A nomogram based on contrast-enhanced ultrasound for evaluating the glomerulosclerosis rate in transplanted kidneys
Introduction
End-stage renal disease (ESRD) consists of renal failure necessitating kidney replacement therapy. Compared to other kidney replacement therapies, kidney transplantation has proven effective in improving the survival and health-related quality of life of patients with ESRD (1-3). The development of innovative immunosuppressive agents and human leukocyte antigen (HLA) typing technology has substantially improved 1-year graft survival rates. However, the rate of late graft loss has not shown much decline, remaining at 2–5% per year (4,5). Transplant glomerulopathy (TG), a pivotal pathological process, has garnered significant attention as one of the principal causes of late graft loss (6,7). Glomerular sclerosis is a noninflammatory glomerular lesion instigated by podocyte injury, which indicates the terminal stage of TG and is an irreparable histological change (8). Recent investigations have suggested that a greater proportion of glomeruli progressing to global or segmental sclerosis serves as a vital signal of poor response to treatment and a high risk of disease progression or adverse prognosis (9-11). Notably, Srivastava et al.’s study showed that patients with global a glomerulosclerosis rate >50% have a 6.3-fold increased risk of kidney disease progression (12).
Renal biopsy is considered the gold standard for evaluating glomerulosclerosis. However, as an invasive procedure, biopsy carries the risk of complications such as hematuria and arteriovenous fistula, and may be prone to sampling errors due to insufficient specimen collection. Thus, the development of efficacious noninvasive techniques to gauge the glomerulosclerosis rate of transplanted kidneys is imperative. These techniques could reduce the dependency on biopsies, aid in treatment strategies, and facilitate the prediction of patient prognosis and response to therapy. Contrast-enhanced ultrasound (CEUS) employs nonnephrotoxic ultrasound (US) contrast agents that have good safety when administered to kidney recipients (13). The microbubbles utilized in CEUS are confined to the intravascular space and exhibit rheological properties similar to those of red blood cells, enabling the precise evaluation of regional blood flow perfusion (14). Moreover, CEUS boasts higher temporal and spatial resolution than does contrast-enhanced computed tomography (CT) and magnetic resonance imaging (MRI) (15). Studies have revealed significant associations between CEUS parameters and the glomerulosclerosis rate or interstitial fibrosis and tubular atrophy (IFTA) in patients with chronic kidney disease (CKD) (16,17). However, there has been no published research on the use of CEUS to evaluate the glomerulosclerosis rate in transplanted kidneys.
Therefore, this study investigated the clinical significance of CEUS in assessing the glomerulosclerosis rate in renal allografts. A further aim was to develop a model based on clinical and ultrasound parameters to predict the glomerulosclerosis rate in transplanted kidneys, and subsequently, to rigorously validate this model. We present this article in accordance with the TRIPOD reporting checklist (available at https://qims.amegroups.com/article/view/10.21037/qims-23-1514/rc).
Methods
Patients
A retrospective single-center study was conducted on a cohort of 165 patients (≥18 years old) who had undergone renal biopsy in Jinling Hospital from January 2021 to May 2023. All patients involved satisfied the indications for transplant kidney biopsy as recommended by the Kidney Disease: Improving Global Outcomes (KDIGO) clinical practice guidelines (18) and underwent both conventional ultrasound (CUS) and CEUS examinations within 3 days before the biopsy. The exclusion criteria were as follows: (I) presence of renal artery thrombosis, renal artery stenosis, or arteriovenous fistula; (II) incomplete data; and (III) a biopsy specimen with fewer than 10 glomeruli or fewer than 2 arteries (19).
The clinical and US image data of 143 kidney recipients were collected according to the inclusion and exclusion criteria (Figure 1). The study was conducted in accordance with the Declaration of Helsinki (as revised in 2013) and was approved by the Ethics Committee of Jinling Hospital. As a retrospective design was employed, the need to obtain written informed consent was waived. Patient demographics, including sex, age, body mass index (BMI), time since transplantation, estimated glomerular filtration rate (eGFR), serum creatinine, urea nitrogen, uric acid, albumin, and proteinuria, were collected. The time since transplantation was considered to be the months between the end of kidney transplant surgery and the most recent kidney biopsy.
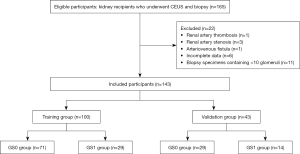
CUS and CEUS examinations
US examinations were performed using the Logiq E9 ultrasound machine (GE HealthCare) with a 2.5- to 6-MHz convex array transducer (C1–6). Before the examination, all machine parameters were set to the default values. All US examinations were performed by a radiologist with over 10 years of experience who was blinded to the patients’ clinicopathological and laboratory findings.
All patients underwent US examinations between 10:30 AM and 11:30 AM. They were instructed to rest for at least 10 minutes before the scan and were examined in a supine position. The patient’s systolic and diastolic blood pressure was measured before the US examinations were performed. CUS examinations of the renal graft were performed with 2D grayscale, color, and spectral Doppler ultrasound included. The length, cortical echogenicity, resistance index (RI), and peak systolic velocity (PSV) of the allograft were measured in the largest longitudinal section of the renal graft. Figure 2 shows typical ultrasound images from two patients. The classification of cortical echogenicity was based on the criteria proposed by Yang et al. (20). Hyperechoic was defined as a comparable echogenicity between the renal cortical and collecting systems, as shown in Figure 2A. Nonhyperechoicity was defined as a cortical echogenicity lower than that of the collecting system (Figure 2D). During color and spectral Doppler examinations, the sampling volume was kept at 0.5 mm, and the Doppler angle correction was kept parallel to the arteries in the range of 0–60°.
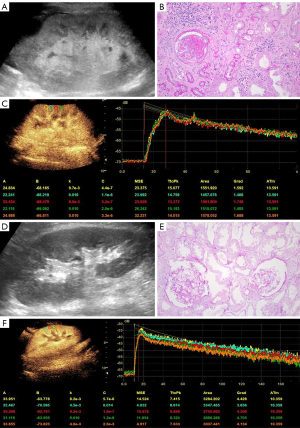
The largest longitudinal section of the renal graft was selected as the reference plane for CEUS following the CUS examination. Via the dual-image mode, 2 mL of contrast agent (SonoVue, Bracco) was injected through the patient’s cubital vein, which was followed by a 5-mL normal saline flush delivered at a rate of approximately 2 mL/s (21). The contrast procedure was continuously monitored, and images were stored in DICOM format (with a storage time of more than 2 minutes) for analysis. The mechanical index during CEUS was set to 0.10.
