White matter biomarker for predicting de novo Parkinson’s disease using tract-based spatial statistics: a machine learning-based model
Introduction
Parkinson’s disease (PD) is among the most prevalent neurodegenerative disorders (1), the incidence of which has been steadily rising in recent years. In the early stages of PD, symptoms are typically quite mild, making it challenging for physicians to establish a definitive diagnosis (2). During the prodromal phase, PD patients may present with non-motor symptoms such as a diminished sense of olfaction and cognitive deficits (3). As the disease progresses, patients may develop classic motor symptoms such as tremors. The onset of motor symptoms generally indicates that the disease has reached an intermediate or advanced stage, and the optimal window for diagnosis and treatment has elapsed. Unlike visible symptoms such as tremors, cognitive decline is a gradual process, and its underlying causes and neuroanatomical basis remain incompletely understood (4). Currently, there is no effective cure for PD, and available treatments mainly focus on symptom management (5). Consequently, early diagnosis and detection of PD are of paramount importance (6). An efficient early diagnosis enables the timely detection of health issues and the implementation of intervention to minimize the severe health risks associated with the disease (1,2).
In the early stages, accurately and promptly identifying PD poses a significant challenge (7). Currently, the diagnosis of PD primarily relies on clinical rating scales and the expertise of clinicians. These diagnostic methods are imperfect, time-consuming, and labor-intensive, lacking standardized and effective quantitative indicators. Routine non-invasive neuroimaging examinations for PD patients, such as magnetic resonance imaging (MRI), contain a wealth of potentially valuable information, including grayscale range, intensity, and intracellular changes in brain tissue characteristics. These details can aid in better understanding the cerebral alterations in PD patients. However, recognizing this information solely based on the clinical expertise of radiologists can be challenging (8).
Significant research efforts have been devoted to innovative PD diagnostic methods (9,10), aiming to enhance disease detection capabilities. The Parkinson’s Progression Markers Initiative (PPMI) is an observational clinical cohort study that has been enrolling early-stage PD patients diagnosed within 2 years from approximately 50 research institutions since 2010. Following enrollment, PPMI continuously tracks the progression of various types of PD patients for over a decade. This study is based on the diffusion tensor imaging (DTI) series of cases participating in the PPMI.
Although the precise pathogenesis of PD remains unclear, growing evidence suggests that structural changes may occur in the white matter (WM) of PD patients during the early stage of the disease (4-11). These changes could potentially serve as indicators of cognitive decline in PD patients and warrant further exploration for their potential in disease diagnosis.
DTI, a non-invasive neuroimaging technique based on MRI, is capable of detecting early brain changes that may precede those observed through traditional structural MRI. It has been used extensively in elucidating alteration in brain WM (12,13). DTI is highly sensitive to the movement of water molecules, particularly their diffusion along WM axons. Consequently, DTI-derived metrics are adept at capturing WM changes associated with neurological disorders (14).
One such DTI metric is fractional anisotropy (FA), which reflects the extent of water molecule diffusion in different directions. Another important metric is the mean diffusivity (MD), which provides an overview of water molecule movement throughout the brain (12). FA and MD have been increasingly employed in quantitative studies of PD (15,16). These metrics, by highlighting abnormalities in WM, may offer insights into cognitive decline, attention deficits, and other prodromal non-motor symptoms associated with the disease (17).
The tract-based spatial statistics (TBSS) (18-20) method, developed based on DTI, represents an advanced spatial statistics approach rooted in the analysis of WM fiber bundles (21). Unlike voxel-based analysis (VBA) methods, TBSS effectively addresses issues related to significant registration errors and variations in smoothing kernel selection. It confines the analysis to the central region of the major WM bundle, thereby enhancing the precision of the detection of WM abnormalities (22).
In cases with varying anatomical structures, TBSS excels at accurately aligning major fiber bundles and quantifying their characteristics. This capability provides valuable insights for clinicians seeking to understand the impact of PD on the brain from a WM fiber perspective, elucidate its cognitive damage mechanisms, and offer guidance for clinical diagnosis and treatment (22-24).
A bioimaging marker is typically characterized by the volume or average intensity of images or regions. Neuroimaging studies of PD create essential conditions for the development of bioimaging markers for the disease (25). The utilization of TBSS technology to analyze DTI data from both early PD patients and normal cases can provide a deeper understanding of the WM structural characteristics in PD patients and pinpoint areas of WM pathology associated with PD. Through further experimentation, it becomes possible to explore the feasibility of using the potential neuroimaging information extracted from these areas as a neuroimaging biomarker for PD diagnosis. Early diagnosis and assessing disease risk based on biomarker changes hold significant importance (26).
In recent years, alongside traditional quantitative and semi-quantitative analysis methods, machine learning (ML) techniques have gained significant traction in the realm of medical imaging analysis (7,8,15,27). Particularly in studies involving brain tumors and neurodegenerative disorders, the automated extraction and analysis of potential high-dimensional image information have demonstrated their potential for the detection and diagnosis of neurodegenerative diseases. This automated approach may prove more effective than manual detection processes (5,23,28).
This study aimed to identify PD-related WM lesion regions through TBSS analysis, validate their potential as imaging biomarkers, and employ them as regions of interest (ROIs) for extracting distinctive features to develop machine learning-based prediction models. The specific process unfolded as follows: Firstly, cases were selected based on their inclusion in the PPMI database, with both imaging and non-imaging information utilized to establish an early PD database. To be more precise, the early PD imaging database comprised cases diagnosed within 3 years, who had not yet initiated medication. Following image preprocessing and TBSS analysis, areas of WM exhibiting statistically significant differences were employed as masks to extract features from each case’s FA image. Subsequently, these features underwent screening and analysis through geometric dimension reduction and least absolute shrinkage and selection operator (LASSO) logistic regression (29). These obtained features, when combined with demographic and clinical data of the cases, were used to construct an individual-level PD prediction model. This model was designed for the early diagnosis of PD patients utilizing the RF classifier, incorporating 5-fold cross-validation, and predicting individual-level PD risk within the test set.
The contributions and innovations of this study are as follows:
- On the public PPMI dataset, for the first time, the principles of data screening and inclusion for constructing an early PD database based on DTI images were explicitly proposed. A convincing early multicenter PD DTI database was created, and data processing and analysis were conducted. The research results demonstrated good generalizability.
- The study exclusively utilized DTI images, combined with demographic and clinical information, to train a predictive model using single-modal MRI data, and obtained high early PD identification indicators.
- The study used the TBSS method to calculate the imaging biomarkers of PD, and leverage the biomarkers to assist clinicians in understanding PD from the perspective of WM damage, providing valuable insights for clinical diagnosis and disease treatment; Subsequently, the ML method was used to verify the ability of the biomarker to distinguish early PD from NC, and an early PD diagnostic model was developed, achieving good predictive performance.
We present this article in accordance with the TRIPOD reporting checklist (available at https://qims.amegroups.com/article/view/10.21037/qims-23-1478/rc).
Methods
Figure 1 illustrates the fundamental framework of the prediction model proposed in this paper. Firstly, the process begins with preprocessing the cases’ DTI images, which involves the removal of non-brain tissues, such as skulls. This step enables the calculation of DTI quantitative values and the generation of scalar value images, including FA, radial diffusivity (RD), MD, and axial diffusivity (AD). Secondly, the TBSS method is employed to compute the WM fibrous skeleton (ROI area) by identifying regions with significant differences (P<0.05) between the PD group and the NC group. This ROI area serves as a mask to extract corresponding regions from each case’s FA map. The FA values within this area collectively form the 3-dimensional (3D) spatial features for each case. Thirdly, the 3D spatial features of the cases are geometrically partitioned. Each partition’s value is determined as the sum of the pixel values within the block, resulting in reduced 3D spatial features composed of the superblock values. These are then flattened to create the corresponding 1-dimensional (1D) spatial feature vectors. Fourthly, the LASSO regression algorithm (29-31) is utilized for feature selection and the selected features are arranged according to their regression coefficients. A 5-fold cross-validation method is further employed to identify the optimal feature combination, which is fed into the RF method for PD prediction, constituting our neuroimaging prediction model, referred to as NeuroM. Simultaneously, the non-neuroimaging information from the cases for basic statistics and disease predictions is gathered to form the clinical prediction model, denoted as ClinicalM. The integration of these two models yields our comprehensive prediction model, referred to as CombineM. The subsequent section provides a detailed exposition of our methods. The study was conducted in accordance with the Declaration of Helsinki (as revised in 2013).
