07), possibly because of the sample size limitations. However, meningiomas with IFW are relatively rare, accounting for only 3–10% of all spinal meningiomas (26). Similarly, in our study, we observed only one case of meningioma with IFW in the test cohort, which is consistent with these findings. The RF model included the IFW as a predictor with an importance of 4.21%. Meanwhile, IFW was found to have an approximate variable importance of 23.99% in the CART model. Therefore, it was included in the construction of the CART model, highlighting the significance of IFW in the context of differential diagnosis.
According to the findings of Yamaguchi et al. (12), it was determined that the presence of the GLS exhibited a high degree of specificity in identifying spinal meningiomas that originate laterally or ventrolaterally to the spinal cord. The expansion of tumors leads to the deformation of the spinal cord resembling a fan shape, as the denticulate ligaments, which are stretched, connect the outer side of the spinal cord to the dura mater. Schwannomas do not exhibit the GLS, possibly due to differences in their growth pattern (12). In our study, the GLS was only observed in four cases of meningiomas. Statistically significant differences exist between the schwannomas and meningiomas in the training group with regards to the GLS (P=0.04). However, there was no statistically significant differences in the test group between two tumors because of the sample size limitations (P=0.39). Meanwhile, the importance of GLS in the CART and RF model is relatively low. Therefore, the variable contributes little to the two models.
In this study, we established a CART model and an RF model integrating the clinical data with MRI findings, achieved the AUCs of 0.890, 0.956, and 0.838, 0.922 in the training and test cohorts, respectively. Furthermore, we also compared the performance of the ML models to the radiologists. Previous studies have shown that the RF model showed higher differential ability than the CART model in many diseases (27). Our study displayed a similar tendency in distinguishing the schwannomas and meningiomas. The results demonstrate that the RF model seems to be a better model than CART model for differentiating the schwannomas from meningiomas. The diagnostic abilities of the RF and CART models were better than those of experienced clinicians. The performance of the two radiologists indicates that clinicians achieved high sensitivity and relatively low specificity compared to the ML models, which means that they tended to misdiagnose the meningiomas as schwannomas. The results are consistent with previous research (28). The reason for this misdiagnosis may be that radiologists tend to misdiagnose difficult cases as schwannomas in terms of perspective of tumor incidence. A previous study reported that the accuracy of plain MRI findings in distinguishing the spinal schwannomas from meningiomas was 91.3% (29). Maki et al. suggested that the deep convolutional neural network based on MRI images could differentiate the two tumors, with an AUC of 0.876 (28). Ito et al. proposed that a deep learning model based on MRI could differentiate the two tumors with accuracy of 82.1% in the validation group (30). However, the sample sizes in these studies were relatively small which may have resulted in overfitting and overly optimistic estimates (31). Lee et al. built a logistic regression model based on MRI findings to differentiate between schwannomas and meningiomas with a sensitivity, specificity, and accuracy of 89.8%, 97.1%, and 93.5% respectively (32). Nevertheless, the limited variables included in the study above, as compared to ours, may have an impact on the accuracy of the logistic regression model (33).
Limitations
There were several limitations in our study. Firstly, the study was retrospective and conducted by experts in differentiating between schwannomas and meningiomas. In addition, the limited MRI sequences may influence the performance of the model. Our future study will employ more sequences, such as diffusion-weighted imaging (DWI) and arterial spin labeling (ASL) to improve the diagnostic ability of the model. Meanwhile, it did not include other intradural extramedullary tumors such as neurofibromas, capillary hemangiomas, or cysts, which could potentially impact the sensitivity of MRI imaging for distinguishing between the two types of lesions. In order to address these limitations, our future objective is to conduct a similar prospective study with a larger cohort. Finally, our forthcoming research proposal intends to employ radiomics for the purpose of extracting imaging characteristics of both tumor classifications, with the objective of discerning imperceptible dissimilarities that could facilitate their distinction.
Conclusions
Our investigation integrates conventional MRI imaging characteristics with basic clinical data to construct two ML models named CART and RF. The RF model exhibited more exceptional efficacy than the CART model and experienced clinician in discriminating between the two categories of tumors, suggesting that the RF model could be considered a reliable and effective tool for improving clinical decision-making and prognosis.
Acknowledgments
Funding: This work was supported by
Footnote
Reporting Checklist: The authors have completed the TRIPOD reporting checklist. Available at https://qims.amegroups.com/article/view/10.21037/qims-23-1194/rc
Conflicts of Interest: All authors have completed the ICMJE uniform disclosure form (available at https://qims.amegroups.com/article/view/10.21037/qims-23-1194/coif). The authors have no conflicts of interest to declare.
