Compare deep learning model and conventional logistic regression model for the identification of unstable saccular intracranial aneurysms in computed tomography angiography
Introduction
As imaging diagnostic technology has advanced, more intracranial aneurysms (IAs) have been diagnosed (1). Although ultrahigh field magnetic resonance imaging is a powerful diagnostic tool for differentiating true small IAs from normal anatomical variants (2), computed tomography angiography (CTA) remains the first-line imaging modality due to its more widespread availability. IAs can rupture, resulting in subarachnoid hemorrhage (SAH) and significant morbidity and mortality (3). Although endovascular therapy (EVT) or neurosurgical therapy (NST) can prevent the rupture of unruptured IAs, postoperative complications (e.g., cerebral infarction and intracerebral hemorrhage) can lead to poor outcomes (4,5). At present, the management of unruptured IAs is still controversial. The progression of IAs is correlated with hypertension, age, earlier SAH, size, location, and shape (PHAESE score) (6-8) and results in a higher risk of rupture, in which case the IA is defined as an unstable IA (9). Thus, it is crucial to distinguish unstable from stable IAs as early as possible to derive optimal clinical decision-making for further treatment or follow-up.
Many previous studies used conventional logistic regression (LR) and the PHAESE score system to discriminate IA stability based on patient clinical and IA morphological features. However, their discriminatory abilities have not been satisfactory. For example, Foreman et al. and Pagiola et al. showed that most SAH patients had low PHAESE scores (≤5) (10,11), which indicated that the PHAESE score is not useful in daily clinical practice. With the continuous development of artificial intelligence (AI), some machine learning models have performed better than conventional LR and the PHAESE score method (12-14). Even so, a recent multicenter study indicated that machine learning models did not outperform conventional LR in predicting the future rupture status of unruptured IAs (15).
Deep learning models (DLMs) have shown significant potential for lesion detection and analysis of medical imaging in different specialties (16). Convolutional neural networks (CNNs) are a subset of DLMs, and they are particularly well suited for image feature selection, classification and segmentation (17). CNNs can interpret medical images objectively. Several previous studies used CNNs to detect and segment IAs (18-20), which suggested that DLMs could help clinicians detect IAs earlier and more sensitively. However, few studies have focused on the identification of unstable IAs based on DLMs from CTA images. Hence, the aim of this study is to construct a CNN-based DLM to distinguish unstable IAs from CTA images and compare discriminatory ability with a conventional logistic regression model (LRM) and to help clinicians make more appropriate decisions for patients with IAs. We present this article in accordance with the TRIPOD reporting checklist (available at https://qims.amegroups.com/article/view/10.21037/qims-23-1732/rc).
Methods
Patients
The study was conducted in accordance with the Declaration of Helsinki (as revised in 2013). The local ethics committees approved this retrospective study (Banan Hospital, approval No. 2021015; Xinqiao Hospital, approval No. 202248201). Individual consent for this retrospective analysis was waived. The clinical and imaging data of patients with IAs at our participating centers (Xinqiao Hospital and Banan Hospital) from August 2011 to May 2021 were retrospectively analyzed. The inclusion criteria for this study were (I) age ≥18 years; (II) diagnosis of saccular IAs; and (III) complete clinical and imaging data. The exclusion criteria were (I) diagnosis of fusiform, dissection, traumatic, or infectious IAs; (II) IA complicated with other vascular diseases (such as vascular malformations and moyamoya disease); (III) lack of complete clinical or imaging data; and (IV) surgical or interventional procedures performed before CTA examination.
All IAs that met the above inclusion criteria were ultimately divided into two groups: stable IAs and unstable IAs. Following previous studies (6,7,21-23), the IAs were diagnosed as unstable IAs if they met the following criteria: (I) history of SAH due to ruptured but untreated, (II) rupture or growth (the increase in any size to ≥1 mm) or shape change (e.g., formation of blebs and lobes) during follow-up, and (III) neurological symptoms associated with the IA (e.g., sudden headache, blepharoptosis). Of note, ruptured IAs were collected and defined as the unstable group because such IAs are more prone to rebleed. When a patient had multiple IAs, the ruptured IA was confirmed based on the nonenhanced computed tomography (CT), angiographic, or operative findings. For patients with multiple unruptured IAs and neurological symptoms, the largest one was identified as the responsible IA. The remaining IAs, which were detected incidentally, were followed for ≥3 months via CTA or magnetic resonance angiography (MRA) and diagnosed as stable IAs. The patient inclusion flow chart is displayed in Figure 1. Notably, 93 IAs presented with changes in size, shape, or clinical symptoms simultaneously. Patient clinical characteristics, such as age, sex, alcohol consumption, smoking, hypertension, diabetes mellitus, cerebrovascular sclerosis, heart disease, history of aneurysmal SAH and multiplicity, were collected. Finally, a total of 1,237 IAs (681 unstable IAs and 556 stable IAs) from 1,049 patients (Xinqiao Hospital) were selected for this study (Tables 1,2).
