Deep learning image reconstruction of diffusion-weighted imaging in evaluation of prostate cancer focusing on its clinical implications
Introduction
Prostate cancer (PCa) is the second most prevalent cancer in men and ranks fifth in terms of cancer-related mortality worldwide (1). Clinical suspicion of PCa is largely based on prostate-specific antigen (PSA) levels in combination with digital rectal examinations and other patient characteristics. Recently, pre-biopsy prostate magnetic resonance imaging (MRI) has been incorporated into the diagnostic workflow for selecting Prostate Imaging-Reporting and Data System (PI-RADS) ≥3 lesions as biopsy targets on MRI as recommended by the 2020 European Association of Urology guidelines (2). In the management of PCa, the choice of treatment intensity ranges from active surveillance to prostatectomy or radiotherapy, depending on various parameters, including PSA concentration, tumor stage, histological grade, and Gleason score (3). Discrimination of clinically significant prostate cancer (csPCa) from silent PCa has become more clinically relevant, as active management is required due to its aggressiveness and poor prognosis (4). Among the various criteria for csPCa, tumors with an International Society of Urological Pathology (ISUP) high grade (i.e., Gleason score 3+4 or higher) constitute the most common and important criterion for csPCa (5). For treatment decisions, 2023 NCCN Clinical Practice Guidelines in Oncology (NCCN Guidelines®) suggest that initial risk stratification according to overlapping features of csPCa and clinical T staging, along with life expectancy should be considered (6). Various applications in multiparametric prostate MRI have been investigated for the image-based prediction of csPCa (7,8).
The most recent PI-RADS v2.1 relies on T2-weighted image (T2WI) and diffusion weighted image (DWI) for the detection of csPCa based on imaging findings (9). Limitations in the positive predictive value of PI-RADS assessment arise primarily from the qualitative assessment of images, which heavily relies on (I) image quality, especially in DWI scans and apparent diffusion coefficient (ADC) maps (10,11), and (II) readers. A review article has reported only moderate to substantial inter-reader agreement for PI-RADS v.2.1, with k-statistics ranging from 0.42 to 0.70, lacking significant improvement since the last version of PI-RADS v2.0 (12). Advances in MRI technology with the implementation of deep learning (DL) algorithms for image reconstruction have improved image quality, reduced noise, increased signal-to-noise ratio (SNR), and shortened overall scan time (13). The application of DL in image reconstruction has resulted in a 65% reduction in acquisition time in prostate Turbo Spin Echo imaging without a significant loss of image quality (14). Furthermore, improvements in image quality with denoising algorithms and DL-based post-processing (15) have been successfully implemented for fast acquisition sequences (16), providing images with sufficient quality comparable to that of conventional images (17,18). Recently, DL-based reconstruction techniques have been validated on DWI with high-b-values of 1,000, 3,000, and 5,000 in the prostate gland to show a significant improvement in SNR and contrast-to-noise ratio (CNR), along with image quality, with a significant reduction in the overall scan time (19). However, its clinical relevance and influence on radiologist reporting, especially PI-RADS scoring, has yet to be reported.
Therefore, we hypothesized that DL-based reconstruction of prostate gland DWI can improve overall image quality and affect PI-RADS scoring regarding clinically significant tumors. We present this article in accordance with the STARD reporting checklist (available at https://qims.amegroups.com/article/view/10.21037/qims-23-1379/rc).
Methods
Study design
In this retrospective study, 97 patients who underwent prostate MRI scans between November 2021 and July 2022 at Korea University Ansan Hospital were examined. After excluding patients without deep learning reconstruction (DLR) (n=8), previous treatments such as surgery (n=3), local ablation/radiotherapy (n=7), or medication (n=5), lack of biopsy due to general condition (n=3), and no PCa on biopsy (n=1), 70 patients with biopsy-proven PCa were enrolled (Figure 1). Due to the current clinical workflow in our institution, all 70 patients had received prostate MRI scans post-biopsy.
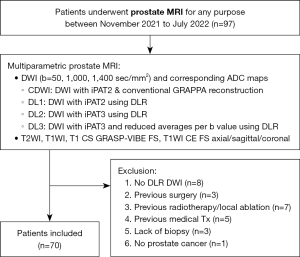
The study was conducted in accordance with the Declaration of Helsinki (as revised in 2013). The study was approved by the institutional review board of Korea University Ansan Hospital in the Republic of Korea (IRB No. 2023AS0046), and individual consent for this retrospective analysis was waived.
Image acquisition and reconstruction
All patients underwent prostate MRI using a 3.0 T scanner (MAGNETOM Vida; Siemens Healthcare, Erlangen, Germany) with a 30-channel body coil. T2WIs, DWI scans, and dynamic T1-weighted images with compressed sensing golden-angle radial sparse parallel-volumetric interpolated breath-hold examination (CS GRASP-VIBE) sequences were acquired. DWI scans were obtained with b-values of 50, 1,000, and 1,400 sec/mm2. In total, four different DWI image sets were acquired for each patient using the following settings: (I) conventional DWI (CDWI): DWI with acceleration factor 2 (iPAT2) and conventional Generalized Auto-calibrating Partially Parallel Acquisition (GRAPPA) reconstruction; (II) DL1: DWI with iPAT2 using DLR; (III) DL2: DWI with acceleration factor 3 (iPAT3) using DLR; and (IV) DL3: DWI with iPAT3 and reduced averages per b-value using DLR. All DL images were generated with a research application package. The specific acquisition parameters are listed in Table 1.
Table 1
Parameters (unit) | CDWI | DL1 | DL2 | DL3 | T2WI axial |
---|---|---|---|---|---|
Median acquisition time (min) | 04:46 | 04:46 | 03:40 | 02:00 | 03:06 |
TR/TE (ms) | 5,200/65 | 5,200/65 | 4,000/65 | 4,000/65 | 2,640/103 |
Flip angle (o) | 90 | 90 | 90 | 90 | 136 |
Diffusion mode | 3-Scan Trace | 3-Scan Trace | 3-Scan Trace | 3-Scan Trace | – |
Slice thickness/gap (mm) | 3/0 | 3/0 | 3/0 | 3/0 | 3/0.3 |
FOV (mm2) | 200×180 | 200×180 | 200×180 | 200×180 | 180×180 |
Acquisition matrix | 120×108 | 120×108 | 120×108 | 120×108 | 320×320 |
Reconstruction matrix | 240×216 | 240×216 | 240×216 | 240×216 | 640×640 |
b-values (s/mm2) | 50/1,000/1,400 | 50/1,000/1,400 | 50/1,000/1,400 | 50/1,000/1,400 | – |
Bandwidth, Hz/pixel | 1,667 | 1,667 | 1,667 | 1,667 | 200 |
No. averages | 3/6/8 | 3/6/8 | 3/6/8 | 2/3/4 | 2 |
Echo train length | 54 | 54 | 36 | 36 | 18 |
Parallel imaging factor | 2 | 2 | 3 | 3 | 2 |
MRI, magnetic resonance imaging; CDWI, conventional diffusion weighted image; DL1, DWI with iPAT2 using DLR; DL2, DWI with iPAT3 using DLR; DL3, DWI with iPAT3 and reduced averages per b-value using DLR; T2WI, T2-weighted image; TR, repetition time; TE, echo time; FOV, field of view; DWI, diffusion weighted image; DLR, deep learning reconstruction; iPAT2, acceleration factor 2; iPAT3, acceleration factor 3.
