Evaluation of four computed tomography reconstruction algorithms using a coronary artery phantom
Introduction
Coronary computed tomography angiography (CTA) is widely used for the screening of coronary artery disease (CAD) (1,2). Coronary CTA has a high negative predictive value, making it useful for excluding significant CAD and avoiding unnecessary invasive coronary angiography (3). However, the positive predictive value and specificity of coronary CTA are still insufficient, and one of the most important reasons might be the lack of spatial resolution (4,5). Imaging technology that improves the spatial resolution and diagnostic accuracy of coronary CTA is needed. Recently, ultra-high-resolution computed tomography (U-HRCT) scanners with a collimation of 160 rows × 0.25 mm have been introduced (6-9). The high spatial resolution of U-HRCT has been reported to provide better intraluminal visibility of coronary vessels, and the use of U-HRCT is expected to lead to improvement of the diagnostic capability of coronary CTA. However, the clinical use of U-HRCT is limited mainly by its narrow detector width of 40 mm (160 rows × 0.25 mm) and its high radiation dose (6,7). Balance between the spatial resolution and scan range is desirable.
Recently, 2nd generation deep learning-based reconstruction (DLR), which is a newly developed DLR algorithm that uses U-HRCT data as supervised data with further noise reduction, has been clinically introduced for conventional 320-row multidetector CT (MDCT) (10). The use of 2nd generation DLR is expected to improve image quality (e.g., vessel sharpness) with minimal image noise in the prospective ECG-gating coronary CTA scan at 320-row MDCT (11,12). We posit that 2nd generation DLR can potentially improve the diagnostic capability and widespread clinical use in CAD patient management; however, basic data on the effects of 2nd generation DLR on the vessel visualization in coronary CTA remain to be clarified with a vessel phantom of known true stenosis severity. Thus, the purpose of our phantom study using a coronary arterial model was to investigate the effects of 2nd generation DLR on the visibility of arterial stenosis by comparison with hybrid, model-based iterative reconstruction (MBIR), and prior DLR methods at 320-row coronary CTA.
Methods
Coronary vessel model and experiment
A vessel tube with a non-calcified plaque (Fuyo, Tokyo, Japan) was used for the coronary vessel model (13). The vessel-wall part of the phantom was made from machined acrylic pipe. The length and the lumen diameter of the coronary vessel model were 50.0 and 3.0 mm, respectively. Figure 1 shows a schematic diagram of a vessel model with stepwise non-calcified plaque. The degrees of stepped stenosis inside the lumen were 25%, 50%, and 75% (Figure 1). The plaque was composed of polystyrene, mono-cast nylon, and acrylonitrile butadiene styrene copolymer. The plaque component, which simulated a non-calcified fibrous plaque, was made with a CT number of 80 HU at 120-kVp scanning. Figure 2 shows a photograph of the experimental study. The vessel tube was filled with a diluted iodinated contrast medium (Omnipaque-300; GE Healthcare Pharma, Tokyo, Japan) to reach a target lumen CT number of approximately 400 HU. The coronary vessel models with and without a stent were fixed in a water-filled polypropylene cylinder-shaped container (diameter, 20 cm; height, 7 cm) (Figure 2). This study only utilized images from the non-stented model because of air contamination in the stented model.
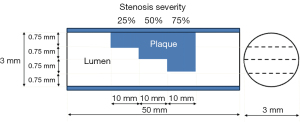
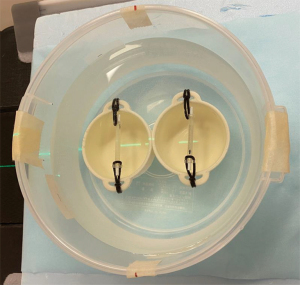
CT imaging and reconstruction
The coronary vessel model was examined using a 320-row MDCT scanner (Aquilion ONE Genesis edition; Canon Medical Systems, Otawara, Japan). The CT imaging was performed in the volume scanning mode. The data acquisition parameters were a detector collimation of 320 rows × 0.5 mm, a 275-ms tube rotation time, a tube voltage of 120 kVp, and a tube current of 210 mA. The CT scans were repeated three times. The images were reconstructed using the following four reconstructions: hybrid iterative reconstruction (HIR) (AIDR3De Standard; Canon Medical Systems), MBIR (FIRST Cardiac Standard; Canon Medical Systems), DLR (AiCE Cardiac Standard; Canon Medical Systems), and 2nd generation DLR (PIQE Cardiac Standard; Canon Medical Systems). HIR combines iterative reconstruction techniques with traditional filtered back projection (FBP). This method iteratively refines the image by reducing noise and artifacts, while preserving image quality (14). MBIR incorporates complex models of the imaging system, including the scanner geometry and physical processes such as photon statistics and detector characteristics. MBIR algorithms, so-called “fully iterative reconstruction”, iteratively optimize the image based on these models (15). DLR uses deep neural networks to reconstruct images. It is trained on large datasets of high-quality images, allowing the network to learn the optimal way to reconstruct images from raw data. DLR can provide superior noise reduction and detail preservation compared to traditional methods (16). The reconstruction kernel of FC43 was used. In all reconstructed images, the matrix size was 512×512. The display field of view (FOV) was 80 mm. The visible streak artifact was not identified on each CT image.