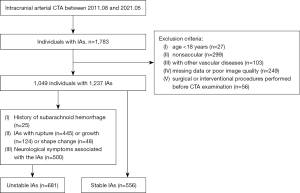
Table 1
Patients clinical information | Unstable (n=647) | Stable (n=402) | P |
---|---|---|---|
Female (%) | 411 (63.5) | 236 (58.7) | 0.133 |
Age (years, mean ± SD) | 57.15±12.60 | 60.19±12.35 | <0.001 |
Hypertension (%) | 269 (41.6) | 185 (46.0) | 0.159 |
Heart disease (%) | 36 (5.6) | 54 (13.4) | <0.001 |
Diabetes mellitus (%) | 30 (4.6) | 43 (10.7) | <0.001 |
Cerebrovascular sclerosis (%) | 61 (9.4) | 96 (23.9) | <0.001 |
Alcohol consumption (%) | 136 (21.0) | 81 (20.1) | 0.754 |
Smoking (%) | 165 (25.5) | 103 (25.6) | >0.99 |
SAH history (%) | 22 (3.4) | 13 (3.2) | >0.99 |
SD, standard deviation; SAH, subarachnoid hemorrhage.
Table 2
Aneurysms parameters | Unstable (n=681) | Stable (n=556) | P |
---|---|---|---|
Location (%) | |||
ACoA | 178 (26.1) | 43 (7.7) | <0.001 |
ACA | 37 (5.4) | 19 (3.4) | 0.1 |
MCA | 109 (16.0) | 99 (17.8) | 0.402 |
PCoA | 241 (35.4) | 76 (13.7) | <0.001 |
ICA | 80 (11.7) | 309 (55.6) | <0.001 |
PCA | 36 (5.3) | 10 (1.8) | 0.001 |
Multiple aneurysms (%) | 154 (22.6) | 193 (34.7) | <0.001 |
Bifurcation (%) | 444 (65.2) | 183 (32.9) | <0.001 |
Irregular shape (%) | 450 (66.1) | 32 (5.8) | <0.001 |
Daughter sac (%) | 303 (44.5) | 18 (3.2) | <0.001 |
Neck width (mm) | 5.03±2.23 | 4.07±1.13 | <0.001 |
Height (mm) | 6.56±3.62 | 3.23±1.14 | <0.001 |
Depth (mm) | 7.16±3.82 | 3.42±1.24 | <0.001 |
Width (mm) | 6.49±4.05 | 3.64±1.26 | <0.001 |
Maximum size (mm) | 8.45±4.24 | 4.47±1.44 | <0.001 |
Parent artery diameter (mm) | 3.34±0.86 | 4.00±0.92 | <0.001 |
Mean artery diameter (mm) | 3.03±0.81 | 3.72±0.92 | <0.001 |
AR | 1.48±0.62 | 0.85±0.24 | <0.001 |
DW | 1.19±0.38 | 0.96±0.22 | <0.001 |
BF | 1.30±0.52 | 0.89±0.18 | <0.001 |
SR | 2.46±1.35 | 0.95±0.37 | <0.001 |
FA | 121.00±25.28 | 102.37±28.46 | <0.001 |
Data are represented as mean ± standard deviation. ACoA, anterior communicating artery; ACA, anterior cerebral artery; MCA, middle cerebral artery; PCoA, posterior communicating artery; ICA, internal carotid artery; PCA, posterior circulation artery; AR, aspect ratio; DW, depth-to-width ratio; BF, bottleneck factor; SR, size ratio; FA, flow angle.
An independent data set from another Hospital (Banan Hospital) was used for external validation. This set included 197 patients and 229 IAs, which contained 106 unstable IAs and 123 stable IAs (Tables S1,S2).
Acquisition of aneurysm parameters
All CTA images were obtained with a 64-channel multidetector CT scanner (LightSpeed VCT 64 or Revolution 64, GE Medical Systems, Milwaukee, WI, USA). Both the slice thickness and reconstruction interval were 0.625 mm. The acquired images were transferred to the GE Advantage workstation (Advantage Windows 4.5), where 3D volume renderings (VRs) and maximum-intensity projections (MIPs) were generated. According to published definitions, morphological parameters were measured directly from 3D CTA images (6,22). All images were reviewed independently by two neuroradiologists with 10 and 20 years of experience. Continuous data were calculated as the means; to conduct subsequent statistical analysis, any differences in categorical data between the two readers were re-evaluated by a third reader (with 25 years of experience in neuroradiology).
We obtained 17 parameters from the CTA image of each IA: multiplicity; the location of the IA [internal carotid artery (ICA), middle cerebral artery (MCA), anterior cerebral artery (ACA), anterior communicating artery (ACoA), posterior communicating artery (PCoA), and posterior circulation artery (PCA)]; the origin of the IA (sidewall or bifurcation type); the shape of the IA (simple or irregular: an IA with a lobular or daughter sac was defined as having an irregular shape); the size of the IA (neck width, depth, width, height, maximum size); four secondary geometric morphology indices: aspect ratio (AR, depth/neck width), size ratio (SR, depth/parent artery diameter), depth-to-width ratio (DW, depth/width) and bottleneck factor (BF, width/neck width); and parameters related to the parent artery, including the parent artery diameter, mean artery diameter and the flow angle (FA). These parameters have been described clearly in previous studies (6,12,22).
Image preprocessing and splitting for deep learning
Each IA was defined as a region of interest (ROI), and two neuroradiologists who were aware of the patient’s actual diagnosis independently and manually annotated the aneurysm contours layer-by-layer on CTA images using the Dr. Wise Multimodal Research Platform (https://keyan.deepwise.com). If these two annotations differed, a third radiologist with 25 years of working experience joined the discussion to determine the final annotation (mask).