The DLR consisted of two steps. First, DLR of the k-space data was performed following the scheme of a variational neural network (20). The k-space and pre-calculated coil sensitivity maps were used as inputs. Seventeen iterations were performed in total with Nesterov-type extrapolation and gradient steps with trainable step sizes. Of these 17 iterations, the first 6 focused on parallel imaging without applying additional regularization. The remaining 11 iterations employed a convolutional neural network with a hierarchical down-up architecture as the regularizer. Training of the reconstruction scheme was performed in a supervised fashion on approximately 500,000 single-shot DWI images acquired from volunteers using clinical 1.5T and 3T scanners (MAGNETOM, Siemens Healthcare, Erlangen, Germany). Based on this data, the training pairs were built by retrospectively doubling the parallel imaging acceleration factor.
Following the DL-based k-space for image reconstruction, a DL-based super-resolution network was applied to the single-shot images to increase sharpness. For this purpose, a network based on the pixel shuffle architecture (21) was trained using volunteer magnetic resonance images of various body parts acquired from several volunteers. To avoid modifying the acquired image information, hard data consistency was applied, and only non-measured frequencies were extrapolated. For training, image pairs were built by reducing the spatial resolution two-fold along the readout and phase-encoding directions.
After training the networks in Python, they were frozen and integrated into the C++-based reconstruction pipeline on the scanner. Following the DL-based reconstruction steps, DWI processing of single-shot images, including averaging and apparent diffusion coefficient (ADC) calculations, was performed analogously to CDWI.
Image assessment
Two radiologists with varying experience (18 and 2 years of clinical experience) who were blinded to the DWI techniques, independently evaluated four data sets with 2-week intervals per data set. Each data set consisted of one of b-value =50, 1,000, 1,400 sec/mm2 DWI reconstruction images (CDWI, DL1–3) with corresponding ADC maps, and T2-weighted axial and coronal images. For the qualitative assessment of b-value =1,400 sec/mm2 DWI reconstruction images, noise (Noise Level: 1, high; 2, moderate; 3, mild, 4; minimal), lesion conspicuity, and overall image quality (Lesion conspicuity and Lesion conspicuity: 1, poor; 2, equivocal; 3, good; 4, excellent) were assessed using a four-point visual scoring system. Any lesion suspected of malignancy was scored using PI-RADS v2.1 by two radiologists, independently. To account for the positive select bias in our cohort, readers were asked to rate any lesion in the 12 sections of the prostate given in Figure S1. The largest lesion was evaluated for the scope of this study.
For quantitative evaluation, circular regions of interest (ROIs) were placed over the dominant prostate lesion, the internal obturator muscle for reference of contrast, and extracorporeal air for noise evaluation in the four sets of b-value =1,400 sec/mm2 DWI reconstruction images (CDWI, DL1–3) and corresponding ADC maps by one reviewer. For each patient, signal intensity (SI) of all ROIs were measured twice and averaged to obtain final values. SNR and CNR were calculated as follows: SNR = SIlesion/SDnoise and CNR = (SIlesion – SImuscle)/SDnoise, SI is the average signal intensity of the ROI, and SD is the standard deviation of the signal intensity measured in the ROI (22).
Histopathology data and analysis
Lesions were confirmed pathologically using transrectal ultrasonography (TRUS)-guided 12-core sampling needle biopsy following the recommendations of the American Urological Association (23), including the apex and far-lateral regions of the prostate gland. Biopsy specimens were acquired and labeled according to the panel shown in Figure S1. The biopsy results were analyzed for malignancy and the associated Gleason score sums by experienced pathologists who were blinded to the MRI findings. Radical prostatectomy or transurethral resection specimens were obtained in 31 patients. The sensitivity of TRUS-guided systemic biopsy and MRI-detected lesions was compared based on location matching of the 12 core-needle specimens and the 12 zones based on previous literature (24).
Statistical analysis
To determine the capability of DLR for image quality improvement, quantitative analysis (SNR, CNR, and ADC) was conducted on each high b-value image series with and without DLR using paired t-tests with Bonferroni correction, and qualitative analysis (four-point visual scoring system) was compared using the Wilcoxon signed-rank test with Bonferroni correction. To determine the clinical significance of this difference, PI-RADS scoring was grouped into highly malignant (PI-RADS 4 and 5) versus PI-RADS <4 lesions. The marginal probability of discriminating highly malignant lesions was compared among the DLR techniques using McNemar’s test. In addition, inter-reader agreement for qualitative assessment of image quality and PI-RADS scoring was evaluated using κ statistics, followed by the x2 test. The agreement was considered poor for κ of less than 0.21, fair for κ of 0.21–0.40, moderate for κ of 0.41–0.60, substantial for κ of 0.61–0.80, and excellent for κ of 0.81–1.00 (25). Subgroup analysis was conducted for csPCa (Gleason score ≥7) and higher than unfavorable intermediate risk group according to 2023 NCCN Guidelines® (6), determined by a combination of cT2b–cT2c, Gleason score 7, PSA 10–20 ng/mL (Table S1). The average of quantitative measurements (SNR, CNR, and ADC) was compared between groups using independent t-tests with Bonferroni correction. Two-sided P value <0.05 was considered indicative of a significant difference for each statistical analysis. Statistical analysis was performed using IBM SPSS software package version 26.0 (Statistical Package for Social Sciences™, Chicago, IL, USA).
Results
Clinical characteristics
A total of 70 patients (mean age, 70.8±9.7 years; age range, 41–88 years) were enrolled in this study. The general characteristics of the study participants are presented in Table 2. The mean PSA level was 76.45±263.54 ng/mL at the time of MRI. TRUS-guided biopsy confirmed PCa located only in the peripheral zone (PZ) in 20 patients (28.6%), in the transitional zone (TZ) in 3 patients (4.3%), and in both the PZ and TZ in 47 patients (67.1%). The Gleason score of surgical or biopsied specimens ranged from 6 to 10, with 51 (72.9%) patients with csPCa (Gleason score ≥7).
Table 2
Characteristics | Value (n=70) |
---|---|
Age, years (mean ± standard deviation) | 70.8±9.7 |
PSA, ng/mL, median (range) | 10.05 (0.73, 1,906) |
<10 | 34 (48.6%) |
10–20 | 16 (22.9%) |
>20 | 20 (28.6%) |
Tumor location* | |
TZ only | 3 (4.3%) |
PZ only | 20 (28.6%) |
TZ + PZ | 47 (67.1%) |
Gleason score*, median (range) | 7 (6, 10) |
6 | 19 (27.1%) |
7 | 30 (42.9%) |
8 | 14 (20.0%) |
9 | 1 (1.4%) |
10 | 6 (8.6%) |
T staging | |
cT2a | 18 (25.7%) |
cT2b | 4 (5.7%) |
cT2c | 20 (28.6%) |
cT3a | 8 (11.4%) |
cT3b | 11 (15.7%) |
cT4 | 8 (11.4%) |
Lymph node metastasis | |
cN0 | 53 (75.7%) |
cN1 | 17 (24.3%) |
Metastasis | |
cM0 | 58 (82.9%) |
cM1a | 3 (4.3%) |
cM1b | 9 (12.9%) |
Treatment | |
Surgery | 31 (44.3%) |
Radiotherapy | 19 (27.1%) |
Active surveillance | 13 (18.6%) |
Others | 7 (10.0%) |
Data are given as number of cases with percentile in parentheses if not otherwise stated. *, Gleason score and tumor location was assessed on 12-core-systemic biopsy or transurethral resection/total prostatectomy specimens when available. PSA, prostate-specific antigen; TZ, transitional zone; PZ, peripheral zone.