Quantitative analysis
Peak CT number and contrast-to-noise ratio (CNR)
The peak CT numbers in the vascular phantom lumen were obtained from the peak value of the profile curve in the lumen with 25%, 50%, and 75% stenoses using four types of reconstruction images. To avoid pixel variability, the peak CT numbers were measured using 21 images (3 scans × 7 slice levels) in each stenosis and the median value [interquartile range (IQR)] was calculated. Image noise, determined as the standard deviation of the CT number, was measured using the water in the background portion of the multiplanar reformation (MPR) image. The region of interest was set at 7.8 mm × 7.8 mm (50×50 pixels), and the median value was calculated from 63 images (3 scans × 7 slice levels × 3 stenoses). The CNR was then calculated as
Edge rise slope (ERS) analysis
To analyze the ERS, MPRs were conducted with a 0.5-mm slice thickness and a 0.3-mm slice interval. The MPR data were created using three repeated scans and four reconstruction algorithms. The rectangular region of interest was set at 2.3 mm × 9.4 mm (15×60 pixels) across the lumens with 25%, 50%, and 75% stenotic portions (Figure 3A). Subsequently, the luminal CT numbers of pixels, including the contrast medium and the plaque, were measured and a profile curve was obtained in each stenosis. The profile curve with luminal CT numbers was also normalized between 0 and 1 to determine the 10% and 90% luminal CT numbers (Figure 3B). The distance was calculated from the two x-coordinates corresponding to 10% and 90% luminal CT numbers of the profile curve. The 10–90% ERS was calculated using the following formula (17):
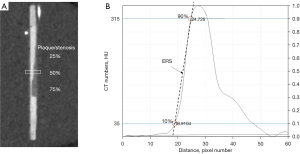
We acquired a total of 63 ERS scores, with 21 images for each stenosis degree (25%, 50%, and 75%) obtained from 3 scans and 7 slice levels, for each reconstruction technique. The median value (IQR) was calculated.
Percent stenosis measurement
The percent stenosis was measured in the lumen with 25%, 50%, and 75% stenoses using four types of reconstruction images on the MPR images. Two observers (board-certified radiologists with 20 years of experience and 14 years of experience in cardiovascular CT imaging, respectively) manually measured the diameter of the lumen of the phantom model independently because we considered the visual evaluation is common method in assessing coronary stenosis severity in clinical practice. Disagreements between the observers were resolved by consensus. The degree of the stenosis was calculated by the following formula, where L(0) was luminal diameter at no stenosis (0% stenosis), and L(stenosis) was luminal diameter at stenosed segment, e.g. L(25) was luminal diameter at 25% stenosed segment:
Visual evaluation
The qualitative analysis was performed using commercially available software (Ziostation2, version 2.9.7.1; Ziosoft, Tokyo, Japan). The window setting was fixed as a window level of 139 HU and a width of 600 HU. Two observers (board-certified radiologists with 20 years of experience and 14 years of experience in cardiovascular CT imaging, respectively) visually evaluated the image quality of the phantom model independently. Disagreements between the observers were resolved by consensus. We used a five-point scale for visual assessment of the following three items: graininess, lumen sharpness, and overall lumen visibility based on the degree of confidence. The visual scores were rated for 4 stenosis segments (0%, 25%, 50%, and 75% stenosis) under 4 reconstructions (HIR, MBIR, DLR, 2nd generation DLR).
The graininess was rated on the following scale for the presence of noise on the plaque portion: 5 = no or minimum noise with preserved lumen contour and natural texture; 4 = mild image noise; 3 = moderate image noise; 2 = severe image noise and unnatural texture that partially obscures the lumen contour; and 1 = unacceptable image noise that obscures the entire lumen. The sharpness of the plaque margins was assessed using the following scale: 5 = very clear margins with high spatial resolution; 4 = clear margins; 3 = acceptable sharpness of the margins; 2 = unclear margins; and 1 = blurred margin that cannot be identified. The overall lumen visibility according to the certainty for the stenosis severity was rated as follows: 5 = excellent certainty; 4 = good certainty; 3 = moderate certainty; 2 = low certainty; and 1 = poor certainty. That is, the method assigns a score of 5 if the area of 75% stenosis shows undoubtedly 75% stenosis.
Statistical analysis
We performed a normality test (Shapiro-Wilk test) on the peak CT number, CNR, ERS, percent stenosis results, and the qualitative assessment of the image quality (graininess, sharpness, and overall visibility) among the four reconstruction algorithms. Because a non-normal distribution was observed in some of the subjects, we conducted non-parametric tests (Kruskal-Wallis test), followed by post-hoc tests (Dunn-Bonferroni test). Additionally, we presented the central tendency using median values (IQR). Statistical significance was set at P<0.05. All statistical analyses were performed using the statistical software SPSS 28.0.1 (IBM, Armonk, NY, USA).
