A nomogram based on neuron-specific enolase and substantia nigra hyperechogenicity for identifying cognitive impairment in Parkinson’s disease
Introduction
Parkinson’s disease (PD) is the second most prevalent neurodegenerative disease worldwide, and its global burden has more than doubled over the past two decades (1,2). Both motor and nonmotor symptoms (NMSs) are clinical manifestations of PD, with cognitive impairment (CI) being one of the most common and devastating NMSs, influencing approximately 25–30% of the PD population (3). One study indicated that almost half of the patients with PD and normal cognition will develop CI within 6 years, and all newly diagnosed mild CI cases will progress to dementia within 5 years (4). CI not only severely impairs functioning and reduces quality of life but also decreases life expectancy while increasing mortality rates (5), imposing a tremendous burden on families and public healthcare systems. In 2020, the Lancet Commission reported that over 40% of dementia cases worldwide could be theoretically prevented or delayed, especially in low-income and middle-income countries (6). Given the heterogeneous nature of the mechanisms underlying CI in PD, different biomarker patterns suggest unique yet interconnected alternations in the brain. Therefore, identifying and integrating several types of markers to create different models for accurately predicting individuals at higher risk of early or rapid cognitive deterioration is important for prognosis and effective patient management.
Several cerebrospinal fluid (CSF) biomarkers, such as Aβ42, total tau, p-tau, and α-synuclein, have been studied to determine their association with CI in PD (7). Nevertheless, clinically administering a lumbar puncture to retrieve a CSF sample can be uncomfortable for certain patients. Although neuron-specific enolase (NSE), a glycolytic enzyme found in neuronal and neuroendocrine tissues, is not biologically released, its elevated level in the circulation can indicate neuronal damage. A meta-analysis revealed that CSF NSE levels are significantly higher in patients with Alzheimer disease (AD), PD with dementia (PDD), and dementia with Lewy bodies but not in those with PD without dementia or multiple system atrophy (8).
The use of structural, functional, and molecular neuroimaging has significantly enhanced researchers’ understanding of clinical features and pathological processes (9-11). Nevertheless, studies exploring the pathological link between the substantia nigra (SN) and CI in PD have yielded conflicting results (12). One study found that iron deposition in the SN assessed via quantitative susceptibility mapping (QSM) increased as early as the prodromal stage of PD but was not significantly associated with Montreal Cognitive Assessment (MoCA) scores (13). Another study discovered that the susceptibility value of the bilateral hippocampus and SN might predict CI in patients with chronic cerebral hypoperfusion (14). However, functional magnetic resonance imaging (MRI) is not a routine clinical examination; in contrast, transcranial sonography (TCS) is an economical and convenient method with a high sensitivity in detecting SN hyperechogenicity (SNH) (15). In our previous study, SN grading and certain clinical characteristics demonstrated good predictive potential for CI in PD (16). Therefore, we conducted this subsequent study to investigate the correlation between circulating NSE levels, SNH, and cognitive function in PD. We also sought to develop a nomogram based on clinical and neuroimaging biomarkers to predict CI in patients with PD. We present this article in accordance with the TRIPOD reporting checklist (available at https://qims.amegroups.com/article/view/10.21037/qims-23-1778/rc).
Methods
Patient selection and study design
This cross-sectional study was conducted in accordance with the Declaration of Helsinki (as revised in 2013) and was approved by the Ethics Board of Beijing Tiantan Hospital, Capital Medical University (No. KT2022-015-04). Informed consent was obtained from all the patients. Patients who were treated in the Department of Dyskinesia and who underwent TCS examinations from January 2021 to December 2022 were selected as the training set. A separate test cohort was recruited from patients who attended the same medical center from January 2023 to December 2023 to validate the reliability of the prediction model. The allocation ratio of the training set and test set was 7:3. Eligible participants were individuals with PD aged 18 years and above, with complete clinical data and a TCS result. The exclusion criteria were the following: Parkinson syndrome caused by cerebrovascular disease, craniocerebral tumors, trauma, or medications; insufficient clinical history; ambiguous SN results from TCS examination; and patients with implanted deep brain stimulation (DBS) electrodes, stem cell therapy, or pallidotomy. The diagnosis of PD adhered to the criteria set by the International Parkinson and Movement Disorder Society (17).
Cognitive and behavioral assessments
Two neurologists, each with more than 5 years of experience in handling patients with movement disorders, conducted neurological examinations on the participants and evaluated them using the Movement Disorder Society Unified Parkinson’s Disease Rating Scale (MDS-UPDRS). If a consistent diagnosis could not be reached, the final diagnosis would be made by a senior expert with over 10 years of experience in managing movement disorders. Prior to the clinical assessments, the patients refrained from antiparkinsonian medications for at least 12 hours. All patients underwent neuropsychological tests via the MoCA (18) and the Mini-Mental State Examination (MMSE) (19). Global cognitive function was evaluated using MoCA according to the critiques and recommendations set by the International Parkinson and Movement Disorders Society (18). The overall MoCA score ranges from 0 to 30, with a value below 26 indicating CI (20). The participants were then classified into two groups: PD patients with normal cognition (PD-NCI) and PD patients with CI (PD-CI).
Clinical data collection
Demographic and clinical data, including gender, age, disease duration, education level, body mass index, and smoking and drinking habits, in addition to the medical history of hypertension, diabetes mellitus (DM), and hyperlipemia, were collected. A fasting venous blood sample of 5 ml was collected in the morning, and the serum was immediately centrifuged, preserved at –80 ℃, and analyzed using an immunoturbidimetric assay. Ferritin, folate, and vitamin B12 (VitB12) levels were measured using a chemiluminescent assay (Cobas E601, Roche Diagnostics, USA), and homocysteine (Hcy) levels were determined through a cyclase assay with an electrochemiluminescence immunoassay analyzer (AU 5800, Roche Diagnostics). The plasma concentration of NSE was determined using the Elecsys NSE assay (Roche Diagnostics).