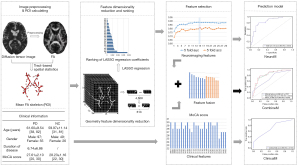
Cases screening and early PD database construction
Based on the Hoehn and Yahr (H&Y) score (≤2.5) (32), Montreal Cognitive Assessment (MoCA) score (≥20), the duration of the patient’s disease (duration of disease ≤14 months), the patient’s treatment and medication status (not yet receiving any relevant treatment and not taking PD-related medications), and after excluding DTI images of cases with significant noise and artifacts, a total of 227 cases, comprising 75 NC and 152 PD patients, were meticulously chosen to construct an early PD database from the PPMI database (https://www.ppmi-info.org/data). The non-neuroimaging data and clinical scores of the cases are presented in Table 1. According to the H&Y score (≤2.5), these cases were regarded as de novo PD patients, who were in the early stages of PD. The DTI data from the year they were first enrolled in PPMI were utilized in the study.
Table 1
Demographic/clinical scale | PD (n=152) | NC (n=75) | P | t value |
---|---|---|---|---|
Age (years) | 61.60±9.54 [38, 82] | 59.97±11.14 [31, 81] | 0.23 | 1.192 |
Gender | 0.82 | 0.224 | ||
Male | 97 (66.4) | 49 (33.6) | ||
Female | 55 (67.9) | 26 (32.1) | ||
Years of education | 15.22±3.15 [8, 22] | 15.73±2.97 [9, 22] | 0.24 | −1.167 |
Duration of disease (month) | 6.74±6.86 [0, 32] | – | – | – |
MoCA score | 27.61±2.10 [20, 30] | 28.23±1.16 [22, 30] | 0.02* | −2.359 |
Statistical analysis was conducted and the data for each category was represented as n (%) or mean ± standard deviation [range]. *, P<0.05, considered statistically significant. PD, Parkinson’s disease; NC, normal control; MoCA, Montreal Cognitive Assessment.
The cases’ enrollment years were selected as shown in Table S1. To ensure the robustness of our prediction model, the data from each case’s baseline year was specifically selected. It should be noted that the baseline years of enrollment varied among cases (indicated by the circle), and not all cases’ data was continuous (marked by the checkmark). Some cases only underwent examination for a single year (e.g., case with ID 4139, examined solely in 2013). Importantly, during the baseline year examination of the cases, these individuals had not yet initiated PD-related treatment and did not take any medication.
All cases’ DTIs were acquired using Siemens scanners (Siemens, Erlangen, Germany), utilizing an axial fast gradient echo sequence (MPRAGE) along the AC-PC plane. This scan covered the entire brain, from the base of the cerebellum to the top of the brain, and adhered to the following main parameters: acquisition type: 4D; field strength: 3.0 Tesla; flip angle: 90°; in-plane pixel resolution: 1.98 mm × 1.98 mm (X & Y); slice thickness: 2.0 mm; b value: 1,000 s/mm2; diffusion gradient directions: 64; image matrix: 116×116×72; number of non-diffusion (b0) image: 1; repetition time (TR): 900 ms; echo time (TE): 88 ms.
Data preprocessing and diffusion quantitative indicators calculation
In order to obtain the differential region between the PD group and NC group and verify its ability to predict early PD at the individual level, it is necessary to first preprocess the DTI images of the cases and calculate the diffusion tensor and quantitative parameter images. Figure 2 shows the basic data preprocessing process.
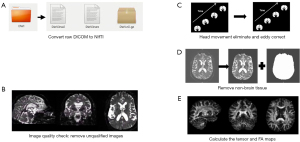
For each case’s 4-dimensional (4D) diffusion-weighted image sequence, the preprocessing process is as follows: (I) convert the original data from digital imaging and communications in imaging (DICOM) format into neuroimaging informatics technology initiative (NIfTI) format that can be easily utilized by FSL software (http://www.fmrib.ox.ac.uk/fsl/) using MRIcron software, and the diffusion gradient table and b-value table are obtained simultaneously; (II) visually inspect the image, including resolution, size, and gradient direction. Then, use the fsleyes plugin of FSL software to check the image quality of each volume layer by layer, and exclude images with signal loss, artifacts, and severe head movements from this study; (III) use Flirt in the FSL software package to affine transform all images of each case to its first b0 image to eliminate small head motion offsets, and use the eddy_correct function of the FDT plugin to eliminate eddy current effects, and adjust the gradient direction based on the changes in the eddy current correction; (IV) before calculating the tensor, a mask image needs to be generated to determine the calculation range, so that the analyzed voxels are limited to the brain area, reducing the amount of computation and improving the accuracy of spatial registration. Generally, the corresponding binary brain mask is obtained through the b0 image. In this study, the BET tool of the FSL software is used to remove the skull and other non-brain images from the b0 image, and the threshold is set to 0.2 for removing non-brain tissue; (V) calculate the tensor-fitting and scalar indicators such as FA or MD values using the DTIFIT tool of FSL software.
Group-level analysis in TBSS
The TBSS method allows for the identification of distinct WM regions distinguishing the PD group from the NC group, providing a comprehensive whole-brain perspective. In this study, the standard TBSS program from the FSL software is utilized to preprocess FA images, as illustrated in Figure S1.
The specific TBSS process comprises the following steps:
- Image alignment: linear and non-linear registration are employed to align each case’s individual FA image with the reference FA image, FMRIB58_FA. This alignment is followed by affine transformation to map the individual FA images to the standard space.
- Skeleton construction: the average FA image and WM skeleton of all cases are constructed, applying an FA threshold of 0.2 to all FA images registered in the standard space.
- Data projection: each case’s aligned FA data is projected onto the skeleton, enabling comparisons within a common framework.
- Statistical analysis: the randomize method is employed to perform 5,000 permutation tests, aimed at identifying WM regions exhibiting significant differences. The result is a family-wise error (FWE) corrected 1 − P_value image, represented by the red skeleton in Figure S1. The red skeleton denotes areas where there is a statistically significant difference between the PD group and the NC group exists, with P<0.05 (FWE corrected), indicating statistical significance.
Skeleton geometric dimensionality reduction and feature sparse representation
In this study, regions exhibiting statistically significant differences between the PD and NC groups were identified as ROIs and subsequently transformed into binary masks, with voxel values outside these masks set to 0. The procedure involves extracting voxel values within the mask area from each case’s FA image, resulting in the creation of a 3D skeleton. This skeleton can be further processed to derive a 1D feature vector through a series of dimensionality reduction operations.
All FA images of the cases were meticulously registered to the standard space. The average FA map boasts dimensions of 182×218×182, comprising a total of 7,221,032 voxels. The voxel values are uniformly distributed in the interval [0, 1], where the minimum (min) value is 0, and the maximum value (max) is 0.94. Of all these voxels, those falling outside the ROI were set to 0. This led to 46,831 voxels, constituting a mere 0.65% of the total voxels, surpassing the minimum threshold of 0. Moreover, 20,808 voxels, which account for a significant 44.43% of voxels greater than the midpoint value of max/2=0.47, were found. The cumulative voxel values summed up to 21,580.93, yielding a mean value of 0.003 and a standard deviation of 0.040. It is worth noting that the WM fiber skeleton, characterized by voxel values exceeding max/2, occupies only a minute portion of the entire image space. Consequently, a more rational representation is imperative to bolster the performance of the prediction model.
To effectively represent the WM fiber skeleton within each case’s individual FA image, the FA images, originally sized at 182×218×182 in the standard space, were downscaled with a step size of 12, resulting in images of dimensions 16×18×16. Here, each voxel’s value represents the summation of the voxel values within the corresponding 12×12×12 block in the original image. After arranging these reduced-dimensional images in a row-column-page order, a 4,608-dimension vector was obtained. Following the removal of zero elements from this sparse matrix, a streamlined 613-dimensional vector can be obtained, as illustrated in Figure 3 and detailed in Table S2. It should be noted that for computational convenience, the FA image with dimensions of 182×218×182 was padded with additional rows and columns, ultimately yielding FA image of dimensions 192×216×192.