Results
Quantitative analysis
Peak CT number and CNR
The median peak CT number and CNR at different stenosis severities under the four reconstruction algorithms are shown in Table 1. Figure 4 shows box and whisker plots representing the median and IQR values of peak CT number for each reconstruction method. The median peak CT numbers with 2nd generation DLR were 357.7, 341.8, and 223.4 HU for 25%, 50%, and 75% stenosis, respectively. The median peak CT number at 75% stenosis was lower than those at 25% and 50% stenoses under each reconstruction method (Table 1). For 25% stenosis, the median peak CT number in the lumen was significantly different between 2nd generation DLR and MBIR, DLR and MBIR, as well as DLR and HIR. However, for 50% and 75% stenosis, no statistically significant differences were found among the four reconstructions (Figure 4).
Table 1
Percent stenosis | HIR | MBIR | DLR | 2nd generation DLR |
---|---|---|---|---|
25% plaque | ||||
Peak CT number (HU) | 342.4 (338.6–354.3) | 334.5 (325.3–341.0) | 365.3 (335.6–375.1) | 357.7 (347.0–365.4) |
CNR | 15.5 (14.7–16.1) | 15.9 (15.5–17.9) | 22.1 (20.6–23.5) | 38.3 (36.4–40.2) |
50% plaque | ||||
Peak CT number (HU) | 321.5 (301.3–339.5) | 319.3 (298.1–335.3) | 336.4 (312.3–352.4) | 341.8 (321.1–349.7) |
CNR | 14.4 (13.3–15.0) | 15.1 (14.5–16.9) | 20.4 (18.8–21.3) | 36.5 (34.6–38.2) |
75% plaque | ||||
Peak CT number (HU) | 209.5 (181.8–238.9) | 216.3 (181.4–237.5) | 210.9 (180.7–234.9) | 223.4 (185.3–247.7) |
CNR | 9.4 (8.2–10.5) | 10.2 (8.9–11.7) | 13.1 (10.5–14.3) | 24.3 (20.1–26.3) |
Background portion | ||||
Mean CT number (HU) | 0.6 | −1.3 | 0.1 | 0.4 |
Standard deviation (HU) | 23.0 | 21.0 | 16.9 | 9.5 |
The peak CT number and CNR values are presented as median (interquartile range). CT, computed tomography; CNR, contrast-to-noise ratio; DLR, deep learning-based reconstruction; HIR, hybrid iterative reconstruction; MBIR, model based iterative reconstruction.
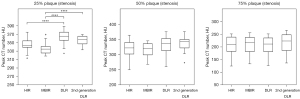
The image noise [standard deviation (SD) of the background (water portion)] was 23.0 HU with HIR, 21.0 HU with MBIR, 16.9 HU with DLR, and 9.5 HU with 2nd generation DLR. The image noise with 2nd generation DLR was substantially lower than that with the other reconstruction algorithms. Consequently, the 2nd generation DLR image showed the highest CNR (38.3, 36.5, and 24.3 for 25%, 50%, and 75% stenoses, respectively) (Table 1). Figure 5 shows box and whisker plots representing the median and IQR values of CNR for each reconstruction method. Among the four reconstruction algorithms, 2nd generation DLR achieved the highest CNR score (P<0.01 between HIR and 2nd generation DLR, P<0.01 between MBIR and 2nd generation DLR, P<0.01 between DLR and 2nd generation DLR) (Figure 5).
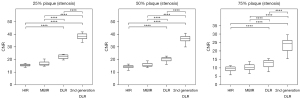
ERS
Figure 6 shows MPR images of a coronary vessel model reconstructed by 4 reconstruction techniques. Figure 7 shows magnifications of key features. Luminal contour and stepped stenosis (arrows) and plaque contour (arrowheads) are clearly depicted with substantially reduced noise in 2nd generation DLR compared with other reconstructions (Figure 7). Figure 8 shows the profile curve of the luminal CT number at 25%, 50%, and 75% stenoses, as obtained from HIR, MBIR, DLR, and 2nd generation DLR images. The 2nd generation DLR images show a steeper rise in the profile curve compared with the rises in the curves based on the other reconstruction images. The difference in the shape of the rising portion of the profile curve was pronounced in 25% and 50% stenoses. At any degree of stenosis, the 2nd generation DLR image showed the highest median ERS (262.4, 259.5, and 169.9 for 25%, 50%, and 75% stenoses, respectively) (Table 2). Figure 9 shows box and whisker plots of ERS median and IQR for each reconstruction method. Among the four reconstructions, significant differences were observed between the ERS values achieved with the 2nd generation DLR and HIR, with the 2nd generation DLR and MBIR methods at any degree of stenosis (P=0.03) (Figure 9).