To accurately crop the ROI image, we performed the following preprocessing operations. First, we adjusted the delineated CT image window width and level to (400, 1,000). Then, we positioned the ROI according to the mask (aneurysm outline) and selected the lesions in the middle layer.
After image segmentation, the final dataset included 1,237 images, which were randomly divided into training [n=795 (64%), 352 stable and 443 unstable IAs], internal validation [n=204 (16%), 93 stable and 111 unstable IAs], and testing sets [n=238 (19%), 111 stable and 127 unstable IAs]. There was no duplication of data among between the three sets.
Deep learning algorithm
The modeling process was divided into image preprocessing, feature extraction and classification. For image preprocessing, we first resized all images to a fixed scale of 112×112 (this specific size could contain most IA sizes: for IAs with maximum slices larger than this size, the IA image was scaled to match the image size). Considering the computational constraints of the model, the maximum slices of IA images were used for two-dimensional (2D) modeling. Then, to increase the amount of data and improve the utilization of the training data set, we applied random horizontal flip, random vertical flip and random rotation by 30 degrees. For model training, we chose the classic CNN model, a residual pretrained network (ResNet-34). In the process of training the model, the parameters were updated. The output size was changed to 2 to match the number of classes.
When training, we set the batch size to 128 and the initial learning rate to 0.001. The number of epochs for model iteration was 500. The CrossEntropyLoss function was used to calculate the loss between the prediction result of the model and the real class. The Adam optimization algorithm was used to iteratively update the neural network weights. The initial values of the weights were randomly selected from a uniform distribution, and the bias was initialized to 0. The model with the highest accuracy values within 500 training epochs was chosen as the final model to output classification predictions.
Statistical analysis
Clinical information and morphological parameters
All statistical analyses of patient clinical information and IA morphological parameters were performed using SPSS software (version 25.0, Chicago, IL, USA). Continuous variables were reported as the mean ± standard deviation. Categorical variables are presented as numbers (%). One-way analysis of variance or the Kruskal-Wallis H test was used to compare quantitative variables, and the Chi-square test was used to compare qualitative variables between the training, internal validation and testing sets. Multiple comparison correction was performed by Bonferroni correction. When comparing the stable and unstable groups, the two-tailed t-test or the Mann-Whitney U test was used for quantitative variables, and the Chi-square test was used for qualitative variables. P<0.05 indicated statistical significance.
Model effect evaluation
For LRM, the clinical and IA variables with P<0.05 were further selected for multiple LR analysis. The forward conditional stepwise method was used to calculate the independent risk factors, and the assignment score of these independent risk factors depended on the β coefficient. For DLM, statistical analysis was performed using “SciPy.stats”, an open-source package of Python (24). To evaluate the discriminating ability of the model, we computed different indicators: accuracy, sensitivity, specificity and area under the curve (AUC). We drew the calibration curve to observe whether the predicted probability of the classification model was close to the true probability and used the clinical decision curve to measure the clinical practical value.
Results
Characteristics of patients and IAs
The clinical characteristics of the subjects and the morphological parameters of the IAs in the stable and unstable groups are summarized in Tables 1,2. The groups differed significantly in age, with the unstable group being younger (P<0.001). Heart disease, diabetes mellitus and cerebrovascular sclerosis were more common in the stable group (P<0.001).
Most morphological parameters were correlated with IA stability. The location of the IAs was associated with their stability: unstable IAs were more often located in the ACoA, PCoA and PCAs (P<0.001, P<0.001, and P=0.001, respectively), and stable IAs were more often located in the ICA (P<0.001). Compared with the unstable group, multiple IAs were more common in the stable group (22.6% vs. 34.7%). IA located at the bifurcation, with an irregular shape and daughter sac, was more common in the unstable group than in the stable group (P<0.001). Except for parent artery diameters and mean artery diameters, other manual parameters in the unstable group were larger than those in the stable group (P<0.001).
For the external validation set, cerebrovascular sclerosis was mildly more common in the stable group (P=0.049); unstable IAs were more often located in the ACoA, bifurcation, with an irregular shape and daughter sac than stable IAs. Similarly, except for parent artery diameters and mean artery diameters, other manual parameters in the unstable group were larger than those in the stable group (P<0.001).
Diagnostic performance of the LRM
Based on forward conditional stepwise LR analysis, the model showed that patients with heart disease, cerebral atherosclerosis, multiple IAs, IAs at the ICA and a large parent artery diameter tended to be associated with stable IA. Whereas IAs located at the ACoA, or PCoA; located at the bifurcation; those with an irregular shape; with a large maximum size and a high AR tended to be unstable IAs (Table 3). The cutoff values of the parent artery diameter, maximum size and AR were 3.53, 6.15, and 1.13 mm, respectively, which were calculated by the receiver operating characteristic (ROC) curve with the maximum Youden’s index. According to the β coefficient and presence or absence of these factors, a predictive scoring model was established as follows: total predictive score = heart disease (−0.7/0) + cerebral atherosclerosis (−1.4/0) + multiple IAs (−0.8/0) + IAs at the ICA (−1.1/0), ACoA (1.1/0), or PCoA score (1.1/0) + located at the bifurcation (1.2/0) + irregular shape (1.8/0) + parent artery diameter (>3.53 mm, −0.9/0) + maximum size (>6.15 mm, 0.7/0) + AR (>1.13, 2.6/0). The total predictive score ranged ranging from −4.9 to 8.5 points, the cutoff value of this score was 1.75 on the basis of maximum Youden’s index, the AUC was 0.945, and the sensitivity, specificity and diagnostic accuracy for the detection of unstable IAs were 0.709, 0.885, and 0.867, respectively (Figure 2 and Table 4).
