Association of epicardial fat volume with subclinical myocardial damage in patients with type 2 diabetes mellitus
Introduction
Based on the data provided by the International Diabetes Federation (IDF), the worldwide occurrence of type 2 diabetes mellitus (T2DM) in individuals aged 20 to 79 years was estimated to be 10.5% (536.6 million individuals) in the year 2021 (1). Diabetic cardiomyopathy, a major diabetic cardiovascular complication, is the leading cause of morbidity in T2DM, characterized by systolic function impairment and difficulties in early diagnosis (2). Left ventricular ejection fraction (LVEF) is the most common clinical parameter for quantifying global ventricular systolic function. However, due to the compensatory mechanism, at the initial stages of diabetic cardiomyopathy, the LVEF could be preserved (3). A study showed that in the early stage, diabetic cardiomyopathy patients had normal myocardial perfusion, with alterations in mitochondrial, myocardial, and metabolic, and function, whereas the abnormality of myocardial perfusion and LVEF eventually appeared in the late stage (4). Our previous study found that left ventricular systolic dyssynchrony (LVSD) was present among 21.1% of individuals with T2DM who exhibited normal LVEF and normal myocardial perfusion, which is also known as subclinical myocardial damage, and need early intervention to improve poor prognosis. Besides, we also found that overweight is closely correlated with subclinical myocardial damage (5).
LVSD refers to losing synchronicity of mechanical contraction in different segments of the left ventricle (6). Several imaging techniques can be used to assess left ventricle synchronization, including single photon emission computed tomography-computed tomography (SPECT-CT), cardiac magnetic resonance imaging (MRI), and echocardiography. MRI is expensive and involves intricate operations. In addition, some patients with metal implants are contraindicated for MRI. Echocardiography is operator dependent and may have low accuracy in patients with a poor acoustic window. SPECT-CT is a widely used, cost-effective, non-invasive imaging modality that facilitates simultaneous evaluation of myocardial perfusion and left ventricular systolic synchrony with good repeatability. LVSD significantly contributes to both the pathologic process of heart failure (HF) and ventricular remodeling (7,8).
Epicardial adipose tissue (EAT) is the visceral fat depot of the heart, mainly surrounding major epicardial coronary arteries or the myocardium, which can be quantitatively assessed according to epicardial fat volume (EFV) by SPECT-CT and has been considered as a promising risk indicator for cardiovascular disease (9). Studies have shown a significant association between EFV and various cardiovascular conditions, including atherosclerosis, coronary artery disease, HF, and atrial fibrillation (10-12). A study on adults with uncomplicated obesity found that visceral adipose tissue was independently associated with left ventricular global longitudinal systolic strain (GLS), a marker of early subclinical left ventricular dysfunction (13). Besides, a transformation in lifestyle habits or medicinal interventions (statins or dapaglifozin) can proficiently mitigate the accumulation of EFV (14), which highlights the potentiality of EAT as a therapeutic target. However, the relationship between EAT and subclinical myocardial impairment among T2DM patients remains uncertain.
Thus, the aim of this study was to explore the correlation between EFV and LVSD in T2DM patients with normal LVEF and normal myocardial perfusion, which may be a new imaging marker and potential therapeutic target for early evaluating subclinical myocardial damage. We present this article in accordance with the STROBE reporting checklist (available at https://qims.amegroups.com/article/view/10.21037/qims-23-1413/rc).