TCS examination
Two sonographers with at least 5 years of expertise in neuroimaging performed the ultrasound examination. Hyperechoic areas of the SN were identified and measured by two experienced sonographers, each with over 10 years of experience in neuroimaging, who were blinded to the patient’s clinical diagnosis. The ultrasound examination was conducted with an ultrasound machine (Aplio i900, Canon, Japan) equipped with an I6SX1 phased array probe (2.0–3.0 MHz) under a scanning setting of 14–16 cm of depth and a dynamic range of 45–55 dB. Image brightness and gain compensation were adjusted according to the patient’s condition.
The patient was placed in the supine position, with the temporal window fully exposed. The probe was placed close to the temporal window on one side, and the different axial sections of the midbrain were explored via slight adjustments of the angle of the sound beam in the direction of the auricular frame line (the line connecting the tip of the ear and the corner of the eye). A butterfly-shaped midbrain is located near the cerebral midline, and its peripheral and internal nuclei could be observed in the presence of a good bone window. The area of SNH was calculated if there was hyperechogenicity observed on the left or right sides of the SN. The echogenicity of the SN was graded as described by Bartova et al. (21) (Figure 1). Interclass correlation coefficients (ICCs) were employed to test interobserver agreement for SN grading and the SNH area calculation.
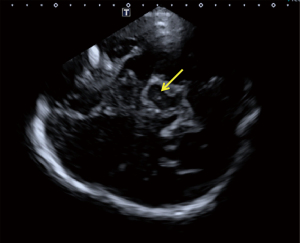
Statistical analysis
The measurement data are reported as the mean±standard deviation (SD) or median with interquartile range (IQR), whereas discrete variables are expressed as percentages. Differences between groups were compared using unpaired independent t-tests for continuous variables with normal distributions, the nonparametric Mann-Whitney test for continuous variables without normal distribution, and the chi-squared test for discrete variables. Individual missing values were replaced with the average of adjacent values rather than deleted. Spearman correlation analysis and partial correlation analysis were performed to detect the correlation between NSE, area of SNH, and demographic data. Univariate logistic regression analysis was used to identify potential risk factors for CI. Variables with significance (P<0.1) in the univariate analysis were incorporated into the multivariable model. The final prediction model was visualized with a nomogram for predicting CI. The model’s discriminative ability was assessed using the concordance index (C-index) or area under the curve (AUC) of the receiver operating characteristic (ROC) curve, while the model’s calibration was evaluated with a calibration curve. P<0.05 was considered statistically significant. All statistical analyses were performed using SPSS version 25.0 (IBM Corp., USA) or RStudio (version 4.3.2).
Results
Demographics and clinical characteristics
The demographic and clinical data of the training and test cohorts are summarized in Table 1. The training set consisted of 385 patients with an average age of 63.33±9.69 years, 246 of whom were males. The mean disease duration in this set was 6.0±4.83 years. The test set comprised 165 patients with an average age of 64.68±8.83 years, 99 of whom were male. The patient selection process is presented in Figure S1A,S1B. The mean MoCA score was 23±5.85 in the training set and 21.47±5.38 in the test set. Apart from the MoCA score, status of dementia, and history of hyperlipemia, there were no statistically significant differences in the characteristics between the two sets. More details can be found in Table S1.
Table 1
Parameter | Training group | Test group | |||||
---|---|---|---|---|---|---|---|
PD-CI (n=204) | PD-NCI (n=181) | P value | PD-CI (n=115) | PD-NCI (n=50) | P value | ||
Male gender (%) | 140 (68.6) | 106 (58.6) | 0.04a | 75 (65.2) | 24 (48.0) | 0.03a | |
Age (years) | 64.85±9.15 | 61.61±10.02 | 0.001b | 65.86±8.87 | 61.96±8.17 | 0.009b | |
BMI (kg/m2) | 24.12±3.83 | 24.28±3.26 | 0.67b | 21.43±3.61 | 23.87±3.46 | 0.35b | |
Duration of disease (years) | 6.33±4.84 | 5.63±4.81 | 0.15b | 6.76±4.06 | 6.78±3.62 | 0.97b | |
Education level (years) | 9 (0, 19) | 12 (0, 21) | <0.001c | 9 (0,22) | 12 (6,17) | <0.001c | |
Smoking, n (%) | 52 (25.5) | 34 (18.8) | 0.11a | 22 (19.1) | 9 (18.0) | 0.86a | |
Drinking, n (%) | 47 (23.0) | 32 (17.7) | 0.19a | 22 (19.1) | 8 (16.0) | 0.63a | |
Hypertension, n (%) | 70 (34.3) | 53 (59.3) | 0.29a | 49 (42.6) | 17 (34.0) | 0.30a | |
Diabetes mellitus, n (%) | 34 (16.7) | 24 (13.3) | 0.35a | 19 (16.5) | 6 (12.0) | 0.45a | |
Hyperlipidemia, n (%) | 39 (19.1) | 18 (9.9) | 0.01a | 27 (23.5) | 9 (18.0) | 0.43a | |
H-Y stage | 2.81±1.00d | 2.47±0.86 | <0.001b | 2.93±0.82 | 2.56±0.93d | 0.01b | |
MoCA score | 18.50±5.16 | 27.30±1.34 | <0.001b | 19.05±4.59 | 27.025±1.61 | <0.001b | |
PDD, n (%) | 97 (47.5) | 0 | <0.001a | 57 (49.6) | 0 | <0.001a | |
UPDRS-III | 41.70±16.79 | 33.51±16.02 | <0.001b | 42.21±16.94e | 35.19±13.88 | 0.01b | |
Hcy (μmol/L) | 16.59±8.62 | 14.70±7.88 | 0.02b | 17.02±9.52 | 13.93±4.65 | 0.03b | |
Folate (ng/mL) | 7.67±5.67 | 8.11±4.54 | 0.40b | 8.75±6.49 | 7.32±4.17f | 0.15b | |
Vitamin B12 (pg/mL) | 470.38±103.15 | 512.38±306.47 | 0.30b | 490.93±376.15 | 487.14±331.92g | 0.95b | |