Ethical Statement: The authors are accountable for all aspects of the work in ensuring that questions related to the accuracy or integrity of any part of the work are appropriately investigated and resolved. The study was conducted in accordance with the Declaration of Helsinki (as revised in 2013). The study was approved by the Ethics Committee of the First Affiliated Hospital of Jinan University, and individual consent for this retrospective analysis was waived.
Open Access Statement: This is an Open Access article distributed in accordance with the Creative Commons Attribution-NonCommercial-NoDerivs 4.0 International License (CC BY-NC-ND 4.0), which permits the non-commercial replication and distribution of the article with the strict proviso that no changes or edits are made and the original work is properly cited (including links to both the formal publication through the relevant DOI and the license). See: https://creativecommons.org/licenses/by-nc-nd/4.0/.
References
- Hung ND, Dung LT, Huyen DK, Duy NQ, He DV, Duc NM. The value of quantitative magnetic resonance imaging signal intensity in distinguishing between spinal meningiomas and schwannomas. Int J Med Sci 2022;19:1110-7. [Crossref] [PubMed]
- Zhai X, Zhou M, Chen H, Tang Q, Cui Z, Yao Y, Yin Q. Differentiation between intraspinal schwannoma and meningioma by MR characteristics and clinic features. Radiol Med 2019;124:510-21. [Crossref] [PubMed]
- Takashima H, Takebayashi T, Yoshimoto M, Onodera M, Terashima Y, Iesato N, Tanimoto K, Ogon I, Morita T, Yamashita T. Differentiating spinal intradural-extramedullary schwannoma from meningioma using MRI T(2) weighted images. Br J Radiol 2018;91:20180262. [Crossref] [PubMed]
- Nakamizo A, Suzuki SO, Shimogawa T, Amano T, Mizoguchi M, Yoshimoto K, Sasaki T. Concurrent spinal nerve root schwannoma and meningioma mimicking single-component schwannoma. Neuropathology 2012;32:190-5. [Crossref] [PubMed]
- Liu WC, Choi G, Lee SH, Han H, Lee JY, Jeon YH, Park HS, Park JY, Paeng SS. Radiological findings of spinal schwannomas and meningiomas: focus on discrimination of two disease entities. Eur Radiol 2009;19:2707-15. [Crossref] [PubMed]
- Nakamura M, Tsuji O, Fujiyoshi K, Hosogane N, Watanabe K, Tsuji T, Ishii K, Toyama Y, Chiba K, Matsumoto M. Long-term surgical outcomes of spinal meningiomas. Spine (Phila Pa 1976) 2012;37:E617-23. [Crossref] [PubMed]
- Ahn DK, Park HS, Choi DJ, Kim KS, Kim TW, Park SY. The surgical treatment for spinal intradural extramedullary tumors. Clin Orthop Surg 2009;1:165-72. [Crossref] [PubMed]
- Breiman L, Friedman J, Olshen RA, Stone CJ. Classification and regression trees. New York: Routledge, 2017.
- Lewis RJ, editor. An introduction to classification and regression tree (CART) analysis. Annual meeting of the society for academic emergency medicine in San Francisco, California, 2000.
- Breiman L. Random forests. Mach Learn 2001;45:5-32.
- Alorainy IA. Dural tail sign in spinal meningiomas. Eur J Radiol 2006;60:387-91. [Crossref] [PubMed]
- Yamaguchi S, Takeda M, Takahashi T, Yamahata H, Mitsuhara T, Niiro T, Hanakita J, Hida K, Arita K, Kurisu K. Ginkgo leaf sign: a highly predictive imaging feature of spinal meningioma. J Neurosurg Spine 2015;23:642-6. [Crossref] [PubMed]
- Wiemels J, Wrensch M, Claus EB. Epidemiology and etiology of meningioma. J Neurooncol 2010;99:307-14. [Crossref] [PubMed]
- Michaud DS, Gallo V, Schlehofer B, Tjønneland A, Olsen A, Overvad K, et al. Reproductive factors and exogenous hormone use in relation to risk of glioma and meningioma in a large European cohort study. Cancer Epidemiol Biomarkers Prev 2010;19:2562-9. [Crossref] [PubMed]
- Gu R, Liu JB, Zhang Q, Liu GY, Zhu QS. MRI diagnosis of intradural extramedullary tumors. J Cancer Res Ther 2014;10:927-31. [Crossref] [PubMed]
- Friedman DP, Tartaglino LM, Flanders AE. Intradural schwannomas of the spine: MR findings with emphasis on contrast-enhancement characteristics. AJR Am J Roentgenol 1992;158:1347-50. [Crossref] [PubMed]
- Demachi H, Takashima T, Kadoya M, Suzuki M, Konishi H, Tomita K, Yonezawa K, Ubukata A. MR imaging of spinal neurinomas with pathological correlation. J Comput Assist Tomogr 1990;14:250-4. [Crossref] [PubMed]
- Schroth G, Thron A, Guhl L, Voigt K, Niendorf HP, Garces LR. Magnetic resonance imaging of spinal meningiomas and neurinomas. Improvement of imaging by paramagnetic contrast enhancement. J Neurosurg 1987;66:695-700. [Crossref] [PubMed]
- Wilms G, Lammens M, Marchal G, Van Calenbergh F, Plets C, Van Fraeyenhoven L, Baert AL. Thickening of dura surrounding meningiomas: MR features. J Comput Assist Tomogr 1989;13:763-8. [Crossref] [PubMed]
- Guermazi A, Lafitte F, Miaux Y, Adem C, Bonneville JF, Chiras J. The dural tail sign--beyond meningioma. Clin Radiol 2005;60:171-88. [Crossref] [PubMed]
- Gupta S, Gupta RK, Banerjee D, Gujral RB. Problems with the "dural tail" sign. Neuroradiology 1993;35:541-2. [Crossref] [PubMed]
- Bourekas EC, Wildenhain P, Lewin JS, Tarr RW, Dastur KJ, Raji MR, Lanzieri CF. The dural tail sign revisited. AJNR Am J Neuroradiol 1995;16:1514-6.