Qualitative assessment
The subjective image quality scores (noise, lesion conspicuity, and overall image quality) were assessed by two readers with fair to moderate agreement, with Cohen’s kappa values ranging from 0.31–0.44 (κ values; 0.44, 0.38, 0.31, respectively).
The results of the qualitative assessment of image quality are summarized in Table 3. For reader 1, statistically significant improvements in lesion conspicuity were observed in the DL1 and DL2 compared to CDWI (mean conspicuity scores of CDWI, DL1, DL2, DL3: 3.1, 3.5, 3.3, and 3.3, respectively), with no significant difference in noise levels or overall image quality between CDWI and DL1 images (mean noise scores of CDWI, DL1, DL2, DL3: 4.0, 3.9, 3.7, 3.2; mean overall image quality scores of CDWI, DL1, DL2, DL3: 3.8, 3.8, 3.6, 3.4, respectively).
Table 3
Image quality scores | Reader 1 | Reader 2 | |||||||||||||||||
---|---|---|---|---|---|---|---|---|---|---|---|---|---|---|---|---|---|---|---|
CDWI | DL1 | DL2 | DL3 | CDWI | DL1 | DL2 | DL3 | ||||||||||||
Mean ± SD (range) | P value | Mean ± SD (range) | P value | Mean ± SD (range) | P value | Mean ± SD (range) | P value | Mean ± SD (range) | P value | Mean ± SD (range) | P value | ||||||||
Noise | 4.0±0.2 (3–4, 4) |
3.9±0.2 (3–4, 4) |
0.32 | 3.7±0.5 (2–4, 4) |
<0.001 | 3.2±0.5 (2–4, 3) |
<0.001 | 3.8±0.4 (3–4, 4) |
3.8±0.4 (3–4, 4) |
0.53 | 3.7±0.5 (2–4, 4) |
0.02 | 3.0±0.7 (2–4, 3) |
<0.001 | |||||
Lesion conspicuity | 3.1±0.9 (1–4, 3) |
3.5±0.6 (2–4, 4) |
<0.001 | 3.3±0.7 (2–4, 3) |
<0.001 | 3.3±0.8 (2–4, 3) |
0.01 | 2.9±0.8 (1–4, 3) |
3.6±0.5 (2–4, 4) |
<0.001 | 3.2±0.8 (1–4, 3) |
0.01 | 3.1±0.9 (1–4, 3) |
0.34 | |||||
Overall image quality | 3.8±0.4 (3–4, 4) |
3.8±0.4 (3–4, 4) |
0.71 | 3.6±0.5 (2–4, 4) |
0.01 | 3.4±0.6 (2–4, 3) |
<0.001 | 3.9±0.4 (2–4, 4) |
3.9±0.4 (2–4, 4) |
1 | 3.7±0.6 (2–4, 4) |
0.03 | 3.6±0.6 (2–4, 4) |
0.001 |
Noise (Noise Level: 1, high; 2, moderate; 3, mild; 4, minimal), lesion conspicuity and overall image quality (Lesion conspicuity and Lesion conspicuity: 1, poor; 2, equivocal; 3, good; 4, excellent) were assessed using a four-point visual scoring system on b-value =1,400 sec/mm2 DWI reconstruction images. Data are given as mean ± standard deviation with range and medians in parentheses. P values are provided by Wilcoxon signed-rank test to compare values between DL1–3 and CDWI for each reader. DWI, diffusion weighted image; DLR, deep learning reconstruction; CDWI, conventional diffusion weighted image; DL1, DWI with iPAT2 using DLR; DL2, DWI with iPAT3 using DLR; DL3, DWI with iPAT3 and reduced averages per b-value using DLR; iPAT2, acceleration factor 2; iPAT3, acceleration factor 3.
For reader 2, a statistically significant improvement (P<0.05) in lesion conspicuity was also observed in the DL1, and DL2 images compared to CDWI images (mean conspicuity scores of CDWI, DL1, DL2: 2.9, 3.6, and 3.2, respectively). No significant difference in noise and overall image quality was observed in CDWI and DL1 images, while DL2 and DL3 images displayed significantly higher noise levels represented by lower noise assessment scores (P<0.05) (mean noise score CDWI, DL1, DL2, DL3: 3.8, 3.8, 3.7, 3.0; mean overall image quality score CDWI, DL1, DL2, DL3: 3.9, 3.9, 3.7, 3.6, respectively) (Figure 2).
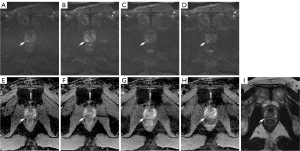
Quantitative assessment
In Table 4, the measurements of SNR, CNR, and ADC values of PCa lesions on each DWI are listed. The SNR, CNR values of all DLR images (DL1, DL2, DL3) was significantly higher than those of CDWI images (SNR: CDWI, 38.4±14.7, DL1, 56.9±21.0, DL2, 48.5±18.5, DL3, 42.5±14.7; CNR: CDWI, 25.1±11.5, DL1, 43.1±17.8, DL2, 36.3±15.8, DL3, 32.0±12.7; P<0.001). The mean ADC value was significantly higher in all DWI with DLR compared to CDWI (mean ADC value, 10−3 mm2/sec: CDWI, 650.5±143.2, DL1, 712.5±150.1, DL2, 748.7±153.8, DL3, 747.1±145.0, P<0.001).
Table 4
Measurements | CDWI (mean ± SD) |
DL1 | DL2 | DL3 | |||||
---|---|---|---|---|---|---|---|---|---|
Mean ± SD | P value | Mean ± SD | P value | Mean ± SD | P value | ||||
SNR | 38.4±14.7 | 56.9±21.0 | <0.001 | 48.5±18.5 | <0.001 | 42.5±14.7 | <0.001 | ||
CNR | 25.1±11.5 | 43.1±17.8 | <0.001 | 36.3±15.8 | <0.001 | 32.0±12.7 | <0.001 | ||
ADC value (×10−3 mm2/sec) | 650.5±143.2 | 712.5±150.1 | <0.001 | 748.7±153.8 | <0.001 | 747.1±145.0 | <0.001 | ||
*Pearson’s coefficient | 0.85 | 0.79 | 0.78 |
P values are provided by paired t-tests to compare values between DL1–3 and CDWI. *, Pearson’s coefficient between CDWI ADC values and DL1–3 ADC values are shown. CDWI, conventional diffusion weighted image; DL1, DWI with iPAT2 using DLR; DL2, DWI with iPAT3 using DLR; DL3, DWI with iPAT3 and reduced averages per b-value using DLR; SD, standard deviation; SNR, signal-to-noise ratio; CNR, contrast-to-noise ratio; ADC, apparent diffusion coefficient; DWI, diffusion weighted image; DLR, deep learning reconstruction; iPAT2, acceleration factor 2; iPAT3, acceleration factor 3.