Ferritin (ng/mL) | 124.78±119.02 | 116.93±103.15 | 0.53b | 137.42±100.51 | 108.17±79.5h | 0.72b | |
NSE (ng/mL) | 16.38±3.66 | 13.96±2.07 | <0.001b | 16.22±3.65 | 13.19±2.57 | <0.001b | |
SNH, n (%) | 139 (68.1) | 91 (50.3) | <0.001a | 83 (72.2) | 27 (54.0) | 0.02a | |
Area of SNH (cm2) | 0.32 (0, 1.11) | 0 (0,1.03) | 0.001c | 0.19 (0,0.83) | 0.32 (0,0.85) | 0.09c |
Education level and area of SNH are presented as the median (interquartile range); gender, smoking, drinking, hypertension, diabetes mellitus, hyperlipidemia, PDD, and SNH are presented as number (frequency). The other parameters are presented as the mean ± standard deviation. a, χ2 test; b, unpaired independent t-test; c, nonparametric Mann-Whitney test; d, missing value: H-Y stage, training group (PD-CI) 1 and test group (PD-NCI) 1; e, missing value: UPDRS-III, test group (PD-CI) 1; f, missing value: folate, test group (PD-NCI) 1; g, missing value: vitamin B12, test group (PD-NCI) 1; h, missing value: ferritin, test group (PD-NCI) 1. PD-CI, Parkinson’s disease with cognitive impairment; PD-NCI, Parkinson’s disease without cognitive impairment; BMI, body mass index; H-Y, Hoehn and Yahr; MoCA, Montreal Cognitive Assessment; PDD, Parkinson’s disease with dementia; UPDRS-III, Unified Parkinson’s Disease Rating Scale Part III; Hcy, homocysteine; NSE, neuron-specific enolase; SNH, substantial nigra hyperechogenicity.
Based on the MoCA threshold of 26, 53.0% (204/385) of patients in the training set had CI, 47.5% (97/204) of whom were diagnosed with PDD. In the test set, 69.7% (115/165) exhibited CI, with 49.6% (57/115) diagnosed with PDD. In the training set, significant differences were observed in gender, age, hyperlipidemia, Hoehn and Yah (H-Y) stage, education level, PDD, and UPDRS-III score, whereas differences in other variables, such as disease duration, BMI, history of smoking and drinking, hypertension, and DM, were not statistically significant. These findings were consistent with those of the test cohort (Table 1).
Serum and imaging biomarkers
The ICC values for SN grading and calculation of SNH area were 0.80 (range, 0.78–0.83) and 0.73 (range, 0.71–0.77), respectively, indicating an acceptable level of agreement between raters. In both sets, the levels of NSE (P<0.001), Hcy concentration (P=0.03), and percentage of SNH (P<0.001) were higher in the PD-CI group than in the PD-NCI group. In the training set, a larger area of SN (P=0.001) was found in the PD-CI group. There were no significant differences observed in the levels of plasma folate, VitB12, or ferritin between the two groups (Table 1).
Factor selection for the predictive model
In the training set, Spearman correlation analysis showed that age and disease duration were associated with education level, H-Y stage, UPDRS-III score, Hcy, and NSE (Table S2). Additionally, gender differences in education level, Hcy, and area of SNH were significantly different (Table S3). These three factors were employed as covariates in subsequent analyses. Spearman partial correlation analysis indicated that H-Y stage (r=–0.198; P<0.001), UPDRS-III (r=–0.269; P<0.001), education status (r=0.401; P<0.001), Hcy (r=–0.134; P<0.001), and NSE level (r=0.303; P<0.001) were all correlated to MoCA score after adjustments were made for age, disease duration, and gender (Table 2).
Table 2
Parameter | H-Y stage | UPDRS-III | MoCA | Education level | Hcy | Folate | Vitamin B12 | Ferritin | NSE | Area of SNH |
---|---|---|---|---|---|---|---|---|---|---|
H-Y stage | – | −0.399 | 0.198 | −0.132 | −0.222 | 0.017 | −0.085 | −0.006 | −0.087 | 0.060 |
P | <0.001 | <0.001 | 0.01 | <0.001 | 0.74 | 0.10 | 0.90 | 0.09 | 0.24 | |
UPDRS-III | 0.399 | – | −0.269 | 0.177 | 0.109 | −0.111 | −0.035 | −0.005 | 0.111 | 0.003 |
P | <0.001 | <0.001 | 0.001 | 0.03 | 0.03 | 0.49 | 0.92 | 0.03 | 0.95 | |
MoCA | −0.198 | −0.269 | – | 0.401 | −0.134 | 0.030 | 0.034 | −0.061 | −0.303 | −0.082 |
P | <0.001 | <0.001 | <0.001 | 0.009 | 0.56 | 0.5 | 0.23 | <0.001 | 0.10 | |
Education level | −0.132 | −0.177 | 0.401 | – | −0.109 | 0.035 | −0.015 | −0.169 | −0.089 | 0.049 |
P | 0.01 | 0.001 | <0.001 | 0.03 | 0.50 | 0.76 | 0.001 | 0.08 | 0.34 | |
Hcy | −0.222 | 0.109 | −0.134 | −0.109 | – | −0.264 | −0.234 | 0.076 | 0.005 | 0.037 |
P | <0.001 | 0.03 | 0.009 | 0.03 | <0.001 | <0.001 | 0.14 | 0.92 | 0.47 | |
Folate | 0.017 | −0.111 | 0.03 | 0.035 | −0.264 | – | 0.192 | −0.060 | −0.024 | −0.024 |
P | 0.74 | 0.03 | 0.56 | 0.50 | <0.001 | <0.001 | 0.24 | 0.64 | 0.64 | |
Vitamin B12 | −0.085 | −0.035 | 0.034 | −0.015 | −0.234 | 0.192 | – | 0.070 | −0.042 | −0.042 |
P | 0.10 | 0.49 | 0.50 | 0.76 | <0.001 | <0.001 | 0.17 | 0.41 | 0.41 | |
Ferritin | −0.006 | −0.005 | −0.061 | −0.169 | 0.076 | −0.063 | 0.070 | – | −0.012 | −0.023 |
P | 0.90 | 0.92 | 0.23 | 0.001 | 0.14 | 0.24 | 0.17 | 0.81 | 0.65 | |
NSE | −0.087 | 0.111 | −0.303 | −0.089 | 0.005 | −0.024 | −0.042 | −0.012 | – | 0.106 |
P | 0.09 | 0.03 | <0.001 | 0.08 | 0.92 | 0.64 | 0.41 | 0.81 | 0.03 | |
Area of SNH | 0.06 | 0.003 | −0.082 | 0.049 | 0.037 | −0.024 | −0.042 | −0.023 | 0.106 | – |
P | 0.24 | 0.95 | 0.10 | 0.34 | 0.47 | 0.64 | 0.41 | 0.65 | 0.03 |
H-Y, Hoehn and Yahr; UPDRS-III, unified Parkinson’s Disease Rating Scale Part III; MoCA, Montreal Cognitive Assessment; Hcy, homocysteine; NSE, neuron-specific enolase; SNH, substantial nigra hyperechogenicity.
