Experimental and clinical validation of an artificial intelligence metal artifact correction algorithm for low-dose following up CT of percutaneous vertebroplasty
Introduction
Osteoporotic vertebral compression fracture is a rather common issue with the elderly population. Patients may suffer from severe pain in the lower back, limited mobility, and kyphosis, while some have asymptomatic spinal canal compromise or posterior vertebral wall disruption (1). The minimally invasive interventions include percutaneous vertebroplasty (PVP) and kyphoplasty, where the polymethyl methacrylate bone cement is used to restore stability of fractured vertebral bodies and reduce pain. The reinforcing effect is immediate and obvious such that these surgeries are becoming a routine procedure (2-4). However, complications are being found, some of which may even lead to paraplegia and death. Those reported most often are re-collapse of the cemented vertebrae, pulmonary embolism, as well as soft tissue damage and nerve root compression that are related to the extravasation of bone cement (5-9). Moreover, a growing number of studies suggest that vertebral compression fracture is both a cause and a consequence of sarcopenia, which manifests as a reduction of density and strength in skeletal muscles (10).
As such, it is important to be able to continuously monitor the postoperative state of the bone cement as well as the soft tissue surrounding the surgical site. Computed tomography (CT), in this circumstance, may be the most accessible and convenient option for the following up observation. The only problem is that bone cement in clinical use may contain various radiopaque agents, such as barium sulfate, zirconium dioxide, tungsten, tantalum, iodinated methacrylate, etc., which are slightly more attenuating compared to the bony tissue (11). In CT imaging, the property of such materials compares to lightweight metal implants. These could result in streak artifacts due to beam hardening, making it difficult or impossible for detailed evaluation of the cemented region.
To tackle with the metal artifacts in CT, both the interpolation-based metal artifact correction (MAC) algorithms and the dual-energy CT (DECT)-based optimal virtual mono-energetic imaging have been proven to be useful (12). In case of the bone cement, MAC and DECT are supposed to be sufficient. Unfortunately, due to the high frequency of postoperative follow-up, radiologists often recommend low-dose CT to reduce the long-term radiation burden for patients. This is a problem for the conventional MAC and for DECT because increased noise could certainly affect the interpolation and the decomposition processing (13,14). Those circumstances make doctors difficult to comprehensively consider radiation burden, image quality, and frequency of following up. Since very few studies on CT metal artifacts dealt with low dose, the artifacts caused by bone cement in following up examinations remain a specific point of challenge.
Recently, an artificial intelligence (AI)-based MAC (AI-uMAC, United Imaging Healthcare, Shanghai, China) algorithm was introduced, which was developed using the deep-learning technique and was in principle different than the conventional MAC (15). It might be a potentially better solution in low-dose CT scenarios. This study aimed to validate the capability of this AI-based MAC in dealing with the artifacts caused by bone cement in low-dose following up CT. The first-step validation was conducted using an experimental phantom, where it was easy to establish the ground truth and was possible to make side-by-side comparison of the stages prior to and after the cemented surgery. The second step involved a patient test such that the diagnostic impact of the obtained CT images was able to be evaluated in terms of the reliability to help identify a common complication following PVP, which is the sarcopenia. We present this article in accordance with the STARD reporting checklist (available at https://qims.amegroups.com/article/view/10.21037/qims-23-1645/rc).
Methods
Phantom design
As shown in Figure 1, the vertebral phantom was designed in three main parts: one segment of ovine vertebra, a water base, and a cylindrical insert as a holder within the base. The vertebra segment (approx. 330 g) was obtained on the day of slaughter from a 1-year-old ovine. Bones and paraspinal muscles were kept as intact as possible without any chemical treatment. The drilling was 10.0 mm in diameter, made through the vertebra body in a direction vertical to the intervertebral disc. It was then filled with 2.5 mL fluid polymethyl methacrylate bone cement (Vertecem V+, Johnson Medical, New Jersey, USA). To maximally simulate the extravasation of bone cement, we injected approximately 2.5 mL of extra bone cement into the paraspinal muscle next to the vertebra. The processed vertebra was allowed to stand still for 15 minutes at room temperature until the bone cement was completely cured and cooled. Based on the average waist circumference among patients with a high risk of vertebral fracture, the water base was made 36.0 cm in diameter to simulate the abdominal shape (16). The size of the cylindrical insert was designed to fit the vertebra segment. Both the water base and the cylindrical insert were three-dimensional (3D) printed using a stereolithographic 3D printer with photosensitive resin (17). Phantom experiments were performed under a project license (No. SH9H-2021-T397-2) granted by the Institution Review Board of Shanghai Ninth People’s Hospital for involving animal specimen, in compliance with institutional guidelines for the use of animal specimen.