PI-RADSv2.1 evaluation
Table 5 shows the distribution of DWI scores in PI-RADS v2.1 evaluation for each DWI. A majority of the lesions were scored as DWI score 5, while the portion increased with the implementation of DLR in both readers (CDWI, DL1, DL2: Reader 1 64.3%, 67.1%, 78.6% and Reader 2 54.3%, 68.6%, 62.9%, respectively). Inter-reader agreement of PI-RADS scoring was moderate to substantial with κ value ranging from 0.33–0.61, with DL1 showing the highest agreement (CDWI, DL1, DL2, DL3: 0.40, 0.61, 0.33, 0.50, respectively). DL3 images were excluded for statistical comparison of PI-RADS scoring, based on the aforementioned loss in overall image quality.
Table 5
DWI scores | Reader 1 | Reader 2 | |||||
---|---|---|---|---|---|---|---|
CDWI | DL1 | DL2 | CDWI | DL1 | DL2 | ||
5 | 45 (64.3) | 47 (67.1) | 55 (78.6) | 38 (54.3) | 48 (68.6) | 44 (62.9) | |
4 | 21 (30.0) | 22 (31.4) | 11 (15.7) | 17 (24.3) | 16 (22.9) | 17 (24.3) | |
3 | 4 (5.7) | 1 (1.4) | 4 (5.7) | 14 (20.0) | 6 (8.6) | 9 (12.9) | |
2 | 0 | 0 | 0 | 1 (1.4) | 0 | 0 | |
1 | 0 | 0 | 0 | 0 | 0 | 0 | |
Total | 70 | 70 | 70 | 70 | 70 | 70 |
Data are given as the number of cases (%). PI-RADS, Prostate Imaging-Reporting and Data System; DWI, diffusion weighted image; DLR, deep learning reconstruction; CDWI, conventional diffusion weighted image; DL1, DWI with iPAT2 using DLR; DL2, DWI with iPAT3 using DLR; iPAT2, acceleration factor 2; iPAT3, acceleration factor 3.
Among the 70 PCa patients enrolled in this study, a significant number of PI-RADS <4 lesions on CDWI images were upgraded to PI-RADS 4 or 5 in DL1 images by both readers as shown in Table 6 (P<0.05). Significant differences in the number of PI-RADS ≥4 lesions were also observed on DL2 images for reader 2 (P=0.03).
Table 6
PI-RADS scores | Reader 1 | Reader 2 | |||||||||
---|---|---|---|---|---|---|---|---|---|---|---|
DL1 | DL2 | DL1 | DL2 | ||||||||
PI-RADS <4 | PI-RADS ≥4 | PI-RADS <4 | PI-RADS ≥4 | PI-RADS <4 | PI-RADS ≥4 | PI-RADS <4 | PI-RADS ≥4 | ||||
CDWI | |||||||||||
PI-RADS <4 | 3 | 6 | 2 | 7 | 6 | 10 | 10 | 6 | |||
PI-RADS ≥4 | 0 | 61 | 2 | 59 | 1 | 53 | 0 | 54 | |||
P value | 0.03 | 0.18 | 0.01 | 0.03 |
Data are given as the number of cases. P values are provided by Mcnemar test for marginal homogeneity between DL1–2 and CDWI for each reader. PI-RADS, Prostate Imaging-Reporting and Data System; DL1, DWI with iPAT2 using DLR; DL2, DWI with iPAT3 using DLR; CDWI, conventional diffusion weighted image; DWI, diffusion weighted image; DLR, deep learning reconstruction; iPAT2, acceleration factor 2; iPAT3, acceleration factor 3.
Subgroup analysis was performed based on Gleason scores (high grade ≥7) and 2023 NCCN Guidelines unfavorable or high-risk groups. SNR and CNR did not show a significant difference, whereas the average ADC value was significantly lower in the Gleason score ≥7 high-grade group (n=51) compared to the Gleason score <7 low-grade group (n=19) in all DWI images (Table 7). Clinically significant PCa according to Gleason score grade did not show a statistically significant difference in marginal probability for PI-RADS scoring in DL1 and 2 as seen in Table 8. Also, subgroup analysis of unfavorable intermediate or higher risk groups (n=54) according to NCCN Guidelines is shown in Table 9. Interestingly, most of the previously mentioned PI-RADS upgraded lesions were from the higher risk subgroup with a statistically significant difference in marginal probability of PI-RADS scoring in DL1 and DL2 for both readers (P<0.05).
Table 7
Measurements | CDWI | DL1 | DL2 | DL3 | |||||||||||
---|---|---|---|---|---|---|---|---|---|---|---|---|---|---|---|
Gleason score ≥7 |
Gleason score <7 | P value | Gleason score ≥7 | Gleason score <7 | P value | Gleason score ≥7 | Gleason score <7 | P value | Gleason score ≥7 |
Gleason score <7 |
P value | ||||
SNR | 39.7±15.1 | 35.0±13.4 | 0.24 | 58.8±21.4 | 51.5±19.6 | 0.2 | 49.2±18.7 | 46.6±18.3 | 0.61 | 44.0±15.8 | 38.5±10.8 | 0.16 | |||
CNR | 26.6±11.5 | 21.3±11.0 | 0.09 | 45.2±17.9 | 37.5±16.7 | 0.11 | 37.4±16.1 | 33.2±14.8 | 0.32 | 33.7±13.5 | 27.4±9.2 | 0.07 | |||
ADC value (×10−3 mm2/sec) | 626.5±129.7 | 715.0±160.8 | 0.02 | 668.9±114.9 | 829.5±172.9 | 0.001 | 707.2±125.5 | 860.1±170.2 | 0.001 | 709.8±121.3 | 847.1±158.8 | <0.001 |
Data are given as mean ± standard deviation. P values are provided by independent t-test to compare values between Gleason score ≥7 and Gleason score <7 subgroups for each DWI. csPCa, clinically significant prostate cancer; CDWI, conventional diffusion weighted image; DL1, DWI with iPAT2 using DLR; DL2, DWI with iPAT3 using DLR; DL3, DWI with iPAT3 and reduced averages per b-value using DLR; SNR, signal-to-noise ratio; CNR, contrast-to-noise ratio; ADC, apparent diffusion coefficient; DWI, diffusion weighted imaging; DLR, deep learning reconstruction; iPAT2, acceleration factor 2; iPAT3, acceleration factor 3.