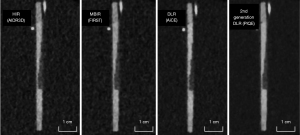
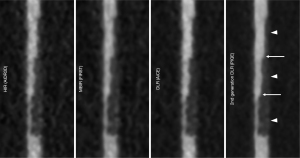
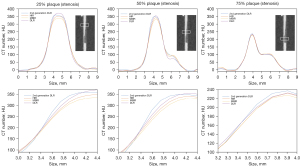
Table 2
Percent stenosis | ERS (HU/mm) | |||
---|---|---|---|---|
HIR | MBIR | DLR | 2nd generation DLR | |
25% plaque | 203.2 (168.5–218.9) | 198.6 (165.2–225.6) | 228.9 (196.1–245.8) | 262.4 (215.3–286.5) |
50% plaque | 174.5 (156.5–198.3) | 187.6 (157.4–218.3) | 206.3 (195.1–352.2) | 259.5 (215.6–300.1) |
75% plaque | 128.7 (93.2–144.1) | 132.6 (97.9–158.2) | 146.5 (115.6–165.3) | 169.9 (137.4–188.5) |
The ERS values are presented as median (interquartile range). ERS, edge rise slope; HIR, hybrid iterative reconstruction; MBIR, model based iterative reconstruction; DLR, deep learning-based reconstruction.
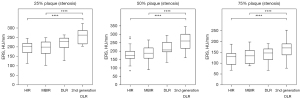
Percent stenosis measurement
Table 3 presents the mean (± SD) values of lumen diameter and percent stenosis at 25%, 50%, and 75% stenosis levels, derived from HIR, MBIR, DLR, and 2nd generation DLR images. Although the results indicated no statistically significant differences between the reconstruction methods, the percent stenosis on 2nd generation DLR images closely approximated the true percent stenosis, with mean (± SD) values of 26.4% (±3.8%), 49.9% (±5.2%), and 73.1% (±2.9%) for 25%, 50%, and 75% stenosis levels, respectively.
Table 3
HIR | MBIR | DLR | 2nd generation DLR | |
---|---|---|---|---|
25% plaque | ||||
Diameter (mm) | 1.9 (0.2) | 2.0 (0.2) | 2.0 (0.2) | 2.0 (0.1) |
Percent stenosis (%) | 27.7 (8.0) | 27.6 (4.3) | 27.1 (4.5) | 26.4 (3.8) |
50% plaque | ||||
Diameter | 1.3 (0.2) | 1.3 (0.2) | 1.4 (0.2) | 1.4 (0.1) |
Percent stenosis (%) | 52.3 (9.5) | 53.2 (6.8) | 49.7 (6.4) | 49.9 (5.2) |
75% plaque | ||||
Diameter | 0.7 (0.1) | 0.7 (0.1) | 0.8 (0.1) | 0.7 (0.1) |
Percent stenosis (%) | 72.5 (5.8) | 73.0 (3.8) | 70.6 (4.6) | 73.1 (2.9) |
The diameter and percent stenosis values are presented as mean (standard deviation). HIR, hybrid iterative reconstruction; MBIR, model based iterative reconstruction; DLR, deep learning-based reconstruction.
Visual assessment
Figure 10 shows a comparison of the visual scores (graininess, sharpness, and lumen visibility) under the four reconstructions. The score of 2nd generation DLR in all the evaluation points tended to be higher than the scores of HIR, MBIR, and DLR.
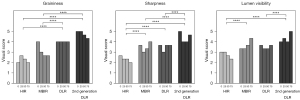
The mean graininess score was 2.3±0.5, 2.8±0.4, 4.0±0.0, and 4.8±0.5 for the HIR, MBIR, DLR, and 2nd generation DLR, respectively. Among the four reconstruction algorithms, 2nd generation DLR achieved the highest graininess score (P<0.01 between HIR and 2nd generation DLR, P<0.01 between MBIR and 2nd generation DLR), although the difference was not significant between DLR and 2nd generation DLR (P=0.62) (Figure 10).
The corresponding score for the lumen sharpness was 2.3±0.5, 3.5±0.5, 3.5±0.5, and 4.6±0.5. Among the four reconstruction algorithms, 2nd generation DLR achieved the highest sharpness score, and the difference was significant (P<0.01 between HIR and 2nd generation DLR, P=0.03 between MBIR and 2nd generation DLR, P=0.03 between DLR and 2nd generation DLR) (Figure 10).
The corresponding score for overall lumen visibility was 2.6±0.5, 3.8±0.6, 3.7±0.5, and 4.6±0.5. Among the four reconstruction algorithms, 2nd generation DLR achieved the highest visibility score (P<0.01 between HIR and 2nd generation DLR, P=0.01 between MBIR and 2nd generation DLR), although the difference was not significant between DLR and 2nd generation DLR (P=0.15) (Figure 10).