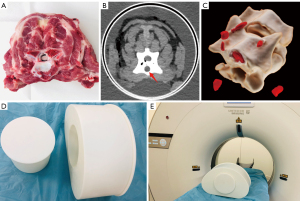
Phantom image acquisition
The scan was performed on a 64-row CT (uCT 760, United Imaging Healthcare, Shanghai, China). Two different protocols were used, one with routine dose before the PVP in order to establish a reference, and one with low dose after the injection as in clinical following up examinations of PVP. The parameters in routine dose scan were: 100 kVp tube voltage, 200 mAs reference tube current with automatic modulation, 40 mm beam collimation, 0.5 s rotation time, and 1.0 helical pitch. The volume CT dose index (CTDIvol) was 9.19 mGy. The different settings for low dose scans were: 100 kVp tube voltage and 40 mAs reference tube current with automatic modulation. The CTDIvol was 1.95 mGy, which was approximately 20% of the routine dose.
The routine dose image, i.e., the preoperative reference, was reconstructed with the routine hybrid iterative reconstruction (HIR) algorithm (Karl 3D, United Imaging Healthcare, Shanghai, China). The low dose images, i.e., the following up, were reconstructed using the same HIR with two correction algorithm: the conventional MAC (uMAC, United Imaging Healthcare, Shanghai, China) and the AI-MAC. The conventional MAC followed a similar methodology to that reported by Meyer et al. (18).
Greater details of the AI-MAC algorithm are available in (15), where the development of the algorithm was to establish a deep learning model with a prepared hybrid set of image data. Briefly speaking, a CT image without metal artifacts is combined with a theoretical implant model to create an artifact-free, mixed image. This mixed image is categorized into bone, implant, and soft tissue, and is projected forward using a polychromatic spectrum to simulate the generation of metal artifacts in a physical manner; it is then reconstructed with and without conventional MAC to form two input datasets showing artifacts of different degrees. Meanwhile, the mixed image is mathematically directly forward projected and reconstructed to generate an ideal, “artifact-corrected” dataset, serving as the training target. The deep learning model, a modified U-Net architecture, uses these datasets to learn artifact correction.
All images were reconstructed with a slice thickness and slice increment of 1.0 mm using a medium-smooth kernel.
Quantitative analysis
The following up images of the phantom was compared to the reference image in the CT attenuation, image noise, signal-to-noise ratio (SNR), as well as contrast-to-noise ratio (CNR). Image noise was characterized by the standard deviation (SD) within the specified region of interest (ROI). A radiologist with 7 years of experience was invited to delineate two ROIs surrounding the cement injection site. One ROI was set on the trabecular bone and the other on the paraspinal muscle. Both ROIs were approx. 60 mm2 in size. Each ROI was measured 6 times across slices where the bone cement was most visible. The SNR was calculated as:
The CNR between the bone and the soft tissue was calculated as:
where µ and SD represent the mean and the SD of the CT attenuation within the ROI, respectively, in Hounsfield units (HU).
Analysis of artifact correction accuracy
The correction accuracy of MAC and AI-MAC was further compared for their ability to preserve the muscular region surrounding the bone and the injected cement, which was quantified in terms of the segmented volume of the paraspinal muscle. The segmentation was carried out with adaptive thresholding on the post-processing workstation. The range of CT attenuation for muscle was measured on the reference image, where no cement was present, and was initially determined to be 18–80 HU. Segmentation was achieved via 3D region growing. The seed point was placed on the paraspinal muscle that was determined to be locally free of artifacts. The volume of interest was cuboid-shaped and included the entire phantom with the water-filled cylindrical insert. The number of voxels with a CT attenuation falling within the defined threshold range was counted, giving a quantitative measurement of the volume of paraspinal muscle in the image. The comparison was made with respect to both the reference image and the following up images with and without correction, where the same segmentation was conducted.
Patient study
Patient study was conducted in accordance with the Declaration of Helsinki (as revised in 2013) and was approved by the Institution Review Board of Shanghai Ninth People’s Hospital for patient data research (No. SH9H-2021-T258-2). Individual consent for this retrospective analysis was waived.
For clinical validation, a total of ten cases of low-dose following up CT after PVP, where the raw CT data remained available for additional reconstructions, were found between March and June 2023 and were retrospectively enrolled for investigation. The low-dose CT images, acquired with the same CT protocol as in the phantom experiment above, were tested for its reliability in diagnosing sarcopenia. Specifically, the CT attenuation was measured on the posterior paraspinal muscle and compared against a predetermined threshold below which the case, given the recent history of PVP, was categorized as sarcopenia. This method was based on the fact that CT attenuation has a strong correlation to radiodensity (19-22) and was proven effective with a threshold value of 30.0 HU in previous studies (23-26). The gold standard of the diagnosis was the SARC-F questionnaire survey, which is routinely obtained by adding the scores regarding strength, assistance walking, rising from a chair, climbing stairs, and falls (27-31). A SARC-F score ≥4 was a positive indication of sarcopenia.
To increase the number of samples, the test on image was performed per segment, requiring one radiologist to repeat the measurement at the level of each cemented vertebral body across the axial CT images. At each position, it was measured for three times and averaged. The ROIs were set as large as possible, with areas ranging from 150 to 250 mm2, and were carefully placed on the posterior paraspinal muscle, avoiding the adjacent vertebral body and adipose tissue. The diagnostic accuracy resulting from the uncorrected, MAC, and AI-MAC images was compared in a receiver operating characteristic (ROC) analysis.