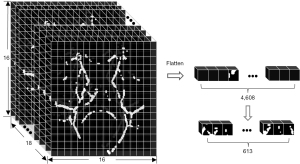
Upon inspecting Figure 3 and Table S2 and post-gridding dimensionality reduction, the resulting image comprised 4,608 voxels, with a voxel value distribution spanning the interval [min, max]=[0, 205.233]. Within this voxel set, 613 voxels, constituting 13.3% of the total voxel count, surpassed the minimum threshold of 0. Additionally, 62 voxels, accounting for 10.11% of voxels with values greater than 0, exceeded the threshold of 103 (max/2=205.233/2≈103). The sum of voxel values remained consistent at 21,580.93, with a mean value of 4.683 and a standard deviation of 18.820.
After gridding the skeleton maps, the mean value expanded from 0.003 to 4.683, and the maximal value expanded from 0.94 to 205.233. Consequently, the difference between the mean and maximal voxel values increased significantly. Accordingly, the percentage of voxels with voxel values greater than max/2 decreased from 44.43% to 10.11%. Gridding clustered the voxel values of the original image, concentrated them, amplified the values in the ‘grid voxels’, and resulted in a more extreme and sparse distribution of voxel values.
The primary advantage of gridding is dimensionality reduction while preserving positional information. However, whether gridded or not, the positions of values greater than 0 remain fixed. Therefore, non-zero values can be directly extracted and combined into feature vectors.
For any given case’s DTI image, following preprocessing steps such as diffusion index calculation, TBSS group-level analysis, individual skeleton geometric downscaling, and sparse representation, a reshaped feature vector of size 1×613 could be obtained from the case’s FA image.
Selection of LASSO regression features
In scenarios involving high-dimensional and multi-regional features, it is often desirable to identify a smaller subset of regional features that exhibit strong discriminative capabilities, highlighting the key regions with the most significant impact. To address this, we employed the LASSO algorithm, which effectively filters relevant features while mitigating the challenges posed by high-dimensional data redundancy.
LASSO integrates the L1-norm into the classical linear regression least squares estimation. This integration allows LASSO to assign unimportant feature coefficients to 0, thereby enhancing parameter estimation accuracy. This process facilitates variable selection, ultimately leading to feature dimensionality reduction.
In our experiment, 15 positional features with the highest weight coefficients were selected to be incorporated into the construction of the PD-NC prediction model.
Dataset partition and individual prediction model construction
To assess the discriminative potential of features extracted by LASSO from differential regions between the early PD group and NC groups, the widely employed RF algorithm was utilized. At the same time, in order to construct and train a reliable PD prediction model, theoretically, the number of cases should be at least 10 times the number of features (15,33-36). Therefore, as shown in Figure 4, this study randomly divided the dataset into training and testing sets in 7:3 ratios, with the training group consisting of 103 PD and 55 NC participants, and the testing group consisting of 49 PD and 20 NC participants. The selected features were subjected to a 5-fold cross-validation on the training set for case classification.
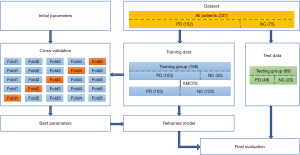
In this study, we noted a slight class imbalance among the enrolled cases. To address this, the Synthetic Minority Over-sampling Technique (SMOTE) algorithm was employed, which generated additional samples within the training set to facilitate model training. To mitigate the limitations associated with fixed dataset feature selection and the risk of overfitting, the 5-fold cross-validation method was adopted for model training. We selected the most appropriate model, characterized by the smallest average error (including hyper-parameters), and subsequently evaluated its performance on the test set. Model classification prediction performance was assessed using metrics such as accuracy (ACC), precision, recall, F1 score, and area under the receiver operating characteristic (ROC) curve (AUC).
Furthermore, we evaluated the classification performance of combining regional location feature information (i.e., neuroimaging information) with clinical scale information, specifically the MoCA. Additionally, we assessed the classification performance when using MoCA data alone.
Statistical analysis of non-neuroimaging information
The non-neuroimaging data in this study encompassed case demographics and their clinical MoCA scales. These were subjected to analysis using IBM SPSS Statistics 20 (IBM Corp., Armonk, NY, USA). A comparison between the PD group and the NC group was conducted using independent sample t-tests, with measurements presented as mean ± standard deviation. A significance level of P<0.05 was employed to determine statistical significance.
Results
Demographics and clinical scores of cases
Table 1 presents the demographic information and clinical scores statistics of the study cases. The MoCA is a tool employed for the rapid screening of mild cognitive impairment (MCI) (37). The assessment of cognitive domains encompasses memory, language, abstract thinking, attention and concentration, visual-spatial skills, executive function, calculation, and orientation, among others. The normal range of the scale test falls within [26, 30], with a maximum total score of 30 points.
Notably, the MoCA score levels indicated that the cases included in this study were in the prodromal stage of PD. There were no significant differences observed in gender, age, or years of education between the PD group and the NC group. However, it is worth mentioning that the MoCA scores in the PD group were lower than those in the NC group, and this disparity was statistically significant (P<0.05). This observation aligns with prior research findings suggesting that non-motor symptoms may manifest in PD patients during the prodromal phase of the disease.
Differences in FA skeleton between PD and NC groups
The statistical findings concerning the disparities in WM skeleton regions on FA images between the PD and NC groups were visualized using the fsleyes plugin within the FSL software, as depicted in Figure 5. It is important to note that the highlighted areas in this context signify regions with significant differences(P<0.05). Furthermore, the ‘cluster’ command provides relevant information about key cluster regions, which is detailed in Table 2. Additionally, the ‘atlasquery’ command yields information regarding important WM fiber bundles, as illustrated in Figure 6, and queries their presence within the standard template areas.
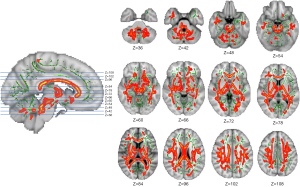
Table 2
Cluster index | Voxels | Probability in the region | Peak center coordinate | Coordinates of the center of gravity |
---|---|---|---|---|
1 | 47899 | 0.979 | 55, 108, 98 | 85.7, 103, 84.1 |
FA, fractional anisotropy.
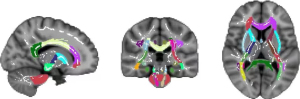
Notably, nearly the entire WM region in the brains of PD group patients exhibited substantial alterations, characterized by a significant decrease in FA values when compared to the NC group. In Figure 5, the red areas represent coronal planes with statistically significant differences, whereas the green areas represent FA fiber skeletons demonstrating disparities between the PD and NC groups.
Figure 6, based on the TBSS standard process, further overlays the WM fiber skeleton (depicted in white) with statistical differences (P<0.05) associated with PD onto the Johns Hopkins University (JHU) white-matter tractography atlas (https://identifiers.org/neurovault.collection:264). This overlay allows for the observation of the anatomical distribution of distinct WM regions.
Table 3 presents the primary distinguishing fiber bundle structures between the PD group and the NC group using the standard JHU white-matter tractography atlas template. In this context, higher average probability values indicate greater impairment to the corresponding brain regions.
Table 3
Atlas label | Average probability |
---|---|
Anterior thalamic radiation L | 1.5189 |
Anterior thalamic radiation R | 1.1459 |
Corticospinal tract L | 0.9842 |
Corticospinal tract R | 0.8636 |
Cingulum (cingulate gyrus) L | 0.1523 |
Cingulum (cingulate gyrus) R | 0.1270 |
Cingulum (hippocampus) L | 0.0877 |
Cingulum (hippocampus) R | 0.0630 |
Forceps major L | 0.7363 |
Forceps major R | 1.3485 |
Inferior fronto-occipital fasciculus L | 1.5463 |
Inferior fronto-occipital fasciculus R | 1.7804 |
Inferior longitudinal fasciculus L | 1.2493 |
Inferior longitudinal fasciculus R | 1.0993 |
Superior longitudinal fasciculus L | 1.6912 |
Superior longitudinal fasciculus R | 1.5079 |
Uncinate fasciculus L | 0.4126 |
Superior longitudinal fasciculus R | 0.2309 |
Superior longitudinal fasciculus (temporal part) L | 0.8229 |
Superior longitudinal fasciculus (temporal part) R | 0.5507 |
PD, Parkinson’s disease; NC, normal control; JHU, Johns Hopkins University; L, left; R, right.
Feature selection
We employed LASSO (29) for feature selection and dimension reduction. A larger LASSO coefficient indicated a stronger predictive capability of the associated feature. In Figure 7, features corresponding to different superblock regions are filtered and ranked based on their LASSO coefficients, with the feature corresponding to the 45th superblock region demonstrating the highest predictive potential. Figure 8 illustrates that retaining the features of 15 superblocks yields the highest AUC and ACC values for early PD prediction.