Discussion
We investigated the effects of a new coronary CTA reconstruction method (i.e., 2nd generation DLR) by comparing it with three other methods (HIR, MBIR, and DLR). Our findings are summarized as follows: (I) 2nd generation DLR showed the lowest image noise among the 4 reconstructions; (II) the CNR was highest in the 2nd generation DLR; (III) the percent stenosis index was different among the 4 reconstructions, and it might be most consistent with the true stenosis severity in the 2nd generation DLR; (IV) 2nd generation DLR resulted in the highest ERS, which was greater than that of the HIR method by approximately 25%; (V) 2nd generation DLR provided the best image quality in the subjective evaluation (graininess, sharpness, and overall image quality). These fundamental data suggest that the 2nd generation DLR method might contribute to improving the diagnostic accuracy of coronary CTA. The luminal CT number fluctuated according to the reconstruction techniques, but the difference was relatively small. The peak CT number was significantly higher for DLR and 2nd generation DLR in the 25% stenosis model, but it did not differ among four reconstructions in the 50% and 75% stenosis model. We posit that DLR and 2nd generation DLR might slightly increase the attenuation of contrast material. Larger amount of contrast material was filled in the vessel model of 25% stenosis, and the peak CT attenuation in the lumen was higher in the DLR and 2nd generation DLR than iterative reconstructions (HIR and MBIR). On the other hand, the amount of contrast material was smaller in 50% and 75% stenosis model, and the effect of DLR on the peak CT attenuation of contrast material might be also smaller than 25% stenosis model, resulting in no significant difference in the peak CT number among the four reconstructions for 50% and 75% stenosis model. The noise reduction of 2nd generation DLR was substantial compared with HIR, and the CNR of the 2nd generation DLR was more than twice the CNR value of the HIR.
FBP was previously used as a traditional technique for CT image reconstruction; however, IR replaced FBP over the past decade because of reduced image noise and preserved image quality even under low-dose CT scanning (2). However, a trade-off relationship exists between the image quality (e.g., spatial resolution and image texture) and noise reduction for both FBP and IR (2,18). A prior-generation deep learning-based image denoising technique was a clinically useful method that provided lower image noise than HIR in a short reconstruction time (approximately 1–2 min for coronary CTA) without degradation of the spatial resolution (19). Still, the trade-off relationship between image quality and noise reduction was present because the first-generation DLR was based on HIR and MBIR images (19). Our study results demonstrate that 2nd generation DLR improves the quantitative and qualitative image quality, i.e., CNR, spatial resolution, and the visual evaluation of graininess, sharpness, diagnostic confidence. Regarding peak CT number, all reconstruction methods indicated a trend in which higher stenosis severity correlated with reduced CT number. While, in theory, one would anticipate constant CT number within the lumen regardless of the stenosis degree, the observed fluctuations in CT number are also attributed to partial volume effects. Regarding percent stenosis, all reconstruction methods allowed relatively accurate measurements. On the other hand, 2nd generation DLR exhibited the smallest SD in the luminal diameter measurements, indicating higher measurement stability and increased confidence to judge the stenosis severity. We speculate that this may be related to the superior visual evaluation of overall lumen visibility based on the degree of confidence of 2nd generation DLR. We consider that the combined effects of the higher spatial resolution and effective denoising with relatively preserved luminal CT number of the contrast material of 2nd generation DLR led to the improved subjective vessel visibility and confidence about the vessel stenosis. Furthermore, significant differences in peak CT number were observed at a small stenosis degree (25% plaque) in this study. We hypothesize that small difference arose from obtaining the peak CT number from the profile curve instead of measuring it using the mean CT number.
With substantial technological advances, coronary CTA has become an indispensable diagnostic test in the management of ischemic heart disease (2), and the ACC/AHA Chest Pain Guidelines recommend coronary CTA as Class 1 with evidence level “A” for patients with stable and acute chest pain (1). Still, several problems related to relatively low specificity for the detection of obstructive CAD and overestimation of stenosis severity on coronary CTA remain unsolved (3). In addition, although inter-observer agreement in identifying CAD lesions is good, inter-observer variability remains problematic in evaluating the degree of stenosis and plaque burden on the coronary CT (20,21). These problems are speculated to be caused mainly by the insufficient spatial resolution and partial volume effects of CT. In addition, a previous study regarding inter-scanner agreement for evaluating coronary plaque in serial coronary CTA scans (22) demonstrated that scanner variability increased with different-vendor follow-up. We posit that the characteristics and performance of CTA reconstruction algorithms among different vendors might affect the variability of coronary CTA evaluations. In the present study, the diameter of the vessel phantom (cylinder) was 3 mm, and we considered that the shortage of the pixels might affect the accuracy of the radial edge method of the modulation transfer function (MTF) (23). Therefore, we adopted the ERS as a quantitative parameter for the evaluation of the resolution. Our initial results indicate that the 2nd generation DLR might have the potential to estimate CAD severity and achieve higher inter-observer agreement more accurately. Further studies are underway in our laboratory to verify the effects of 2nd generation DLR on calcified plaque and stent phantoms and in clinical practice. Current 2nd generation DLR can produce 512×512-matrix images alone, although 1,024×1,024 matrix may be desirable for 2nd generation DLR. The limiting spatial resolution of current 2nd generation DLR (512×512 matrix) is 0.3 mm. Development of 1,024×1,024-matrix 2nd generation DLR is underway in the vendor (Canon Medical Systems), and it may be clinically introduced in the future, providing 0.15-mm limiting spatial resolution. U-HRCT reportedly provides new possibilities for the diagnosis of highly calcified coronary arteries and small in-stent restenosis (6,7,9). It has been reported that 2nd generation DLR improves the image quality of coronary arteries and in-stent lumen in coronary CTA compared to conventional MBIR (12). The influence of 2nd generation DLR on the blooming and beam-hardening artifacts and the lumen visibility of coronary stents and calcified lesions should be investigated.