- Tien RD, Yang PJ, Chu PK. "Dural tail sign": a specific MR sign for meningioma? J Comput Assist Tomogr 1991;15:64-6.
- De Verdelhan O, Haegelen C, Carsin-Nicol B, Riffaud L, Amlashi SF, Brassier G, Carsin M, Morandi X. MR imaging features of spinal schwannomas and meningiomas. J Neuroradiol 2005;32:42-9. [Crossref] [PubMed]
- Iwanami A, Kobayashi Y, Takano M, Mikami S, Toyama Y, Nakamura M. Invasive dumbbell spinal meningiomas: report of four cases and a review of the literature. J Orthop Sci 2015;20:1148-54. [Crossref] [PubMed]
- Ozaki M, Nakamura M, Tsuji O, Iwanami A, Toyama Y, Chiba K, Matsumoto M. A rare case of dumbbell meningioma of the upper cervical spinal cord. J Orthop Sci 2013;18:1042-5. [Crossref] [PubMed]
- Mao Y, He Y, Liu L, Chen X. Disease Classification Based on Eye Movement Features With Decision Tree and Random Forest. Front Neurosci 2020;14:798. [Crossref] [PubMed]
- Maki S, Furuya T, Horikoshi T, Yokota H, Mori Y, Ota J, Kawasaki Y, Miyamoto T, Norimoto M, Okimatsu S, Shiga Y, Inage K, Orita S, Takahashi H, Suyari H, Uno T, Ohtori S. A Deep Convolutional Neural Network With Performance Comparable to Radiologists for Differentiating Between Spinal Schwannoma and Meningioma. Spine (Phila Pa 1976) 2020;45:694-700. [Crossref] [PubMed]
- Iwata E, Shigematsu H, Yamamoto Y, Kawasaki S, Tanaka M, Okuda A, Morimoto Y, Masuda K, Koizumi M, Akahane M, Tanaka Y. Preliminary algorithm for differential diagnosis between spinal meningioma and schwannoma using plain magnetic resonance imaging. J Orthop Sci 2018;23:408-13. [Crossref] [PubMed]
- Ito S, Nakashima H, Segi N, Ouchida J, Oda M, Yamauchi I, Oishi R, Miyairi Y, Mori K, Imagama S. Automated Detection and Diagnosis of Spinal Schwannomas and Meningiomas Using Deep Learning and Magnetic Resonance Imaging. J Clin Med 2023;12:5075. [Crossref] [PubMed]
- Jonassaint CR, Kang C, Prussien KV, Yarboi J, Sanger MS, Wilson JD, De Castro L, Shah N, Sarkar U. Feasibility of implementing mobile technology-delivered mental health treatment in routine adult sickle cell disease care. Transl Behav Med 2020;10:58-67. [Crossref] [PubMed]
- Lee JH, Kim HS, Yoon YC, Cha MJ, Lee SH, Kim ES. Differentiating between spinal schwannomas and meningiomas using MRI: A focus on cystic change. PLoS One 2020;15:e0233623. [Crossref] [PubMed]
- Yang L, Li S, Liu X, Liu J, Zheng F, Deng W, Liu W, Fu B, Xiong J. A nomogram model for determining optimal patients for local therapy in metastatic prostate cancer: a SEER database-based study. BMC Urol 2023;23:12. [Crossref] [PubMed]