The ROC curve showed that plasma NSE alone exhibited a moderate discriminatory power for identifying cognitive decline in PD, with an AUC of 0.774 [95% confidence interval (CI): 0.725–0.823; P<0.001]. The sensitivity and specificity of NSE in diagnosing CI in PD were 79.4% and 82.7%, respectively, with a cutoff value of 15.255 (Figure 2A). In the test group, the AUC for NSE in predicting PD-CI was 0.760 (95% CI: 0.684–0.837; P<0.001), and the corresponding sensitivity and specificity were 79.6% and 85.7%, respectively (Figure 2B).
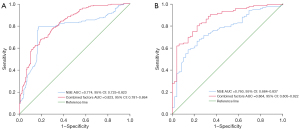
For the identification of risk factors affecting cognitive function, univariate logistic regression showed that age, gender, hyperlipidemia, H-Y stage, education level, UPDRS-III, Hcy, NSE, SNH, and area of SNH were potential risk factors. After the influence of age, disease duration, and gender was accounted for, the final multivariable logistic model incorporated six predictors (age, gender, education level, UPDRS-III, NSE, and SNH) in both the training and test sets (Table 3, Table S4). A nomogram was generated based on these risk factors and presented in Figure 3.
Table 3
Parameter | Cognitive impairment (univariate analysis) | Cognitive impairment (multivariate analysis) | |||
---|---|---|---|---|---|
OR (95% CI) | P value | OR (95% CI) | P value | ||
Gender (male) | 0.492 (0.251, 0.967) | 0.03 | 0.645 (0.220, 0.972) | 0.03 | |
Age | 1.053 (1.012, 1.095) | 0.01 | 1.081 (1.030, 1.134) | 0.002 | |
BMI | 1.046 (0.951, 1.150) | 0.35 | |||
Duration of disease | 0.986 (0.918, 1.058) | 0.69 | |||
Education year | 0.846 (0.774, 0.925) | <0.001 | 0.804 (0.714, 0.905) | <0.001 | |
Drinking history | 0.805 (0.332, 1.956) | 0.63 | |||
Smoking history | 0.928 (0.393, 2.189) | 0.86 | |||
Hypertension | 0.694 (0.347, 1.386) | 0.30 | |||
Diabetes mellitus | 0.689 (0.257, 1.845) | 0.45 | |||
Hyperlipidemia | 0.715 (0.309, 1.658) | 0.44 | |||
H-Y stage | 1.723 (1.128, 2.631) | 0.01 | 1.091 (0.565, 2.108) | 0.79 | |
PDD | 1.096 (0.547, 2.196) | 0.79 | |||
UPDRS-III | 1.029 (1.006, 1.052) | 0.01 | 1.023 (1.009, 1.051) | 0.03 | |
Hcy | 1.070 (1.005, 1.138) | 0.03 | 1.048 (0.974, 1.127) | 0.20 | |
Folate | 1.047 (0.983, 1.115) | 0.15 | |||
Ferritin | 1.004 (1.000, 1.008) | 0.08 | 1.001 (0.935, 1.013) | 0.05 | |
Vitamin B12 | 1.00 (0.999, 1.001) | 0.95 | |||
NSE | 1.398 (1.214, 1.609) | <0.001 | 1.434 (1.218, 1.688) | <0.001 | |
SNH | 0.453 (0.227, 0.902) | 0.02 | 0.527 (0.208, 0.804) | 0.03 | |
Area of SNH | 0.324 (0.093, 1.132) | 0.07 | 0.320 (0.091, 1.127) | 0.08 |
OR, odds ratio; CI, confidence interval; BMI, body mass index; H-Y, Hoehn and Yahr; PDD, Parkinson’s disease with dementia; UPDRS-III, Unified Parkinson’s Disease Rating Scale Part III; Hcy, homocysteine; NSE, neuron-specific enolase; SNH, substantial nigra hyperechogenicity.
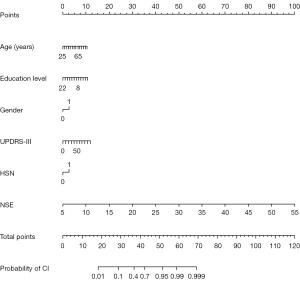
Validation and calibration of the nomogram
To verify the accuracy of the nomogram, internal validation was conducted using the bootstrap method with 1000 resamples. The final model had a C-index of 0.823 (95% CI: 0.781–0.864; P<0.001), along with a sensitivity of 67.6% and a specificity of 81.0% (Figure 2A). In the test group, the final model achieved an AUC of 0.864 (95% CI: 0.805–0.922; P<0.001) for predicting PD-CI (Figure 2B), with a sensitivity and specificity of 80.0% and 77.6%, respectively. These results indicated the nomogram’s discriminatory ability. Furthermore, the calibration curves in both cohorts demonstrated a high level of agreement between the predicted and ideal models (Figure S2A,S2B).