The presence of high-risk plaque on coronary CTA increases the likelihood of ACS independent of significant stenosis and clinical risk assessment (24,25). Therefore, a coronary artery disease reporting and data system (CAD-RADS) (i.e., a standardized reporting system) is important for evaluating high-risk plaques as one of the modifiers added to the degree of stenosis on coronary CTA (26). Low attenuation plaque is one of the characteristics of high risk, and the measurement of an accurate CT number is necessary. Our results show that the 2nd generation DLR provides not only effective denoising and higher image quality but also preserves the accuracy of the peak CT number measurement in 50% and 75% stenosis segments, although peak CT number fluctuated slightly (approximately 30 HU or less) for 25% stenosis segment. There was a tendency for the peak CT number to decrease as the stenosis severity increased across all reconstruction methods. This phenomenon is believed to be influenced significantly by the partial volume effect. We speculate that our result of improved confidence in vessel stenosis is attributable to improved visualization of the plaque portion as well as the vessel lumen. Recently, the concept of myocardial infarction with nonobstructive coronary artery (MINOCA) disease has been proposed (27-29), and evaluations of coronary artery plaque rupture, erosion, dissection, and thrombus are critical to diagnose MINOCA. Therefore, improving the quality of coronary CTA is desirable to enable an accurate evaluation of coronary plaque morphology and possibly diagnose MINOCA noninvasively. The 2nd generation DLR might provide additional information for plaque characterization, although further studies are necessary.
Several study limitations should be acknowledged. First, our phantom study has small sample size; thus, a large-scale clinical study is needed to confirm our preliminary results. Second, 2nd generation DLR was trained using patient data. However, the present study was performed using a vessel phantom, and therefore the study results might be affected by the different behavior of the algorithm between human and phantom subjects. Third, the coronary artery model was not placed in a dynamic cardiac phantom. The cardiac motion might affect the results, and a further evaluation using a dynamic phantom, as used in a previous study might be desirable (30). Fourth, we evaluated the straight and 3-mm lumen of the coronary arterial model alone. The relationship between 2nd generation DLR images and the shape and size of the vessels should be clarified in future clinical studies. Fifth, we evaluated only low attenuation plaque. Further studies are underway in our laboratory to verify the influence of 2nd generation DLR on blooming and beam-hardening artifacts and the lumen visibility of coronary stents and calcified lesions. Sixth, percent stenosis was measured through manual assessments. Estimating stenosis visually based on the image’s appearance carries the risk of introducing bias. Similarly, visual evaluation of image quality was also vulnerable to bias. It was difficult to rigorously blind the observers to peculiar impressions and texture produced by the CT images according to the reconstruction methods. Seventh, our study did not evaluate reconstruction time and the potential for radiation dose reduction, but a previous study reported that the reconstruction time was significantly shorter than MBIR (12). We posit that the reconstruction time of 2nd generation DLR might be acceptable in clinical practice. Further clinical studies are imperative to investigate the effects of 2nd generation DLR on the radiation dose reduction.
Conclusions
In conclusion, the present experimental study demonstrated that, compared with HIR, MBIR, and prior-generation DLR, 2nd generation DLR can improve CNR and ERS in coronary CTA images, resulting in the best subjective image quality for evaluating coronary vessel stenosis.
Acknowledgments
Funding: None.
Footnote
Conflicts of Interest: All authors have completed the ICMJE uniform disclosure form (available at https://qims.amegroups.com/article/view/10.21037/qims-23-1204/coif). D.U. reports that he has received research grants from Canon Medical Systems paid to Department of Diagnostic Radiology at Yokohama City University Graduate School of Medicine. T.T. reports that he is an employee of Canon Medical Systems Corporation. The other authors have no conflicts of interest to declare.
Ethical Statement: The authors are accountable for all aspects of the work in ensuring that questions related to the accuracy or integrity of any part of the work are appropriately investigated and resolved.
Open Access Statement: This is an Open Access article distributed in accordance with the Creative Commons Attribution-NonCommercial-NoDerivs 4.0 International License (CC BY-NC-ND 4.0), which permits the non-commercial replication and distribution of the article with the strict proviso that no changes or edits are made and the original work is properly cited (including links to both the formal publication through the relevant DOI and the license). See: https://creativecommons.org/licenses/by-nc-nd/4.0/.