Table 8
PI-RADS scores | Reader 1 | Reader 2 | |||||||||
---|---|---|---|---|---|---|---|---|---|---|---|
DL1 | DL2 | DL1 | DL2 | ||||||||
PI-RADS <4 | PI-RADS ≥4 | PI-RADS <4 | PI-RADS ≥4 | PI-RADS <4 | PI-RADS ≥4 | PI-RADS <4 | PI-RADS ≥4 | ||||
CDWI | |||||||||||
PI-RADS <4 | 1 | 5 | 1 | 5 | 5 | 7 | 8 | 4 | |||
PI-RADS ≥4 | 0 | 45 | 1 | 44 | 1 | 38 | 0 | 39 | |||
P value | 0.06 | 0.22 | 0.07 | 0.13 |
Data are given as the number of cases. P values are provided by Mcnemar test for marginal homogeneity between DL1–2 and CDWI for each reader. PI-RADS, Prostate Imaging-Reporting and Data System; csPCa, clinically significant prostate cancer; DL1, DWI with iPAT2 using DLR; DL2, DWI with iPAT3 using DLR; CDWI, conventional diffusion weighted image; DWI, diffusion weighted imaging; DLR, deep learning reconstruction; iPAT2, acceleration factor 2; iPAT3, acceleration factor 3.
Table 9
PI-RADS scores | Reader 1 | Reader 2 | |||||||||
---|---|---|---|---|---|---|---|---|---|---|---|
DL1 | DL2 | DL1 | DL2 | ||||||||
PI-RADS <4 | PI-RADS ≥4 | PI-RADS <4 | PI-RADS ≥4 | PI-RADS <4 | PI-RADS ≥4 | PI-RADS <4 | PI-RADS ≥4 | ||||
CDWI | |||||||||||
PI-RADS <4 | 1 | 6 | 2 | 6 | 3 | 8 | 5 | 6 | |||
PI-RADS ≥4 | 0 | 47 | 0 | 46 | 1 | 42 | 0 | 43 | |||
P value | 0.03 | 0.03 | 0.04 | 0.03 |
Data are given as the number of cases. P values are provided by Mcnemar test for marginal homogeneity between DL1–2 and CDWI for each reader. PI-RADS, Prostate Imaging-Reporting and Data System; NCCN, National Comprehensive Cancer Network; DL1, DWI with iPAT2 using DLR; DL2, DWI with iPAT3 using DLR; CDWI, conventional diffusion weighted image; DWI, diffusion weighted imaging; DLR, deep learning reconstruction; iPAT2, acceleration factor 2; iPAT3, acceleration factor 3.
Location matching
The sensitivity of MRI to detect any-grade cancer or clinically significant cancer (Gleason score ≥7) as PI-RADS ≥4 using location matching is shown in Table 10. Only the sensitivity is listed, as all patients included in this study had biopsy-proven PCa. The sensitivity of location matching between systemic biopsy specimens and clinically significant cancer ranged between 78.4–86.3%.
Table 10
Sensitivity | Reader 1 | Reader 2 | |||||||
---|---|---|---|---|---|---|---|---|---|
CDWI | DL1 | DL2 | DL3 | CDWI | DL1 | DL2 | DL3 | ||
Any prostate cancer, sensitivity (%) | 71.4 | 67.1 | 75.7 | 70.0 | 64.3 | 72.9 | 72.9 | 68.6 | |
csPCa, sensitivity (%) | 80.4 | 78.4 | 86.3 | 82.4 | 78.4 | 84.3 | 86.3 | 84.3 | |
95% CI | 66.9–90.2 | 64.7–88.7 | 73.7–94.3 | 69.1–91.6 | 66.9–90.2 | 64.7–88.7 | 73.7–94.3 | 69.1–91.6 |
MRI, magnetic resonance imaging; csPCa, clinically significant prostate cancer (Gleason score ≥3+4); CDWI, conventional diffusion weighted image; DL1, DWI with iPAT2 using DLR; DL2, DWI with iPAT3 using DLR; DL3, DWI with iPAT3 and reduced averages per b-value using DLR; CI, confidence interval; DWI, diffusion weighted imaging; DLR, deep learning reconstruction; iPAT2, acceleration factor 2; iPAT3, acceleration factor 3.
Discussion
Our study shows that the use of DLR in DWI of PCa allows for better image quality with better lesion conspicuity, or faster acquisition without significant difference in image quality. Moreover, the application of DLR in DWI resulted in better SNR and CNR of PCa lesions in all cases compared to CDWI. The improvements in image quality, lesion conspicuity, SNR, and CNR in DL1 resulted in a better inter-reader agreement and upgrading of PCa lesions from PI-RADS <4 to PI-RADS ≥4 csPCa. Therefore, our study has demonstrated the successful implementation of DLR in prostate DWI and its clinical relevance in assessing PCa lesions with substantial agreement between readers of varying clinical experience.
To increase sensitivity, the most recent version of PI-RADS v2.1 recommends using DWI images with a high b-value greater than 1,400 sec/mm2 to improve the conspicuity of cancer lesions (26,27). Although high b-values greater than 2,000 sec/mm2 may improve the sensitivity of tumor detection, previous studies have reported a significant loss in image quality (28) and a significant SNR (29). However, the DLR methods applied in our study produced DWI of PCa with better lesion conspicuity in DL1 and DL2 images, and better SNR and CNR in all iPAT2 and iPAT3-based images. This is in accordance with previously reported implementations of DLR methods in DWI showing higher SNR and better image quality in assessing PCa (19), intra-abdominal organs (17), and hepatic mass (17,30), while a study on breast cancer DWI DLR showed no significant increase in SNR but increase in CNR (31). Due to its signal scarcity, the trade-off between high b-value and SNR is an intrinsic challenge in DWI, and signal averaging is traditionally used for better SNR and noise reduction as opposed to higher parallel imaging acceleration factors. However, signal averaging is time-consuming as acquisition time is proportional to the number of signals averaged. As DWI naturally contributes considerably to overall scan time, echoplanar imaging in combination with parallel imaging, compressed sensing, and DLR are important techniques for scan time reduction in DWI while maintaining significant image quality (32). In this study, the role of DLR in overcoming such image quality trade-offs has been carefully examined by applying different acceleration parameters in combination with DLR. Better lesion conspicuity, SNR, and CNR in the DL2 scan of this study have shown that DLR can be successfully implemented in DWI to increase parallel imaging acceleration factor up to 3 without compromising image quality or lesion characterization.
To note, an increase in SNR was seen despite the higher mean ADC values of cancer lesions on DLR images (DL1, DL2, and DL3) than on CDWI in this study. So far, the effects of DLR on ADC values have not been thoroughly investigated, as there have been contradictory reports of higher ADC values (30,33), of no significant differences (19,31), or of lower ADC values (17) in lesions on DLR images. Previous reports concerning DLR have attributed the differences in ADC values to limitations in SNR in high b-value images above 3,000 sec/mm2 (19), and differences in imaging protocols such as different fat saturation techniques, acceleration factors, and signal averages (17) or higher ADC values due to increased signal losses due to cardiac motion in the left hepatic lobe (30). In this study, the trend of higher ADC values with DLR imaging was consistent in all DLR images and was statistically significant, especially with no change in imaging parameters between CDWI and DL1. To note, the increase in ADC value between conventional images and DLR images was less than 100×10−3 mm2/sec, while analysis with Pearson’s coefficient showed that the values were increased proportionately. Although the reason for the increase in ADC values with DLR is yet to be validated, results of this study indicate that such an increase in ADC value had not significantly counteracted the improvement in SNR, CNR, and image quality in high b-value images.