CEUS image analysis
Upon completion of CEUS imaging, we used time-intensity curve (TIC) analysis software integrated within the US machine to analyze the dynamic images obtained. Five equally spaced regions of interest (ROIs) measuring 5 mm × 5 mm were placed in the renal cortex on the proximal skin side, and the gamma variate function was selected following motion compensation. To obtain the best-fitting TIC, the first frame time was adjusted, and the average values of the following quantitative parameters were determined: (I) arrival time (AT), (II) time to peak (TTP), (III) absolute time to peak (ATTP), and (IV) peak intensity (PI) (with TTP = AT + ATTP. The details of the TIC are presented in Figure 2C,2F. Two radiologists who had more than a decade of professional experience and who were blinded to the patients’ clinicopathological and laboratory findings independently analyzed the TICs of all patients and recorded the average value of their respective analyses.
Renal allograft biopsy
A renal allograft biopsy is the medical procedure that obtains a small sample of tissue from a transplanted kidney for histopathological examination for the purposes of assessing the health and function of the transplanted kidney and to diagnose any potential issues such as rejection, infection, or other complications. All biopsies in this study were performed using the percutaneous technique, in which a puncture needle was inserted through the skin into the transplanted kidney under the guidance of US. Prior to renal allograft biopsy, patients routinely underwent thromboelastography and coagulation tests. After patients signed an informed consent form, a 16 G automatic biopsy needle was used to puncture the lower pole of the renal allograft, and 1–2 renal tissue specimens measuring 0.5–1.0 cm in length and 1 mm in diameter were obtained for pathological examination. These specimens were analyzed by two pathologists with over 5 years of experience. The glomerulosclerosis rate was used to divide transplant patients into the glomerulosclerosis rate ≤50% group (GS0) and glomerulosclerosis rate >50 (GS1) group according to the standardized grading proposal for chronic changes in renal biopsy specimens published by Sethi et al. (22). The glomerulosclerosis rate was calculated by dividing the sum of the global and segmental glomerulosclerosis by the total number of glomeruli and then multiplying the quotient by 100%. GS0 corresponded to grades 0–2 (glomerulosclerosis rate ≤50%, Figure 2E), and GS1 corresponded to grade 3 (glomerulosclerosis rate >50%, Figure 2B).
Statistical analysis
Statistical analysis was conducted using SPSS 26.0 (IBM Corp., USA), MedCalc 19.5.6 (MedCalc Software), and packages in R 4.0.3 (The R Foundation for Statistical Computing). Baseline characteristics were compared using an independent samples t test or one-way analysis variance (ANOVA) for continuous variables conforming to a normal distribution and are expressed as the mean ± standard deviation (SD). For continuous variables not following a normal distribution, the Mann-Whitney test or the Kruskal-Wallis test was applied, with the results being reported as the median (M) and interquartile range (IQR). Categorical variables were compared using the chi-squared test or Fisher exact test. Pearson correlation analysis was used to explore the relationships between the glomerulosclerosis rate and clinical and US indicators.
Patients were randomly allocated to a training group or a validation group in a 7:3 ratio. Univariate logistic analysis was conducted in the training group to identify significant variables (P<0.05) for subsequent multivariate analysis. Forward stepwise regression was applied in the multivariate analysis to identify independent predictors (P<0.05) associated with the glomerulosclerosis rate in the training group and to construct the nomogram. Receiver operating characteristic (ROC) curves were drawn to calculate their area under the curve (AUC) and to determine the best sensitivity and specificity of the ROC based on the Youden index. The DeLong test was employed to compare AUCs between the nomogram and independent predictors. Interval validation was performed using 1,000 bootstrap resamples. The calibration curve was drawn to compare the nomogram predictions with actual observations, while decision curve analysis (DCA) was used to evaluate the usefulness of the nomogram for decision-making. A two-sided P value <0.05 was considered statistically significant.
Results
Demographics and baseline characteristics
A total of 143 transplanted kidney recipients were included, including 106 men (106/143, 74.1%) and 37 women (37/143, 25.9%), with a median age of 39 (IQR 32–46) years and median time since transplantation of 35 (IQR 12–84) months. Among the recipient, 78.32% (112/143) received kidneys from deceased donors, and 20 recipients experienced delayed graft function (DGF) after transplantation. The pathological reasons for kidney transplantation were immunoglobin A nephropathy (19 patients), polycystic kidney disease (4 patients), purpura nephritis (4 patients), hypertensive nephropathy (4 patients), diabetic nephropathy (3 patients), lupus nephritis (3 patients), membranous nephropathy (3 patients), mesangial proliferative glomerulonephritis (3 patients), focal segmental glomerulosclerosis (2 patients), membranoproliferative glomerulonephritis (1 patient), crescentic glomerulonephritis (1 patient), acute kidney injury (1 patient), bilateral ureteral obstruction (1 patient), and CKD of unknown etiology (94 cases). Among the 94 recipients with CKD, 8 had abnormal renal function but were left untreated, 34 had abnormal renal function acknowledged without subsequent biopsy, for which only conservative management was selected, and 52 had indications for dialysis that were already met at the time of the initial consultation.
Table 1 displays the other baseline characteristics of the 143 kidney recipients. All recipients underwent renal biopsy, which revealed that 100 patients had a glomerulosclerosis rate of ≤50% and 43 had a rate of >50%. Detailed information on GS0 and GS1 is shown in Table 2. The age, time since transplantation, serum creatinine, urea nitrogen, uric acid, proteinuria, proportion of cortical hyperechoicity, RI of the interlobar artery, TTP and ATTP of GS1 were significantly higher than those of GS0. The eGFR, albumin, urine specific gravity, PSV of the interlobar artery, PSV of the arcuate artery, and PI of GS1 were significantly lower than those of GS0.