Methods
Study population
This was a retrospective study that enrolled T2DM patients who underwent stress-rest gated SPECT-myocardial perfusion imaging (MPI) at The Third Affiliated Hospital of Soochow University from January 2018 to December 2022. The exclusion criteria were as follows: (I) history of coronary revascularization, history of acute coronary syndrome, and coronary artery disease confirmed by coronary angiography. (II) Severe valvular heart disease or severe arrhythmia. (III) History of malignant tumors, (IV) abnormal renal or hepatic function, (V) hypertrophic cardiomyopathy and other cardiomyopathies, (VI) LVEF <50%. (VII) Abnormal MPI by SPECT-CT, (VIII) poor image quality (15). Finally, 117 patients with T2DM with normal LVEF and normal MPI were enrolled for analysis. Additionally, to obtain the reference threshold of LVSD, we also included 83 individuals as a control group who were matched by age and gender and did not have diabetes or cardiovascular disease. Figure 1 displays the study flowchart. The study was approved by the Ethics Committee of The Third Affiliated Hospital of Soochow University (No. 2023-062) and was conducted according to the Declaration of Helsinki (as revised in 2013). The requirement for informed consent was waived due to the retrospective nature of the study.
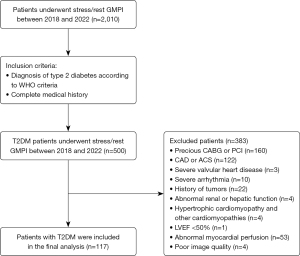
Clinical data
Clinical and laboratory data were extracted retrospectively from medical records, including age, gender, height, weight, fasting blood glucose (FBG), glycosylated hemoglobin (HbA1c), triglycerides (TG), total cholesterol (TC), low-density lipoprotein (LDL)-cholesterol, high-density lipoprotein (HDL)-cholesterol, smoking status, alcohol intake, and medications (including statin therapy and antidiabetic medications). Body mass index (BMI) was calculated as weight in kilograms divided by the square of height in meters. Hypertension was defined as blood pressure (BP) ≥140/90 mmHg or the use of antihypertensive drugs. Hyperlipidemia was defined as TC ≥220 mg/dL, TG ≥150 mg/dL, or history of treatment for hyperlipidemia. Active smoking was defined as the act of currently smoking or having smoked within the past year. Active drinking referred to drinking alcohol at least once a week and lasting for more than 6 months. In addition, we estimated LVEF by echocardiography. All patients underwent echocardiography within 1 month before or after MPI, and the LVEF was evaluated using the modified Simpson method on 2-dimensional echocardiography.
Gated SPECT-MPI acquisition and analysis
Patients underwent a stress/rest protocol using 99mTc-sestamibi (99mTc-MIBI). Stress testing included exercise stress MPI based on the Bruce protocol, or pharmacological stress MPI with adenosine intravenously (i.v.) infused at 0.14 mg/kg/min for 6 minutes. After being injected with 740–925 MBq of 99mTc-MIBI, the patients were scanned with a 2-detector 90° SPECT-CT camera (Symbia T16; Siemens Medical Systems, Erlangen, Germany). Electrocardiogram (ECG)-gated SPECT imaging was performed according to standards of the American Society of Nuclear Cardiology (16). A total of eight frames were obtained in each cardiac cycle by an ECG R-wave trigger. Images were acquired by using a low-energy, high-spatial-resolution parallel hole collimator, with a 128×128 matrix by using a magnification of 1.45, with a 20% window centered around a 140 keV photo peak. The dual detectors rotated 90° each, collectively covering 180°, with an angle step of 6° and 35 seconds at each step. After reconstruction, the horizontal long-axis, vertical long-axis, and short-axis images were obtained. For semiquantitative assessment of myocardial perfusion abnormality, 17-segment visual interpretation of myocardial perfusion images was performed by 2 experienced nuclear physicians using a standard 5-point scoring system (0: normal to 4: absence of tracer uptake). The summed stress score (SSS), summed rest score (SRS), and summed difference score (SDS) were calculated. SSS ≥4 or SDS ≥2 were considered as abnormal (17).
Synchronicity assessment
Automatic phase analysis (ECTb SyncTool version 4.0; Emory University/Syntermed, Atlanta, GA, USA) using raw images of rest gated-MPI was performed for the evaluation of LVSD. Phase standard deviation (PSD; unit: degree) and phase histogram bandwidth (PBW; unit: degree) are two quantitative indices to assess LVSD (18). Based on previous research, LVSD was defined as above the mean + 2 standard deviations (2SDs) of PSD or PBW obtained (19). The mean values of PSD and PBW in the control cohort were 8.74°±2.18° and 8.74°±2.18°, respectively. LVSD is defined as PSD >13.1° or PBW >37.6°.