The findings of our study also revealed that the DL1 reconstruction method for DWI can improve the inter-reader agreement of PI-RADS v2.1 evaluation to a substantial level (κ=0.61), compared to the moderate agreement observed in other reconstruction methods in this study (κ value range 0.33–0.50) and previous literature (12). Specifically, this was achieved regardless of the large differences in the clinical experiences of the two readers in this study. Similarly, a recent study demonstrated that inter-reader agreement and the level of confidence for PI-RADS v2.1 scoring was higher with better image quality assessed by the prostate imaging quality (PI-QUAL) score, regardless of the reader’s clinical experience (34). In addition, a meta-analysis of PI-RADS v2.1 assessment has revealed that inter-reader agreement declines especially with the inclusion of transitional zone PCa, and PI-RADS score 3 lesions (35). In our study, inter-reader agreement maintained a substantial level with DL1 images even with the inclusion of PI-RADS score 3 lesions. The improvement in inter-reader agreement in combination with lesion conspicuity, SNR, and CNR may be helpful in better localization of suspicious lesions on pre-biopsy MRI and targeted biopsy.
Over the years, it has been observed that surgically confirmed PCa with large tumor volume ≥0.5 cm3, Gleason score ≥7, or the presence of extra-prostatic extension is clinically significant. According to the recent version of PI-RADS v2.1, the detection rate of csPCa is over 90% for PI-RADS 5 lesions (36), 22–60% for PI-RADS 4 lesions (37), and only up to 12% for PI-RADS 3 lesions (38). In this regard, clinical implications of our study are suggested, as evaluation with DL1 DWI resulted in the upgrading of PI-RADS <4 lesions on conventional images to clinically significant tumors (PI-RADS 4 or 5), prompting further management. The reason for the difference in evaluation from PI-RADS <4 to PI-RADS 4 or 5 was largely due to DWI scores of 3 in CDWI being upgraded to DWI 4 or 5 in DLR DWI, possibly due to a better correlation of diffusion restriction of lesions in both the ADC map and high b-value images owing to improvements in SNR, CNR and image quality. An example of such an upgrade in PI-RADS assessment is demonstrated in Figure 2, in which an increase in SNR from 16.9 in CDWI to high SNR in DL1–3 (27.8, 21.0, 21.1, respectively) led to better correlation of the lesion in high b-value and ADC map images. This resulted in a change of PI-RADS score 3 to PI-RADS score 5 after considering the DLR images, and the lesion was pathologically confirmed as Gleason score 8 csPCa. These results suggest that the observed improvements in lesion conspicuity, SNR, and CNR on DL1 images can aid in better characterization of PCa lesions on DWI. To date, only one pilot study has investigated the clinical impact of using DLR DWI for PI-RADS scoring, resulting in no significant difference between CDWI (39). A bladder cancer study on another radiological reporting system which also relies heavily on DWI, showed that using DLR might further improve the diagnostic accuracy for determining the Vesical Imaging Reporting and Data System (VI-RADS) and the presence of muscle invasion (40). Furthermore, without change in PI-RADS ≥4 scoring, DWI score changes from 4 to 5 were observed in up to 10 patients in DLR images. This was largely due to increased measurement of size on DLR DWI. Further investigation with surgical specimens is needed in the future to determine whether DLR images may overestimate the size of lesions or aid in determining extra-prostatic extension or T staging.
Lastly, the current management of PCa is a complex decision taking into account various clinical factors such as life PSA level, clinical T staging, and Gleason score groups defined by the ISUP. 2023 NCCN Guidelines for PCa suggest categorizing patients into 5 risk groups from very low to very high to aid decisions in treatment. According to these guidelines, higher than unfavorable intermediate risk and longer life expectancy warrants a more aggressive initial treatment such as radical prostatectomy or radiotherapy as opposed to active surveillance in low-risk groups. In this study, the upgrade to PI-RADS ≥4 with the use of DLR on DWI was seen mostly in these higher-risk groups according to NCCN Guidelines. Thus, upgrading to PI-RADS ≥4 may be correlated with the need for more aggressive treatments rather than active surveillance, which needs to be investigated further in future studies.
This was a retrospective study of patients with a relatively small sample size. The prostate MRI scans were taken post-biopsy and this study included patients confirmed with PCa, which leaves room for a positive selection bias. In the conventional diagnostic workflow, MRI has been reserved mainly for further staging of extra-prostatic lesions rather than for the diagnosis or localization of PCa. However, due to the increasing emphasis on pre-biopsy MRI, further study with the inclusion of patients with intermediate clinical suspicion for cancer and the screening population is needed to investigate the full clinical implications of DL image reconstruction and diagnostic performance of assessing csPCa.
Moreover, although the sensitivity of location matching between TRUS biopsy-confirmed lesions and PI-RADS ≥4 lesions determined on MRI was comparable to previous literature (24), the lack of surgical resection in the majority of patients may have allowed for sampling error and/or operator bias in the histological grading and localization of tumors in our study. Ghosting artifacts were observed in DL2 and DL3 images in the pilot scans, which were fixed by adjusting the field of view. Additionally, long-term follow-up data of the patients were not available to investigate its effects on clinical outcomes. Finally, further investigations and study designs are warranted for statistical validation of the non-inferiority of DL2 images compared to CDWI.
Conclusions
Despite the limitations, the results of implicating DLR for prostate DWI in our study have shown significant improvement in lesion conspicuity, SNR, CNR, inter-reader agreement of PI-RADS evaluation, and more clinically relevant PI-RADS scoring (PI-RADS score ≥4) for malignant lesions using iPAT2 images (DL1), without additional scan time. Moreover, DWI with iPAT3 (DL2) resulted in a 23% reduction in scan time compared to conventional imaging and better lesion conspicuity, without compromising consistent reporting of PI-RADS v2.1 evaluation. These results can be employed by urologists and radiologists in selecting imaging reconstruction methods for better characterization of lesions (DL1), or faster (DL2) scan methods in screening and for incorporating pre-biopsy prostate MRI in the diagnostic workflow. Moreover, better inter-reader agreement of PI-RADS scoring along with lesion conspicuity may help localize lesions in pre-biopsy MRI aiding targeting biopsy. Lastly, further investigation is needed for the clinical implications of upgraded PI-RADS lesions and their correlation with higher NCCN Guidelines risk groups.
Acknowledgments
Funding: None.
Footnote
Reporting Checklist: The authors have completed the STARD reporting checklist. Available at https://qims.amegroups.com/article/view/10.21037/qims-23-1379/rc.
Conflicts of Interest: All authors have completed the ICMJE uniform disclosure form (available at https://qims.amegroups.com/article/view/10.21037/qims-23-1379/coif). C.H.L. serves as an unpaid editorial board member of Quantitative Imaging in Medicine and Surgery. T.B. and E.W. are employees of Siemens Healthineers, GmbH and work in the department for MR Application Predevelopment. T.B. and E.W. provided technical information only. I.K. is an employee of Siemens Healthcare, Ltd., and work in the department for MR application Predevelopment. I.K. provided technical information only. The other authors have no conflicts of interest to declare.