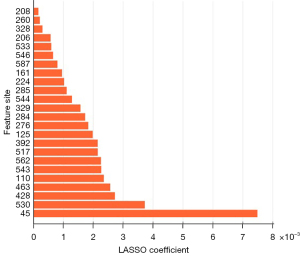
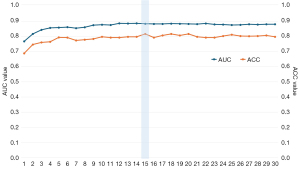
Model validation and classification results
Table 4 presents the performance comparison of various prediction models on both the training and testing datasets. These models encompass the following: the NeuroM model exclusively relies on neuroimaging features. The ClinicalM model utilizes solely clinical scales. The CombineM model is constructed by amalgamating neuroimaging features with clinical scales. The evaluation aimed to assess the predictive capabilities of these models in the context of PD diagnosis.
Table 4
Model | Dataset | F1_score | Recall | Precision | AUC | ACC |
---|---|---|---|---|---|---|
ClinicalM | Training dataset | 0.481 | 0.433 | 0.684 | 0.677 | 0.576 |
Testing dataset | 0.617 | 0.644 | 0.592 | 0.707 | 0.609 | |
NeuroM | Training dataset | 0.772 | 0.755 | 0.794 | 0.865 | 0.778 |
Testing dataset | 0.767 | 0.733 | 0.805 | 0.831 | 0.783 | |
CombineM | Training dataset | 0.816 | 0.812 | 0.829 | 0.883 | 0.816 |
Testing dataset | 0.800 | 0.800 | 0.800 | 0.844 | 0.804 |
AUC, area under the receiver operating characteristic curve; ACC, accuracy.
Using solely neuroimaging markers for early PD prediction, the validation set yielded AUC and ACC scores of 0.865 and 0.778, respectively, whereas the test resulted in scores of 0.831 (AUC) and 0.783 (ACC). When utilizing only clinical information for early PD prediction, the evaluation set showed AUC and ACC scores of 0.667 and 0.576, respectively, with the test set producing scores of 0.707 (AUC) and 0.609 (ACC). However, by amalgamating neuroimaging features and clinical data, the predictive performance for early PD improved significantly. In the validation set, AUC and ACC reached 0.883 and 0.816, respectively, whereas in the test set, they reached 0.844 (AUC) and 0.804 (ACC). Additionally, F1_score, recall, and precision values all exceeded 0.800.
Figure 9 visually depicts the prediction performance of various models in the test set using ROC curves. Neuroimaging features exhibited strong predictive capabilities, and when combined with clinical indicators, the CombineM model demonstrated superior predictive performance (AUC =0.844).
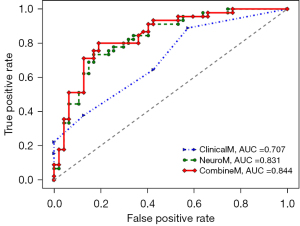
Ablation experiment
Table 5 illustrates the influence of varying feature numbers on the predictive performance of both the training and test sets. Notably, when the feature count was set to 15, both the training and test sets exhibited robust predictive performance.
Table 5
Feature numbers | Training dataset | Testing dataset | |||||||||
---|---|---|---|---|---|---|---|---|---|---|---|
AUC | ACC | F1_score | Recall | Precision | AUC | ACC | F1_score | Recall | Precision | ||
1 | 0.767 | 0.688 | 0.707 | 0.748 | 0.675 | 0.796 | 0.739 | 0.676 | 0.556 | 0.862 | |
2 | 0.816 | 0.746 | 0.743 | 0.741 | 0.75 | 0.816 | 0.739 | 0.755 | 0.822 | 0.698 | |
3 | 0.843 | 0.76 | 0.762 | 0.767 | 0.762 | 0.766 | 0.739 | 0.721 | 0.689 | 0.756 | |
4 | 0.856 | 0.764 | 0.771 | 0.786 | 0.758 | 0.797 | 0.761 | 0.756 | 0.756 | 0.756 | |
5 | 0.858 | 0.793 | 0.789 | 0.777 | 0.812 | 0.79 | 0.772 | 0.759 | 0.733 | 0.786 | |
6 | 0.861 | 0.792 | 0.795 | 0.796 | 0.81 | 0.796 | 0.783 | 0.773 | 0.756 | 0.791 | |
7 | 0.854 | 0.773 | 0.77 | 0.748 | 0.807 | 0.817 | 0.815 | 0.805 | 0.778 | 0.833 | |
8 | 0.86 | 0.778 | 0.782 | 0.786 | 0.783 | 0.805 | 0.804 | 0.8 | 0.8 | 0.8 | |
9 | 0.874 | 0.783 | 0.786 | 0.786 | 0.794 | 0.8 | 0.783 | 0.756 | 0.689 | 0.838 | |
10 | 0.877 | 0.797 | 0.797 | 0.795 | 0.807 | 0.81 | 0.772 | 0.764 | 0.756 | 0.773 | |
11 | 0.875 | 0.792 | 0.794 | 0.795 | 0.799 | 0.811 | 0.793 | 0.782 | 0.756 | 0.81 | |
12 | 0.886 | 0.792 | 0.796 | 0.805 | 0.796 | 0.835 | 0.793 | 0.782 | 0.756 | 0.81 | |
13 | 0.885 | 0.797 | 0.799 | 0.794 | 0.807 | 0.819 | 0.783 | 0.783 | 0.8 | 0.766 | |
14 | 0.886 | 0.797 | 0.797 | 0.785 | 0.814 | 0.825 | 0.783 | 0.767 | 0.733 | 0.805 | |
15 | 0.883 | 0.816 | 0.816 | 0.812 | 0.829 | 0.844 | 0.804 | 0.8 | 0.8 | 0.8 | |
16 | 0.882 | 0.792 | 0.789 | 0.775 | 0.811 | 0.843 | 0.804 | 0.791 | 0.756 | 0.829 | |
17 | 0.882 | 0.806 | 0.806 | 0.803 | 0.816 | 0.842 | 0.783 | 0.773 | 0.756 | 0.791 | |
18 | 0.884 | 0.816 | 0.819 | 0.831 | 0.812 | 0.835 | 0.783 | 0.778 | 0.778 | 0.778 | |
19 | 0.883 | 0.806 | 0.804 | 0.794 | 0.827 | 0.837 | 0.772 | 0.764 | 0.756 | 0.773 | |
20 | 0.882 | 0.816 | 0.818 | 0.822 | 0.82 | 0.838 | 0.772 | 0.764 | 0.756 | 0.773 | |
21 | 0.881 | 0.797 | 0.793 | 0.775 | 0.822 | 0.841 | 0.772 | 0.769 | 0.778 | 0.761 | |
22 | 0.885 | 0.792 | 0.786 | 0.766 | 0.822 | 0.833 | 0.761 | 0.744 | 0.711 | 0.78 | |
23 | 0.879 | 0.792 | 0.79 | 0.775 | 0.811 | 0.834 | 0.783 | 0.773 | 0.756 | 0.791 | |
24 | 0.878 | 0.802 | 0.798 | 0.784 | 0.824 | 0.838 | 0.772 | 0.753 | 0.711 | 0.8 | |
25 | 0.875 | 0.811 | 0.81 | 0.803 | 0.826 | 0.836 | 0.761 | 0.744 | 0.711 | 0.78 | |
26 | 0.876 | 0.802 | 0.8 | 0.794 | 0.814 | 0.836 | 0.761 | 0.744 | 0.711 | 0.78 | |
27 | 0.88 | 0.801 | 0.797 | 0.784 | 0.823 | 0.834 | 0.772 | 0.759 | 0.733 | 0.786 | |
28 | 0.878 | 0.802 | 0.798 | 0.784 | 0.824 | 0.841 | 0.761 | 0.761 | 0.778 | 0.745 | |
29 | 0.88 | 0.806 | 0.804 | 0.794 | 0.825 | 0.836 | 0.783 | 0.75 | 0.667 | 0.857 | |
30 | 0.88 | 0.797 | 0.795 | 0.784 | 0.816 | 0.837 | 0.772 | 0.779 | 0.822 | 0.74 |
AUC, area under the receiver operating characteristic curve; ACC, accuracy.