Measurement of EFV
As the “one-stop shop” examination, SPECT-CT could quantitatively assess EFV through non-contrast CT after performing rest MPI. The CT scan parameters included a tube current of 100 mA, a voltage of 130 kV, and a slice thickness of 3 mm. EFV was quantified with the volume tool (Syngo Volume, Siemens Healthineers, Erlangen, Germany) and was measured using a semi-automatic segmentation technique on every axial slice from the pulmonary artery bifurcation to the diaphragmatic surface of heart. The CT value of −190 to −30 HU is set for adipose tissue (20). The volume of fat (cm3) selected is automatically calculated.
Statistical analysis
The statistical analyses were carried out through SPSS 25.0 (IBM Corp., Armonk, NY, USA) and R3.4.3 (software packages: glmnet, pROC, rms, and dca; R Foundation for Statistical Computing, Vienna, Austria). Data conforming to a normal distribution were expressed as mean ± SD. Data not conforming to a normal distribution were expressed as median and interquartile range. Normal distribution of data in each group was tested by Shapiro-Wilk test. Continuous variables were compared by using either independent-sample t-test or Mann-Whitney U test according to normality. Categorical variables were compared using the Pearson’s chi-square test. T2DM patients were divided into tertiles according to their EFV levels. Generalized additive model (GAM) and cubic spline smoothing technique were performed to evaluate the association between EFV and LVSD in T2DM patients. Multivariable logistic regression models were produced to assess the association between EFV and LVSD; three models were generated: (I) a univariable model with EFV as a predictor; (II) multivariable-adjusted model 1, adjusted for age and sex; (III) multivariable-adjusted model 2 was constructed based on our team’s interpretation of a broad review of the literature, and adjusted for age, sex, BMI, DM duration, presence of hypertension, active smoking, FBG, HbA1c, and TG (19,21,22). Receiver operating characteristic (ROC) curve analysis was performed to determine the predictive value of EFV for LVSD. All P values were 2-sided, and P values <0.05 were considered statistically significant.
Results
Comparison of baseline characteristics between T2DM patients with LVSD and the non-LVSD group
Baseline characteristics of the T2DM patients (n=117) are shown in Table 1. A total of 32 (27.4%) T2DM patients had LVSD. Compared with non-LVSD group, the BMI and EFV were higher in patients with LVSD (25.83±2.66 vs. 23.94±3.13 kg/m2; 142.41±44.17 vs. 108.01±38.24 cm3, respectively, both P<0.05). There were no statistical differences between the LVSD and non-LVSD groups in age, gender, T2DM duration, smoking, alcohol intaking, hypertension, hyperlipidemia history, FBG, HbA1c, lipid profile, LVEF, and the use of statins and hypoglycemic agents (all P>0.05).