Statistical analysis
A commercial software (SPSS v22.0, IBM, Illinois, USA) was used for all statistical analyses. Continuous data are presented as mean ± standard division or median (interquartile range) as appropriate. Categorical data are presented as No. (percentage). In phantom study, the CT attenuation, image noise, SNRs, and CNR were compared using the Mann-Whitney U test. In patient study, the area under the curve (AUC) was used to compare the difference in the ROC analysis. A P<0.05 was considered statistically significant.
Results
Image quality metrics
Figure 2 displays the representative uncorrected, MAC, and AI-MAC images of the phantom, which were acquired with the low-dose following up CT protocol. Table 1 presents the mean CT attenuation and the noise measured on the reference image as well as the three sets of low-dose images. The noise of the AI-MAC images showed no significant difference compared with the reference image (all P>0.05), whereas uncorrected and MAC images showed significantly higher noise than the reference image (all P<0.05). No statistically significant difference was found between the reference images and the low-dose images for the mean CT attenuation of trabecular bone (all P>0.05). The mean CT attenuation of the paraspinal muscle acquired with uncorrected and MAC images were substantially lower than that of the reference image (all P<0.001). However, the CT attenuation of the paraspinal muscle obtained from AI-MAC images showed no significant difference compared with the reference image (P=0.63).
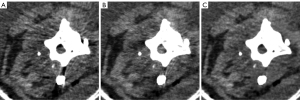
Table 1
Image acquisition (processing method) |
Routine CT (100 kVp, 100 mAs) |
Following up CT (100 kVp, 40 mAs) | ||||||||
---|---|---|---|---|---|---|---|---|---|---|
Uncorrected (as reference) |
Uncorrected | MAC | AI-MAC | |||||||
Mean ± SD | P | Mean ± SD | P | Mean ± SD | P | |||||
Trabecular bone | ||||||||||
CT attenuation (HU) | 361.5±26.4 | 365.6±25.6 | 0.80 | 366.7±44.5 | 0.93 | 360.9±20.8 | 0.84 | |||
Noise (HU) | 26.0±6.0 | 38.8±11.2 | 0.01 | 39.2±9.67 | 0.002 | 28.5±5.6 | 0.48 | |||
Paraspinal muscle | ||||||||||
CT attenuation (HU) | 49.5±3.9 | 25.8±13.0 | <0.001 | 27.6±12.1 | <0.001 | 46.8±8.2 | 0.63 | |||
Noise (HU) | 10.1±0.9 | 21.4±5.8 | <0.001 | 19.5±3.9 | <0.001 | 11.8±2.4 | 0.06 |
Values are expressed in mean ± standard division. The P values represent the differences between the values on studied images and the reference image. CT, computed tomography; HU, Hounsfield units; MAC, metal artifact correction; AI-MAC, artificial intelligence metal artifact correction.
Figure 3 compares the measured SNR and CNR for all four images. The AI-MAC image showed no significant difference to the reference image in SNR or in CNR (all P>0.05). However, the SNR and CNR of the uncorrected and MAC images were dramatically reduced (all P<0.05).
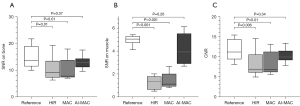
Correction accuracy
On the reference image, the volume of the segmented paraspinal muscle was 296.4 cm3. The segmentation result on AI-MAC image was closest to the reference image, which was 277.1 cm3, followed by 252.6 and 222.1 cm3 for the MAC and the uncorrected images respectively. In respect to that on the reference image, the paraspinal muscles segmented on the AI-MAC image were 18.6% and 8.3% more complete than those segmented on the uncorrected and MAC images, respectively. The shape of the segmented muscle on the AI-MAC image is also closest to that on the reference image, as illustrated in Figure 4. On the uncorrected images, bone cement caused severe beam-hardening and photon starvation artifacts, appearing as high and low attenuation streaks. These artifacts obviously affected the segmentation of muscle in positions adjacent to and at a distance from the bone cement. Furthermore, additional noise was induced by the bone cement, which is also a problem to the automatic segmentation program. The conventional MAC image failed to make large difference, with considerably evident artifacts left in the region. With AI-MAC, the suppression of both artifacts and noise has been substantially improved. In particular, the boundary between paraspinal muscle and fat was clearly presented.
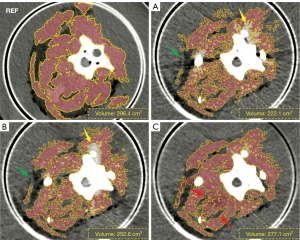
Diagnostic impact
Table 2 summarizes the characteristics of the patient collection. Of the 10 enrolled patients, median following up time after PVP was 6.0 months (IQR 4.0, 11.0 months). According to the SARC-F score, three patients were found with sarcopenia. Among all patients, five had one cemented vertebra, four had two cemented vertebrae, and one had four cemented vertebrae, providing a total of 17 available samples, i.e., 6 cemented vertebra bodies from patients with sarcopenia and 11 cemented vertebra bodies from patients without sarcopenia, for the ROC analysis.