Discussion
The predictive model for clinical diagnosis and prognosis is a valuable tool and has been partially applied in clinical practice (22,23). In a previous study, a nomogram was constructed by combining dopamine transporter (DAT) imaging, CSF biomarkers (p-tau/α-syn and p-tau), and the rs55785911 genotype to predict mild CI in patients with PD and rapid-eye-movement sleep behavior disorder (24). However, the high costs associated with both DAT imaging and genotypic sequencing, as well as the unsuitability of lumbar puncture for certain individuals, limit the broad application of this model in clinical settings. TCS is a cost-effective and reproducible technique, and its qualitative and quantitative analysis of SN echogenicity has been clinically recommended as an auxiliary diagnostic indicator of PD (25,26). Plasma markers are also readily available parameters in daily practice. Therefore, the primary purpose of this study was to develop an objective and practical nomogram based on clinical and TCS imaging biomarkers to assist clinicians in identifying CI in patients with PD. Our results identified six independent risk predictors of CI in PD, including age, gender, UPRDS-III scores, education status, plasma NSE level, and SNH. A visualizable nomogram was developed based on these factors, which yielded a satisfactory diagnostic power for CI in PD with an AUC of 0.823 (95% CI: 0.781–0.864; P<0.001). The AUC of the test set also supported the reliability of the model.
The prominent neurodegeneration in the SN is the main cause of striatal dopamine dysfunction, leading to cardinal motor symptoms and cognitive deficits in PD (27). Iron deposition and microglia activation play an important role in the progression of SNH. In an animal study, the injection of a dopamine-6-hydroxylase-induced PD model resulted in poor results in cognitive-behavioral tests, and immunofluorescence analysis also indicated massive death of ipsilateral SN tyrosine hydroxylase-positive neurons (28), providing indirect evidence of the correlation between the decline in cognitive function and the loss of SN dopaminergic neurons. Recently, several studies have explored the correlation between SN and NMSs (29-31). Increased SN echogenicity is correlated with visual hallucinations (31), and the MoCA score has been reported to have a positive correlation with the correlated phase value of SN as assessed by QSM (30). Our previous research found significant differences in the ultrasound grading of the SN between NCI and CI in patients with PD (16), but the relationship between CI and area of SNH has not been investigated to date. In this study, we quantified CI in patients with PD by employing the MoCA test since it has a better performance and a higher sensitivity in identifying mild CI than does the MMSE (32,33). Univariate logistic analysis showed that SNH and area of SNH were potential risk factors for CI in PD, but multivariable logistic analysis revealed only SNH assessed by TCS was an independent marker of CI. Furthermore, correlation analysis revealed there to be no obvious correlation between the area of SNH and the severity of disease (H-Y stage and UPDRS-III score), which is consistent with a prior study (34). This suggests that the alternation of SN echogenicity evaluated by TCS may be able to qualitatively assess changes in cognitive function in patients with PD but cannot be used as a quantitative index. The SN is subdivided into two distinct parts: the SN pars compacta (SNc) and the SN pars reticulata (SNr). Dorsolateral sensorimotor, dorsomedial limbic, and ventral associative are three main subregions in the SNc (35). Studies reported spatiotemporal changes in nigrostriatal iron content in PD (36), which were correlated with MoCA and could be quantified with QSM (37). However, TCS exhibits limitations in the subregional segmentation of the SN. Therefore, further exploration of the relationship between the subregional distribution characteristics of SNH and cognitive function is needed. This exploration can be guided by TCS-MRI fusion imaging in conjunction with volumetric imaging, which may offer a means to gaining a deeper understanding of the pathology of SNH development.
NSE is a soluble protein found mostly in the cytoplasm of neurons within the cortex and subcortical areas, constituting a considerable portion of the brain’s total soluble protein. Elevated CSF and circulating NSE reflect neuronal damage after traumatic brain injury and oxidative damage associated with neurodegenerative diseases (38). However, the literature on NSE in PD is contradictory (8,39). Regarding the correlation between NSE and CI, circulation NSE values were found to be an independent predictor of CI 6 months after patients experienced out-of-hospital cardiac arrest with a seemingly favorable outcome (40). These values also play a crucial role in predicting CI in patients undergoing hemodialysis (41). In our study, an increased plasma NSE was observed in patients with PD and CI, which aligns with the result of a meta-analysis by Katayama et al. (8). Univariate and multivariable regression analyses further indicated NSE level as an independent factor affecting cognition in PD. Although the pathological mechanism of CI in PD is unclear, amyloid- and tau-related pathological changes, as well as other pathologies, such as mitochondrial and neurotransmitter systems and α-synuclein in the brain (which can induce neurotoxicity and alleviate neuroinflammation and apoptotic neuronal cell death), are likely to contribute to cognitive dysfunction in PD (3). Circulating NSE level has been recommended as a biomarker for predicting the neurological outcome of adults who are comatose after cardiac arrest (42). Elevated NSE levels can stimulate inflammatory glial cell proliferation and actin remodeling, influencing the migration of activated macrophages and microglia to the injury site and encouraging neuronal cell death (38).
Besides NSE and SNH, our results demonstrated that age, education level, and UPDRS-III were independent determinants of CI in patients with PD. It is widely recognized that cognitive ability tends to diminish with advancing age, primarily due to the correlation between age and brain atrophy, which directly affects cognitive function. Higher education levels correlate with better cognitive performance. Nguyen et al.’s systematic review indicated that education has the potential to act as a preventive factor against dementia (43). A study in China involving 1,286 older adult individuals supports the key role of education in maintaining cognitive health in disadvantageous settings (44). The UPDRS-III is a test used to assess motor function in patients with PD. A higher initial UPDRS-III score suggests a more severe underlying pathology. Research has shown that the UPDRS-III score is positively correlated with the improvement rate of cognitive function after DBS implantation (45). Chen and colleagues generated a predictive model for CI in PD that combines age of onset, hypertension, baseline MoCA scores, UPDRS-III scores, and APOE ε4, achieving an AUC of 0.80 (46). These investigations provide additional evidence for the association between cognitive status and the progression of PD.