Ethical Statement: The authors are accountable for all aspects of the work in ensuring that questions related to the accuracy or integrity of any part of the work are appropriately investigated and resolved. The study was conducted in accordance with the Declaration of Helsinki (as revised in 2013). The study was approved by the institutional review board of Korea University Ansan Hospital in the Republic of Korea (IRB No. 2023AS0046), and individual consent for this retrospective analysis was waived.
Open Access Statement: This is an Open Access article distributed in accordance with the Creative Commons Attribution-NonCommercial-NoDerivs 4.0 International License (CC BY-NC-ND 4.0), which permits the non-commercial replication and distribution of the article with the strict proviso that no changes or edits are made and the original work is properly cited (including links to both the formal publication through the relevant DOI and the license). See: https://creativecommons.org/licenses/by-nc-nd/4.0/.
References
- Bray F, Ferlay J, Soerjomataram I, Siegel RL, Torre LA, Jemal A. Global cancer statistics 2018: GLOBOCAN estimates of incidence and mortality worldwide for 36 cancers in 185 countries. CA Cancer J Clin 2018;68:394-424. [Crossref] [PubMed]
- Mottet N, van den Bergh RCN, Briers E, Van den Broeck T, Cumberbatch MG, De Santis M, et al. EAU-EANM-ESTRO-ESUR-SIOG Guidelines on Prostate Cancer-2020 Update. Part 1: Screening, Diagnosis, and Local Treatment with Curative Intent. Eur Urol 2021;79:243-62. [Crossref] [PubMed]
- Sandhu S, Moore CM, Chiong E, Beltran H, Bristow RG, Williams SG. Prostate cancer. Lancet 2021;398:1075-90. [Crossref] [PubMed]
- Epstein JI, Walsh PC, Carmichael M, Brendler CB. Pathologic and clinical findings to predict tumor extent of nonpalpable (stage T1c) prostate cancer. JAMA 1994;271:368-74.
- Epstein JI, Egevad L, Amin MB, Delahunt B, Srigley JR, Humphrey PA. The 2014 International Society of Urological Pathology (ISUP) Consensus Conference on Gleason Grading of Prostatic Carcinoma: Definition of Grading Patterns and Proposal for a New Grading System. Am J Surg Pathol 2016;40:244-52. [Crossref] [PubMed]
- Referenced with permission from the NCCN Clinical Practice Guidelines in Oncology (NCCN Guidelines®) for Prostate Cancer V.2.2024. ©National Comprehensive Cancer Network, Inc. 2024. All rights reserved. Accessed March 7, 2024. To view the most recent and complete version of the guideline, go online to NCCN.org. NCCN makes no warranties of any kind whatsoever regarding their content, use or application and disclaims any responsibility for their application or use in any way. Available online: https://www.nccn.org/guidelines/guidelines-detail?category=1&id=1459
- Gresser E, Schachtner B, Stüber AT, Solyanik O, Schreier A, Huber T, Froelich MF, Magistro G, Kretschmer A, Stief C, Ricke J, Ingrisch M, Nörenberg D. Performance variability of radiomics machine learning models for the detection of clinically significant prostate cancer in heterogeneous MRI datasets. Quant Imaging Med Surg 2022;12:4990-5003. [Crossref] [PubMed]
- Winkel DJ, Breit HC, Shi B, Boll DT, Seifert HH, Wetterauer C. Predicting clinically significant prostate cancer from quantitative image features including compressed sensing radial MRI of prostate perfusion using machine learning: comparison with PI-RADS v2 assessment scores. Quant Imaging Med Surg 2020;10:808-23. [Crossref] [PubMed]
- Purysko AS, Baroni RH, Giganti F, Costa D, Renard-Penna R, Kim CK, Raman SS. PI-RADS Version 2.1: A Critical Review, From the AJR Special Series on Radiology Reporting and Data Systems. AJR Am J Roentgenol 2021;216:20-32. [Crossref] [PubMed]
- Herrmann J, Gassenmaier S, Nickel D, Arberet S, Afat S, Lingg A, Kündel M, Othman AE. Diagnostic Confidence and Feasibility of a Deep Learning Accelerated HASTE Sequence of the Abdomen in a Single Breath-Hold. Invest Radiol 2021;56:313-9. [Crossref] [PubMed]
.Han YS Yoo J Ye JC Deep Residual Learning for Compressed Sensing CT Reconstruction via Persistent Homology Analysis. arXiv:1611.06391.- Lee CH, Vellayappan B, Tan CH. Comparison of diagnostic performance and inter-reader agreement between PI-RADS v2.1 and PI-RADS v2: systematic review and meta-analysis. Br J Radiol 2022;95:20210509. [Crossref] [PubMed]
- Gassenmaier S, Küstner T, Nickel D, Herrmann J, Hoffmann R, Almansour H, Afat S, Nikolaou K, Othman AE. Deep Learning Applications in Magnetic Resonance Imaging: Has the Future Become Present? Diagnostics (Basel) 2021.
- Gassenmaier S, Afat S, Nickel D, Mostapha M, Herrmann J, Othman AE. Deep learning-accelerated T2-weighted imaging of the prostate: Reduction of acquisition time and improvement of image quality. Eur J Radiol 2021;137:109600.
- Gassenmaier S, Afat S, Nickel MD, Mostapha M, Herrmann J, Almansour H, Nikolaou K, Othman AE. Accelerated T2-Weighted TSE Imaging of the Prostate Using Deep Learning Image Reconstruction: A Prospective Comparison with Standard T2-Weighted TSE Imaging. Cancers (Basel) 2021.
- Almansour H, Gassenmaier S, Nickel D, Kannengiesser S, Afat S, Weiss J, Hoffmann R, Othman AE. Deep Learning-Based Superresolution Reconstruction for Upper Abdominal Magnetic Resonance Imaging: An Analysis of Image Quality, Diagnostic Confidence, and Lesion Conspicuity. Invest Radiol 2021;56:509-16. [Crossref] [PubMed]
- Bae SH, Hwang J, Hong SS, Lee EJ, Jeong J, Benkert T, Sung J, Arberet S. Clinical feasibility of accelerated diffusion weighted imaging of the abdomen with deep learning reconstruction: Comparison with conventional diffusion weighted imaging. Eur J Radiol 2022;154:110428. [Crossref] [PubMed]
- Kim DH, Kim B, Lee HS, Benkert T, Kim H, Choi JI, Oh SN, Rha SE. Deep Learning-Accelerated Liver Diffusion-Weighted Imaging: Intraindividual Comparison and Additional Phantom Study of Free-Breathing and Respiratory-Triggering Acquisitions. Invest Radiol 2023;58:782-90. [Crossref] [PubMed]
- Ueda T, Ohno Y, Yamamoto K, Murayama K, Ikedo M, Yui M, Hanamatsu S, Tanaka Y, Obama Y, Ikeda H, Toyama H. Deep Learning Reconstruction of Diffusion-weighted MRI Improves Image Quality for Prostatic Imaging. Radiology 2022;303:373-81. [Crossref] [PubMed]
- Hammernik K, Klatzer T, Kobler E, Recht MP, Sodickson DK, Pock T, Knoll F. Learning a variational network for reconstruction of accelerated MRI data. Magn Reson Med 2018;79:3055-71. [Crossref] [PubMed]
- Shi W, Caballero J, Huszár F, Totz J, Aitken AP, Bishop R, Rueckert D, Wang Z. Real-Time Single Image and Video Super-Resolution Using an Efficient Sub-Pixel Convolutional Neural Network. 2016 IEEE Conference on Computer Vision and Pattern Recognition (CVPR), Las Vegas, NV, USA, 2016:1874-83.