Discussion
PD represents an irreversible, chronic, degenerative central nervous system disorder. In its early asymptomatic stages, subtle changes can occur in the brain structure, with ongoing structural alterations as the disease progresses over time. PD’s etiology remains unclear, and no cure currently exists; medications merely aim to alleviate symptoms. The development of biomarkers for early PD diagnosis is crucial for comprehending disease progression early on and intervening to reduce health risks.
The PPMI project has been tracking various PD patient types for over a decade, conducting comprehensive observational clinical research involving multiple centers, sequences, and time series. However, prior studies utilizing the PPMI database (38,39) often lacked clear criteria for the neuroimaging data selection. They either listed the numbers of PD patients and NC without detailed matching criteria or used discontinuously matched data, typically lacking records over a continuous 10-year period, leading to potential bias. In this study, we meticulously screened and investigated DTI data from cases enrolled at the baseline of the project’s initiation. We employed DTI-based TBSS methods to explore WM changes in early-stage PD patients, analyzing and identifying lesion regions. Furthermore, we assessed the potential of this area as an image biomarker for early PD diagnosis through ML. Our individual level prediction model achieved an impressive AUC of 0.844 in the test set.
Presently, many studies employ multimodal data to predict PD disease states. However, obtaining multimodal data is more challenging and costly compared to single MRI modality, and it often involves lengthy scanning times. Additionally, due to its high noise and complexity, multimodal data may not be suitable for clinical applications (40). In our study, we utilized a single DTI modality to achieve high classification accuracy, reducing patient discomfort from extended scanning times, minimizing detection errors due to head movement, and saving the patients’ expenses and medical resources while maximizing data utility. Furthermore, our proposed predictive model offers preliminary screening assistance to radiologists in their diagnostic efforts.
DTI data can be collected automatically using a 3T scanner in under 12 minutes, eliminating the need for radioactive tracers. Implementing our approach could positively impact the clinical management of PD patients and reduce misdiagnoses in clinical trials.
Despite the absence of visually apparent brain MRI changes in PD, DTI analysis with ML algorithms offers highly accurate individual PD patient detection, presenting potential applications in clinical neuroradiology. Leveraging existing MRI data through advanced data analysis methods can provide a cost-effective means for early and specific diagnosis. Our aim is not to replace existing methods but rather to complement them comprehensively.
Impact of PD on brain WM
Numerous neuroimaging studies have employed ROI approaches (41) to explore microscopic brain structure alterations associated with PD. These studies typically rely on a priori knowledge to guide ROI and feature selection, which can be subjective and may overlook changes occurring beyond the selected areas. Notably, no single brain region, such as substantia nigra (SN), has consistently demonstrated DTI-derived image metrics as reliable a priori markers for validating the course and severity of early PD (42). Moreover, conflicting studies exist regarding whether the FA value of the SN can serve as a diagnostic imaging marker (42-44).
In this study, we employed the TBSS method to analyze FA values, investigating abnormal WM microstructure in PD patients. Previous PD research has identified changes in the overall integrity of specific brain WM regions (24). FA, a comprehensive measure of microstructural integrity, is linked to myelin integrity and fiber density (45). Although the precise mechanisms underlying extensive microstructural disruptions in early-stage PD remain unclear, our findings suggest the presence of highly relevant ROIs within brain WM. Information related to the structure of these ROIs may hold implications for early and accurate PD prediction, as well as cognitive brain function.
Through the standard TBSS process, FA images from all cases are mapped onto the average FA skeleton through non-linear registration. Skeletonization enhances the accuracy of inter-group comparisons, with skeleton areas representing statistically significant (P<0.05) WM regions between the PD and NC groups. Figure S1 displays the WM skeleton (red) extracted from any case’s brain image. The WM skeleton highlights brain regions potentially associated with PD, with deeper red indicating higher relevance to PD. Consequently, the skeleton (ROI) can serve as an image biomarker for PD, and changes in its diffusion quantitative parameters can provide valuable diagnostic information for physicians (46).
Preliminary research results on the skeleton ROI (Figures 5,6, Table 3) demonstrate that FA values in PD patients are significantly reduced compared to those in NC cases, primarily distributed in commissural fibers and projection fibers. This observation aligns with previous research findings (24,44). Notably, decreased FA in PD patients’ WM is evident in bilateral anterior thalamic radiations (ATRs), corticospinal tracts (CSTs), cingulum (cingulate gyrus) (CgC), cingulum (hippocampus) (CgH), forceps major (Fma), inferior fronto-occipital fasciculus (IFOF), inferior longitudinal fasciculus (ILF), superior longitudinal fasciculus (SLF), uncinate fasciculus (UF), and superior longitudinal fasciculus (temporal part) (tSLF). Among these, bilateral ATR, IFOF, ILF, SLF, and right Fma notably exhibit reduced FA values.
The ATR (47) consists of fibers connecting the medial thalamic nucleus and the frontal cortex, with the former related to memory and emotional regulation and the latter associated with motor, memory, and emotional functions. The IFOF, spanning from the occipital lobe to the frontal lobe, is the longest combined fiber bundle in the brain and plays a role in cognitive and attention functions (48). The ILF, positioned outside the visual radiation, links the anterior temporal lobe and the dorsolateral region of the occipital lobe, contributing to visual learning and memory (49). The SLF comprises 2 parallel pathways connecting the temporal, parietal, occipital, and frontal lobes and is associated with language processes (50). The Fma, a major fiber bundle, originates from the splenium of the corpus callosum and extends to connect the occipital lobe. Prior studies have implicated these regions in various cognitive and emotional processes. The microstructural damage in these regions suggests abnormalities in the brain WM of early-stage PD patients, correlating with cognitive, attentional, emotional, and other related functional impairments occurring in the early stages of PD. Future research focusing on changes in these brain WM regions and their potential links to specific symptoms may provide valuable insights.
The study cohort consisted of 152 PD and 75 NC participants, all of whom completed the MoCA. When considering the individuals with values from other assessment scales (such as Movement Disorder Society Unified Parkinson’s Disease Rating Scale, MDS-UPDRS; H&Y; University of Pennsylvania Smell Identification Test, UPSIT; Geriatric Depression Scale, GDS; Scales for Outcomes in Parkinson’s Disease - Autonomic, SCOPA-AUT, and so on), the number of participants reduces to 146 in the PD group and 56 in the NC group. Due to the limited number of participants, the primary experimental framework of this study did not include data from other scales. However, statistical analysis of the relevant scales for this part of the data is provided in Table S3 (Appendix 1). There were significant statistical differences in the results of the MDS-UPDRS, H&Y, UPSIT, MoCA, GDS, and SCOPA-AUT between the two groups of participants. Therefore, the WM fiber bundles with differential regions between the two groups are related to both advanced cognitive function and motor symptoms, showing that in the early stages of PD, the impact of disease on brain structure has already affected cognitive and motor functions.
In the early stages of PD, the patient’s cognitive function has already experienced certain damage. Due to different cognitive functions, there may also be certain differences in the structural damage of WM fibers caused by PD (51). In this case, MoCA results could indeed reflect cognitive dysfunction itself, and therefore can serve as an important factor in identifying early suspected PD patients. The WM damage reflects the cognitive function of PD patients, which can serve as one of the effective diagnostic factors and further improve the diagnostic accuracy of the model. This is consistent with the research results of this article.
The purpose of this study was to screen and identify PD patients early. Cognitive function, as a complex brain function, exhibits significant differences between NC and PD patients. Therefore, cognitive decline can be one of the effective indicators for early diagnosis models of PD. This study developed an early diagnosis model for PD based on imaging data and clinical MoCA results, which can assist clinicians in identifying individuals with early-stage PD as much as possible, and initiate intervention measures as early as possible to reduce the serious health threats caused by the disease.
Artificial intelligence (AI)-based explainable early PD prediction model
In the realm of AI, the decision-making process of numerous ML or deep learning models often remains opaque to human understanding (5,10,40), lacking tangible and reproducible results. Particularly in high-risk medical research areas, interpretability becomes a pivotal concern (8). This study’s strength lies in the model’s ability to select highly disease-correlated and interpretable features. Moreover, the results demonstrate minimal deviation between the unseen dataset (test set) and the training set (5-fold cross-validation results). This illustrates the model’s smooth operation and robust generalization, fostering a certain level of trust among end-users, including clinicians (Table 4 and Figure 9).
