18F-fluorodeoxyglucose (18F-FDG) positron emission tomography/computed tomography (PET/CT) imaging of pediatric neuroblastoma: a multi-omics parameters method to predict MYCN copy number category
Introduction
Neuroblastoma (NB) is an embryonic tumor arising from primitive neural crest cells of the autonomic nervous system. It is the most common extracranial solid tumor in children, most often occurring in those under 5 years of age, with a median age of 17 months, and accounts for 15% of childhood cancer-related deaths (1). Pediatric NB is characterized by histological and biological heterogeneity, which is reflected in the diversity of its clinical behavior (2). MYCN amplification (MNA) can be detected in 20–30% of NB cases and is an independent risk factor for accurately identifying rapid disease progression and predicting poor outcome, regardless of age and clinical stage of the disease (3). Campbell et al. reported that in MNA tumors, a high MYCN copy number was associated with a poorer outcome than that of a low MYCN copy number (4). As genetically distinct clones could have different biologic aggressiveness, MYCN status varies within the tumor and in metastases. In order to acquire the MYCN status of these sites, several diagnostic biopsies are often required at different sites. Meanwhile, the phenomena may also reasonably explain the differences in response to treatment, and overall survival and MYCN copy number may change with tumor treatment and/or metastatic spread (5). However, a study (5) showed that histology was not predictive of the temporal or spatial pattern of MNA for NB, and its definition process was complex, expensive, invasive, and lagging. The most frequent problems associated with biopsy procedures include hemorrhage requiring transfusion, infection, intestinal obstruction, and pneumothorax, with reported major complication rates for core needle biopsy ranging from 0% to 10% and 3% to 48%, respectively (6,7). Therefore, a simple, non-invasive, accurate, and real-time method is needed to predict MYCN copy number preoperatively or during treatment, which would be more useful for patient risk stratification, disease management, and prognostic assessment.
18F-fluorodeoxyglucose (18F-FDG) positron emission tomography/computed tomography (PET/CT) is well known as a very useful imaging modality in the clinical oncology field. Previous studies have reported the clinical significance of 18F-FDG PET/CT in the diagnosis, treatment response, and prognosis evaluation of NB (8-10). Routine PET measures, however, frequently fail to capture intra-tumoral heterogeneity via the spatial distribution of metabolic activity throughout the entire tumor.
The primary objective of radiogenomics is to identify the relationships between picture features and genomic data (11). Imaging genotyping has the benefit of providing a comprehensive, non-invasive image of the tumor over biopsy-based techniques. More recently, radiogenomics has demonstrated potential for predicting particular gene expression patterns in a range of tumors utilizing CT-based radiomic analysis (12-14). In particular, the role of 18F-FDG PET/CT has been reported in the prediction of MNA and 1p and 11q aberrations (15). However, there has been limited research to predict MYCN copy number category in NB preoperatively. Therefore, we hypothesized that radiogenomics features extracted from PET/CT images could predict the MYCN copy number category.
The purpose of this study was to investigate the efficacy of 18F-FDG PET/CT multi-omics parameters (bio-omics and radiomics) for the prediction of MYCN copy number category of NB. Additionally, we investigated whether these clinical biological characteristics and PET metabolic parameters were correlated with MYCN copy number. We present this article in accordance with the STARD reporting checklist (available at https://qims.amegroups.com/article/view/10.21037/qims-23-494/rc).
Methods
Patients
All of the included NB patients underwent pre-therapy 18F-FDG PET/CT scans between March 2018 and September 2020 in Beijing Friendship Hospital Affiliated to Capital Medical University. The inclusion criteria were as follows: (I) pathologically confirmed NB; (II) age ≤18 years at first diagnosis; (III) patients who had not received any radiotherapy, chemotherapy, or surgical treatment before the first PET/CT examination; (IV) complete clinical information (mentioned in the text); (V) available MYCN copy number. A total of 33 instances were ultimately excluded, 20 of which lacked clinical data, and 13 of which had 1 of the aforementioned medications at the time of the initial diagnosis. This retrospective study was conducted in accordance with the Declaration of Helsinki (as revised in 2013). The study was approved by the Institutional Review Board of Beijing Friendship Hospital Affiliated to Capital Medical University (No. 2020-P2-091-02) and the requirement for written informed consent was waived due to the retrospective nature of the study.
Evaluation of the MYCN copy number
By employing frozen or paraffin-embedded tumor tissue or bone marrow with verified NB cells, interphase fluorescence in situ hybridization (FISH) was used to determine the MYCN copy number in the Neuroblastoma Reference Laboratory at Nationwide Children’s Hospital. According to the European Neuroblastoma Quality Assessment group’s recommendations (16), MYCN gain was defined as a 2- to 4-fold increase in signal in 20% or less of cells compared to the reference probe for MYCN wild-type. A >4-fold increase in MYCN signal in comparison to a reference probe was used to define MNA.
PET/CT image acquisition
All patients underwent whole body (from vertex to toes) scan on the PET/CT scanner (Biograph mCT-64 PET/CT; Siemens, Erlangen, Germany) in accordance with European Association of Nuclear Medicine guidelines (17). Before the injection, they were told to stop any vigorous exercise for at least 24 hours and fast for at least 6 hours. A quantity of 0.14 mCi/kg of 18F-FDG (provided by Beijing Atomic High-tech Co., Ltd., Beijing, China) was injected intravenously 40–60 minutes before the PET/CT scan. First, a low-dose CT scan could use an automated modulated tube current and 120 kV tube voltage was carried out for anatomical reference and attenuation correction. The CT image parameters were as follows: pixel size 0.586×0.586 mm, 2 mm slice thickness, and matrix size 512×512. The whole-body CT scan was followed immediately by a 2-minute PET scan for each bed position. PET images were reconstructed using time-of-flight (ToF) and the ordered subsets-expectation maximization (OSEM) technique. Attenuation corrections were applied during the reconstruction and a 5 mm Gaussian filter was applied to the PET images. The PET image parameters were as follows: pixel size 4.07×4.07 mm, 3 mm slice thickness, and matrix size 200×200.