- Vranic JE, Cross NM, Wang Y, Hippe DS, de Weerdt E, Mossa-Basha M. Compressed Sensing-Sensitivity Encoding (CS-SENSE) Accelerated Brain Imaging: Reduced Scan Time without Reduced Image Quality. AJNR Am J Neuroradiol 2019;40:92-8. [Crossref] [PubMed]
- Taneja SS, Bjurlin MAH, Carter BH, Cookson M, Gomella LG, Penson DF, Schellhammer P, Schlossberg SM, Troyer DA, Wheeler T, Stinchcomb SN. AUA/Optimal Techniques of Prostate Biopsy and Specimen Handling. 2013. Accessed on 10 May 2023. Available online: https://www.auanet.org/Documents/education/clinical-guidance/Prostate-Biopsy-WhitePaper.pdf
- Gavin DJ, Kam J, Krelle M, Louie-Johnsun M, Sutherland T, Koschel S, Jenkins M, Yuminaga Y, Kim R, Aluwihare K, Skinner S, Brennan J, Wong LM. Quantifying the Effect of Location Matching on Accuracy of Multiparametric Magnetic Resonance Imaging Prior to Prostate Biopsy-A Multicentre Study. Eur Urol Open Sci 2020;20:28-36. [Crossref] [PubMed]
- Svanholm H, Starklint H, Gundersen HJ, Fabricius J, Barlebo H, Olsen S. Reproducibility of histomorphologic diagnoses with special reference to the kappa statistic. APMIS 1989;97:689-98. [Crossref] [PubMed]
- Barentsz JO, Richenberg J, Clements R, Choyke P, Verma S, Villeirs G, Rouviere O, Logager V, Fütterer JJ. ESUR prostate MR guidelines 2012. Eur Radiol 2012;22:746-57. [Crossref] [PubMed]
- Turkbey B, Rosenkrantz AB, Haider MA, Padhani AR, Villeirs G, Macura KJ, Tempany CM, Choyke PL, Cornud F, Margolis DJ, Thoeny HC, Verma S, Barentsz J, Weinreb JC. Prostate Imaging Reporting and Data System Version 2.1: 2019 Update of Prostate Imaging Reporting and Data System Version 2. Eur Urol 2019;76:340-51. [Crossref] [PubMed]
- Feng Z, Min X, Margolis DJ, Duan C, Chen Y, Sah VK, Chaudhary N, Li B, Ke Z, Zhang P, Wang L. Evaluation of different mathematical models and different b-value ranges of diffusion-weighted imaging in peripheral zone prostate cancer detection using b-value up to 4500 s/mm2. PLoS One 2017;12:e0172127. [Crossref] [PubMed]
- Kitajima K, Kaji Y, Kuroda K, Sugimura K. High b-value diffusion-weighted imaging in normal and malignant peripheral zone tissue of the prostate: effect of signal-to-noise ratio. Magn Reson Med Sci 2008;7:93-9. [Crossref] [PubMed]
- Afat S, Herrmann J, Almansour H, Benkert T, Weiland E, Hölldobler T, Nikolaou K, Gassenmaier S. Acquisition time reduction of diffusion-weighted liver imaging using deep learning image reconstruction. Diagn Interv Imaging 2023;104:178-84. [Crossref] [PubMed]
- Lee EJ, Chang YW, Sung JK, Thomas B. Feasibility of deep learning k-space-to-image reconstruction for diffusion weighted imaging in patients with breast cancers: Focus on image quality and reduced scan time. Eur J Radiol 2022;157:110608. [Crossref] [PubMed]
- Filli L, Ghafoor S, Kenkel D, Liu W, Weiland E, Andreisek G, Frauenfelder T, Runge VM, Boss A. Simultaneous multi-slice readout-segmented echo planar imaging for accelerated diffusion-weighted imaging of the breast. Eur J Radiol 2016;85:274-8. [Crossref] [PubMed]
- Ursprung S, Herrmann J, Joos N, Weiland E, Benkert T, Almansour H, Lingg A, Afat S, Gassenmaier S. Accelerated diffusion-weighted imaging of the prostate using deep learning image reconstruction: A retrospective comparison with standard diffusion-weighted imaging. Eur J Radiol 2023;165:110953. [Crossref] [PubMed]
- Forookhi A, Laschena L, Pecoraro M, Borrelli A, Massaro M, Dehghanpour A, Cipollari S, Catalano C, Panebianco V. Bridging the experience gap in prostate multiparametric magnetic resonance imaging using artificial intelligence: A prospective multi-reader comparison study on inter-reader agreement in PI-RADS v2.1, image quality and reporting time between novice and expert readers. Eur J Radiol 2023;161:110749. [Crossref] [PubMed]
- Wen J, Ji Y, Han J, Shen X, Qiu Y. Inter-reader agreement of the prostate imaging reporting and data system version v2.1 for detection of prostate cancer: A systematic review and meta-analysis. Front Oncol 2022;12:1013941. [Crossref] [PubMed]
- Cash H, Maxeiner A, Stephan C, Fischer T, Durmus T, Holzmann J, Asbach P, Haas M, Hinz S, Neymeyer J, Miller K, Günzel K, Kempkensteffen C. The detection of significant prostate cancer is correlated with the Prostate Imaging Reporting and Data System (PI-RADS) in MRI/transrectal ultrasound fusion biopsy. World J Urol 2016;34:525-32. [Crossref] [PubMed]
- Mehralivand S, Bednarova S, Shih JH, Mertan FV, Gaur S, Merino MJ, Wood BJ, Pinto PA, Choyke PL, Turkbey B. Prospective Evaluation of PI-RADS™ Version 2 Using the International Society of Urological Pathology Prostate Cancer Grade Group System. J Urol 2017;198:583-90. [Crossref] [PubMed]
- Mertan FV, Greer MD, Shih JH, George AK, Kongnyuy M, Muthigi A, Merino MJ, Wood BJ, Pinto PA, Choyke PL, Turkbey B. Prospective Evaluation of the Prostate Imaging Reporting and Data System Version 2 for Prostate Cancer Detection. J Urol 2016;196:690-6. [Crossref] [PubMed]
- Johnson PM, Tong A, Donthireddy A, Melamud K, Petrocelli R, Smereka P, Qian K, Keerthivasan MB, Chandarana H, Knoll F. Deep Learning Reconstruction Enables Highly Accelerated Biparametric MR Imaging of the Prostate. J Magn Reson Imaging 2022;56:184-95. [Crossref] [PubMed]
- Taguchi S, Tambo M, Watanabe M, Machida H, Kariyasu T, Fukushima K, Shimizu Y, Okegawa T, Yokoyama K, Fukuhara H. Prospective Validation of Vesical Imaging-Reporting and Data System Using a Next-Generation Magnetic Resonance Imaging Scanner-Is Denoising Deep Learning Reconstruction Useful? J Urol 2021;205:686-92. [Crossref] [PubMed]