In conjunction with traditional methods, leveraging statistically significant disease lesion areas as neuroimaging biomarkers not only enhances our understanding of the disease but also assists physicians in formulating personalized treatment plans and gauging patient’s responses to those plans (52). This approach facilitates real-time monitoring of disease progression and the evaluation of clinical treatment effectiveness, ultimately playing a crucial role in treatment selection and prognosis prediction.
Scientific advancements and clinical applications rely heavily on independently reproducible research results, yet most current research algorithms heavily depend on trained models. When replicating methods from the literature, the source of biases in the results remains uncertain, especially regarding the methods and parameter settings employed in the preprocessing phase, which can significantly impact outcomes (53). The merit of this study lies in its meticulous documentation of key steps and parameters utilized in data grouping and preprocessing from the outset, ensuring the reliability and reproducibility of the results.
The MoCA (37), developed by Professor Nasreddine in 2004, served as a rapid screening tool for MCI. It assesses various cognitive domains, including attention, executive function, memory, language, visual-spatial skills, abstract thinking, calculation, and orientation. The scale’s total score is 30 points, with a normal control typically scoring no less than 26 points. MCI is common in patients with early PD before the onset of motor impairment, and is associated with the risk of dementia (54). MoCA is currently utilized as a global cognitive screening tool and has been recommended as a simplified diagnostic tool for assessing MCI within the broader context of global cognitive function (55). Consequently, it is reasonable to incorporate the MoCA scale as a clinical information tool for predicting diseases in the development of clinical predictive models (56).
Some previous AI-based interpretable PD prediction models have been hindered by small dataset sizes, incorporating as few as 45 PD patients (57), 29 PD patients (58), and 44 PD patients (59). Additionally, some studies have involved longer disease durations, with the primary dataset indicating disease duration of 10.0±6.2 years, 15.8±13.3 years in external datasets (60), or 6.3±4.1 years in mid-to-late stage PD (61). The strength of our model lies in its utilization of publicly available multi-center datasets for early PD prediction, achieving high accuracy (ACC =0.804, AUC =0.844 in the test set), underscoring its generalizability.
Limitations, challenges, and future prospects
In the diagnosis of PD, DTI can provide information about the WM fiber bundles in the midbrain SN, including their integrity and connectivity. By evaluating the microstructure and connectivity of the SN in the midbrain, DTI can help doctors make a clear diagnosis of PD.
The melanin images and magnetic susceptibility images of the SN in the midbrain are usually obtained using other MRI techniques. Magnetic susceptibility imaging (MSI) is used to display the structure of tissues, whereas melanin imaging typically uses a special MRI sequence to display the melanin content in the SN of the midbrain.
Although DTI is not directly used to evaluate melanin images or magnetic susceptibility images, combining DTI and these MRI techniques can provide a more comprehensive assessment of the nervous system, helping to diagnose and study diseases related to the midbrain SN, such as PD (62).
In future research, we aim to conduct a comprehensive analysis of the SN by integrating DTI images with other modal MRI images such as MSI. Our goal is to provide additional perspectives for the study of diseases related to the midbrain SN, including PD.
The TBSS method can effectively compare the structural differences of WM fiber bundles in different groups. The brain regions with structural differences between PD patients and normal cases may be affected by PD lesions and require special attention.
Currently, TBSS is primarily applied to DTI images. To further extend the application of TBSS, diffusion kurtosis imaging (DKI) (63) scans can be performed on suspected PD patients in the future, and TBSS calculations can be performed on DKI.
DKI is an advanced DTI technique, and compared to DTI, DKI is more suitable for detecting WM properties in complex fiber regions and can provide richer and relatively more detailed microstructure information. In addition to obtaining traditional diffusion parameters, such as anisotropy of diffusion tensors (FA) and mean diffusion rate (MD), DKI introduces unique parameters such as mean kurtosis (MK), which can better reflect the complexity and non-Gaussian nature or tissue microstructure.
Compared to DTI, DKI can provide a more comprehensive set of scalar metrics. MK is more sensitive to complex fiber regions than FA and can more accurately estimate the diffusion distribution function of water molecules. This makes DKI, and particularly MK, valuable for exploring PD pathological regions with greater accuracy.
Although this study has yielded highly satisfactory results, several limitations need to be recognized: Firstly, brain changes in the early stages of PD may already exist before clinical symptoms manifest. Consequently, DTI may not be the optimal tool for evaluating early PD abnormalities, as functional changes might precede structural abnormalities. Nevertheless, DTI analysis can still serve as a valuable means to distinguish disease stages, particularly as FA and MD values could potentially serve as quantitative markers for tracking disease progression. Secondly, this study adopted a cross-sectional research design, whereas longitudinal research methods would be better suited to investigate disease progression over time. Thirdly, the generalizability of our findings should be further validated in a larger population, with particular attention to variations in disease severity. The patient population in this study is more than twice the size of the control group, leading to potential exaggeration of AUC and other accuracy metrics; also, the experiment lacked external validation, and a larger database of cases could solve this problem. To address these limitations, we plan to refine our research direction and content in future investigations.
Conclusions
This study has successfully constructed an early PD prediction model using a large-sample multi-center dataset from PPMI. Rigorous screening of DTI sequences from cases enrolled at the project’s baseline year, alongside a standardized data processing pipeline and data-driven cross-validation across multiple centers, facilitated the systematic evaluation of WM abnormality patterns in PD. Moreover, the study assessed the utility and generalizability of WM features in PD identification, affirming their potential as imaging marker for PD recognition. These findings offer a promising avenue for future clinical translational applications in PD diagnosis and management.
Acknowledgments
Funding: This study was supported by
Footnote
Reporting Checklist: The authors have completed the TRIPOD reporting checklist. Available at https://qims.amegroups.com/article/view/10.21037/qims-23-1478/rc
Conflicts of Interest: All authors have completed the ICMJE uniform disclosure form (available at https://qims.amegroups.com/article/view/10.21037/qims-23-1478/coif). The authors have no conflicts of interest to declare.
Ethical Statement: The authors are accountable for all aspects of the work in ensuring that questions related to the accuracy or integrity of any part of the work are appropriately investigated and resolved. The study was conducted in accordance with the Declaration of Helsinki (as revised in 2013).
Open Access Statement: This is an Open Access article distributed in accordance with the Creative Commons Attribution-NonCommercial-NoDerivs 4.0 International License (CC BY-NC-ND 4.0), which permits the non-commercial replication and distribution of the article with the strict proviso that no changes or edits are made and the original work is properly cited (including links to both the formal publication through the relevant DOI and the license). See: https://creativecommons.org/licenses/by-nc-nd/4.0/.
References
- Amoroso N, La Rocca M, Monaco A, Bellotti R, Tangaro S. Complex networks reveal early MRI markers of Parkinson's disease. Med Image Anal 2018;48:12-24. [Crossref] [PubMed]
- Yap TE, Balendra SI, Almonte MT, Cordeiro MF. Retinal correlates of neurological disorders. Ther Adv Chronic Dis 2019;10:2040622319882205. [Crossref] [PubMed]
- Miller-Patterson C, Han J, Yaffe K, Rosso AL, Launer LJ, Kritchevsky SB, Boudreau RM, Rosano C. Clinical and neuroimaging correlates of progression of mild parkinsonian signs in community-dwelling older adults. Parkinsonism Relat Disord 2020;75:85-90. [Crossref] [PubMed]
- Koirala N, Anwar AR, Ciolac D, Glaser M, Pintea B, Deuschl G, Muthuraman M, Groppa S. Alterations in White Matter Network and Microstructural Integrity Differentiate Parkinson's Disease Patients and Healthy Subjects. Front Aging Neurosci 2019;11:191. [Crossref] [PubMed]
- Chaki J, Woźniak M. Deep learning for neurodegenerative disorder (2016 to 2022): A systematic review. Biomed Signal Process Control 2023;80:104223.