Table 1
Characteristic | Total (n=143) | Training group (n=100) | Validation group (n=43) | P value |
---|---|---|---|---|
Clinical index | ||||
Age (years) | 39 (32, 46) | 40 (33, 47) | 34 (30, 44) | 0.127 |
Sex (male/female) | 106/37 | 71/29 | 35/8 | 0.429 |
BMI (kg/m²) | 22.4 (20.4, 25.1) | 22.1 (19.7, 25.0) | 22.5 (20.8, 25.2) | 0.883 |
Systolic blood pressure (mmHg) | 131.1±14.4 | 131.5±14.6 | 130.4±14.2 | 0.926 |
Diastolic blood pressure (mmHg) | 85.3±10.1 | 85.0±10.3 | 85.9±9.7 | 0.899 |
Time since transplantation (months) | 35 (12, 84) | 38 (12, 96) | 24 (13, 54) | 0.209 |
eGFR [mL/(min·1.73 m²)] | 37 (23, 54) | 37 (22, 52) | 38 (26, 59) | 0.821 |
Serum creatinine (mg/dL) | 2.0 (1.5, 2.9) | 2.0 (1.6, 3.1) | 2.0 (1.5, 2.7) | 0.989 |
Urea nitrogen (mg/dL) | 34.1 (23.6, 50.8) | 34.9 (24.5, 49.3) | 32.1 (20.3, 52.6) | 0.887 |
Uric acid (mg/dL) | 399.5±99.3 | 405.0±98.1 | 387.0±102.3 | 0.611 |
Albumin (g/L) | 38.7 (35.3, 41.9) | 38.2 (33.4, 41.8) | 39.6 (36.2, 42.2) | 0.263 |
Proteinuria (g/24 h) | 0.9 (0.4, 2.2) | 1.0 (0.4, 2.7) | 0.8 (0.4, 1.8) | 0.616 |
Ultrasound index | ||||
Kidney length (cm) | 112.5 (106.0, 120.0) | 113.5 (105.0, 120.3) | 112.5 (106.8, 119.0) | 0.994 |
Cortical echogenicity (hyperechoic/nonhyperechoic) |
21/122 | 15/85 | 6/37 | 0.987 |
PSV of interlobar artery (cm/s) | 33.2±7.5 | 31.9±7.2 | 36.3±7.2 | 0.006 |
RI of interlobar artery | 0.61 (0.56, 0.65) | 0.62 (0.56, 0.65) | 0.60 (0.57, 0.63) | 0.609 |
PSV of arcuate artery (cm/s) | 22.5±5.5 | 21.9±5.5 | 23.8±5.5 | 0.160 |
RI of arcuate artery | 0.58 (0.54, 0.62) | 0.58 (0.55, 0.62) | 0.56 (0.52, 0.60) | 0.202 |
AT (s) | 11.0 (8.9, 13.0) | 11 .0 (9.0, 13.0) | 11.0 (8.4, 13.0) | 0.939 |
TTP (s) | 20.2 (16.9, 23.8) | 20.3 (16.8, 24.9) | 20.1 (16.9, 23.1) | >0.99 |
ATTP (s) | 9.0 (7.5, 11.0) | 9.5 (7.6, 11.0) | 8.9 (7.4, 11.0) | 0.305 |
PI (dB) | 28.3 (25.0, 31.0) | 28.7 (25.0, 31.4) | 28.0 (24.0, 30.4) | 0.609 |
Data are presented as the mean ± standard deviation or median (upper and lower quartiles). AT, arrival time; ATTP, absolute time to peak; BMI, body mass index; eGFR, estimated glomerular filtration rate; PI, peak intensity; PSV, peak systolic velocity; RI, resistance index; TTP, time to peak.
Table 2
Characteristic | GS0 group (n=100) | GS1 group (n=43) | t/Z/χ² value | P value |
---|---|---|---|---|
Clinical index | ||||
Age (years) | 37.9±8.9 | 41.4±9.9 | −2.066 | 0.041 |
Sex (male/female) | 75/25 | 31/12 | 0.132 | 0.716 |
BMI (kg/m²) | 22.2 (19.8, 25.1) | 22.2 (20.8, 24.7) | −0.004 | 0.996 |
Systolic blood pressure (mmHg) | 130.4±14.6 | 132.9±14.1 | −0.973 | 0.332 |
Diastolic blood pressure (mmHg) | 84.5±9.4 | 87.1±11.5 | −1.425 | 0.156 |
Time since transplantation (months) | 18 (10, 53) | 72 (48, 108) | −6.152 | <0.001 |
eGFR [mL/(min·1.73 m²)] | 41 (28, 59) | 28 (17, 46) | −3.082 | 0.002 |
Serum creatinine (mg/dL) | 1.9 (1.5, 2.6) | 2.5 (1.8, 3.9) | −2.969 | 0.003 |
Urea nitrogen (mg/dL) | 31.0 (22.7, 50.0) | 41.6 (29.2, 52.2) | −2.089 | 0.037 |
Uric acid (mg/dL) | 383.9±99.7 | 436.0±89.5 | −2.953 | 0.004 |
Albumin (g/L) | 40.5 (37.5, 42.6) | 35.5 (32.5, 37.0) | −5.391 | <0.001 |
Proteinuria (g/24 h) | 0.6 (0.4, 1.3) | 2.1 (1.1, 3.7) | −5.076 | <0.001 |
Ultrasound index | ||||
Kidney length (cm) | 112.9±10.4 | 113.8±12.8 | −0.447 | 0.655 |
Cortical echogenicity (hyperechoic/nonhyperechoic) |
2/98 | 19/24 | 42.713 | <0.001 |
PSV of interlobar artery (cm/s) | 34.7±6.7 | 29.8±8.0 | 3.766 | <0.001 |
RI of interlobar artery | 0.60 (0.55, 0.64) | 0.62 (0.58, 0.66) | −1.995 | 0.046 |
PSV of arcuate artery (cm/s) | 23.5±5.6 | 20.0±4.5 | 3.637 | <0.001 |
RI of arcuate artery | 0.58 (0.53, 0.61) | 0.58 (0.55, 0.63) | −0.849 | 0.396 |
AT (s) | 11.0 (8.6, 13.0) | 11.0 (8.9, 14.0) | −0.659 | 0.510 |
TTP (s) | 19.5 (16.1, 23.0) | 22.0 (17.5, 26.0) | −2.226 | 0.026 |
ATTP (s) | 9.0 (7.2, 10.9) | 10.0 (8.5, 13.0) | −2.880 | 0.004 |
PI (dB) | 30.0 (27.0, 32.0) | 25.0 (23.0, 27.9) | −5.050 | <0.001 |
Data are presented as the mean ± standard deviation or median (upper and lower quartiles). GS0, glomerulosclerosis rate ≤50%; GS1, glomerulosclerosis rate >50%; BMI, body mass index; eGFR, estimated glomerular filtration rate; PSV, peak systolic velocity; RI, resistance index; AT, arrival time; TTP, time to peak; ATTP, absolute time to peak; PI, peak intensity.