Bio-omics parameters acquisition
Serum ferritin (SF), homovanillic acid (HVA), vanillylmandelic acid (VMA), neuron-specific enolase (NSE), age, International Neuroblastoma Staging System (INSS) stage (18), Children’s Oncology Group (COG) risk group (19), and lactate dehydrogenase (LDH) were the clinical parameters that were dichotomized at the group median. Biological variables of interest, determined centrally by the NB reference pathologists, included 11q aberration (unbalanced LOH15), 1p LOH (20), International Neuroblastoma Pathology Classification (INPC) histologic classification (21), and mitosis-karyorrhexis index (MKI) (22).
PET/CT radiographic features: 2 nuclear medicine specialists with 8 and 3 years of pediatric nuclear medicine experience respectively, independently reviewed the conventional images on a workstation (syngo.via, Siemens) and reported the following lesion features: (I) International Neuroblastoma Risk Group Staging System (INRGSS) (23); (II) primary site; (III) infiltration across the midline; (IV) calcification; (V) necrosis. All results were based on the consensus agreement between 2 nuclear medicine physicians.
PET quantitative parameters: the primary tumor delineation was performed using the fixed relative standardized uptake value (SUV) threshold method (24). In this method, 3-dimensional (3D) contours [i.e., volume of interest (VOI)] were drawn around voxels equal to or greater than 40% maximum SUV (SUVmax). Moreover, for manual validation due to high physiological urine tracer activity, after automatic segmentation, particular focus was placed on the tumor close to the bladder.
All steps were discussed and agreed upon by the 2 preceding nuclear medicine physicians. In the aforementioned VOI, standard PET quantitative parameters were assessed including SUVmax, metabolic tumor volume (MTV), and total lesion glycolysis (TLG) (TLG = SUVmean× MTV).
PET/CT radiomics feature selection
Pre-processing of the data, including voxel size adjustment of CT and PET data, is typically conducted before feature extraction. Radiomics features from both CT and PET images were computed within the same VOI.
In the previously mentioned VOI, radiomics features of CT and PET images were computed using LIFEx software (www.lifexsoft.org), which specifically computes radiomics features for VOIs containing a minimum of 64 voxels (25). A total of 1,016 radiomics features were obtained from PET and CT images. The feature selection procedure was performed as follows: (I) Mann-Whitney U test retention of features with P values less than 0.05; (II) Spearman correlation analysis and removal of features with a correlation coefficient less than 0.9; (III) least absolute shrinkage and selection operator (LASSO) was used to explore the informative features that correlated best with MYCN copy number. Radiomics features underwent a multistep selection process to overcome the limitations of traditional logistic regression methods related to overfitting and multicollinearity problems in modeling by using high-dimensional radiomics features. The workflow is presented in Figure 1.
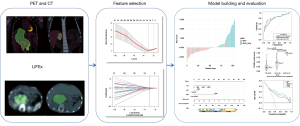
Establishment of the models
Univariable analysis was conducted to assess differences in clinical and biological features, PET/CT radiographic characteristics, and PET quantitative parameters between MYCN wild, MYCN gain, and MNA groups. Significantly distinct variables were incorporated into the multivariable backward stepwise logistic regression analysis. Utilizing the selected features, logistic regression models were individually established for each type of feature and then amalgamated to construct the Bio-omics model (B-model).
The significantly different radiomics features were screened to establish radiomics models (R-model). A radiomics score (Rad-score) was computed for each patient by taking a linear combination of selected features, weighted by their corresponding coefficients. The R-model was created as a binary classification model to distinguish between MYCN wild and MYCN gain and MNA. Additionally, Bio-omics features combined with radiomics features were used to construct the Multi-omics model (M-model).
Meanwhile, we used post hoc analysis to compare whether the constructed M-model can distinguish MYCN gain and MNA. The output score of M-model were used to analyze by receiver operating characteristic (ROC) analysis to obtain the best cutoff between the MYCN gain and MNA groups.
Statistical analysis
Statistical analyses were performed with Python (ver. 3.7.8, www.python.org) and R (ver. 4.0.3, www.r-project.org). The Python packages of “sklearn,” “numpy,” and “pandas” were used for LASSO binary logistic regression and ROC curve analysis. The R package “rms” was employed to create nomograms. A 2-sided P value of less than 0.05 was considered statistically significant. Cochran–Armitage tests for trend were used to evaluate associations between MYCN copy number categories and all clinical characteristics. These tests pitted the option of a linear trend with at least 1 tight inequality across groups versus the null hypothesis of no linear trend. Univariate analysis was used to compare differences in the clinical factors, the independent t-test or Mann-Whitney U test for quantitative data, and the chi-squared test for categorical variables. To mitigate the impact of data deviation or inadequate sample size, all models were constructed using logistic regression with 10-fold cross-validation, repeated 20 times. The DeLong test was utilized to evaluate variations in area under the curve (AUC) values among different models. Based on the cutoff value that corresponded to the greatest Youden index, accuracy (ACC), specificity (SPE), and sensitivity (SEN) were calculated. Each model’s prediction performance was assessed using calibration curves.