Table 2
Patients’ characteristics | Value (N=10) |
---|---|
Age (years) | 79 (68, 84) |
No. of male patients | 3 (30.0) |
Body mass index (kg/m2) | 20.5 (16.7, 23.6) |
Type of vertebra fracture | |
Fragility | 7 (70.0) |
Traumatic | 3 (30.0) |
SARC-F score | |
≥4 | 3 (30.0) |
<4 | 7 (70.0) |
CTDIvol of following-up CT (mGy) | 1.8 (1.5, 2.2) |
Total cemented vertebra | n=17 |
T6 | 1 (5.9) |
T8 | 1 (5.9) |
T11 | 1 (5.9) |
T12 | 2 (11.8) |
L1 | 3 (17.6) |
L2 | 3 (17.6) |
L3 | 1 (5.9) |
L4 | 5 (29.4) |
Volume of injected cement (mL per vertebra body) |
2.8 (1.8, 4.0) |
Values are expressed in median (interquartile range) or in No. (percentage). CT, computed tomography; CTDIvol, volume CT dose index.
For the patients with sarcopenia, the median CT attenuation measured on the paraspinal muscle next to the cemented vertebra bodies was 30.1 HU (IQR 26.3, 32.2 HU), 25 HU (IQR 16.2, 29.6 HU), and 15.5 HU (IQR 13.7, 23.4 HU) for the uncorrected, MAC, and AI-MAC images, respectively. For the patients without sarcopenia, that was 37 HU (IQR 30.2, 46.2 HU), 36.7 HU (IQR 29, 44.2 HU), and 35.7 HU (IQR 34.2, 38.7 HU) for the three sets of images, respectively. Figure 5 represents the low-dose following up CT images of a sarcopenia patient.
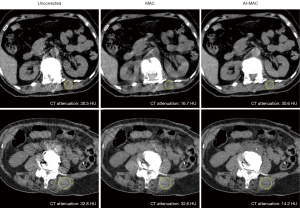
The diagnostic performance of sarcopenia with three different sets of images was compared in Figure 6. Significant improvement was found on the AI-MAC image compared to the uncorrected (P=0.01) and MAC images (P=0.04), with an AUC being 0.92 (95% CI, 0.68–1.00) versus 0.61 (95% CI, 0.35–0.83) and 0.70 (95% CI, 0.43–0.89), respectively. As detailed in Table 3, the AI-MAC achieved superior numbers in all statistical terms including sensitivity, specificity, positive predictive value, negative predictive value, and accuracy.
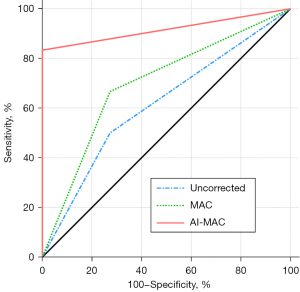
Table 3
Diagnostic value | Uncorrected | MAC | AI-MAC |
---|---|---|---|
Sensitivity | 50.0 (3/6) | 66.7 (4/6) | 83.3 (5/6) |
Specificity | 72.7 (8/11) | 72.7 (8/11) | 100.0 (11/11) |
PPV | 50.0 (3/6) | 57.1 (4/7) | 100.0 (5/5) |
NPV | 72.7 (8/11) | 80.0 (8/10) | 91.7 (11/12) |
Accuracy | 64.7 (11/17) | 70.6 (12/17) | 94.1 (16/17) |
AUC (95% CI) | 0.61 (0.35–0.83) | 0.70 (0.43–0.89) | 0.92 (0.68–1.00) |
Values are expressed in percentage (n/N). CT, computed tomography; MAC, metal artifact correction; AI-MAC, artificial intelligence metal artifact correction; PPV, positive predictive value; NPV, negative predictive value; AUC (ROC), area under the receiver operating characteristic curve; CI, confidence interval.
Discussion
In post-PVP following up CT, bone cement can often cause severe artifacts, similar to lightweight metals. It hinders the monitoring and quantification of tissue at the surgical site. In this study, we evaluated the capability of an AI-based MAC in dealing with the artifacts caused by bone cement in low-dose CT scenarios. Comparisons were made between HIR without MAC, with conventional MAC, and with AI-MAC, on both experimental phantom and clinical datasets. The vertebra phantom provides a preoperative ground truth for quantitative validation of the algorithm’s capabilities. The results of patient datasets can further demonstrate the algorithm’s potential in terms of diagnostic reliability.
In comparison to the AI-MAC, the conventional interpolation-based MAC, though widely implemented, suffers from several inherent deficiencies as reported in previous investigations. Regarding depiction of the implant, Wang et al. (32) reported that the conventional MAC might mistake the metal acetabular cup as artifacts and remove it during calculation. Andersson et al. (33) also reported that with the conventional MAC, the acetabular cup appeared very thin and even partially disappeared. In terms of the applicability to different metal materials, Lee et al. (34) indicated that the conventional MAC may not be effective in handling artifacts for titanium implants. In addition, Kidoh et al. (35) found that the conventional MAC caused unnatural texture in images of dental fillings, resulting in a loss of spatial resolution near the implant. Similarly, Boas et al. (36) also noted the spatial resolution issue in their clinical evaluation.