- Nilashi M, Abumalloh RA, Minaei-Bidgoli B, Samad S, Yousoof Ismail M, Alhargan A, Abdu Zogaan W. Predicting Parkinson's Disease Progression: Evaluation of Ensemble Methods in Machine Learning. J Healthc Eng 2022;2022:2793361. [Crossref] [PubMed]
- Bian J, Wang X, Hao W, Zhang G, Wang Y. The differential diagnosis value of radiomics-based machine learning in Parkinson's disease: a systematic review and meta-analysis. Front Aging Neurosci 2023;15:1199826. [Crossref] [PubMed]
- Papadimitroulas P, Brocki L, Christopher Chung N, Marchadour W, Vermet F, Gaubert L, Eleftheriadis V, Plachouris D, Visvikis D, Kagadis GC, Hatt M. Artificial intelligence: Deep learning in oncological radiomics and challenges of interpretability and data harmonization. Phys Med 2021;83:108-21. [Crossref] [PubMed]
- Guan X, Huang P, Zeng Q, Liu C, Wei H, Xuan M, Gu Q, Xu X, Wang N, Yu X, Luo X, Zhang M. Quantitative susceptibility mapping as a biomarker for evaluating white matter alterations in Parkinson's disease. Brain Imaging Behav 2019;13:220-31. [Crossref] [PubMed]
- Sun X, Ge J, Li L, Zhang Q, Lin W, Chen Y, Wu P, Yang L, Zuo C, Jiang J. Use of deep learning-based radiomics to differentiate Parkinson's disease patients from normal controls: a study based on [18F]FDG PET imaging. Eur Radiol 2022;32:8008-18.
- Sanjari Moghaddam H, Dolatshahi M, Mohebi F, Aarabi MH. Structural white matter alterations as compensatory mechanisms in Parkinson's disease: A systematic review of diffusion tensor imaging studies. J Neurosci Res 2020;98:1398-416. [Crossref] [PubMed]
- Deng XY, Wang L, Yang TT, Li R, Yu G. A meta-analysis of diffusion tensor imaging of substantia nigra in patients with Parkinson's disease. Sci Rep 2018;8:2941. [Crossref] [PubMed]
- Xue T, Zhang F, Zhang C, Chen Y, Song Y, Golby AJ, Makris N, Rathi Y, Cai W, O'Donnell LJ. Superficial white matter analysis: An efficient point-cloud-based deep learning framework with supervised contrastive learning for consistent tractography parcellation across populations and dMRI acquisitions. Med Image Anal 2023;85:102759. [Crossref] [PubMed]
- Bergamino M, Keeling EG, Mishra VR, Stokes AM, Walsh RR. Assessing White Matter Pathology in Early-Stage Parkinson Disease Using Diffusion MRI: A Systematic Review. Front Neurol 2020;11:314. [Crossref] [PubMed]
- Cao X, Wang X, Xue C, Zhang S, Huang Q, Liu W. A Radiomics Approach to Predicting Parkinson's Disease by Incorporating Whole-Brain Functional Activity and Gray Matter Structure. Front Neurosci 2020;14:751. [Crossref] [PubMed]
- Lorio S, Sambataro F, Bertolino A, Draganski B, Dukart J. The Combination of DAT-SPECT, Structural and Diffusion MRI Predicts Clinical Progression in Parkinson's Disease. Front Aging Neurosci 2019;11:57. [Crossref] [PubMed]
- Cnyrim CD, Kupsch A, Ebersbach G, Hoffmann KT. Diffusion tensor imaging in idiopathic Parkinson's disease and multisystem atrophy (Parkinsonian type). Neurodegener Dis 2014;13:1-8. [Crossref] [PubMed]
- Zhong Y, Liu H, Liu G, Liang Y, Dai C, Zhao L, Lai H, Mo L, Tan C, Deng F, Liu X, Chen L. Cerebellar and cerebral white matter changes in Parkinson's disease with resting tremor. Neuroradiology 2023;65:1497-506. [Crossref] [PubMed]
- Gou L, Zhang W, Li C, Shi X, Zhou Z, Zhong W, Chen T, Wu X, Yang C, Guo D. Structural Brain Network Alteration and its Correlation With Structural Impairments in Patients With Depression in de novo and Drug-Naïve Parkinson's Disease. Front Neurol 2018;9:608. [Crossref] [PubMed]
- Wen MC, Heng HSE, Lu Z, Xu Z, Chan LL, Tan EK, Tan LCS. Differential White Matter Regional Alterations in Motor Subtypes of Early Drug-Naive Parkinson's Disease Patients. Neurorehabil Neural Repair 2018;32:129-41. [Crossref] [PubMed]
- Duan F, Zhao T, He Y, Shu N. Test-retest reliability of diffusion measures in cerebral white matter: A multiband diffusion MRI study. J Magn Reson Imaging 2015;42:1106-16. [Crossref] [PubMed]
- Wei X, Luo C, Li Q, Hu N, Xiao Y, Liu N, Lui S, Gong Q. White Matter Abnormalities in Patients With Parkinson's Disease: A Meta-Analysis of Diffusion Tensor Imaging Using Tract-Based Spatial Statistics. Front Aging Neurosci 2020;12:610962. [Crossref] [PubMed]
- Li D, Xu H, Yang Q, Zhang M, Wang Y. Cerebral White Matter Alterations Revealed by Multiple Diffusion Metrics in Cervical Spondylotic Patients with Pain: A TBSS Study. Pain Med 2022;23:895-901. [Crossref] [PubMed]
- Li XR, Ren YD, Cao B, Huang XL. Analysis of white matter characteristics with tract-based spatial statistics according to diffusion tensor imaging in early Parkinson's disease. Neurosci Lett 2018;675:127-32. [Crossref] [PubMed]
- Cova I, Priori A. Diagnostic biomarkers for Parkinson's disease at a glance: where are we? J Neural Transm (Vienna) 2018;125:1417-32. [Crossref] [PubMed]
- Khan AR, Hiebert NM, Vo A, Wang BT, Owen AM, Seergobin KN, MacDonald PA. Biomarkers of Parkinson's disease: Striatal sub-regional structural morphometry and diffusion MRI. Neuroimage Clin 2019;21:101597. [Crossref] [PubMed]
- Shastry KA. An ensemble nearest neighbor boosting technique for prediction of Parkinson’s disease. Healthcare Analytics 2023;3:100181.
- Cui X, Chen N, Zhao C, Li J, Zheng X, Liu C, Yang J, Li X, Yu C, Liu J, Liu X. An adaptive weighted attention-enhanced deep convolutional neural network for classification of MRI images of Parkinson's disease. J Neurosci Methods 2023;394:109884. [Crossref] [PubMed]
- Tibshirani R. Regression Shrinkage and Selection Via the Lasso. J R Stat Soc 1996;58:267-88.