Results
Patient characteristics
The patient demographics are summarized in Table 1. Of the 104 patients (mean age: 33.5 months; range, 17.0–52.3 months), 63 (60.6%) had MYCN wild-type tumors, 28 (26.9%) had tumors with MYCN gain, and 13 (12.5%) had MNA. Significant changes were noted, including a rise in the proportion of individuals with adverse clinical characteristics in the higher MYCN copy number category. The percentage of patients with high LDH showed the biggest variations (19% for MYCN wild-type, 46% for MYCN gain, and 85% for MNA; P<0.001). Interestingly, INSS stage, COG risk group, NSE, LDH, VMA, 1p LOH, 11q aberration, INPC histologic categorization, and MKI (P<0.05) were some of the significant variations between all clinical parameters when comparing MYCN gain and MNA. An elevated MYCN copy number was more commonly linked to primary adrenal cancers (67% for MYCN wild, 75% for MYCN gain, and 77% for MNA). Conversely, the proportion of patients who had a primary thoracic tumor declined (23% for MYCN wild, 14% for MYCN gain, and 0% for MNA), but no statistical significance was found. PET quantitative parameters (SUVmax, TLG) can identify MYCN wild and MNA (P<0.05).
Table 1
Features | A: MYCN wild-type, n (%) | B: MYCN gain (signal 2–4×), n (%) | C: MYCN amp (signal >4×), n (%) | All patients | P value (A vs. B vs. C) | P value for non-amplified groups (A vs. B) | P value for non-wild-type groups (B vs. C) | P value for non-gain-type groups (B vs. C) |
---|---|---|---|---|---|---|---|---|
Male | 21 (33.3) | 14 (50.0) | 7 (53.8) | 42 (40.4) | >0.99 | >0.99 | >0.99 | 0.21 |
Female | 42 (66.7) | 14 (50.0) | 6 (46.2) | 62 (59.6) | ||||
Age ≥18 months at diagnosis | 50 (79.4) | 18 (64.3) | 12 (92.3) | 80 (76.9) | >0.99 | >0.99 | 0.13 | 0.44 |
Age <18 months at diagnosis | 13 (20.6) | 10 (35.7) | 1 (7.7) | 24 (23.1) | ||||
INSS stage 4 | 16 (25.397) | 19 (73.1) | 9 (69.2) | 65 (63.7) | 0.006 | >0.99 | 0.02 | 0.007 |
All other stages | 47 (74.603) | 7 (26.9) | 4 (30.8) | 37 (36.3) | ||||
High COG risk | 31 (49.2) | 17 (60.7) | 13 (100.0) | 61 (58.7) | 0.003 | >0.99 | 0.008 | 0.002 |
Low/intermediate COG risk | 32 (50.8) | 11 (39.3) | 0 (0.0) | 43 (41.3) | ||||
High NSE (≥16.3 ng/mL) | 62 (98.4) | 28 (100.0) | 13 (100.0) | 103 (99.0) | >0.99 | >0.99 | 0.04 | >0.99 |
Normal NSE (<16.3 ng/mL) | 1 (1.6) | 0 (0.0) | 0 (0.0) | 1 (1.0) | ||||
High ferritin (≥115 ng/mL) | 28 (44.4) | 12 (42.9) | 9 (69.2) | 49 (47.1) | >0.99 | >0.99 | 0.22 | 0.19 |
Normal ferritin (<115 ng/mL) | 35 (55.6) | 16 (57.1) | 4 (30.8) | 55 (52.9) | ||||
High LDH (<662.5 U/L) | 12 (19.0) | 13 (46.4) | 11 (84.6) | 36 (34.6) | 0.001 | 0.01 | 0.05 | 0.001 |
Normal LDH (≥662.5 U/L) | 51 (81) | 15 (53.6) | 2(15.4) | 68 (65.4) | ||||
High VMA (≥68.6 umo/L) | 43 (68.3) | 24 (85.7) | 3 (23.1) | 70 (67.3) | 0.001 | 0.14 | 0.001 | 0.01 |
Normal VMA (<68.6 umo/L) | 20 (31.7) | 4 (14.3) | 10 (76.9) | 34 (32.7) | ||||
High HVA (≥40 μmol/L) | 32 (50.8) | 19 (67.9) | 5 (38.5) | 56 (53.8) | >0.99 | 0.20 | 0.15 | 0.61 |
Normal HVA (<40 μmol/L) | 31 (49.2) | 9 (32.1) | 8 (61.5) | 48 (46.2) | ||||
LOH/aberration at 1p | 14 (22.2) | 12 (42.9) | 12 (92.3) | 38 (36.5) | 0.001 | 0.08 | 0.01 | 0.001 |
No 1p LOH/aberration | 49 (77.8) | 16 (57.1) | 1 (7.7) | 66 (63.5) | ||||
Aberration at 11q | 17 (26.9) | 20 (71.4) | 2 (15.4) | 41 (39.4) | 0.001 | <0.001 | 0.003 | 0.50 |
No 11q Aberration | 46 (73.1) | 8 (28.6) | 11 (84.6) | 63 (60.6) | ||||
Unfavorable histology | 28 (44.4) | 15 (53.6) | 12 (92.3) | 65 (62.5) | 0.001 | 0.23 | 0.001 | 0.004 |
Favorable histology | 35 (55.6) | 13 (46.4) | 1 (7.7) | 39 (37.5) | ||||
High MKI | 1 (1.9) | 2 (7.1) | 13 (100.0) | 16 (15.4) | 0.001 | 0.22 | 0.001 | 0.001 |
Low/intermediate MKI | 62 (98.4) | 26 (92.9) | 0 (0.0) | 88 (84.6) | ||||
INRG stage (M) | 37 (58.7) | 19 (67.9) | 9 (69.2) | 65 (62.5) | >0.99 | 0.55 | >0.99 | 0.69 |
INRG stage (L1, L2, MS) | 26 (41.3) | 9 (32.1) | 4 (30.8) | 39 (37.5) | ||||
Adrenal primary | 9 (14.3) | 4 (14.3) | 0 (0.0) | 13 (12.5) | >0.99 | >0.99 | 0.29 | 0.34 |
Other primary sites | 54 (85.7) | 24 (85.7) | 13 (100.0) | 91 (87.5) | ||||
Thoracic primary | 42 (66.7) | 21 (75.0) | 10 (76.9) | 73 (70.2) | >0.99 | 0.58 | >0.99 | 0.74 |
Other primary sites | 21 (33.3) | 7 (25.0) | 3 (23.1) | 31 (29.8) | ||||
Infiltrating across midline | 25 (39.7) | 14 (50.0) | 9 (69.2) | 48 (46.2) | >0.99 | 0.49 | 0.41 | 0.10 |
Not infiltrating across midline | 38 (60.3) | 14 (50.0) | 4 (30.8) | 56 (53.8) | ||||
Calcification | 44 (69.8) | 21 (75.0) | 7 (53.8) | 72 (69.2) | >0.99 | 0.80 | 0.28 | 0.33 |