Table 3
Variable | Univariate analysis | Multivariate analysis | |||||
---|---|---|---|---|---|---|---|
β | OR (95% CI) | P | β | OR (95% CI) | P | ||
Heart disease | −0.835 | 0.434 (0.285–0.661) | <0.001 | −0.745 | 0.475 (0.237–0.953) | 0.036 | |
Cerebrovascular sclerosis | −0.986 | 0.373 (0.269–0.517) | <0.001 | −1.402 | 0.246 (0.135–0.447) | <0.001 | |
Location | |||||||
ACoA | 1.440 | 4.222 (2.960–6.021) | <0.001 | 1.084 | 2.956 (1.492–5.858) | 0.002 | |
PCoA | 1.241 | 3.459 (2.592–4.616) | <0.001 | 1.127 | 3.087 (1.608–5.925) | 0.001 | |
ICA | −2.241 | 0.106 (0.080–0.142) | <0.001 | −1.060 | 0.346 (0.151–0.794) | 0.012 | |
Multiple aneurysms | −0.599 | 0.550 (0.428–0.706) | <0.001 | −0.797 | 0.451 (0.278–0.731) | 0.001 | |
Bifurcation | 1.340 | 3.818 (3.013–4.840) | <0.001 | 1.212 | 3.359 (1.868–6.042) | <0.001 | |
Irregular shape | 3.463 | 31.899 (21.585–47.141) | <0.001 | 1.782 | 5.944 (3.368–10.490) | <0.001 | |
Maximum size | 0.816 | 2.260 (2.055–2.487) | <0.001 | 0.722 | 2.059 (1.755–2.415) | <0.001 | |
Parent artery diameter | −0.825 | 0.438 (0.381–0.504) | <0.001 | −0.878 | 0.415 (0.290–0.596) | <0.001 | |
AR | 4.633 | 102.860 (59.572–177.601) | <0.001 | 2.626 | 13.820 (6.414–29.775) | <0.001 |
β, partial regression coefficient; OR, odds ratio; CI, confidence interval; ACoA, anterior communicating artery; PCoA, posterior communicating artery; ICA, internal carotid artery; AR, aspect ratio.
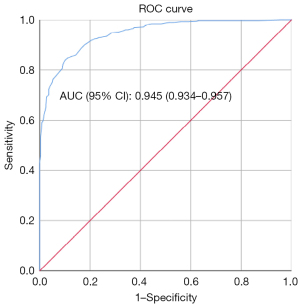
Table 4
Data sets | AUC (95% CI) | Sensitivity | Specificity | Accuracy |
---|---|---|---|---|
Training + validation + test set | 0.945 (0.934–0.957) | 0.709 | 0.885 | 0.867 |
External validation set | 0.963 (0.941–0.986) | 0.922 | 0.906 | 0.913 |
AUC, area under the curve; LRM, logistic regression model; IA, intracranial aneurysm; CI, confidence interval.
For the external validation set, we applied the predictive score (1.75) in 229 IAs and found that the sensitivity, specificity, and diagnostic accuracy were 0.922, 0.906, and 0.913, respectively (Table 4).
Diagnostic performance of the DLM
The model training was based on PyTorch framework 1.7.0, an NVIDIA TITAN XP GPU and 12 GB memory, and the whole process lasted for 40 h. Except for cerebral atherosclerosis, there were no significant differences in the clinical characteristics or morphological parameters of IAs between the training set, validation set, and test set (Tables 5,6).
Table 5
Patients clinical information | Training set (n=669) | Internal validation set (n=170) | Testing set (n=210) | P |
---|---|---|---|---|
Female (%) | 413 (61.7) | 111 (65.3) | 113 (53.8) | 0.052 |
Age (years, mean ± SD) | 58.31±12.33 | 57.12±12.17 | 59.29±13.66 | 0.824 |
Hypertension (%) | 285 (42.6) | 73 (42.9) | 96 (45.7) | 0.719 |
Heart disease (%) | 57 (8.5) | 16 (9.4) | 17 (8.1) | 0.839 |
Diabetes mellitus (%) | 41 (6.1) | 14 (8.2) | 18 (8.6) | 0.320 |
Cerebrovascular sclerosis (%) | 93 (13.9) | 20 (11.8) | 44 (21.0) | 0.024 |
Alcohol consumption (%) | 141 (21.1) | 37 (21.8) | 39 (18.6) | 0.69 |
Smoking (%) | 177 (26.5) | 45 (26.5) | 46 (21.9) | 0.397 |
SAH history (%) | 21 (3.1) | 7 (4.1) | 7 (3.3) | 0.773 |
SD, standard deviation; SAH, subarachnoid hemorrhage.