Relationship between glomerulosclerosis rate and clinical and US indicators
The correlations between the glomerulosclerosis rate and clinical and US indicators are shown in Table 3. Except for renal length, RI of the arcuate artery, and AT, all the indicators were significantly correlated with the glomerulosclerosis rate (all P values <0.05).
Table 3
Characteristic | Correlation coefficient | P value |
---|---|---|
Clinical index | ||
Age (years) | 0.257 | 0.002 |
Time since transplantation (months) | 0.481 | <0.001 |
eGFR [mL/(min·1.73 m²)] | −0.254 | 0.002 |
Serum creatinine (mg/dL) | 0.287 | 0.001 |
Urea nitrogen (mg/dL) | 0.199 | 0.017 |
Uric acid (mg/dL) | 0.259 | 0.002 |
Proteinuria (g/24 h) | 0.287 | 0.001 |
Ultrasound index | ||
Kidney length (cm) | 0.068 | 0.418 |
Cortical echogenicity | 0.551 | <0.001 |
PSV of interlobar artery (cm/s) | −0.289 | <0.001 |
RI of interlobar artery | 0.184 | 0.028 |
PSV of arcuate artery (cm/s) | −0.307 | <0.001 |
RI of arcuate artery | 0.064 | 0.444 |
AT (s) | 0.111 | 0.187 |
TTP (s) | 0.214 | 0.010 |
ATTP (s) | 0.238 | 0.004 |
PI (dB) | −0.363 | <0.001 |
eGFR, estimated glomerular filtration rate; PSV, peak systolic velocity; RI, resistance index; AT, arrival time; TTP, time to peak; ATTP, absolute time to peak; PI, peak intensity.
Independent predictors of glomerulosclerosis rate
The training group comprised 100 cases, including 71 in the GS0 group and 29 in the GS1 group, while the validation group comprised 43 cases, including 29 in the GS0 group and 14 in the GS1 group.
Following univariate and multivariate logistic analyses of various variables in the training group, the independent predictors of a glomerulosclerosis rate >50% were found to be PI, the PSV of the interlobar artery, cortical echogenicity, and time since transplantation (Table 4). Further analysis of the diagnostic performance of these four independent predictors is presented in Table 5.
Table 4
Characteristic | Univariate | Multivariate | |||
---|---|---|---|---|---|
OR (95% CI) | P value | OR (95% CI) | P value | ||
Clinical index | |||||
Age (years) | 1.044 (0.995–1.095) | 0.077 | |||
Sex (male/female) | 1.102 (0.422–2.881) | 0.842 | |||
BMI (kg/m²) | 1.018 (0.914–1.133) | 0.751 | |||
Time since transplantation (months) | 1.016 (1.008–1.025) | <0.001 | 1.017 (1.006–1.028) | 0.003 | |
eGFR [mL/(min·1.73 m²)] | 0.961 (0.935–0.988) | 0.004 | |||
Serum creatinine (mg/dL) | 1.778 (1.209–2.615) | 0.003 | |||
Urea nitrogen (mg/dL) | 1.020 (0.994–1.047) | 0.132 | |||
Uric acid (mg/dL) | 1.005 (1.000–1.010) | 0.035 | |||
Proteinuria (g/24 h) | 1.108 (0.983–1.249) | 0.093 | |||
Ultrasound index | |||||
Kidney length (cm) | 1.004 (0.968–1.041) | 0.844 | |||
Cortical echogenicity (hyperechoic) | 28.031 (5.745–136.762) | <0.001 | 38.429 (3.695–399.641) | 0.002 | |
PSV of interlobar artery (cm/s) | 0.894 (0.833–0.959) | 0.002 | 0.850 (0.758–0.954) | 0.006 | |
RI of interlobar artery | 1.032 (0.979–1.089) | 0.243 | |||
PSV of arcuate artery (cm/s) | 0.857 (0.776–0.945) | 0.002 | |||
RI of arcuate artery | 0.994 (0.938–1.053) | 0.831 | |||
AT (s) | 1.059 (0.929–1.207) | 0.391 | |||
TTP (s) | 1.069 (0.989–1.156) | 0.094 | |||
ATTP (s) | 1.151 (1.005–1.319) | 0.042 | |||
PI (dB) | 0.795 (0.701–0.902) | <0.001 | 0.852 (0.737–0.986) | 0.031 |
OR, odds ratio; CI, confidence interval; BMI, body mass index; eGFR, estimated glomerular filtration rate; PSV, peak systolic velocity; RI, resistance index; AT, arrival time; TTP, time to peak; ATTP, absolute time to peak; PI, peak intensity.
Table 5
Characteristic | Group | Specificity | Sensitivity | AUC (95% CI) |
---|---|---|---|---|
US model | Training | 0.747 | 0.897 | 0.875 (0.794–0.933) |
Validation | 0.759 | 0.857 | 0.882 (0.747–0.960) | |
Nomogram | Training | 0.761 | 0.897 | 0.914 (0.840–0.960) |
Validation | 0.862 | 0.857 | 0.909 (0.781–0.975) | |
PI (dB) | Training | 0.704 | 0.759 | 0.757 (0.661–0.838) |
Validation | 0.690 | 0.786 | 0.775 (0.622–0.888) | |
PSV of interlobar artery (cm/s) | Training | 0.831 | 0.483 | 0.686 (0.586–0.775) |
Validation | 0.897 | 0.429 | 0.676 (0.516–0.811) | |
Cortical echogenicity (hyperechoic) | Training | 0.972 | 0.448 | 0.710 (0.611–0.796) |
Validation | 0.966 | 0.429 | 0.697 (0.538–0.828) | |
Time since transplantation (months) | Training | 0.592 | 0.966 | 0.821 (0.732–0.891) |
Validation | 0.759 | 0.929 | 0.842 (0.699–0.935) |
AUC, area under the curve; CI, confidence interval; US, ultrasound; PI, peak intensity; PSV, peak systolic velocity.