No calcification | 19 (30.2) | 7 (25.0) | 6 (46.2) | 32 (30.8) | ||||
Necrosis | 43 (68.3) | 23 (82.1) | 11 (84.6) | 77 (74.0) | >0.99 | 0.26 | >0.99 | 0.33 |
No necrosis | 20 (31.7) | 5 (17.9) | 2 (15.4) | 27 (26.0) | ||||
SUVmax [median (IQR)] | 4.5 (3.3, 5.8) | 5.1 (3.9, 7.7) | 5.600 (4.900, 6.300) | 4.600 (3.8, 6.200) | 0.04 | 0.11 | 0.47 | 0.02 |
MTV [median (IQR)] (mL) | 94.6 (35.5, 134.9) | 160.8 (79.4, 261.7) | 132.1 (45.6, 412.5) | 116.8 (40.2, 208.5) | 0.05 | 0.02 | 0.80 | 0.22 |
TLG [median (IQR)] | 193.0 (71.2, 375.8) | 367.4 (191.7, 590.9) | 571.9 (150.1, 1428.9) | 253.5 (81.6, 486.2) | 0.009 | 0.01 | 0.45 | 0.03 |
PET/CT, positron emission tomography/computed tomography; amp, amplification; INSS, International Neuroblastoma Staging System; COG, Children’s Oncology Group; NSE, neuron-specific enolase; LDH, lactate dehydrogenase; VMA, vanillylmandelic acid; HVA, homovanillic acid; MKI, mitosis-karyorrhexis index; INRG, International Neuroblastoma Risk Group; SUVmax, maximum standard uptake values; IQR, interquartile range; MTV, metabolic tumor volume; TLG, total lesion glycolysis.
Bio-omics model assessment
The results of the univariate analysis and multivariate logistic regression analysis are presented in Tables 2 and 3, respectively. Univariate analysis showed that there were significant differences in COG, LDH, No1p, No11q, MKI, SUVmax, MTV, and TLG between MYCN wild and MYCN gain and MNA. After multivariate logistic regression analysis, LDH, MKI, and TLG (P<0.05) were independent predictors of MYCN gain and MNA. Then, a B-model was established based on the above independent variables. The AUC was 0.81 [95% confidence interval (CI): 0.72–0.90], ACC 0.80, SEN 0.68, and SPE 0.87 (Table 4 and Figure 2).
Table 2
Features | Wild, n (%) | Gain + amplification, n (%) | P value |
---|---|---|---|
Male | 21 (33.3) | 21 (51.2) | 0.11 |
Female | 42 (66.7) | 20 (48.8) | |
Age ≥18 months at diagnosis | 50 (79.4) | 30 (73.2) | 0.62 |
Age <18 months at diagnosis | 13 (20.6) | 11 (26.9) | |
INSS stage 4 | 16 (25.4) | 28 (68.3) | 0.21 |
All other stages | 47 (74.6) | 13 (31.7) | |
High COG risk | 31 (49.2) | 30 (73.2) | 0.03 |
Low/intermediate COG risk | 32 (50.8) | 11 (26.8) | |
High NSE (≥16.3 ng/mL) | 62 (98.4) | 41 (100.0) | >0.99 |
Normal NSE (<16.3 ng/mL) | 1 (1.6) | 0 (0.0) | |
High ferritin (≥115 ng/mL) | 28 (44.4) | 21 (51.2) | 0.63 |
Normal ferritin (<115 ng/mL) | 35 (55.6) | 20 (48.8) | |
High LDH (<662.5 U/L) | 12 (19.0) | 24 (58.6) | 0.001 |
Normal LDH (≥662.5 U/L) | 51 (81) | 17 (41.5) | |
High VMA (≥68.6 umo/L) | 43 (68.3) | 27 (65.9) | 0.97 |
Normal VMA (<68.6 umo/L) | 20 (31.7) | 14 (34.1) | |
High HVA (≥40 μmol/L) | 32 (50.8) | 24 (58.5) | 0.57 |
Normal HVA (<40 μmol/L) | 31 (49.2) | 17 (41.5) | |
LOH/aberration at 1p | 14 (22.2) | 24 (58.5) | 0.001 |
No 1p LOH/aberration | 49 (77.8) | 17 (41.5) | |
Aberration at 11q | 17 (26.984) | 22 (53.7) | 0.01 |
No 11q aberration | 46 (73.016) | 19 (46.3) | |
Unfavorable Histology | 28 (44.4) | 20 (48.8) | 0.82 |
Favorable histology | 35 (55.6) | 21 (51.2) | |
High MKI | 1 (1.9) | 15 (36.6) | 0.001 |
Low/intermediate MKI | 62 (98.4) | 26 (63.4) | |
INRG stage (M) | 38 (60.3) | 20 (48.8) | 0.34 |
INRG stage (L1, L2, MS) | 25 (39.7) | 21 (51.2) | |
Adrenal primary | 9 (14.3) | 4 (9.7) | 0.71 |
Other primary sites | 54 (85.7) | 37 (90.2) | |
Thoracic primary | 42 (66.7) | 31 (75.6) | 0.45 |
Other primary sites | 21 (33.3) | 10 (24.4) | |
Infiltrating across midline | 25 (39.683) | 23 (56.1) | 0.15 |
Not infiltrating across midline | 38 (60.317) | 18 (43.9) | |
Calcification | 44 (69.841) | 28 (68.3) | >0.99 |
No calcification | 19 (30.159) | 13 (31.7) | |
Necrosis | 43 (68.3) | 34 (82.9) | 0.15 |
No necrosis | 20 (31.7) | 7 (17.1) | |
SUVmax [median (IQR)] | 4.5 (3.3; 5.8) | 5.6 (4.2; 7.5) | 0.02 |
MTV [median (IQR) (mL) | 94.6 (35.5; 134.9) | 156.80 (62.2; 283.9) | 0.02 |
TLG [median (IQR)] | 193.0 (71.1; 375.8) | 395.20 (158.3; 771.2) | 0.002 |
Data are represented as number (%) or median (IQR). PET/CT, positron emission tomography/computed tomography; amp, amplification; INSS, International Neuroblastoma Staging System; COG, Children’s Oncology Group; NSE, neuron-specific enolase; LDH, lactate dehydrogenase; VMA, vanillylmandelic acid; HVA, homovanillic acid; MKI, mitosis-karyorrhexis index; INRG, International Neuroblastoma Risk Group; SUVmax, maximum standard uptake value; IQR, interquartile range; MTV, metabolic tumor volume; TLG, total lesion glycolysis.