Table 6
Aneurysms parameters | Training set (n=795) | Internal validation set (n=204) | Testing set (n=238) | P |
---|---|---|---|---|
Location (%) | ||||
ACoA | 137 (17.2) | 48 (23.5) | 36 (15.1) | 0.054 |
ACA | 36 (4.5) | 5 (2.5) | 15 (6.3) | 0.157 |
MCA | 126 (15.8) | 33 (16.2) | 49 (20.6) | 0.227 |
PCoA | 204 (25.7) | 54 (26.5) | 59 (24.8) | 0.906 |
ICA | 260 (32.7) | 59 (28.9) | 70 (29.4) | 0.469 |
PCA | 32 (4.0) | 5 (2.5) | 9 (3.8) | 0.638 |
Multiple aneurysms (%) | 230 (28.9) | 62 (30.4) | 55 (23.1) | 0.154 |
Bifurcation (%) | 394 (49.6) | 107 (52.5) | 126 (52.9) | 0.546 |
Irregular shape (%) | 304 (38.2) | 86 (42.2) | 92 (38.7) | 0.556 |
Daughter sac (%) | 210 (26.4) | 60 (29.4) | 51 (21.4) | 0.131 |
Neck width (mm) | 4.62±1.88 | 4.45±1.66 | 4.63±2.07 | 0.910 |
Height (mm) | 5.15±3.23 | 4.88±2.86 | 4.94±3.57 | 0.265 |
Depth (mm) | 5.57±3.50 | 5.30±3.11 | 5.34±3.74 | 0.203 |
Width (mm) | 5.29±3.36 | 4.99±3.06 | 5.14±3.89 | 0.478 |
Maximum size (mm) | 6.74±3.82 | 6.48±3.51 | 6.55±4.18 | 0.321 |
Parent artery diameter (mm) | 3.65± 0.94 | 3.60±0.97 | 3.64±0.96 | 0.818 |
Mean artery diameter (mm) | 3.36±0.91 | 3.32±0.93 | 3.31±0.96 | 0.808 |
AR | 1.21±0.59 | 1.22±0.63 | 1.15±0.51 | 0.065 |
DW | 1.08±0.33 | 1.10±0.37 | 1.08±0.33 | 0.262 |
BF | 1.13±0.46 | 1.12±0.48 | 1.08±0.39 | 0.116 |
SR | 1.79±1.25 | 1.77±1.11 | 1.76±1.43 | 0.180 |
FA | 112.76±27.92 | 112.20±27.96 | 113.09±29.63 | 0.458 |
Data are represented as mean ± standard deviation. ACoA, anterior communicating artery; ACA, anterior cerebral artery; MCA, middle cerebral artery; PCoA, posterior communicating artery; ICA, internal carotid artery; PCA, posterior circulation artery; AR, aspect ratio; SR, size ratio; DW, depth-to-width ratio; BF, bottleneck factor; FA, flow angle.
The AUCs of the final model on the training, validation, and testing sets were 0.939, 0.894, and 0.864, respectively (Figure 3). The optimal cutoff value of the training set was 0.441. The sensitivity, specificity and accuracy on the training set, internal validation set and testing set are listed in Table 7. The calibration curve showed that the predictive models overestimated the risk of disease to some extent, but the overall picture was approximately the same (Figure 4). The decision curve analysis showed that the classification model would bring more benefit than treating none or treating all most frequently when the risk threshold probability ranged from approximately 35% to 80% (Figure 5).
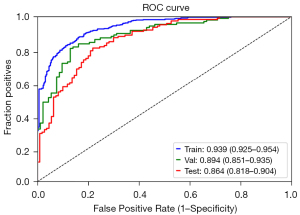
Table 7
Data sets | AUC (95% CI) | Sensitivity | Specificity | Accuracy |
---|---|---|---|---|
Training set | 0.939 (0.925–0.954) | 0.876 | 0.840 | 0.860 |
Validation set | 0.894 (0.851–0.935) | 0.801 | 0.871 | 0.833 |
Test set | 0.864 (0.818–0.904) | 0.815 | 0.742 | 0.784 |
External validation set | 0.771 (0.582–0.960) | 0.694 | 0.929 | 0.782 |
AUC, area under the curve; DLM, deep learning model; IA, intracranial aneurysm; CI, confidence interval.
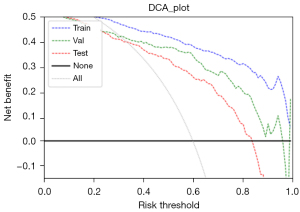
We applied the predictive score (0.441) in the external validation set and found that the sensitivity, specificity and accuracy for the diagnosis of unstable IA were 0.694, 0.929, and 0.782, respectively (Table 7).
Discussion
In this study, we developed two models using conventional LR and deep learning methods for the predicting stability of IAs based on CTA images, which were validated by an external validation set. The LRM results showed that heart disease, cerebral atherosclerosis, multiple IAs, IAs at the ICA, ACoA, or PCoA, located at the bifurcation, shape, parent artery diameter, maximum size and AR were associated with IA stability. The model displayed a good discriminatory ability in discriminating between unstable and stable IAs. The CNN-based DLM achieved high diagnostic performance in differentiating between stable and unstable IAs, but this model cannot outperform conventional LR in predicting the stability of IAs.
IA is a common disease with high mortality and morbidity rates if the IA ruptures. We must look for several contradictions when making treatment decisions for unruptured IAs: IAs have a high prevalence and a low risk of rupture in the population, so patients often choose follow-up observation, especially for small ones (25). However, the catastrophic consequences of IA rupture prompt most patients to undergo preventive treatment (26). Preventive treatment of the majority of unruptured IAs has proven to be a waste of medical resources (27). All of these contradictions suggest that identifying IA stability is extremely important.