References
- Gulati M, Levy PD, Mukherjee D, Amsterdam E, Bhatt DL, et al. 2021 AHA/ACC/ASE/CHEST/SAEM/SCCT/SCMR Guideline for the Evaluation and Diagnosis of Chest Pain: A Report of the American College of Cardiology/American Heart Association Joint Committee on Clinical Practice Guidelines. J Cardiovasc Comput Tomogr 2022;16:54-122. [Crossref] [PubMed]
- Al Rifai M, Ahmed AI, Alahdab F, Al-Mallah MH. Clinical utility of coronary artery computed tomography angiography- What we know and What's new? Prog Cardiovasc Dis 2022;75:12-20. [Crossref] [PubMed]
- Li S, Ni Q, Wu H, Peng L, Dong R, Chen L, Liu J. Diagnostic accuracy of 320-slice computed tomography angiography for detection of coronary artery stenosis: meta-analysis. Int J Cardiol 2013;168:2699-705. [Crossref] [PubMed]
- Raff GL, Gallagher MJ, O'Neill WW, Goldstein JA. Diagnostic accuracy of noninvasive coronary angiography using 64-slice spiral computed tomography. J Am Coll Cardiol 2005;46:552-7. [Crossref] [PubMed]
- Nikolaou K, Knez A, Rist C, Wintersperger BJ, Leber A, Johnson T, Reiser MF, Becker CR. Accuracy of 64-MDCT in the diagnosis of ischemic heart disease. AJR Am J Roentgenol 2006;187:111-7. [Crossref] [PubMed]
- Takagi H, Tanaka R, Nagata K, Ninomiya R, Arakita K, Schuijf JD, Yoshioka K. Diagnostic performance of coronary CT angiography with ultra-high-resolution CT: Comparison with invasive coronary angiography. Eur J Radiol 2018;101:30-7. [Crossref] [PubMed]
- Motoyama S, Ito H, Sarai M, Nagahara Y, Miyajima K, Matsumoto R, Doi Y, Kataoka Y, Takahashi H, Ozaki Y, Toyama H, Katada K. Ultra-High-Resolution Computed Tomography Angiography for Assessment of Coronary Artery Stenosis. Circ J 2018;82:1844-51. [Crossref] [PubMed]
- Iwasawa T, Sato M, Yamaya T, Sato Y, Uchida Y, Kitamura H, Hagiwara E, Komatsu S, Utsunomiya D, Ogura T. Ultra-high-resolution computed tomography can demonstrate alveolar collapse in novel coronavirus (COVID-19) pneumonia. Jpn J Radiol 2020;38:394-8. [Crossref] [PubMed]
- Latina J, Shabani M, Kapoor K, Whelton SP, Trost JC, Sesso J, Demehri S, Mahesh M, Lima JAC, Arbab-Zadeh A. Ultra-High-Resolution Coronary CT Angiography for Assessment of Patients with Severe Coronary Artery Calcification: Initial Experience. Radiol Cardiothorac Imaging 2021;3:e210053. [Crossref] [PubMed]
- Tatsugami F, Nakaura T, Yanagawa M, Fujita S, Kamagata K, Ito R, Kawamura M, Fushimi Y, Ueda D, Matsui Y, Yamada A, Fujima N, Fujioka T, Nozaki T, Tsuboyama T, Hirata K, Naganawa S. Recent advances in artificial intelligence for cardiac CT: Enhancing diagnosis and prognosis prediction. Diagn Interv Imaging 2023; Epub ahead of print. [Crossref]
- Hernandez AM, Shin DW, Abbey CK, Seibert JA, Akino N, Goto T, Vaishnav JY, Boedeker KL, Boone JM. Validation of synthesized normal-resolution image data generated from high-resolution acquisitions on a commercial CT scanner. Med Phys 2020;47:4775-85. [Crossref] [PubMed]
- Orii M, Sone M, Osaki T, Ueyama Y, Chiba T, Sasaki T, Yoshioka K. Super-resolution deep learning reconstruction at coronary computed tomography angiography to evaluate the coronary arteries and in-stent lumen: an initial experience. BMC Med Imaging 2023;23:171. [Crossref] [PubMed]
- Funama Y, Utsunomiya D, Hirata K, Taguchi K, Nakaura T, Oda S, Kidoh M, Yuki H, Yamashita Y. Improved Estimation of Coronary Plaque and Luminal Attenuation Using a Vendor-specific Model-based Iterative Reconstruction Algorithm in Contrast-enhanced CT Coronary Angiography. Acad Radiol 2017;24:1070-8. [Crossref] [PubMed]
- Hou Y, Liu X, Xv S, Guo W, Guo Q. Comparisons of image quality and radiation dose between iterative reconstruction and filtered back projection reconstruction algorithms in 256-MDCT coronary angiography. AJR Am J Roentgenol 2012;199:588-94. [Crossref] [PubMed]
- Lee S, Shima A, Singh S, Kalra M, Kim HJ, Do S. Co-registered image quality comparison in hybrid iterative reconstruction techniques: SAFIRE and SafeCT. SPIE Medical Imaging, SPIE; 2013.