Construction and validation of the US model
The independent predictors, including PI, the PSV of the interlobar artery, and cortical echogenicity, are all US parameters. A predictive US model for a glomerulosclerosis rate >50% was established by combining these US parameters, and its diagnostic performance was evaluated by plotting its ROC curve, as presented in Figure 3 and Table 5.
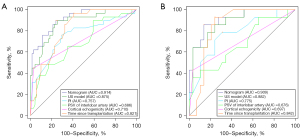
Construction of the nomogram
To enhance the diagnostic efficacy of our US model, we developed a nomogram model to predict whether the glomerulosclerosis rate would be >50% based on the four independent predictors selected by the multivariate analysis. The sum of scores assigned to each variable yielded the predicted probability of a glomerulosclerosis rate >50%. The details of the nomogram are shown in Figure 4.
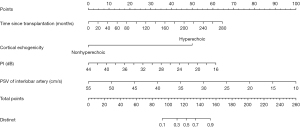
Validation of the nomogram
ROC curves were plotted to assess the diagnostic performance of the nomogram (Figure 3), the details of which are provided in Table 5. The DeLong test showed that the AUC of the nomogram was significantly higher than that of PI, the PSV of the interlobar artery, cortical echogenicity, and time since transplantation (P<0.05). Internal validation was performed by 1000 bootstrap resamples in the training group, and the AUC was 0.877. The calibration curves of the nomogram confirmed the excellent concordance between the model predictions and actual observations, while the DCA verified the clinical utility of the nomogram model (Figure 5).
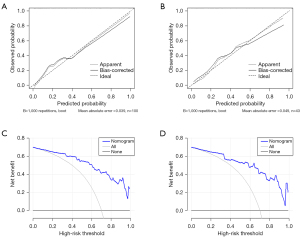
Discussion
The rate of glomerulosclerosis is a reliable indication of the severity of chronic changes, the patient’s response to treatment, and the risk of disease progression in a transplanted kidney (9,10). Multiple studies have substantiated a significant correlation between the glomerulosclerosis rate and tubular atrophy/interstitial fibrosis (r=0.64–0.74; P<0.05), with intraclass correlation coefficients for morphometric measurements of the glomerulosclerosis rate even exhibiting greater consistency (11,12,23). Thus, the glomerulosclerosis rate serves as a potent gauge for the extent of chronicity concerning transplanted kidney pathology. In this study, for the first time, CEUS was used to assess the glomerulosclerosis rate in transplanted kidneys. We constructed and validated a nomogram model to predict whether a glomerulosclerosis rate >50%. The model demonstrated an AUC of 0.914, a sensitivity of 89.7%, and a specificity of 76.1%. To further verify the model’s predictive ability, we set up a validation group and plotted calibration curves and DCA curves. Our results revealed that the PI, the PSV of the interlobar artery, cortical echogenicity, and time since transplantation were independent predictors of a glomerulosclerosis rate >50%.
As a CEUS intensity parameter, PI represents the maximum level of renal cortical blood flow perfusion, which is reflected by the highest concentration of contrast agents within the ROI during the examination. PI is highly sensitive to decreases in renal plasma flow and can well reflect changes in the renal cortical microcirculation (24). It is also significantly associated with the severity of chronic changes and is an independent predictor of CKD progression (25). Our results demonstrated a significant negative correlation between PI and glomerulosclerosis rate (r=–0.363; P<0.001), and the AUC for a diagnosing glomerulosclerosis rate >50% was 0.757, with sensitivities and specificities of 75.9% and 70.4%, respectively. Segmental and global glomerulosclerosis can lead to capillary luminal obliteration (22), impeding the entry of microbubbles into the sclerotic glomerulus and consequently reducing renal cortical microbubble perfusion, thereby leading to a decreased PI.
Consistent with our findings, Wang et al.’s study on diabetic nephropathy showed that PI decreased with increasing severity of glomerular damage and was negatively correlated with the glomerulosclerosis rate (r=–0.331; P=0.01) (17). However, in Wang et al.’s study, the median PI was 2,488 a.u when the glomerulosclerosis rate was >50%, whereas the median PI in our study was 25 dB. Therefore, while our study arrived at a similar conclusion, discrepancies arose due to differences in image intensity displays (arbitrary units) among scanners, nonstandardized linearization software and curve-fitting algorithms, and a nonstandard quantification approach, which renders intensity parameters in CEUS unable to be compared across various centers (26).
Our results indicated there to be a significant positive correlation of the glomerulosclerosis rate with the RI of the interlobar artery and a negative correlation with the PSV of the interlobar artery and of the arcuate artery. Notably, the decrease in the PSV of the interlobar artery was an independent predictor of a glomerulosclerosis rate >50%. These findings suggest that as the glomerulosclerosis rate increases, intrarenal vessel resistance increases and flow velocity declines.
Regarding the time parameters of CEUS, we observed a significant positive correlation between the glomerulosclerosis rate and TTP and ATTP. This could be because both TTP and ATTP encompass the period from the contrast agent’s arrival in the transplanted kidney to when the signal intensity of the renal cortex reaches PI, reflecting changes in intrarenal microcirculation. As the glomerulosclerosis rate increased, TTP and ATTP increased, indicating an extended intrarenal microcirculation time, which aligned with the findings from spectral Doppler US. In contrast, AT was not associated with the glomerulosclerosis rate. This may be because AT represents the time from contrast agent injection from the cubital vein to its arrival in the transplanted kidney, primarily reflecting the patient’s systemic circulation time, which is influenced by factors such as blood pressure and heart rate and has little to do with renal pathological changes.
This study also confirmed that a hyperechoic cortex is a reliable predictor of the glomerulosclerosis rate in transplanted kidneys. As the glomerulosclerosis rate increased, the probability of cortical hyperechoicity grew (r=0.551; P<0.001). A parallel correlation was reported by Sammy et al. in their study of 207 patients with CKD, in which they also noted a connection between glomerulosclerosis rate and cortical echogenicity (r=0.3; P<0.001) (27). Both cortical hyperechoicity and indistinct corticomedullary differentiation are crucial indicators of chronic changes, whether in autologous or transplanted kidneys (20). These findings could be attributed to the deposition of collagen fibers in glomeruli, leading to amplified ultrasound reflection and a cortical hyperechoic appearance on grayscale imaging.