Table 3
Features | Univariate analysis | Multivariate analysis | |||
---|---|---|---|---|---|
OR (95% CI) | P value | OR (95% CI) | P value | ||
TLG | 1.002 (1.000–1.003) | 0.002 | 1.000 (0.999–1.002) | 0.48 | |
LDH | 5.843 (2.452–14.698) | 0.001 | 1.76 (0.544–5.570) | 0.34 | |
MKI | 30.743 (5.733–770.400) | 0.001 | 17.017 (2.784–331.102) | 0.01 | |
Rad-score | NA | NA | 1.907 (1.138–3.550) | 0.03 |
OR, odds ratio; CI, confidence interval; TLG, total lesion glycolysis; LDH, lactate dehydrogenase; MKI, mitosis-karyorrhexis index.
Table 4
Task | Model | AUC (95% CI) | ACC (95% CI) | SEN (95% CI) | SPE (95% CI) | PPV (95% CI) | NPV (95% CI) |
---|---|---|---|---|---|---|---|
Wild vs. gain + amp | Radiomics | 0.787 (0.694–0.880) |
0.731 (0.635–0.813) |
0.756 (0.560–0.879) |
0.714 (0.365–0.842) |
0.633 (0.561–0.667) |
0.818 (0.697–0.841) |
Wild vs. gain + amp | Bio-omics | 0.809 (0.716–0.902) |
0.798 (0.708–0.870) |
0.683 (0.463–0.829) |
0.873 (0.602–0.984) |
0.778 (0.704–0.810) |
0.809 (0.745–0.827) |
Wild vs. gain + amp | Multi-omics | 0.830 (0.743–0.917) |
0.808 (0.719–0.878) |
0.756 (0.512–0.854) |
0.841 (0.492–0.952) |
0.756 (0.677–0.778) |
0.841 (0.756–0.857) |
Gain vs. amp | Multi-omics | 0.953 (0.890–1.000) |
0.902 (0.769–0.973) |
1.000 (0.538–1.000) |
0.857 (0.749–1.000) |
0.765 (0.636–0.765) |
1.000 (1.000–1.000) |
AUC, area under the curve; CI, confidence interval; ACC, accuracy; SEN, sensitivity; SPE, specificity; PPV, positive predictive value; NPV, negative predictive value; amp, amplification.
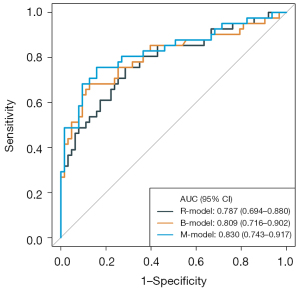
Radiomics model assessment
We selected 5 optima features (3 PET texture features and 2 CT texture features) and the coefficients of the corresponding features were calculated (Figure 3). Finally, the Rad-score was calculated to build the R-model. The AUC was 0.79 (95% CI: 0.69–0.88), ACC 0.73, SEN 0.76, and SPE 0.71 (Table 4 and Figure 2).
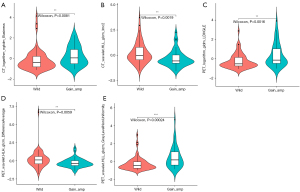
M-model: LDH, MKI, TLG, and 5 optima radiomics features were included in the model. The AUC of the M-model was 0.83 (95% CI: 0.74–0.92), ACC 0.81, SEN 0.76, and SPE 0.84 (Table 4 and Figure 2).
MYCN gain and MNA variance assessment
The results of post hoc analysis showed that the M-model can identify MYCN gain and MNA (Figure 4); the AUC was 0.95 (95% CI: 0.89–1), ACC 0.90, SEN 1.00, and SPE 0.86 (Table 4 and Figure 2).
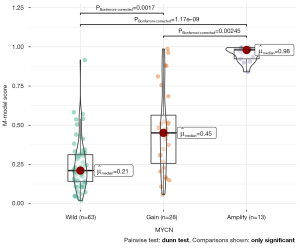
Model comparisons
The M-model showed higher classification accuracy than the B-model in identifying MYCN wild and MYCN gain and MNA (AUC 0.83, 95% CI: 0.74–0.92 vs. AUC 0.81, 95% CI: 0.72–0.90). The predictive performance of the B-model was slightly higher than that of the R-model (AUC 0.81, 95% CI: 0.72–0.90 vs. AUC 0.79, 95% CI: 0.69–0.89). To illustrate the effectiveness of the R-model, the quantitative values of the model for each NB patient using the classification of MYCN wild, MYCN gain, and MNA are presented in Figure S1.