In the past, the prediction of IA rupture was mainly based on the clinical characteristics of patients and the morphological parameters of IAs. In clinical circumstances, the location and size of IAs are important risk factors for IA rupture. The annual rupture rate of IAs in the ICA, PCoA, and MCA is 1.6%, whereas the annual rupture rates of IAs located in the main ACoA and the PCA are higher, at 1.9% and 4%, respectively (28,29). Size is an important determinant of rupture risk. The risk of IA rupture was significantly increases when the IA was ≥7 mm (30). Thanks to technical advances and more detailed radiologic findings, such as irregular IA walls, multilobulation, and hemodynamic patterns, aneurysm wall shear stress (WSS) has gained attention (31). In this study, we also found that many factors, including patient clinical characteristics and morphological parameters of IAs, were associated with IA stability, and our findings are consistent with previous studies. However, in clinical work, the risk assessment of IA stability requires many complex preprocessing steps, which can consume a significant amount of the clinician’s time and energy.
In recent years, with the continuous development of AI, DLMs have shown significant potential in detecting and segmenting IAs. Yang et al. (18) trained a CNN-based algorithm that achieved 97.5% accuracy in detecting IAs. Zhu et al. (19) demonstrated the fast and accurate identification and segmentation of IAs using a deep-learning-based framework from CTA images: 3D-UNet exhibited a better overall segmentation performance under a relatively small sample size. Wang et al. (20) proved that the multiphase fusion DLM with automatic phase selection can automatically detect IAs more sensitively. However, to provide optimal treatment and clinical decision making for patients with unruptured IAs, we should focus more on assessing the risk of IA instability than on diagnosing the IA itself.
While AI, particularly deep learning, is useful for the prediction of IA rupture risk, its applications are still in their infancy. Liu et al. (32) established a two-layer feed-forward artificial neural network (ANN) prediction model for the rupture risk of ACoA aneurysms, which showed a prediction accuracy of 94.8%. However, 90.9% of the samples in their study were ruptured IAs, and the model could only apply to ACoA IAs. Kim et al. (33) used a CNN to evaluate the rupture risk of small IAs, and the sensitivity, specificity and overall diagnostic accuracy were 78.76%, 72.15% and 76.84%, respectively. In their study, the AUC in the CNN was 0.76, which was better than that obtained by a human evaluator (AUC: 0.54). The DLM based on hemodynamic parameters also had good predictive accuracy in assessing IA rupture risk (34). However, these studies only included anterior circulation IAs, and the results may not be applicable to other IA sites.
In this study, we established a CNN-based DLM using CTA images to predict the patient-specific risk of aneurysmal stability. This model had an accuracy of 0.782, a sensitivity of 0.694 and a specificity of 0.929 for predicting IA instability risk and showed an AUC of 0.771 on an external validation set. Unfortunately, the DLM cannot outperform the conventional LRM in predicting the stability of IAs. The potential factors contributing to this outcome are the comprehensive nature of our data, which encompasses all IAs without differentiation based on size or location, and the ongoing advancements in deep learning algorithms. This result also indicated that clinical factors are important in IA stability.
Limitations
This study has some limitations. First, it is a retrospective study. Our results have been only externally validated at one Hospital. A large multicenter prospective study on this topic is still needed. Second, the majority of the unstable IAs in this study were ruptured (65.35%, 445 of 681), and the postrupture morphology may have changed, which could bias our results (35). Third, the IA size was not finely graded in this study. The measurement and delineation of ROIs for small IAs may be more prone to divergence between different observers. Fourth, incidentally discovered IAs with no apparent symptoms were classified as stable, which may have led to misclassification of some unstable IAs; therefore, long-term follow-up is needed for stable IAs. Fifth, we used the maximum slices of IA images for 2D modeling, and some important factors that may affect IA stability may have been missed. More advanced algorithms are needed. Sixth, the lack of blinding regarding the patient’s diagnosis may potentially impact the annotation of IA contours. Finally, we did not use other DLMs for comparison; therefore, we are not sure which model is better. Combining clinical and IA morphology and increasing the training set, e.g., the DLM, may lead to better performance in predicting the stability of IAs.
Conclusions
Our study establishes a conventional LRM and a CNN-based DLM using CTA images to identify the unstable IAs. The DLM did not outperform the LRM in identifying unstable IAs, which was tested in the external validation set. In addition, the predictive scoring model according to patient clinical and IAs morphology is of great value in predicting the stability of IAs and may help clinicians and patients make the right decisions.
Acknowledgments
Funding: This study was supported by
Footnote
Reporting Checklist: The authors have completed the TRIPOD reporting checklist. Available at https://qims.amegroups.com/article/view/10.21037/qims-23-1732/rc
Conflicts of Interest: All authors have completed the ICMJE uniform disclosure form (available at https://qims.amegroups.com/article/view/10.21037/qims-23-1732/coif). Y.J. is an employee of Huiying Medical Technology (Beijing) Company. J.X.X. and C.C.H. are employees of Beijing Deepwise & League of PHD Technology Co., Ltd. The other authors have no conflicts of interest to declare.