Notably, male individuals in our study exhibited inferior cognitive performance compared to females. This finding was consistent with other research (47) indicating a positive correlation between increased striatal dopaminergic activity in females compared to males. This difference may be attributed to the protective effects of estrogen, which decreases DAT activity and enhances dopamine availability at synapses (48). Therefore, gender differences should be taken into account when using a precision medicine approach to preventing cognition decline in PD.
Some limitations to this study need to be addressed. First, although we applied MoCA to evaluate the cognitive state of participants, we did not perform a detailed analysis of the correlation between the seven cognitive domains of MoCA and the risk factors, nor did we carry out a comprehensive analysis of the specific cognitive aspects affected by elevated NSE concentration and SNH. Second, it should be mentioned that PD CI encompasses mild cognitive impairment (MCI) and dementia. In this cross-sectional study, we categorized participants into two groups based on the MoCA criteria: those with normal cognition and those experiencing cognitive decline. Future studies should consider further subdividing CI into MCI and dementia and conducting follow-up assessments to validate the discriminative efficacy and predictive power of the model in MCI and dementia, as well as dementia conversion. Third, we did not examine the impact of PD treatment on cognitive performance, which is an important factor that should not be overlooked. Furthermore, the role of NSE is diverse and somewhat unclear, so other explanations for the levels of serum NSE in PD-related CI cannot be ruled out.
To conclude, we found that circulating NSE concentration and SNH evaluated by TCS were associated with CI in PD. We developed a simple and practical model by combining age, education status, UPDRS-III scores, plasma NSE level, and SNH to predict cognitive condition in patients with PD. The integration of clinical and neuroimaging biomarkers demonstrated good diagnostic performance for identifying CI in patients with PD.
Acknowledgments
Funding: This work was supported by
Footnote
Reporting Checklist: The authors have completed the TRIPOD reporting checklist. Available at https://qims.amegroups.com/article/view/10.21037/qims-23-1778/rc
Conflicts of Interest: All authors have completed the ICMJE uniform disclosure form (available at https://qims.amegroups.com/article/view/10.21037/qims-23-1778/coif). The authors have no conflicts of interest to declare.
Ethical Statement: The authors are accountable for all aspects of the work in ensuring that questions related to the accuracy or integrity of any part of the work are appropriately investigated and resolved. This cross-sectional study was approved by the Ethics Board of Beijing Tiantan Hospital, Capital Medical University (No. KT2022-015-04) and was conducted in accordance with the Declaration of Helsinki (as revised in 2013). Informed consent was obtained from all the patients.
Open Access Statement: This is an Open Access article distributed in accordance with the Creative Commons Attribution-NonCommercial-NoDerivs 4.0 International License (CC BY-NC-ND 4.0), which permits the non-commercial replication and distribution of the article with the strict proviso that no changes or edits are made and the original work is properly cited (including links to both the formal publication through the relevant DOI and the license). See: https://creativecommons.org/licenses/by-nc-nd/4.0/.
References
- Zheng Z, Zhu Z, Zhou C, Cao L, Zhao G. Burden of Parkinson Disease in China, 1990-2019: Findings from the 2019 Global Burden of Disease Study. Neuroepidemiology 2023;57:51-64. [Crossref] [PubMed]
- Global, regional, and national burden of neurological disorders, 1990-2016: a systematic analysis for the Global Burden of Disease Study 2016. Lancet Neurol 2019;18:459-80. [Crossref] [PubMed]
- Aarsland D, Creese B, Politis M, Chaudhuri KR, Ffytche DH, Weintraub D, Ballard C. Cognitive decline in Parkinson disease. Nat Rev Neurol 2017;13:217-31. [Crossref] [PubMed]
- Pigott K, Rick J, Xie SX, Hurtig H, Chen-Plotkin A, Duda JE, Morley JF, Chahine LM, Dahodwala N, Akhtar RS, Siderowf A, Trojanowski JQ, Weintraub D. Longitudinal study of normal cognition in Parkinson disease. Neurology 2015;85:1276-82. [Crossref] [PubMed]
- Bäckström D, Granåsen G, Domellöf ME, Linder J, Jakobson Mo S, Riklund K, Zetterberg H, Blennow K, Forsgren L. Early predictors of mortality in parkinsonism and Parkinson disease: A population-based study. Neurology 2018;91:e2045-56. [Crossref] [PubMed]
- Livingston G, Huntley J, Sommerlad A, Ames D, Ballard C, Banerjee S, et al. Dementia prevention, intervention, and care: 2020 report of the Lancet Commission. Lancet 2020;396:413-46. [Crossref] [PubMed]
- Carceles-Cordon M, Weintraub D, Chen-Plotkin AS. Cognitive heterogeneity in Parkinson's disease: A mechanistic view. Neuron 2023;111:1531-46. [Crossref] [PubMed]