True-to-detail imaging of soft tissue is of special clinical concern in handling spinal disease, osteoporosis, and sarcopenia, since the bone mineral closely correlates with surrounding soft tissue (19,37,38). The AI-MAC images showed the best degree of integrity and correction accuracy in presenting the muscles. A variety of approaches have been proposed in previous studies to define the correction accuracy of MAC algorithms. Ishikawa et al. (39) used thresholding to determine the extent of the low attenuation areas, the mean CT attenuation to evaluate dark bands, and the difference in SD as well as location parameters following the Gumbel method to evaluate fine streak artifacts. Grandmougin et al. (40) evaluated the correction accuracy via estimation of trabecular distortion, size of metal object, and a low-density halo. Yoo et al. (41) introduced the artifact index to quantify the severity of the artifacts. In this study, we quantified the correction accuracy with the segmented volume of the paraspinal muscle. On the one hand, it is well suited for the scenario where comparison is made between paired preoperative and postoperative images. While the spatial location of soft tissue may change during the PVP, the volume is not supposed to vary. Based on such assumption, the proposed accuracy analysis can focus solely on the impact due to artifact. On the other hand, the interaction between the bone, the cement, and the soft tissue in contact region is of greater importance from a clinical perspective, than the cemented region itself. Decreased muscle density has been known to be associated with osteoporosis and fragility fracture. The correction analysis through muscle segmentation, therefore, is clinically more relevant than a straightforward artifact analysis.
Quantification of the musculoskeletal system is essential for assessing patients’ health and is linked to adverse health outcomes (42-47). Specially, the sarcopenia is more common in patients with osteoporosis. The conventional MAC image showed marginal improvement in sarcopenia diagnosis compared to uncorrected image. More importantly, several studies have shown the use of conventional MAC could lead to additional artifacts (12,48-50) that might generate unpredictable results for muscle quantification. In comparison, the AI-MAC algorithm shows not only remarkable performance in correcting the artifacts but also a potential for reliable quantitative assessment of sarcopenia. However, future investigation should address the long-term impact of AI-MAC by monitoring influences on therapeutic decisions, patient prognosis, and clinical workflow efficiency, which would highlight concrete clinical benefits beyond the direct improvement on image quality.
Notably, the location of the bone cement was not fixed in each patient, the vertebra levels we measured was variable. There may be subtle variability in the radiodensity of the paraspinal muscles at different vertebra levels. However, many studies suggested that the diagnostic threshold can be extrapolated to different vertebra levels to accommodate more CT examinations (22,45-47). To comprehensively investigate the clinical significance of AI-MAC in relation to sarcopenia, a larger patient dataset is necessary. The dataset needs to be grouped by surgical site and include paired pre- and post-operative images.
This study still has several limitations. First, the number of patients enrolled in the current study was limited, and all were in the postoperative period. Postoperative complications and prolonged bedrest may result in loss of muscle density, causing baseline deviations in CT attenuation. Second, the conventional MAC algorithm used here for comparison is just one implementation of the methodology. Other implementations that are currently available may have different performance, which is worth investigation. Given the completely different principle of AI-MAC, we would expect the findings to hold when comparing with other conventional MAC algorithms as well. Third, virtual mono-energetic imaging achieved via DECT has been reported in a number of cases to show superior performance for suppressing metal artifacts. This unfortunately was not included for comparison in the present study, due to the availability of DECT on the scanner model being tested. While this is certainly an interesting point to add, it proves again software approaches, via MAC or preferably AI-MAC, is of broader applicability.
Conclusions
In summary, the AI-MAC algorithm has been proven to be useful and more efficient than conventional MAC algorithms in low-dose CT imaging that involves the use of bone cement, providing superior image quality and a true-to-detail depiction of the soft tissue. The AI-MAC has also been found capable of substantially improving the reliability of the post-operative CT images for application in diagnosing sarcopenia. It may be a desirable routine choice for suppressing the beam-hardening artifacts in repeated low-dose following up CT scans of PVP. This study demonstrated one step forward for investigating and improving the generalizability of new MAC algorithms across different implant types, scanning protocols, or image reconstructions.
Acknowledgments
Funding: This study was supported by
Footnote
Reporting Checklist: The authors have completed the STARD reporting checklist. Available at https://qims.amegroups.com/article/view/10.21037/qims-23-1645/rc
Conflicts of Interest: All authors have completed the ICMJE uniform disclosure form (available at https://qims.amegroups.com/article/view/10.21037/qims-23-1645/coif). Y.Z. and G.Z. declare that they are employed researchers of United Imaging Healthcare Co., Ltd. The other authors have no conflicts of interest to declare.
Ethical Statement: The authors are accountable for all aspects of the work in ensuring that questions related to the accuracy or integrity of any part of the work are appropriately investigated and resolved. Phantom experiments were performed under a project license (No. SH9H-2021-T397-2) granted by the Institutional Review Board of Shanghai Ninth People’s Hospital for involving animal specimen, in compliance with institutional guidelines for the use of animal specimen. Patient study was conducted in accordance with the Declaration of Helsinki (as revised in 2013) and was approved by the Institution Review Board of Shanghai Ninth People’s Hospital for patient data research (No. SH9H-2021-T258-2). Individual consent for this retrospective analysis was waived.