With a threshold probability of 10–90%, decision curve analysis (DCA) revealed that the M-model was more clinically useful than the B-model and R-model (Figure 5A). The calibration curve showed that the 3 models could predict the copy number of MYCN category (Figure 5B). Post hoc analysis showed the great potential of the M-model in identifying MYCN gain and MNA (AUC =0.95), which also might support the different clinical behavior between MYCN gain and MNA in NB. To provide a visualization of the MYCN copy number categories, a nomogram figure was plotted for the M-model, as displayed in Figure 6A. Cross-validation confirmed the reliability and stability of the M-model (Figure 6B).
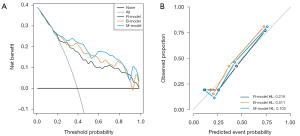
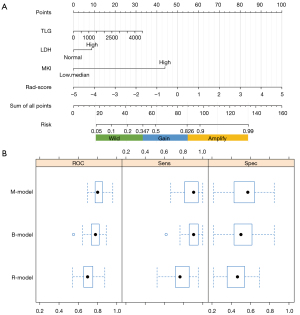
Discussion
Our preliminary analysis showed that the M-model could predict preoperative MYCN copy number category. Therefore, we believe that the M-model based on bio-omics and radiomics features was shown to be an effective tool to distinguish MYCN copy number category in patients with NB.
MNA was associated with unfavorable histology at diagnosis, higher MKI status, more aggressive clinical features, higher tumor stage, rapid early response to cytotoxic chemotherapy, higher progression rate during induction treatment, rapid regeneration of tumor chemoresistance, and other important clinical and biological features (26). A strong association has also been revealed between MNA and genomic features, such as diploidy (27) and loss of heterozygosity at 1p (28). The simultaneous occurrence of 11q aberrations was very frequent in samples with MYCN-gain compared to amplification, but rarely correlated with 11q aberrations and MNA (29). Thus, a study suggested that MYCN gain does not appear to be a precursor to MNA, but rather an independent late event in the complex pattern of aberrations in advanced NB (30). In our analysis of patients, 11q aberrations occurred in 71.4% of patients with MYCN gain tumors, compared with only 26.9% and 15.4% of patients with MYCN wild-type and MNA tumors, respectively. These results were consistent with those of previous studies. In 1985, Seeger et al. (31) reported that for patients with 1, 3–10, or more than 10 copies of MCYN in the tumor, progression-free survival at 18 months was 70%, 30%, and 5%, respectively. Campbell et al. (4) reported that MYCN gain had the greatest impact in subgroups of patients with non-stage 4 diseases and non-high-risk diseases. Therefore, it was very important to identify MYCN copy number categories preoperatively for the stratified management of NB patients.
In the present study, the M-model established by multivariate logistic regression analysis showed that LDH, MKI, TLG, and Rad-score were independent predictors of MYCN gain and MNA. The levels of LDH, MKI, TLG, and Rad-score were higher in MYCN gain and MNA patients. Based on the above parameters, the B-model, R-model, and M-model were established, and the present study demonstrated that the AUCs of the 3 methods for identifying MYCN gain and MNA were all above 0.75. The AUC, ACC, SEN, and negative predictive value (NPV) of the M-model were better than those of the B-model. Although the difference was not statistically significant (P=0.48), it did indicate that the R-model had potential value. Interestingly, post hoc analysis showed that the M-model had higher diagnostic value in differentiating MYCN gain and MNA, which meant that the M-model could distinguish patients with MYCN gain from those with MNA.
Although previous studies have reported the potential role of radiogenomics in predicting molecular biomarkers in NB, these radiomics studies on the prediction of gene mutations were based on single CT images only (32,33). Compared with CT, 18F-FDG PET/CT can provide both anatomical and metabolic information after a single scan. Therefore, we extracted information from PET and CT and built a novel radiomic model for predicting the MYCN copy number category. This radiomics model included 3 PET radiomics features and 2 CT radiomics features, suggesting the importance of PET radiomics features in the prediction model. A reasonable explanation is that PET images could monitor the tumor microenvironment, therefore more signal differences could be observed. In this study, we also selected clinical and biological features, PET/CT radiographic features, PET quantitative parameters, and PET radiomics features for a more comprehensive identification of MYCN copy number category.
Our study has several limitations. Firstly, this was a retrospective study with a small sample size and the results may be biased. Future prospective studies with large sample sizes using standardized imaging techniques are needed. Secondly, the feature selection in this study was performed on the whole dataset and this could have introduced data leakage. Thirdly, this study focused only on the prediction of MYCN copy number categories in NB. The analysis of other genetic abnormalities of prognostic value, including ALK mutations or amplifications and NKAP mutations, will provide a further comprehensive understanding of the image-genetic correlation of NB in the future.
Conclusions
This study demonstrated that the M-model based on bio-omics and radiomics features was an effective tool to distinguish MYCN copy number category in pediatric patients with NB.
Acknowledgments
Funding: This study was supported by grants from
Footnote
Reporting Checklist: The authors have completed the STARD reporting checklist. Available at https://qims.amegroups.com/article/view/10.21037/qims-23-494/rc
Conflicts of Interest: All authors have completed the ICMJE uniform disclosure form (available at https://qims.amegroups.com/article/view/10.21037/qims-23-494/coif). All authors report that this study was supported by grants from the National Natural Science Foundation of China (No. 82272034) and Beijing Municipal Natural Science Foundation (No. 7232031). J.L.R. is an employee of GE HealthCare. The authors have no other conflicts of interest to declare.