We developed a US model based on the PI, the PSV of the interlobar artery, and cortical echogenicity. As indicated by ROC analysis, this model achieved an impressive AUC of 0.875, with a sensitivity of 89.7% and a specificity of 74.7%. Among these US parameters, PI demonstrated the highest AUC and Youden index, thus further confirming the preeminence of CEUS.
Our correlation analysis indicated that time since transplantation was positively associated with the glomerulosclerosis rate (r=0.481; P<0.001) and that it was an independent risk factor for a glomerulosclerosis rate >50%. One plausible explanation for this is that as individuals age, their kidneys undergo a natural aging process, leading to a higher incidence of glomerulosclerosis even in the absence of apparent clinical comorbidities such as hypertension or diabetes, as multiple studies have shown (28,29). Vaulet et al. analyzed 3,549 tissue specimens from different time points after transplantation and observed that the time after transplantation correlated with the overall chronicity of the biopsy specimens (30). Denic et al. reported that the mean percentage of glomerulosclerosis in patients with transplanted kidneys increased from 3.2% at implantation to 13.2% at 5 years after surgery (31). Another study demonstrated that the proportion of patients with a glomerulosclerosis rate >20% increased from 0% to 47% 10 years after transplantation (32). These studies confirm that the sustained influence of immune and nonimmune factors (such as hypertension and drug toxicity) results in a proclivity for chronic renal pathological changes and an increased incidence of glomerulosclerosis.
The significant correlation (P<0.05) between the glomerulosclerosis rate and various clinical indicators of renal function (eGFR, serum creatinine, uric acid, and proteinuria) in our study suggested a strong relationship between the severity of renal impairment and the rate of glomerulosclerosis. Currently, the noninvasive diagnosis of pathological changes mainly relies on eGFR and serum creatinine. However, these two indicators are prone to significant fluctuations and can be influenced by multiple factors, including sex and muscle mass (33). Hyperuricemia is a common clinical manifestation in transplanted kidney recipients, and several studies have shown that uric acid is correlated with renal function and nutritional status. However, after confounding factors such as renal function were adjusted for, uric acid was no longer an independent risk factor for graft loss (34,35), which is consistent with our study. Although some studies have concluded that proteinuria is an independent predictor of long-term graft loss (36,37), we did not find proteinuria to have independent predictive value. This could be because the enrolled patients had a minimum time since transplantation of only 1 month, and early proteinuria after transplantation might be caused by underlying autologous kidney disease and may not be related to the pathological alterations in the transplanted kidney (38).
Our study demonstrated the application value of CEUS in evaluating the glomerulosclerosis rate. Unfortunately, conventional CEUS imaging is limited in its resolution, and the extracted parameters are linked only indirectly to modifications in the microcirculation. Based on CEUS, US localization microscopy (UML) is a novel technique that can generate a super-resolved map of the microvasculature at the micrometer scale by locating individual injected microbubbles and tracking their displacement with subwavelength resolution (39). ULM has been reported to be capable of revealing different renal structures, with ULM maps reaching a 2 to 4-times thinner vessel diameter than the conventional high-resolution Doppler modes. In conjunction with this, ULM is able to provide quantitative information on blood velocities in the cortical area (40). Moreover, the application of sensing ULM (sULM) technology allows for the observation of individual glomeruli within transplanted kidneys (41). Therefore, we believe that inclusion of ULM in subsequent research can improve our results.
Our study has certain limitations that must be accounted for. (I) We employed a single-center, retrospective study design with a small sample, and the diagnostic model was not externally validated. (II) Although kidney biopsy is considered the gold standard, it is limited by sampling error, as is the measurement of CEUS parameters. (III) During the process of constructing the nomogram, there was an excessive reliance on statistical outcomes, resulting in the omission of certain clinically significant indicators, particularly those of renal function. (IV) Our study focused only on the glomerulosclerosis rate, whereas the pathologic changes in transplanted kidneys are complex and varied, and other pathological changes should be included in future studies. (V) Finally, patients with unfavorable US or laboratory findings were excluded from the study because they did not undergo renal biopsy.
Conclusions
Our nomogram, comprising CUS, CEUS, and clinical parameters exhibited sound predictive capability for determining whether the glomerulosclerosis rate of the renal graft would exceed 50%. The application of this model has the potential to reduce the need for repetitive biopsies and provide predictive insight into patients’ treatment response and graft survival.
Acknowledgments
We would like to thank AJE (https://www.aje.cn/) and ChatGPT (https://openai.com) for language editing services.
Funding: None.
Footnote
Reporting Checklist: The authors have completed the TRIPOD reporting checklist. Available at https://qims.amegroups.com/article/view/10.21037/qims-23-1514/rc
Conflicts of Interest: All authors have completed the ICMJE uniform disclosure form (available at https://qims.amegroups.com/article/view/10.21037/qims-23-1514/coif). The authors have no conflicts of interest to declare.
Ethical Statement: The authors are accountable for all aspects of the work in ensuring that questions related to the accuracy or integrity of any part of the work are appropriately investigated and resolved. This study was conducted in accordance with the Declaration of Helsinki (as revised in 2013) and was approved by the ethics committee of Jinling Hospital. Individual consent for this retrospective analysis was waived.
Open Access Statement: This is an Open Access article distributed in accordance with the Creative Commons Attribution-NonCommercial-NoDerivs 4.0 International License (CC BY-NC-ND 4.0), which permits the non-commercial replication and distribution of the article with the strict proviso that no changes or edits are made and the original work is properly cited (including links to both the formal publication through the relevant DOI and the license). See: https://creativecommons.org/licenses/by-nc-nd/4.0/.