Open Access Statement: This is an Open Access article distributed in accordance with the Creative Commons Attribution-NonCommercial-NoDerivs 4.0 International License (CC BY-NC-ND 4.0), which permits the non-commercial replication and distribution of the article with the strict proviso that no changes or edits are made and the original work is properly cited (including links to both the formal publication through the relevant DOI and the license). See: https://creativecommons.org/licenses/by-nc-nd/4.0/.
References
- Abdelgawaad AS, Ezzati A, Govindasamy R, Krajnovic B, Elnady B, Said GZ. Kyphoplasty for osteoporotic vertebral fractures with posterior wall injury. Spine J 2018;18:1143-8. [Crossref] [PubMed]
- Firanescu CE, Venmans A, de Vries J, Lodder P, Schoemaker MC, Smeets AJ, Donga E, Juttmann JR, Schonenberg K, Klazen CAH, Elgersma OEH, Jansen FH, Fransen H, Hirsch JA, Lohle PNM. Predictive Factors for Sustained Pain after (sub)acute Osteoporotic Vertebral Fractures. Combined Results from the VERTOS II and VERTOS IV Trial. Cardiovasc Intervent Radiol 2022;45:1314-21. [Crossref] [PubMed]
- Imamudeen N, Basheer A, Iqbal AM, Manjila N, Haroon NN, Manjila S. Management of Osteoporosis and Spinal Fractures: Contemporary Guidelines and Evolving Paradigms. Clin Med Res 2022;20:95-106. [Crossref] [PubMed]
- Láinez Ramos-Bossini AJ, López Zúñiga D, Ruiz Santiago F. Percutaneous vertebroplasty versus conservative treatment and placebo in osteoporotic vertebral fractures: meta-analysis and critical review of the literature. Eur Radiol 2021;31:8542-53. [Crossref] [PubMed]
- Zhou X, Meng X, Zhu H, Zhu Y, Yuan W. Early versus late percutaneous kyphoplasty for treating osteoporotic vertebral compression fracture: A retrospective study. Clin Neurol Neurosurg 2019;180:101-5. [Crossref] [PubMed]
- Griffoni C, Lukassen JNM, Babbi L, Girolami M, Lamartina C, Cecchinato R, Gasbarrini A, Barbanti Brodano G. Percutaneous vertebroplasty and balloon kyphoplasty in the treatment of osteoporotic vertebral fractures: a prospective randomized comparison. Eur Spine J 2020;29:1614-20. [Crossref] [PubMed]
- Fadili Hassani S, Cormier E, Shotar E, Drir M, Spano JP, Morardet L, Collet JP, Chiras J, Clarençon F. Intracardiac cement embolism during percutaneous vertebroplasty: incidence, risk factors and clinical management. Eur Radiol 2019;29:663-73. [Crossref] [PubMed]
- Barragán-Campos HM, Vallée JN, Lo D, Cormier E, Jean B, Rose M, Astagneau P, Chiras J. Percutaneous vertebroplasty for spinal metastases: complications. Radiology 2006;238:354-62. [Crossref] [PubMed]
- Wei Z, Weng X. Intracardiac and intrapulmonary cement embolisms after percutaneous vertebroplasty. Quant Imaging Med Surg 2023;13:8850-2. [Crossref] [PubMed]
- Wang WF, Lin CW, Xie CN, Liu HT, Zhu MY, Huang KL, Teng HL. The association between sarcopenia and osteoporotic vertebral compression refractures. Osteoporos Int 2019;30:2459-67. [Crossref] [PubMed]
- Libicher M, Vetter M, Wolf I, Noeldge G, Kasperk C, Grafe I, Da Fonseca K, Hillmeier J, Meeder PJ, Meinzer HP, Kauffmann GW. CT volumetry of intravertebral cement after kyphoplasty. Comparison of polymethylmethacrylate and calcium phosphate in a 12-month follow-up. Eur Radiol 2005;15:1544-9. [Crossref] [PubMed]
- Gjesteby L, De Man B, Jin Y, Paganetti H, Verburg J, Giantsoudi D, Wang G. Metal Artifact Reduction in CT: Where Are We After Four Decades? IEEE Access 2016;4:5826-49.