Ethical Statement: The authors are accountable for all aspects of the work in ensuring that questions related to the accuracy or integrity of any part of the work are appropriately investigated and resolved. This retrospective study was conducted in accordance with the Declaration of Helsinki (as revised in 2013). The study was approved by the Institutional Review Board of Beijing Friendship Hospital Affiliated to Capital Medical University (No. 2020-P2-091-02) and the requirement for written informed consent was waived due to the retrospective nature of the study.
Open Access Statement: This is an Open Access article distributed in accordance with the Creative Commons Attribution-NonCommercial-NoDerivs 4.0 International License (CC BY-NC-ND 4.0), which permits the non-commercial replication and distribution of the article with the strict proviso that no changes or edits are made and the original work is properly cited (including links to both the formal publication through the relevant DOI and the license). See: https://creativecommons.org/licenses/by-nc-nd/4.0/.
References
- London WB, Castleberry RP, Matthay KK, Look AT, Seeger RC, Shimada H, Thorner P, Brodeur G, Maris JM, Reynolds CP, Cohn SL. Evidence for an age cutoff greater than 365 days for neuroblastoma risk group stratification in the Children's Oncology Group. J Clin Oncol 2005;23:6459-65. [Crossref] [PubMed]
- Irwin MS, Park JR. Neuroblastoma: paradigm for precision medicine. Pediatr Clin North Am 2015;62:225-56. [Crossref] [PubMed]
- Raitio A, Rice MJ, Mullassery D, Losty PD. Stage 4S Neuroblastoma: What Are the Outcomes? A Systematic Review of Published Studies. Eur J Pediatr Surg 2021;31:385-9. [Crossref] [PubMed]
- Campbell K, Gastier-Foster JM, Mann M, Naranjo AH, Van Ryn C, Bagatell R, Matthay KK, London WB, Irwin MS, Shimada H, Granger MM, Hogarty MD, Park JR, DuBois SG. Association of MYCN copy number with clinical features, tumor biology, and outcomes in neuroblastoma: A report from the Children's Oncology Group. Cancer 2017;123:4224-35. [Crossref] [PubMed]
- Marrano P, Irwin MS, Thorner PS. Heterogeneity of MYCN amplification in neuroblastoma at diagnosis, treatment, relapse, and metastasis. Genes Chromosomes Cancer 2017;56:28-41. [Crossref] [PubMed]
- Georgantzi K, Sköldenberg E, Janson ET, Jakobson Å, Christofferson R. Diagnostic ultrasound-guided cutting needle biopsies in neuroblastoma: A safe and efficient procedure. J Pediatr Surg 2019;54:1253-6. [Crossref] [PubMed]
- Overman RE, Kartal TT, Cunningham AJ, Fialkowski EA, Naik-Mathuria BJ, Vasudevan SA, et al. Optimization of percutaneous biopsy for diagnosis and pretreatment risk assessment of neuroblastoma. Pediatr Blood Cancer 2020;67:e28153. [Crossref] [PubMed]
- Liu J, Li C, Yang X, Lu X, Zhang M, Qian L, Wang W, Kan Y, Yang J. The Diagnostic Value of (18)F-FDG PET/CT Bone Marrow Uptake Pattern in Detecting Bone Marrow Involvement in Pediatric Neuroblastoma Patients. Contrast Media Mol Imaging 2022;2022:7556315. [Crossref] [PubMed]
- Sung AJ, Weiss BD, Sharp SE, Zhang B, Trout AT. Prognostic significance of pretreatment 18F-FDG positron emission tomography/computed tomography in pediatric neuroblastoma. Pediatr Radiol 2021;51:1400-5. [Crossref] [PubMed]
- Kushner BH, Yeung HW, Larson SM, Kramer K, Cheung NK. Extending positron emission tomography scan utility to high-risk neuroblastoma: fluorine-18 fluorodeoxyglucose positron emission tomography as sole imaging modality in follow-up of patients. J Clin Oncol 2001;19:3397-405. [Crossref] [PubMed]
- Pinker K, Shitano F, Sala E, Do RK, Young RJ, Wibmer AG, Hricak H, Sutton EJ, Morris EA. Background, current role, and potential applications of radiogenomics. J Magn Reson Imaging 2018;47:604-20. [Crossref] [PubMed]
- Liu CJ, Lu MY, Liu YL, Ko CL, Ko KY, Tzen KY, Chang HH, Yang YL, Jou ST, Hsu WM, Yen RF. Risk Stratification of Pediatric Patients With Neuroblastoma Using Volumetric Parameters of 18F-FDG and 18F-DOPA PET/CT. Clin Nucl Med 2017;42:e142-8. [Crossref] [PubMed]
- Chang C, Zhou S, Yu H, Zhao W, Ge Y, Duan S, Wang R, Qian X, Lei B, Wang L, Liu L, Ruan M, Yan H, Sun X, Xie W. A clinically practical radiomics-clinical combined model based on PET/CT data and nomogram predicts EGFR mutation in lung adenocarcinoma. Eur Radiol 2021;31:6259-68. [Crossref] [PubMed]
- Umutlu L, Kirchner J, Bruckmann NM, Morawitz J, Antoch G, Ingenwerth M, Bittner AK, Hoffmann O, Haubold J, Grueneisen J, Quick HH, Rischpler C, Herrmann K, Gibbs P, Pinker-Domenig K. Multiparametric Integrated (18)F-FDG PET/MRI-Based Radiomics for Breast Cancer Phenotyping and Tumor Decoding. Cancers (Basel) 2021;13:2928. [Crossref] [PubMed]