References
- Saran R, Robinson B, Abbott KC, Bragg-Gresham J, Chen X, Gipson D, et al. US Renal Data System 2019 Annual Data Report: Epidemiology of Kidney Disease in the United States. Am J Kidney Dis 2020;75:A6-7. [Crossref] [PubMed]
- Kaballo MA, Canney M, O'Kelly P, Williams Y, O'Seaghdha CM, Conlon PJ. A comparative analysis of survival of patients on dialysis and after kidney transplantation. Clin Kidney J 2018;11:389-93. [Crossref] [PubMed]
- Fletcher BR, Damery S, Aiyegbusi OL, Anderson N, Calvert M, Cockwell P, Ferguson J, Horton M, Paap MCS, Sidey-Gibbons C, Slade A, Turner N, Kyte D. Symptom burden and health-related quality of life in chronic kidney disease: A global systematic review and meta-analysis. PLoS Med 2022;19:e1003954. [Crossref] [PubMed]
- Coemans M, Süsal C, Döhler B, Anglicheau D, Giral M, Bestard O, Legendre C, Emonds MP, Kuypers D, Molenberghs G, Verbeke G, Naesens M. Analyses of the short- and long-term graft survival after kidney transplantation in Europe between 1986 and 2015. Kidney Int 2018;94:964-73. [Crossref] [PubMed]
- Stegall MD, Gaston RS, Cosio FG, Matas A. Through a glass darkly: seeking clarity in preventing late kidney transplant failure. J Am Soc Nephrol 2015;26:20-9. [Crossref] [PubMed]
- Denic A, Morales MC, Park WD, Smith BH, Kremers WK, Alexander MP, Cosio FG, Rule AD, Stegall MD. Using computer-assisted morphometrics of 5-year biopsies to identify biomarkers of late renal allograft loss. Am J Transplant 2019;19:2846-54. [Crossref] [PubMed]
- Kovács G, Devercelli G, Zelei T, Hirji I, Vokó Z, Keown PA. Association between transplant glomerulopathy and graft outcomes following kidney transplantation: A meta-analysis. PLoS One 2020;15:e0231646. [Crossref] [PubMed]
- Nagata M. Podocyte injury and its consequences. Kidney Int 2016;89:1221-30. [Crossref] [PubMed]
- Zee J, Liu Q, Smith AR, Hodgin JB, Rosenberg A, Gillespie BW, Holzman LB, Barisoni L, Mariani LH. Kidney Biopsy Features Most Predictive of Clinical Outcomes in the Spectrum of Minimal Change Disease and Focal Segmental Glomerulosclerosis. J Am Soc Nephrol 2022;33:1411-26. [Crossref] [PubMed]
- Tan J, Xu Y, Jiang Z, Pei G, Tang Y, Tan L, Zhong Z, Tarun P, Qin W. Global Glomerulosclerosis and Segmental Glomerulosclerosis Could Serve as Effective Markers for Prognosis and Treatment of IgA Vasculitis With Nephritis. Front Med (Lausanne) 2020;7:588031. [Crossref] [PubMed]
- Denic A, Bogojevic M, Mullan AF, Sabov M, Asghar MS, Sethi S, Smith ML, Fervenza FC, Glassock RJ, Hommos MS, Rule AD. Prognostic Implications of a Morphometric Evaluation for Chronic Changes on All Diagnostic Native Kidney Biopsies. J Am Soc Nephrol 2022;33:1927-41. [Crossref] [PubMed]
- Srivastava A, Palsson R, Kaze AD, Chen ME, Palacios P, Sabbisetti V, Betensky RA, Steinman TI, Thadhani RI, McMahon GM, Stillman IE, Rennke HG, Waikar SS. The Prognostic Value of Histopathologic Lesions in Native Kidney Biopsy Specimens: Results from the Boston Kidney Biopsy Cohort Study. J Am Soc Nephrol 2018;29:2213-24. [Crossref] [PubMed]
- Bertolotto M, Bucci S, Valentino M, Currò F, Sachs C, Cova MA. Contrast-enhanced ultrasound for characterizing renal masses. Eur J Radiol 2018;105:41-8. [Crossref] [PubMed]
- Schneider A, Johnson L, Goodwin M, Schelleman A, Bellomo R. Bench-to-bedside review: contrast enhanced ultrasonography--a promising technique to assess renal perfusion in the ICU. Crit Care 2011;15:157. [Crossref] [PubMed]
- Atri M, Jang HJ, Kim TK, Khalili K. Contrast-enhanced US of the Liver and Kidney: A Problem-solving Modality. Radiology 2022;303:11-25. [Crossref] [PubMed]
- Yang W, Mou S, Xu Y, Du J, Xu L, Li F, Li H. Contrast-enhanced ultrasonography for assessment of tubular atrophy/interstitial fibrosis in immunoglobulin A nephropathy: a preliminary clinical study. Abdom Radiol (NY) 2018;43:1423-31. [Crossref] [PubMed]
- Wang Y, Zhao P, Li N, Dong Z, Lin L, Liu J, Liang S, Wang Q, Tang J, Luo Y. A Study on Correlation between Contrast-Enhanced Ultrasound Parameters and Pathological Features of Diabetic Nephropathy. Ultrasound Med Biol 2022;48:228-36. [Crossref] [PubMed]
- Kasiske BL, Zeier MG, Chapman JR, Craig JC, Ekberg H, Garvey CA, et al. KDIGO clinical practice guideline for the care of kidney transplant recipients: a summary. Kidney Int 2010;77:299-311. [Crossref] [PubMed]
- Racusen LC, Solez K, Colvin RB, Bonsib SM, Castro MC, Cavallo T, et al. The Banff 97 working classification of renal allograft pathology. Kidney Int 1999;55:713-23. [Crossref] [PubMed]
- Yang D, Wang Y, Zhuang B, Xu M, Wang C, Xie X, Huang G, Zheng Y, Xie X. Nomogram based on high-frequency shear wave elastography (SWE) to evaluate chronic changes after kidney transplantation. Eur Radiol 2023;33:763-73. [Crossref] [PubMed]
- Lyshchik A, Kono Y, Dietrich CF, Jang HJ, Kim TK, Piscaglia F, Vezeridis A, Willmann JK, Wilson SR. Contrast-enhanced ultrasound of the liver: technical and lexicon recommendations from the ACR CEUS LI-RADS working group. Abdom Radiol (NY) 2018;43:861-79. [Crossref] [PubMed]