- Katsura M, Sato J, Akahane M, Kunimatsu A, Abe O. Current and Novel Techniques for Metal Artifact Reduction at CT: Practical Guide for Radiologists. Radiographics 2018;38:450-61. [Crossref] [PubMed]
- Kuchenbecker S, Faby S, Sawall S, Lell M, Kachelrieß M. Dual energy CT: how well can pseudo-monochromatic imaging reduce metal artifacts? Med Phys 2015;42:1023-36. [Crossref] [PubMed]
- Guo R, Zou Y, Zhang S, An J, Zhang G, Du X, Gong H, Xiong S, Long Y, Ma J. Preclinical validation of a novel deep learning-based metal artifact correction algorithm for orthopedic CT imaging. J Appl Clin Med Phys 2023;24:e14166. [Crossref] [PubMed]
- Paik JM, Rosen HN, Katz JN, Rosner BA, Rimm EB, Gordon CM, Curhan GC. BMI, Waist Circumference, and Risk of Incident Vertebral Fracture in Women. Obesity (Silver Spring) 2019;27:1513-9. [Crossref] [PubMed]
- Li X, Wu B, Zou Y, Zhang G, Liu S, Zhao L, Zhang Z, Wu W, Liu C, Ai S. Development of a 3D-printed pelvic CT phantom combined with fresh pathological tissues of bone tumor. Quant Imaging Med Surg 2022;12:4647-57. [Crossref] [PubMed]
- Meyer E, Raupach R, Lell M, Schmidt B, Kachelriess M. Normalized metal artifact reduction (NMAR) in computed tomography. Med Phys 2010;37:5482-93. [Crossref] [PubMed]
- Albano D, Messina C, Vitale J, Sconfienza LM. Imaging of sarcopenia: old evidence and new insights. Eur Radiol 2020;30:2199-208. [Crossref] [PubMed]
- Tagliafico AS, Bignotti B, Torri L, Rossi F. Sarcopenia: how to measure, when and why. Radiol Med 2022;127:228-37. [Crossref] [PubMed]
- Yip C, Dinkel C, Mahajan A, Siddique M, Cook GJ, Goh V. Imaging body composition in cancer patients: visceral obesity, sarcopenia and sarcopenic obesity may impact on clinical outcome. Insights Imaging 2015;6:489-97. [Crossref] [PubMed]
- Zopfs D, Theurich S, Große Hokamp N, Knuever J, Gerecht L, Borggrefe J, Schlaak M, Pinto Dos Santos D. Single-slice CT measurements allow for accurate assessment of sarcopenia and body composition. Eur Radiol 2020;30:1701-8. [Crossref] [PubMed]
- Boutin RD, Lenchik L. Value-Added Opportunistic CT: Insights Into Osteoporosis and Sarcopenia. AJR Am J Roentgenol 2020;215:582-94. [Crossref] [PubMed]
- Boutin RD, Yao L, Canter RJ, Lenchik L. Sarcopenia: Current Concepts and Imaging Implications. AJR Am J Roentgenol 2015;205:W255-66. [Crossref] [PubMed]
- Lenchik L, Lenoir KM, Tan J, Boutin RD, Callahan KE, Kritchevsky SB, Wells BJ. Opportunistic Measurement of Skeletal Muscle Size and Muscle Attenuation on Computed Tomography Predicts 1-Year Mortality in Medicare Patients. J Gerontol A Biol Sci Med Sci 2019;74:1063-9. [Crossref] [PubMed]
- Faccioli E, Terzi S, Giraudo C, Zuin A, Modugno A, Labella F, Zambello G, Lorenzoni G, Schiavon M, Gregori D, Pasello G, Calabrese F, Dell'Amore A, Rea F. Sarcopenia as a Predictor of Short- and Long-Term Outcomes in Patients Surgically Treated for Malignant Pleural Mesothelioma. Cancers (Basel) 2022;14:3699. [Crossref] [PubMed]
- Bahat G, Yilmaz O, Kılıç C, Oren MM, Karan MA. Performance of SARC-F in Regard to Sarcopenia Definitions, Muscle Mass and Functional Measures. J Nutr Health Aging 2018;22:898-903. [Crossref] [PubMed]
- Malmstrom TK, Morley JE. SARC-F: a simple questionnaire to rapidly diagnose sarcopenia. J Am Med Dir Assoc 2013;14:531-2. [Crossref] [PubMed]
- Malmstrom TK, Miller DK, Simonsick EM, Ferrucci L, Morley JE. SARC-F: a symptom score to predict persons with sarcopenia at risk for poor functional outcomes. J Cachexia Sarcopenia Muscle 2016;7:28-36. [Crossref] [PubMed]
- Ida S, Kaneko R, Murata K. SARC-F for Screening of Sarcopenia Among Older Adults: A Meta-analysis of Screening Test Accuracy. J Am Med Dir Assoc 2018;19:685-9. [Crossref] [PubMed]
- Woo J, Leung J, Morley JE. Validating the SARC-F: a suitable community screening tool for sarcopenia? J Am Med Dir Assoc 2014;15:630-4. [Crossref] [PubMed]
- Wang F, Xue H, Yang X, Han W, Qi B, Fan Y, Qian W, Wu Z, Zhang Y, Jin Z. Reduction of metal artifacts from alloy hip prostheses in computer tomography. J Comput Assist Tomogr 2014;38:828-33. [Crossref] [PubMed]
- Andersson KM, Norrman E, Geijer H, Krauss W, Cao Y, Jendeberg J, Geijer M, Lidén M, Thunberg P. Visual grading evaluation of commercially available metal artefact reduction techniques in hip prosthesis computed tomography. Br J Radiol 2016;89:20150993. [Crossref] [PubMed]