- Hoehn MM, Yahr MD. Parkinsonism: onset, progression and mortality. Neurology 1967;17:427-42. [Crossref] [PubMed]
- Ortiz A, Munilla J, Martínez-Ibañez M, Górriz JM, Ramírez J, Salas-Gonzalez D. Parkinson's Disease Detection Using Isosurfaces-Based Features and Convolutional Neural Networks. Front Neuroinform 2019;13:48. [Crossref] [PubMed]
- Muñoz-Ramírez V, Kmetzsch V, Forbes F, Meoni S, Moro E, Dojat M. Subtle anomaly detection: Application to brain MRI analysis of de novo Parkinsonian patients. Artif Intell Med 2022;125:102251. [Crossref] [PubMed]
- Guan XJ, Guo T, Zhou C, Gao T, Wu JJ, Han V, Cao S, Wei HJ, Zhang YY, Xuan M, Gu QQ, Huang PY, Liu CL, Pu JL, Zhang BR, Cui F, Xu XJ, Zhang MM. A multiple-tissue-specific magnetic resonance imaging model for diagnosing Parkinson’s disease: a brain radiomics study. Neural Regen Res 2022;17:2743-9. [Crossref] [PubMed]
- Shi D, Ren Z, Zhang H, Wang G, Guo Q, Wang S, Ding J, Yao X, Li Y, Ren K. Amplitude of low-frequency fluctuation-based regional radiomics similarity network: Biomarker for Parkinson's disease. Heliyon 2023;9:e14325. [Crossref] [PubMed]
- Wu Y, Jiang JH, Chen L, Lu JY, Ge JJ, Liu FT, Yu JT, Lin W, Zuo CT, Wang J. Use of radiomic features and support vector machine to distinguish Parkinson's disease cases from normal controls. Ann Transl Med 2019;7:773. [Crossref] [PubMed]
- Ren Q, Wang Y, Leng S, Nan X, Zhang B, Shuai X, Zhang J, Xia X, Li Y, Ge Y, Meng X, Zhao C. Substantia Nigra Radiomics Feature Extraction of Parkinson's Disease Based on Magnitude Images of Susceptibility-Weighted Imaging. Front Neurosci 2021;15:646617. [Crossref] [PubMed]
- Nasreddine ZS, Phillips NA, Bédirian V, Charbonneau S, Whitehead V, Collin I, Cummings JL, Chertkow H. The Montreal Cognitive Assessment, MoCA: a brief screening tool for mild cognitive impairment. J Am Geriatr Soc 2005;53:695-9. [Crossref] [PubMed]
- Gerraty RT, Provost A, Li L, Wagner E, Haas M, Lancashire L. Machine learning within the Parkinson's progression markers initiative: Review of the current state of affairs. Front Aging Neurosci 2023;15:1076657. [Crossref] [PubMed]
- Tafuri B, Lombardi A, Nigro S, Urso D, Monaco A, Pantaleo E, Diacono D, De Blasi R, Bellotti R, Tangaro S, Logroscino G. The impact of harmonization on radiomic features in Parkinson's disease and healthy controls: A multicenter study. Front Neurosci 2022;16:1012287. [Crossref] [PubMed]
- Warren SL, Moustafa AA. Functional magnetic resonance imaging, deep learning, and Alzheimer's disease: A systematic review. J Neuroimaging 2023;33:5-18. [Crossref] [PubMed]
- Amandola M, Sinha A, Amandola MJ, Leung HC. Longitudinal corpus callosum microstructural decline in early-stage Parkinson's disease in association with akinetic-rigid symptom severity. NPJ Parkinsons Dis 2022;8:108. [Crossref] [PubMed]
- Mishra VR, Sreenivasan KR, Zhuang X, Yang Z, Cordes D, Walsh RR. Influence of analytic techniques on comparing DTI-derived measurements in early stage Parkinson's disease. Heliyon 2019;5:e01481. [Crossref] [PubMed]
- Lenfeldt N, Larsson A, Nyberg L, Birgander R, Forsgren L. Fractional anisotropy in the substantia nigra in Parkinson's disease: a complex picture. Eur J Neurol 2015;22:1408-14. [Crossref] [PubMed]
- Schwarz ST, Abaei M, Gontu V, Morgan PS, Bajaj N, Auer DP. Diffusion tensor imaging of nigral degeneration in Parkinson's disease: A region-of-interest and voxel-based study at 3 T and systematic review with meta-analysis. Neuroimage Clin 2013;3:481-8. [Crossref] [PubMed]
- Mei Y, Wang W, Qiu D, Yuan Z, Bai X, Tang H, Zhang P, Zhang X, Zhang Y, Yu X, Sui B, Wang Y. Micro-structural white matter abnormalities in new daily persistent headache: a DTI study using TBSS analysis. J Headache Pain 2023;24:80. [Crossref] [PubMed]
- Salvatore C, Cerasa A, Castiglioni I, Gallivanone F, Augimeri A, Lopez M, Arabia G, Morelli M, Gilardi MC, Quattrone A. Machine learning on brain MRI data for differential diagnosis of Parkinson's disease and Progressive Supranuclear Palsy. J Neurosci Methods 2014;222:230-7. [Crossref] [PubMed]
- Bubb EJ, Metzler-Baddeley C, Aggleton JP. The cingulum bundle: Anatomy, function, and dysfunction. Neurosci Biobehav Rev 2018;92:104-27. [Crossref] [PubMed]
- Conner AK, Briggs RG, Sali G, Rahimi M, Baker CM, Burks JD, Glenn CA, Battiste JD, Sughrue ME. A Connectomic Atlas of the Human Cerebrum-Chapter 13: Tractographic Description of the Inferior Fronto-Occipital Fasciculus. Oper Neurosurg (Hagerstown) 2018;15:S436-43. [Crossref] [PubMed]
- Latini F, Mårtensson J, Larsson EM, Fredrikson M, Åhs F, Hjortberg M, Aldskogius H, Ryttlefors M. Segmentation of the inferior longitudinal fasciculus in the human brain: A white matter dissection and diffusion tensor tractography study. Brain Res 2017;1675:102-15. [Crossref] [PubMed]
- Koshiyama D, Fukunaga M, Okada N, Morita K, Nemoto K, Yamashita F, Yamamori H, Yasuda Y, Matsumoto J, Fujimoto M, Kudo N, Azechi H, Watanabe Y, Kasai K, Hashimoto R. Association between the superior longitudinal fasciculus and perceptual organization and working memory: A diffusion tensor imaging study. Neurosci Lett 2020;738:135349. [Crossref] [PubMed]
- Sang T, He J, Wang J, Zhang C, Zhou W, Zeng Q, Yuan Y, Yu L, Feng Y. Alterations in white matter fiber in Parkinson disease across different cognitive stages. Neurosci Lett 2022;769:136424. [Crossref] [PubMed]
- Walsh RR. Functional imaging markers as outcome measures in clinical trials for Parkinson’s disease. In: Galvez-Jimenez N, Fernandez HH, Espay AJ, et al., editors. Parkinson’s Disease [Internet]. 1st ed. Cambridge University Press; 2016. p. 242-8.
- Baxter JSH, Jannin P. Validation in the age of machine learning: A framework for describing validation with examples in transcranial magnetic stimulation and deep brain stimulation. Intell-Based Med 2023;7:100090.
- Caviness JN, Driver-Dunckley E, Connor DJ, Sabbagh MN, Hentz JG, Noble B, Evidente VG, Shill HA, Adler CH. Defining mild cognitive impairment in Parkinson's disease. Mov Disord 2007;22:1272-7. [Crossref] [PubMed]
- Kletzel SL, Hernandez JM, Miskiel EF, Mallinson T, Pape TL. Evaluating the performance of the Montreal Cognitive Assessment in early stage Parkinson's disease. Parkinsonism Relat Disord 2017;37:58-64. [Crossref] [PubMed]
- Scheffels JF, Fröhlich L, Kalbe E, Kessler J. Concordance of Mini-Mental State Examination, Montreal Cognitive Assessment and Parkinson Neuropsychometric Dementia Assessment in the classification of cognitive performance in Parkinson's disease. J Neurol Sci 2020;412:116735. [Crossref] [PubMed]
- Shabanpour M, Kaboodvand N, Iravani B. Parkinson's disease is characterized by sub-second resting-state spatio-oscillatory patterns: A contribution from deep convolutional neural network. Neuroimage Clin 2022;36:103266. [Crossref] [PubMed]
- Alkhatib R, Diab MO, Corbier C, El Badaoui M. Machine Learning Algorithm for Gait Analysis and Classification on Early Detection of Parkinson. IEEE Sensors Letters 2020;4:1-4.
- Glaab E, Trezzi JP, Greuel A, Jäger C, Hodak Z, Drzezga A, Timmermann L, Tittgemeyer M, Diederich NJ, Eggers C. Integrative analysis of blood metabolomics and PET brain neuroimaging data for Parkinson's disease. Neurobiol Dis 2019;124:555-62. [Crossref] [PubMed]
- Le Berre A, Kamagata K, Otsuka Y, Andica C, Hatano T, Saccenti L, Ogawa T, Takeshige-Amano H, Wada A, Suzuki M, Hagiwara A, Irie R, Hori M, Oyama G, Shimo Y, Umemura A, Hattori N, Aoki S. Convolutional neural network-based segmentation can help in assessing the substantia nigra in neuromelanin MRI. Neuroradiology 2019;61:1387-95. [Crossref] [PubMed]
- Shinde S, Prasad S, Saboo Y, Kaushick R, Saini J, Pal PK, Ingalhalikar M. Predictive markers for Parkinson's disease using deep neural nets on neuromelanin sensitive MRI. Neuroimage Clin 2019;22:101748. [Crossref] [PubMed]
- Cassidy CM, Zucca FA, Girgis RR, Baker SC, Weinstein JJ, Sharp ME, Bellei C, Valmadre A, Vanegas N, Kegeles LS, Brucato G, Kang UJ, Sulzer D, Zecca L, Abi-Dargham A, Horga G. Neuromelanin-sensitive MRI as a noninvasive proxy measure of dopamine function in the human brain. Proc Natl Acad Sci U S A 2019;116:5108-17. [Crossref] [PubMed]
- Jensen JH, Helpern JA, Ramani A, Lu H, Kaczynski K. Diffusional kurtosis imaging: the quantification of non-gaussian water diffusion by means of magnetic resonance imaging. Magn Reson Med 2005;53:1432-40. [Crossref] [PubMed]