- Sethi S, D'Agati VD, Nast CC, Fogo AB, De Vriese AS, Markowitz GS, et al. A proposal for standardized grading of chronic changes in native kidney biopsy specimens. Kidney Int 2017;91:787-9. [Crossref] [PubMed]
- Kawamura T, Joh K, Okonogi H, Koike K, Utsunomiya Y, Miyazaki Y, et al. A histologic classification of IgA nephropathy for predicting long-term prognosis: emphasis on end-stage renal disease. J Nephrol 2013;26:350-7. [Crossref] [PubMed]
- Schneider AG, Hofmann L, Wuerzner G, Glatz N, Maillard M, Meuwly JY, Eggimann P, Burnier M, Vogt B. Renal perfusion evaluation with contrast-enhanced ultrasonography. Nephrol Dial Transplant 2012;27:674-81. [Crossref] [PubMed]
- Xu Y, Li H, Wang C, Zhang M, Wang Q, Xie Y, Shao X, Tian L, Yuan Y, Yan W, Feng T, Li F, Ni Z, Mou S. Improving Prognostic and Chronicity Evaluation of Chronic Kidney Disease with Contrast-Enhanced Ultrasound Index-Derived Peak Intensity. Ultrasound Med Biol 2020;46:2945-55. [Crossref] [PubMed]
- Averkiou MA, Juang EK, Gallagher MK, Cuevas MA, Wilson SR, Barr RG, Carson PL. Evaluation of the Reproducibility of Bolus Transit Quantification With Contrast-Enhanced Ultrasound Across Multiple Scanners and Analysis Software Packages-A Quantitative Imaging Biomarker Alliance Study. Invest Radiol 2020;55:643-56. [Crossref] [PubMed]
- Moghazi S, Jones E, Schroepple J, Arya K, McClellan W, Hennigar RA, O'Neill WC. Correlation of renal histopathology with sonographic findings. Kidney Int 2005;67:1515-20. [Crossref] [PubMed]
- Hommos MS, Glassock RJ, Rule AD. Structural and Functional Changes in Human Kidneys with Healthy Aging. J Am Soc Nephrol 2017;28:2838-44. [Crossref] [PubMed]
- Denic A, Lieske JC, Chakkera HA, Poggio ED, Alexander MP, Singh P, Kremers WK, Lerman LO, Rule AD. The Substantial Loss of Nephrons in Healthy Human Kidneys with Aging. J Am Soc Nephrol 2017;28:313-20. [Crossref] [PubMed]
- Vaulet T, Divard G, Thaunat O, Koshy P, Lerut E, Senev A, Aubert O, Van Loon E, Callemeyn J, Emonds MP, Van Craenenbroeck A, De Vusser K, Sprangers B, Rabeyrin M, Dubois V, Kuypers D, De Vos M, Loupy A, De Moor B, Naesens M. Data-Driven Chronic Allograft Phenotypes: A Novel and Validated Complement for Histologic Assessment of Kidney Transplant Biopsies. J Am Soc Nephrol 2022;33:2026-39. [Crossref] [PubMed]
- Denic A, Bogojevic M, Subramani R, Park WD, Smith BH, Alexander MP, Grande JP, Kukla A, Schinstock CA, Bentall AJ, Rule AD, Stegall MD. Changes in Glomerular Volume, Sclerosis, and Ischemia at 5 Years after Kidney Transplantation: Incidence and Correlation with Late Graft Failure. J Am Soc Nephrol 2023;34:346-58. [Crossref] [PubMed]
- Stegall MD, Cornell LD, Park WD, Smith BH, Cosio FG. Renal Allograft Histology at 10 Years After Transplantation in the Tacrolimus Era: Evidence of Pervasive Chronic Injury. Am J Transplant 2018;18:180-8. [Crossref] [PubMed]
- Randers E, Erlandsen EJ. Serum cystatin C as an endogenous marker of the renal function--a review. Clin Chem Lab Med 1999;37:389-95. [Crossref] [PubMed]
- Kim ED, Famure O, Li Y, Kim SJ. Uric acid and the risk of graft failure in kidney transplant recipients: a re-assessment. Am J Transplant 2015;15:482-8. [Crossref] [PubMed]
- Kalil RS, Carpenter MA, Ivanova A, Gravens-Mueller L, John AA, Weir MR, Pesavento T, Bostom AG, Pfeffer MA, Hunsicker LG. Impact of Hyperuricemia on Long-term Outcomes of Kidney Transplantation: Analysis of the FAVORIT Study. Am J Kidney Dis 2017;70:762-9. [Crossref] [PubMed]
- Weiner DE, Park M, Tighiouart H, Joseph AA, Carpenter MA, Goyal N, House AA, Hsu CY, Ix JH, Jacques PF, Kew CE, Kim SJ, Kusek JW, Pesavento TE, Pfeffer MA, Smith SR, Weir MR, Levey AS, Bostom AG. Albuminuria and Allograft Failure, Cardiovascular Disease Events, and All-Cause Death in Stable Kidney Transplant Recipients: A Cohort Analysis of the FAVORIT Trial. Am J Kidney Dis 2019;73:51-61. [Crossref] [PubMed]
- Raynaud M, Aubert O, Divard G, Reese PP, Kamar N, Yoo D, et al. Dynamic prediction of renal survival among deeply phenotyped kidney transplant recipients using artificial intelligence: an observational, international, multicohort study. Lancet Digit Health 2021;3:e795-805. [Crossref] [PubMed]
- Amer H, Cosio FG. Significance and management of proteinuria in kidney transplant recipients. J Am Soc Nephrol 2009;20:2490-2. [Crossref] [PubMed]
- Christensen-Jeffries K, Couture O, Dayton PA, Eldar YC, Hynynen K, Kiessling F, O'Reilly M, Pinton GF, Schmitz G, Tang MX, Tanter M, van Sloun RJG. Super-resolution Ultrasound Imaging. Ultrasound Med Biol 2020;46:865-91. [Crossref] [PubMed]
- Bodard S, Denis L, Hingot V, Chavignon A, Hélénon O, Anglicheau D, Couture O, Correas JM. Ultrasound localization microscopy of the human kidney allograft on a clinical ultrasound scanner. Kidney Int 2023;103:930-5. [Crossref] [PubMed]
- Denis L, Bodard S, Hingot V, Chavignon A, Battaglia J, Renault G, Lager F, Aissani A, Hélénon O, Correas JM, Couture O. Sensing ultrasound localization microscopy for the visualization of glomeruli in living rats and humans. EBioMedicine 2023;91:104578. [Crossref] [PubMed]