- Lee YH, Park KK, Song HT, Kim S, Suh JS. Metal artefact reduction in gemstone spectral imaging dual-energy CT with and without metal artefact reduction software. Eur Radiol 2012;22:1331-40. [Crossref] [PubMed]
- Kidoh M, Nakaura T, Nakamura S, Tokuyasu S, Osakabe H, Harada K, Yamashita Y. Reduction of dental metallic artefacts in CT: value of a newly developed algorithm for metal artefact reduction (O-MAR). Clin Radiol 2014;69:e11-6. [Crossref] [PubMed]
- Boas FE, Fleischmann D. Evaluation of two iterative techniques for reducing metal artifacts in computed tomography. Radiology 2011;259:894-902. [Crossref] [PubMed]
- Huang CWC, Tseng IJ, Yang SW, Lin YK, Chan WP. Lumbar muscle volume in postmenopausal women with osteoporotic compression fractures: quantitative measurement using MRI. Eur Radiol 2019;29:4999-5006. [Crossref] [PubMed]
- Pickhardt PJ, Graffy PM, Zea R, Lee SJ, Liu J, Sandfort V, Summers RM. Automated Abdominal CT Imaging Biomarkers for Opportunistic Prediction of Future Major Osteoporotic Fractures in Asymptomatic Adults. Radiology 2020;297:64-72. [Crossref] [PubMed]
- Ishikawa T, Suzuki S, Harashima S, Fukui R, Kaiume M, Katada Y. Metal artifacts reduction in computed tomography: A phantom study to compare the effectiveness of metal artifact reduction algorithm, model-based iterative reconstruction, and virtual monochromatic imaging. Medicine (Baltimore) 2020;99:e23692. [Crossref] [PubMed]
- Grandmougin A, Bakour O, Villani N, Baumann C, Rousseau H, Gondim Teixeira PA, Blum A. Metal artifact reduction for small metal implants on CT: Which image reconstruction algorithm performs better? Eur J Radiol 2020;127:108970. [Crossref] [PubMed]
- Yoo HJ, Hong SH, Chung BM, Moon SJ, Choi JY, Chae HD, Chang MY. Metal Artifact Reduction in Virtual Monoenergetic Spectral Dual-Energy CT of Patients With Metallic Orthopedic Implants in the Distal Radius. AJR Am J Roentgenol 2018;211:1083-91. [Crossref] [PubMed]
- Liu J, Yu X, Huang X, Lai Q, Chen J. Associations of muscle and adipose tissue parameters with long-term outcomes in middle and low rectal cancer: a retrospective cohort study. Cancer Imaging 2023;23:5. [Crossref] [PubMed]
- Uchida T, Sekine R, Matsuo K, Kigawa G, Umemoto T, Tanaka K. Impact of body fat and muscle quantity on short- and long-term outcome after gastrectomy for cancer. Clin Nutr 2022;41:1467-74. [Crossref] [PubMed]
- Koitka S, Kroll L, Malamutmann E, Oezcelik A, Nensa F. Fully automated body composition analysis in routine CT imaging using 3D semantic segmentation convolutional neural networks. Eur Radiol 2021;31:1795-804. [Crossref] [PubMed]
- Peterson SJ, Braunschweig CA. Prevalence of Sarcopenia and Associated Outcomes in the Clinical Setting. Nutr Clin Pract 2016;31:40-8. [Crossref] [PubMed]
- Liu J, Ma J, Yang C, Chen M, Shi Q, Zhou C, Huang S, Chen Y, Wang Y, Li T, Xiong B. Sarcopenia in Patients with Cirrhosis after Transjugular Intrahepatic Portosystemic Shunt Placement. Radiology 2022;303:711-9. [Crossref] [PubMed]
- Shafaat O, Liu Y, Jackson KR, Motter JD, Boyarsky BJ, Latif MA, Yuan F, Khalil A, King EA, Zaheer A, Summers RM, Segev DL, McAdams-DeMarco M, Weiss CR. Association between Abdominal CT Measurements of Body Composition before Deceased Donor Liver Transplant with Posttransplant Outcomes. Radiology 2023;306:e212403. [Crossref] [PubMed]
- Huang JY, Kerns JR, Nute JL, Liu X, Balter PA, Stingo FC, Followill DS, Mirkovic D, Howell RM, Kry SF. An evaluation of three commercially available metal artifact reduction methods for CT imaging. Phys Med Biol 2015;60:1047-67. [Crossref] [PubMed]
- Laukamp KR, Lennartz S, Neuhaus VF, Große Hokamp N, Rau R, Le Blanc M, Abdullayev N, Mpotsaris A, Maintz D, Borggrefe J. CT metal artifacts in patients with total hip replacements: for artifact reduction monoenergetic reconstructions and post-processing algorithms are both efficient but not similar. Eur Radiol 2018;28:4524-33.
- Zopfs D, Lennartz S, Pennig L, Glauner A, Abdullayev N, Bremm J, Große Hokamp N, Persigehl T, Kabbasch C, Borggrefe J, Laukamp KR. Virtual monoenergetic images and post-processing algorithms effectively reduce CT artifacts from intracranial aneurysm treatment. Sci Rep 2020;10:6629. [Crossref] [PubMed]