Ethical Statement:
Open Access Statement: This is an Open Access article distributed in accordance with the Creative Commons Attribution-NonCommercial-NoDerivs 4.0 International License (CC BY-NC-ND 4.0), which permits the non-commercial replication and distribution of the article with the strict proviso that no changes or edits are made and the original work is properly cited (including links to both the formal publication through the relevant DOI and the license). See: https://creativecommons.org/licenses/by-nc-nd/4.0/.
References
- Etminan N, Rinkel GJ. Unruptured intracranial aneurysms: development, rupture and preventive management. Nat Rev Neurol 2016;12:699-713. [Crossref] [PubMed]
- Verma Y, Ramesh S, Perera Molligoda Arachchige AS. 7 T Versus 3 T in the Diagnosis of Small Unruptured Intracranial Aneurysms: Reply to Radojewski et al. Clin Neuroradiol 2024;34:51-2. [Crossref] [PubMed]
- Zhang LF, Yang J, Hong Z, Yuan GG, Zhou BF, Zhao LC, Huang YN, Chen J, Wu YFCollaborative Group of China Multicenter Study of Cardiovascular Epidemiology. Proportion of different subtypes of stroke in China. Stroke 2003;34:2091-6. [Crossref] [PubMed]
- Algra AM, Lindgren A, Vergouwen MDI, Greving JP, van der Schaaf IC, van Doormaal TPC, Rinkel GJE. Procedural Clinical Complications, Case-Fatality Risks, and Risk Factors in Endovascular and Neurosurgical Treatment of Unruptured Intracranial Aneurysms: A Systematic Review and Meta-analysis. JAMA Neurol 2019;76:282-93. [Crossref] [PubMed]
- Perera Molligoda Arachchige AS, Vitamin K. Antagonists and Intracranial Hemorrhage After Endovascular Thrombectomy. JAMA 2023;330:1490. [Crossref] [PubMed]
- Wang GX, Liu LL, Yang Y, Wen L, Duan CM, Yin JB, Zhang D. Risk factors for the progression of unruptured intracranial aneurysms in patients followed by CT/MR angiography. Quant Imaging Med Surg 2021;11:4115-24. [Crossref] [PubMed]
- Backes D, Rinkel GJE, Greving JP, Velthuis BK, Murayama Y, Takao H, et al. ELAPSS score for prediction of risk of growth of unruptured intracranial aneurysms. Neurology 2017;88:1600-6. [Crossref] [PubMed]
- Backes D, Vergouwen MD, Tiel Groenestege AT, Bor AS, Velthuis BK, Greving JP, Algra A, Wermer MJ, van Walderveen MA, terBrugge KG, Agid R, Rinkel GJ. PHASES Score for Prediction of Intracranial Aneurysm Growth. Stroke 2015;46:1221-6. [Crossref] [PubMed]
- Brinjikji W, Chung BJ, Jimenez C, Putman C, Kallmes DF, Cebral JR. Hemodynamic differences between unstable and stable unruptured aneurysms independent of size and location: a pilot study. J Neurointerv Surg 2017;9:376-80. [Crossref] [PubMed]
- Foreman PM, Hendrix P, Harrigan MR, Fisher WS 3rd, Vyas NA, Lipsky RH, Walters BC, Tubbs RS, Shoja MM, Griessenauer CJ. PHASES score applied to a prospective cohort of aneurysmal subarachnoid hemorrhage patients. J Clin Neurosci 2018;53:69-73. [Crossref] [PubMed]
- Pagiola I, Mihalea C, Caroff J, Ikka L, Chalumeau V, Iacobucci M, Ozanne A, Gallas S, Marques M, Nalli D, Carrete H, Caldas JG, Frudit ME, Moret J, Spelle L. The PHASES score: To treat or not to treat? Retrospective evaluation of the risk of rupture of intracranial aneurysms in patients with aneurysmal subarachnoid hemorrhage. J Neuroradiol 2020;47:349-52. [Crossref] [PubMed]
- Zhu W, Li W, Tian Z, Zhang Y, Wang K, Zhang Y, Liu J, Yang X. Stability Assessment of Intracranial Aneurysms Using Machine Learning Based on Clinical and Morphological Features. Transl Stroke Res 2020;11:1287-95. [Crossref] [PubMed]
- Ou C, Liu J, Qian Y, Chong W, Zhang X, Liu W, Su H, Zhang N, Zhang J, Duan CZ, He X. Rupture Risk Assessment for Cerebral Aneurysm Using Interpretable Machine Learning on Multidimensional Data. Front Neurol 2020;11:570181. [Crossref] [PubMed]
- Lin M, Xia N, Lin R, Xu L, Chen Y, Zhou J, Lin B, Zheng K, Wang H, Jia X, Liu J, Zhu D, Chen C, Yang Y, Su N. Machine learning prediction model for the rupture status of middle cerebral artery aneurysm in patients with hypertension: a Chinese multicenter study. Quant Imaging Med Surg 2023;13:4867-78. [Crossref] [PubMed]
- Chen G, Lu M, Shi Z, Xia S, Ren Y, Liu Z, Liu X, Li Z, Mao L, Li XL, Zhang B, Zhang LJ, Lu GM. Development and validation of machine learning prediction model based on computed tomography angiography-derived hemodynamics for rupture status of intracranial aneurysms: a Chinese multicenter study. Eur Radiol 2020;30:5170-82. [Crossref] [PubMed]