- Qian L, Yang S, Zhang S, Qin H, Wang W, Kan Y, Liu L, Li J, Zhang H, Yang J. Prediction of MYCN Amplification, 1p and 11q Aberrations in Pediatric Neuroblastoma via Pre-therapy 18F-FDG PET/CT Radiomics. Front Med (Lausanne) 2022;9:840777. [Crossref] [PubMed]
- Ambros PF, Ambros IMSIOP Europe Neuroblastoma Pathology, Biology, and Bone Marrow Group. Pathology and biology guidelines for resectable and unresectable neuroblastic tumors and bone marrow examination guidelines. Med Pediatr Oncol 2001;37:492-504. [Crossref] [PubMed]
- Stauss J, Franzius C, Pfluger T, Juergens KU, Biassoni L, Begent J, Kluge R, Amthauer H, Voelker T, Højgaard L, Barrington S, Hain S, Lynch T, Hahn KEuropean Association of Nuclear Medicine. Guidelines for 18F-FDG PET and PET-CT imaging in paediatric oncology. Eur J Nucl Med Mol Imaging 2008;35:1581-8. [Crossref] [PubMed]
- Brodeur GM, Pritchard J, Berthold F, Carlsen NL, Castel V, Castelberry RP, De Bernardi B, Evans AE, Favrot M, Hedborg F. Revisions of the international criteria for neuroblastoma diagnosis, staging, and response to treatment. J Clin Oncol 1993;11:1466-77. [Crossref] [PubMed]
- Park JR, Eggert A, Caron H. Neuroblastoma: biology, prognosis, and treatment. Hematol Oncol Clin North Am 2010;24:65-86. [Crossref] [PubMed]
- Attiyeh EF, London WB, Mossé YP, Wang Q, Winter C, Khazi D, McGrady PW, Seeger RC, Look AT, Shimada H, Brodeur GM, Cohn SL, Matthay KK, Maris JMChildren's Oncology Group. Chromosome 1p and 11q deletions and outcome in neuroblastoma. N Engl J Med 2005;353:2243-53. [Crossref] [PubMed]
- Shimada H, Ambros IM, Dehner LP, Hata J, Joshi VV, Roald B, Stram DO, Gerbing RB, Lukens JN, Matthay KK, Castleberry RP. The International Neuroblastoma Pathology Classification (the Shimada system). Cancer 1999;86:364-72.
- Bhardwaj N, Rohilla M, Trehan A, Bansal D, Kakkar N, Srinivasan R. Mitosis-Karyorrhexis Index evaluation by digital image visual analysis for application of International Neuroblastoma Pathology Classification in FNA biopsy. Cancer Cytopathol 2022;130:128-35. [Crossref] [PubMed]
- Monclair T, Brodeur GM, Ambros PF, Brisse HJ, Cecchetto G, Holmes K, Kaneko M, London WB, Matthay KK, Nuchtern JG, von Schweinitz D, Simon T, Cohn SL, Pearson AD. INRG Task Force. The International Neuroblastoma Risk Group (INRG) staging system: an INRG Task Force report. J Clin Oncol 2009;27:298-303. [Crossref] [PubMed]
- Ha S, Choi H, Paeng JC, Cheon GJ. Radiomics in Oncological PET/CT: a Methodological Overview. Nucl Med Mol Imaging 2019;53:14-29. [Crossref] [PubMed]
- Nioche C, Orlhac F, Boughdad S, Reuzé S, Goya-Outi J, Robert C, Pellot-Barakat C, Soussan M, Frouin F, Buvat I. LIFEx: A Freeware for Radiomic Feature Calculation in Multimodality Imaging to Accelerate Advances in the Characterization of Tumor Heterogeneity. Cancer Res 2018;78:4786-9. [Crossref] [PubMed]
- Lee JW, Son MH, Cho HW, Ma YE, Yoo KH, Sung KW, Koo HH. Clinical significance of MYCN amplification in patients with high-risk neuroblastoma. Pediatr Blood Cancer 2018;65:e27257. [Crossref] [PubMed]
- Oppedal BR, Storm-Mathisen I, Lie SO, Brandtzaeg P. Prognostic factors in neuroblastoma. Clinical, histopathologic, and immunohistochemical features and DNA ploidy in relation to prognosis. Cancer 1988;62:772-80. [Crossref] [PubMed]
- Maris JM, Guo C, Blake D, White PS, Hogarty MD, Thompson PM, Rajalingam V, Gerbing R, Stram DO, Matthay KK, Seeger RC, Brodeur GM. Comprehensive analysis of chromosome 1p deletions in neuroblastoma. Med Pediatr Oncol 2001;36:32-6. [Crossref] [PubMed]
- Spitz R, Hero B, Skowron M, Ernestus K, Berthold F. MYCN-status in neuroblastoma: characteristics of tumours showing amplification, gain, and non-amplification. Eur J Cancer 2004;40:2753-9. [Crossref] [PubMed]
- Jeison M, Ash S, Halevy-Berko G, Mardoukh J, Luria D, Avigad S, Feinberg-Gorenshtein G, Goshen Y, Hertzel G, Kapelushnik J, Ben Barak A, Attias D, Steinberg R, Stein J, Stark B, Yaniv I. 2p24 Gain region harboring MYCN gene compared with MYCN amplified and nonamplified neuroblastoma: biological and clinical characteristics. Am J Pathol 2010;176:2616-25. [Crossref] [PubMed]
- Seeger RC, Brodeur GM, Sather H, Dalton A, Siegel SE, Wong KY, Hammond D. Association of multiple copies of the N-myc oncogene with rapid progression of neuroblastomas. N Engl J Med 1985;313:1111-6. [Crossref] [PubMed]
- Chen X, Wang H, Huang K, Liu H, Ding H, Zhang L, Zhang T, Yu W, He L. CT-Based Radiomics Signature With Machine Learning Predicts MYCN Amplification in Pediatric Abdominal Neuroblastoma. Front Oncol 2021;11:687884. [Crossref] [PubMed]
- Wu H, Wu C, Zheng H, Wang L, Guan W, Duan S, Wang D. Radiogenomics of neuroblastoma in pediatric patients: CT-based radiomics signature in predicting MYCN amplification. Eur Radiol 2021;31:3080-9.