- Katayama T, Sawada J, Takahashi K, Yahara O, Hasebe N. Meta-analysis of cerebrospinal fluid neuron-specific enolase levels in Alzheimer's disease, Parkinson's disease, dementia with Lewy bodies, and multiple system atrophy. Alzheimers Res Ther 2021;13:163. [Crossref] [PubMed]
- Cao Q, Han X, Tang D, Qian H, Yan K, Shi X, Li Y, Zhang J. Diagnostic value of combined magnetic resonance imaging techniques in the evaluation of Parkinson disease. Quant Imaging Med Surg 2023;13:6503-16. [Crossref] [PubMed]
- Li QQ, Wu K, Xu JL, Yin L. White matter damage in patients with mild cognitive impairment in Parkinson's disease. Quant Imaging Med Surg 2022;12:1290-8. [Crossref] [PubMed]
- Uchida Y, Kan H, Sakurai K, Oishi K, Matsukawa N. Quantitative susceptibility mapping as an imaging biomarker for Alzheimer's disease: The expectations and limitations. Front Neurosci 2022;16:938092. [Crossref] [PubMed]
- Lee YG, Jeon S, Baik K, Kang SW, Ye BS. Substantia nigral dopamine transporter uptake in dementia with Lewy bodies. NPJ Parkinsons Dis 2023;9:88. [Crossref] [PubMed]
- Uchida Y, Kan H, Sakurai K, Inui S, Kobayashi S, Akagawa Y, Shibuya K, Ueki Y, Matsukawa N. Magnetic Susceptibility Associates With Dopaminergic Deficits and Cognition in Parkinson's Disease. Mov Disord 2020;35:1396-405. [Crossref] [PubMed]
- Yu JJ, Li C, Qian ZM, Liu Y. Brain iron deposition is positively correlated with cognitive impairment in patients with chronic cerebral hypoperfusion: a MRI susceptibility mapping study. Clin Radiol 2023;78:601-7. [Crossref] [PubMed]
- Wang LS, Yu TF, Chai B, He W. Transcranial sonography in differential diagnosis of Parkinson disease and other movement disorders. Chin Med J (Engl) 2021;134:1726-31. [Crossref] [PubMed]
- Chen Z, Zhang W, He W, Guang Y, Yu T, Du Y, Li R. Transcranial sonography with clinical and demographic characteristics to predict cognitive impairment in PD: a longitudinal study. BMC Neurol 2023;23:15. [Crossref] [PubMed]
- Postuma RB, Berg D, Stern M, Poewe W, Olanow CW, Oertel W, Obeso J, Marek K, Litvan I, Lang AE, Halliday G, Goetz CG, Gasser T, Dubois B, Chan P, Bloem BR, Adler CH, Deuschl G. MDS clinical diagnostic criteria for Parkinson's disease. Mov Disord 2015;30:1591-601. [Crossref] [PubMed]
- Skorvanek M, Goldman JG, Jahanshahi M, Marras C, Rektorova I, Schmand B, van Duijn E, Goetz CG, Weintraub D, Stebbins GT, Martinez-Martin P. Global scales for cognitive screening in Parkinson's disease: Critique and recommendations. Mov Disord 2018;33:208-18. [Crossref] [PubMed]
- Kang JJ, Chen Y, Xu GD, Bao SL, Wang J, Ge M, Shen LH, Jia ZZ. Combining quantitative susceptibility mapping to radiomics in diagnosing Parkinson's disease and assessing cognitive impairment. Eur Radiol 2022;32:6992-7003. [Crossref] [PubMed]
- Lilja G, Ullen S, Dankiewicz J, Friberg H, Levin H, Nordstrom EB, et al. Effects of Hypothermia vs Normothermia on Societal Participation and Cognitive Function at 6 Months in Survivors After Out-of-Hospital Cardiac Arrest: A Predefined Analysis of the TTM2 Randomized Clinical Trial. JAMA Neurol 2023;80:1070-9. [Crossref] [PubMed]
- Bartova P, Skoloudik D, Bar M, Ressner P, Hlustik P, Herzig R, Kanovsky P. Transcranial sonography in movement disorders. Biomed Pap Med Fac Univ Palacky Olomouc Czech Repub 2008;152:251-8. [Crossref] [PubMed]
- Serra-Burriel M, Juanola A, Serra-Burriel F, Thiele M, Graupera I, Pose E, et al. Development, validation, and prognostic evaluation of a risk score for long-term liver-related outcomes in the general population: a multicohort study. Lancet 2023;402:988-96. [Crossref] [PubMed]
- Clift AK, Dodwell D, Lord S, Petrou S, Brady M, Collins GS, Hippisley-Cox J. Development and internal-external validation of statistical and machine learning models for breast cancer prognostication: cohort study. BMJ 2023;381:e073800. [Crossref] [PubMed]
- Chen F, Li Y, Ye G, Zhou L, Bian X, Liu J. Development and Validation of a Prognostic Model for Cognitive Impairment in Parkinson's Disease With REM Sleep Behavior Disorder. Front Aging Neurosci 2021;13:703158. [Crossref] [PubMed]
- Walter U, Školoudík D. Transcranial sonography (TCS) of brain parenchyma in movement disorders: quality standards, diagnostic applications and novel technologies. Ultraschall Med 2014;35:322-31. [Crossref] [PubMed]
- Sprenger FS, Wurster I, Seppi K, Stockner H, Scherfler C, Sojer M, Schmidauer C, Berg D, Poewe W. Substantia nigra hyperechogenicity and Parkinson's disease risk in patients with essential tremor. Mov Disord 2016;31:579-83. [Crossref] [PubMed]
- Westbrook A, van den Bosch R, Määttä JI, Hofmans L, Papadopetraki D, Cools R, Frank MJ. Dopamine promotes cognitive effort by biasing the benefits versus costs of cognitive work. Science 2020;367:1362-6. [Crossref] [PubMed]
- Yang L, Zhang X, Li S, Wang H, Zhang X, Liu L, Xie A. Intranasal insulin ameliorates cognitive impairment in a rat model of Parkinson's disease through Akt/GSK3β signaling pathway. Life Sci 2020;259:118159. [Crossref] [PubMed]