- LeCun Y, Bengio Y, Hinton G. Deep learning. Nature 2015;521:436-44. [Crossref] [PubMed]
- Suzuki K. Overview of deep learning in medical imaging. Radiol Phys Technol 2017;10:257-73. [Crossref] [PubMed]
- Yang J, Xie M, Hu C, Alwalid O, Xu Y, Liu J, Jin T, Li C, Tu D, Liu X, Zhang C, Li C, Long X. Deep Learning for Detecting Cerebral Aneurysms with CT Angiography. Radiology 2021;298:155-63. [Crossref] [PubMed]
- Zhu G, Luo X, Yang T, Cai L, Yeo JH, Yan G, Yang J. Deep learning-based recognition and segmentation of intracranial aneurysms under small sample size. Front Physiol 2022;13:1084202. [Crossref] [PubMed]
- Wang J, Sun J, Xu J, Lu S, Wang H, Huang C, Zhang F, Yu Y, Gao X, Wang M, Wang Y, Ruan X, Pan Y. Detection of Intracranial Aneurysms Using Multiphase CT Angiography with a Deep Learning Model. Acad Radiol 2023;30:2477-86. [Crossref] [PubMed]
- Wermer MJ, van der Schaaf IC, Algra A, Rinkel GJ. Risk of rupture of unruptured intracranial aneurysms in relation to patient and aneurysm characteristics: an updated meta-analysis. Stroke 2007;38:1404-10. [Crossref] [PubMed]
- Wang GX, Gong MF, Wen L, Liu LL, Yin JB, Duan CM, Zhang D. Computed Tomography Angiography Evaluation of Risk Factors for Unstable Intracranial Aneurysms. World Neurosurg 2018;115:e27-32. [Crossref] [PubMed]
- Headache Classification Committee of the International Headache Society (IHS) The International Classification of Headache Disorders, 3rd edition. Cephalalgia 2018;38:1-211.
- Virtanen P, Gommers R, Oliphant TE, Haberland M, Reddy T, Cournapeau D, et al. Author Correction: SciPy 1.0: fundamental algorithms for scientific computing in Python. Nat Methods 2020;17:352. [Crossref] [PubMed]
- Wiebers DO, Whisnant JP, Huston J 3rd, Meissner I, Brown RD Jr, Piepgras DG, Forbes GS, Thielen K, Nichols D, O'Fallon WM, Peacock J, Jaeger L, Kassell NF, Kongable-Beckman GL, Torner JCInternational Study of Unruptured Intracranial Aneurysms Investigators. Unruptured intracranial aneurysms: natural history, clinical outcome, and risks of surgical and endovascular treatment. Lancet 2003;362:103-10. [Crossref] [PubMed]
- Johnston SC. Leaving Tiny, Unruptured Intracranial Aneurysms Untreated: Why Is It So Hard? JAMA Neurol 2018;75:13-4. [Crossref] [PubMed]
- Takao H, Nojo T. Treatment of unruptured intracranial aneurysms: decision and cost-effectiveness analysis. Radiology 2007;244:755-66. [Crossref] [PubMed]
- Juvela S. Natural history of unruptured intracranial aneurysms: risks for aneurysm formation, growth, and rupture. Acta Neurochir Suppl 2002;82:27-30. [Crossref] [PubMed]
- Clarke G, Mendelow AD, Mitchell P. Predicting the risk of rupture of intracranial aneurysms based on anatomical location. Acta Neurochir (Wien) 2005;147:259-63; discussion 263. [Crossref] [PubMed]
- Korja M, Lehto H, Juvela S. Lifelong rupture risk of intracranial aneurysms depends on risk factors: a prospective Finnish cohort study. Stroke 2014;45:1958-63. [Crossref] [PubMed]
- Jeon JS, Ahn JH, Huh W, Son YJ, Bang JS, Kang HS, Sohn CH, Oh CW, Kwon OK, Kim JE. A retrospective analysis on the natural history of incidental small paraclinoid unruptured aneurysm. J Neurol Neurosurg Psychiatry 2014;85:289-94. [Crossref] [PubMed]
- Liu J, Chen Y, Lan L, Lin B, Chen W, Wang M, Li R, Yang Y, Zhao B, Hu Z, Duan Y. Prediction of rupture risk in anterior communicating artery aneurysms with a feed-forward artificial neural network. Eur Radiol 2018;28:3268-75. [Crossref] [PubMed]
- Kim HC, Rhim JK, Ahn JH, Park JJ, Moon JU, Hong EP, Kim MR, Kim SG, Lee SH, Jeong JH, Choi SW, Jeon JP. Machine Learning Application for Rupture Risk Assessment in Small-Sized Intracranial Aneurysm. J Clin Med 2019;8:683. [Crossref] [PubMed]
- Yang H, Cho KC, Kim JJ, Kim JH, Kim YB, Oh JH. Rupture risk prediction of cerebral aneurysms using a novel convolutional neural network-based deep learning model. J Neurointerv Surg 2023;15:200-4. [Crossref] [PubMed]
- Skodvin TØ, Johnsen LH, Gjertsen Ø, Isaksen JG, Sorteberg A. Cerebral Aneurysm Morphology Before and After Rupture: Nationwide Case Series of 29 Aneurysms. Stroke 2017;48:880-6. [Crossref] [PubMed]