- Jeon PH, Jeon SH, Ko D, An G, Shim H, Otgonbaatar C, Son K, Kim D, Ko SM, Chung MA. Assessment of Image Quality of Coronary CT Angiography Using Deep Learning-Based CT Reconstruction: Phantom and Patient Studies. Diagnostics (Basel) 2023;13:1862. [Crossref] [PubMed]
- Tatsugami F, Higaki T, Sakane H, Fukumoto W, Kaichi Y, Iida M, Baba Y, Kiguchi M, Kihara Y, Tsushima S, Awai K. Coronary Artery Stent Evaluation with Model-based Iterative Reconstruction at Coronary CT Angiography. Acad Radiol 2017;24:975-81. [Crossref] [PubMed]
- Hirata K, Utsunomiya D, Kidoh M, Funama Y, Oda S, Yuki H, Nagayama Y, Iyama Y, Nakaura T, Sakabe D, Tsujita K, Yamashita Y. Tradeoff between noise reduction and inartificial visualization in a model-based iterative reconstruction algorithm on coronary computed tomography angiography. Medicine (Baltimore) 2018;97:e10810. [Crossref] [PubMed]
- Higaki T, Nakamura Y, Tatsugami F, Nakaura T, Awai K. Improvement of image quality at CT and MRI using deep learning. Jpn J Radiol 2019;37:73-80. [Crossref] [PubMed]
- Leber AW, Becker A, Knez A, von Ziegler F, Sirol M, Nikolaou K, Ohnesorge B, Fayad ZA, Becker CR, Reiser M, Steinbeck G, Boekstegers P. Accuracy of 64-slice computed tomography to classify and quantify plaque volumes in the proximal coronary system: a comparative study using intravascular ultrasound. J Am Coll Cardiol 2006;47:672-7. [Crossref] [PubMed]
- Maroules CD, Hamilton-Craig C, Branch K, Lee J, Cury RC, Maurovich-Horvat P, Rubinshtein R, Thomas D, Williams M, Guo Y, Cury RC. Coronary artery disease reporting and data system (CAD-RADS(TM)): Inter-observer agreement for assessment categories and modifiers. J Cardiovasc Comput Tomogr 2018;12:125-30. [Crossref] [PubMed]
- Symons R, Morris JZ, Wu CO, Pourmorteza A, Ahlman MA, Lima JA, Chen MY, Mallek M, Sandfort V, Bluemke DA, Coronary CT. Angiography: Variability of CT Scanners and Readers in Measurement of Plaque Volume. Radiology 2016;281:737-48. [Crossref] [PubMed]
- Takenaga T, Katsuragawa S, Goto M, Hatemura M, Uchiyama Y, Shiraishi J. Modulation transfer function measurement of CT images by use of a circular edge method with a logistic curve-fitting technique. Radiol Phys Technol 2015;8:53-9. [Crossref] [PubMed]
- Otsuka K, Fukuda S, Tanaka A, Nakanishi K, Taguchi H, Yoshiyama M, Shimada K, Yoshikawa J. Prognosis of vulnerable plaque on computed tomographic coronary angiography with normal myocardial perfusion image. Eur Heart J Cardiovasc Imaging 2014;15:332-40. [Crossref] [PubMed]
- Puchner SB, Liu T, Mayrhofer T, Truong QA, Lee H, Fleg JL, Nagurney JT, Udelson JE, Hoffmann U, Ferencik M. High-risk plaque detected on coronary CT angiography predicts acute coronary syndromes independent of significant stenosis in acute chest pain: results from the ROMICAT-II trial. J Am Coll Cardiol 2014;64:684-92. [Crossref] [PubMed]
- Cury RC, Leipsic J, Abbara S, Achenbach S, Berman D, Bittencourt M, Budoff M, Chinnaiyan K, Choi AD, Ghoshhajra B, Jacobs J, Koweek L, Lesser J, Maroules C, Rubin GD, Rybicki FJ, Shaw LJ, Williams MC, Williamson E, White CS, Villines TC, Blankstein R. CAD-RADS™ 2.0 - 2022 Coronary Artery Disease - Reporting and Data System An Expert Consensus Document of the Society of Cardiovascular Computed Tomography (SCCT), the American College of Cardiology (ACC), the American College of Radiology (ACR) and the North America Society of Cardiovascular Imaging (NASCI). Radiol Cardiothorac Imaging 2022;4:e220183. [Crossref] [PubMed]
- Del Buono MG, Montone RA, Camilli M, Carbone S, Narula J, Lavie CJ, Niccoli G, Crea F. Coronary Microvascular Dysfunction Across the Spectrum of Cardiovascular Diseases: JACC State-of-the-Art Review. J Am Coll Cardiol 2021;78:1352-71. [Crossref] [PubMed]
- Reynolds HR, Maehara A, Kwong RY, Sedlak T, Saw J, Smilowitz NR, et al. Coronary Optical Coherence Tomography and Cardiac Magnetic Resonance Imaging to Determine Underlying Causes of Myocardial Infarction With Nonobstructive Coronary Arteries in Women. Circulation 2021;143:624-40. [Crossref] [PubMed]
- Singh T, Chapman AR, Dweck MR, Mills NL, Newby DE. MINOCA: a heterogenous group of conditions associated with myocardial damage. Heart 2021;107:1458-64. [Crossref] [PubMed]
- Kidoh M, Utsunomiya D, Funama Y, Sakabe D, Oda S, Nakaura T, Yuki H, Nagayama Y, Hirata K, Iyama Y, Namimoto T, Yamashita Y. The effect of heart rate on coronary plaque measurements in 320-row coronary CT angiography. Int J Cardiovasc Imaging 2018;34:1977-85. [Crossref] [PubMed]