- Shibata H, Uchida Y, Inui S, Kan H, Sakurai K, Oishi N, Ueki Y, Oishi K, Matsukawa N. Machine learning trained with quantitative susceptibility mapping to detect mild cognitive impairment in Parkinson's disease. Parkinsonism Relat Disord 2022;94:104-10. [Crossref] [PubMed]
- Liu Z, Shen HC, Lian TH, Mao L, Tang SX, Sun L, Huang XY, Guo P, Cao CJ, Yu SY, Zuo LJ, Wang XM, Chen SD, Chan P, Zhang W. Iron deposition in substantia nigra: abnormal iron metabolism, neuroinflammatory mechanism and clinical relevance. Sci Rep 2017;7:14973. [Crossref] [PubMed]
- Wei R, Zhang Y, Li P, Xu Z, Zhang L, He Y, Wu Q, Shi Y, Yuan Y, Aisha A. Substantia Nigra Echogenicity Signal Correlated with Clinical Features in Patients with Parkinson's Disease in Xinjiang. Parkinsons Dis 2023;2023:8867546. [Crossref] [PubMed]
- Ciesielska N, Sokołowski R, Mazur E, Podhorecka M, Polak-Szabela A, Kędziora-Kornatowska K. Is the Montreal Cognitive Assessment (MoCA) test better suited than the Mini-Mental State Examination (MMSE) in mild cognitive impairment (MCI) detection among people aged over 60? Meta-analysis. Psychiatr Pol 2016;50:1039-52. [Crossref] [PubMed]
- Wu Y, Zhang Y, Yuan X, Guo J, Gao X. Influence of education level on MMSE and MoCA scores of elderly inpatients. Appl Neuropsychol Adult 2023;30:414-8. [Crossref] [PubMed]
- Gao HL, Qu Y, Chen SC, Yang QM, Li JY, Tao AY, Mao ZJ, Xue Z. Third ventricular width by transcranial sonography is associated with cognitive impairment in Parkinson's disease. CNS Neurosci Ther 2024;30:e14360. [Crossref] [PubMed]
- Haber SN. The place of dopamine in the cortico-basal ganglia circuit. Neuroscience 2014;282:248-57. [Crossref] [PubMed]
- Biondetti E, Santin MD, Valabrègue R, Mangone G, Gaurav R, Pyatigorskaya N, Hutchison M, Yahia-Cherif L, Villain N, Habert MO, Arnulf I, Leu-Semenescu S, Dodet P, Vila M, Corvol JC, Vidailhet M, Lehéricy S. The spatiotemporal changes in dopamine, neuromelanin and iron characterizing Parkinson's disease. Brain 2021;144:3114-25. [Crossref] [PubMed]
- Uchida Y, Kan H, Sakurai K, Arai N, Kato D, Kawashima S, Ueki Y, Matsukawa N. Voxel-based quantitative susceptibility mapping in Parkinson's disease with mild cognitive impairment. Mov Disord 2019;34:1164-73. [Crossref] [PubMed]
- Haque A, Polcyn R, Matzelle D, Banik NL. New Insights into the Role of Neuron-Specific Enolase in Neuro-Inflammation, Neurodegeneration, and Neuroprotection. Brain Sci 2018;8:33. [Crossref] [PubMed]
- Katayama T, Sawada J, Kikuchi-Takeguchi S, Kano K, Takahashi K, Saito T, Okizaki A, Hasebe N. Cerebrospinal fluid levels of alpha-synuclein, amyloid β, tau, phosphorylated tau, and neuron-specific enolase in patients with Parkinson's disease, dementia with Lewy bodies or other neurological disorders: Their relationships with cognition and nuclear medicine imaging findings. Neurosci Lett 2020;715:134564. [Crossref] [PubMed]
- Brønnick K, Evald L, Duez CHV, Grejs AM, Jeppesen AN, Kirkegaard H, Nielsen JF, Søreide E. Biomarker prognostication of cognitive impairment may be feasible even in out-of hospital cardical arrest survivors with good neurological outcome. Resuscitation 2021;162:396-402. [Crossref] [PubMed]
- Chen JB, Chang CC, Li LC, Lee WC, Lin CN, Li SC, Moi SH, Yang CH. Mutual Interaction of Clinical Factors and Specific microRNAs to Predict Mild Cognitive Impairment in Patients Receiving Hemodialysis. Cells 2020;9:2303. [Crossref] [PubMed]
- Nolan JP, Sandroni C, Böttiger BW, Cariou A, Cronberg T, Friberg H, Genbrugge C, Haywood K, Lilja G, Moulaert VRM, Nikolaou N, Olasveengen TM, Skrifvars MB, Taccone F, Soar J. European Resuscitation Council and European Society of Intensive Care Medicine guidelines 2021: post-resuscitation care. Intensive Care Med 2021;47:369-421. [Crossref] [PubMed]
- Nguyen HXT, Bradley K, McNamara BJ, Watson R, Malay R, LoGiudice D. Risk, protective, and biomarkers of dementia in Indigenous peoples: A systematic review. Alzheimers Dement 2024;20:563-92. [Crossref] [PubMed]
- Wang Y, Jiang Y, Wu W, Xu K, Zhao Q, Tan Z, Liang X, Fan M, Xiao Z, Zheng L, Ding S, Dong Q, Hong Z, Jin L, Chen X, Ding D, Cui M. Education, neighborhood environment, and cognitive decline: Findings from two prospective cohort studies of older adults in China. Alzheimers Dement 2023;19:560-8. [Crossref] [PubMed]
- Chang B, Ni C, Zhang W, Mei J, Xiong C, Chen P, Jiang M, Niu C. Nomogram to Predict Cognitive State Improvement after Deep Brain Stimulation for Parkinson's Disease. Brain Sci 2022;12:759. [Crossref] [PubMed]
- Chen J, Zhao D, Wang Q, Chen J, Bai C, Li Y, Guo X, Chen B, Zhang L, Yuan J. Predictors of cognitive impairment in newly diagnosed Parkinson's disease with normal cognition at baseline: A 5-year cohort study. Front Aging Neurosci 2023;15:1142558. [Crossref] [PubMed]
- Boccalini C, Carli G, Pilotto A, Padovani A, Perani D. Gender differences in dopaminergic system dysfunction in de novo Parkinson's disease clinical subtypes. Neurobiol Dis 2022;167:105668. [Crossref] [PubMed]
- Meoni S, Macerollo A, Moro E. Sex differences in movement disorders. Nat Rev Neurol 2020;16:84-96. [Crossref] [PubMed]