Table 1
Characteristics | LVSD group (n=32) | Non-LVSD group (n=85) | P value |
---|---|---|---|
Clinical characteristics | |||
Age (years) | 57.38±7.56 | 56.28±9.19 | 0.549 |
Male | 23 (71.8) | 45 (52.9) | 0.064 |
Body mass index (kg/m2) | 25.83±2.66 | 23.94±3.13 | 0.003* |
DM duration (years) | 8 [1.3, 10.0] | 6 [1.5, 11.0] | 0.606 |
Hypertension | 11 (34.4) | 22 (25.9) | 0.344 |
Active smoking | 10 (31.3) | 21 (24.7) | 0.475 |
Active drinking | 10 (31.3) | 14 (16.5) | 0.078 |
FBG (mmol/L) | 8.35 [6.42, 12.29] | 7.79 [6.25, 11.45] | 0.106 |
HbA1c (%) | 8.7 [7.1, 11.3] | 8.5 [7, 11.3] | 0.326 |
TG (mmol/L) | 2.07 [1.48, 2.87] | 1.77 [1.20, 2.40] | 0.059 |
TC (mmol/L) | 4.72±1.44 | 4.78±1.03 | 0.812 |
HDL-cholesterol (mmol/L) | 0.98 [0.86, 1.11] | 0.98 [0.88, 1.25] | 0.191 |
LDL-cholesterol (mmol/L) | 2.53±0.83 | 2.74±0.74 | 0.219 |
Hyperlipidemia | 21 (65.6) | 49 (57.6) | 0.433 |
Medications | |||
Statin | 14 (43.8) | 32 (37.6) | 0.547 |
Oral drug | 25 (78.1) | 62 (72.9) | 0.567 |
Insulin | 10 (31.3) | 21 (24.7) | 0.475 |
LVEF (%) | 64.1±2.5 | 63.1±3.2 | 0.136 |
EFV (cm3) | 142.41±44.17 | 108.01±38.24 | <0.001* |
Data are given as mean ± standard deviation (normal distribution), median [Q1 to Q3] (non-normal distribution), or number (%) for categorical variables. *, P<0.05. T2DM, type 2 diabetes mellitus; MPI, myocardial perfusion imaging; LVEF, left ventricular ejection fraction; LVSD, left ventricular systolic dyssynchrony; DM, diabetes mellitus; FBG, fasting blood glucose; HbA1c, glycosylated hemoglobin; TG, triglycerides; TC, total cholesterol; HDL, high-density lipoprotein; LDL, low-density lipoprotein; EFV, epicardial fat volume.
Table 2 demonstrates the influence factors of EFV. Age, BMI, hypertension, and proportion of LVSD increased with tertiles of EFV (all P-trend <0.05). The proportions of LVSD in the EFV of low, middle, and high were 7.7%, 28.2%, and 46.2%, respectively (P<0.001). A statistical analysis of the comparison between the normal group and the diabetes group is presented in Table S1. Figure 2 displays the case example.
Table 2
Characteristics | Bottom | Middle | Top | P for trend |
---|---|---|---|---|
EFV (cm3) | 42.19–96.23 | 96.52–131.89 | 132.07–240.53 | – |
EFV, mean (cm3) | 73.34±16.81 | 114.71±9.91 | 164.22±30.31 | – |
EFV, median (cm3) | 78.60 [58.22, 88.20] | 115.80 [105.90, 122.29] | 153.53 [140.0, 179.89] | – |
Age (years) | 55.1±10.2 | 54.1±6.3 | 60.6±8.1 | 0.005* |
Male | 20 (51.3) | 24 (61.5) | 24 (61.5) | 0.360 |
Body mass index (kg/m2) | 23±3.3 | 24.7±2.5 | 25.7±2.9 | <0.001* |
DM duration (years) | 8 [2, 13] | 5 [2, 10] | 5 [1, 12] | 0.403 |
Hypertension | 6 (15.4) | 11 (28.2) | 16 (41.0) | 0.014* |
Active smoking | 9 (23.1) | 11 (28.2) | 10 (25.6) | 0.780 |
Active drinking | 6 (15.4) | 8 (20.5) | 10 (25.6) | 0.265 |
FBG (mmol/L) | 9.52 [6.47, 13.17] | 7.59 [6.04, 11.31] | 8.48 [6.6, 12.3] | 0.589 |
HbA1c (%) | 9.7 [7.6, 12.0] | 8.1 [6.8, 9.8] | 8.2 [7.1, 11.6] | 0.261 |
TG (mmol/L) | 1.96 [1.29, 2.73] | 1.81 [1.22, 2.57] | 1.76 [1.36, 2.15] | 0.200 |
TC (mmol/L) | 4.76 [4.21, 5.43] | 4.74 [4.08, 5.21] | 4.48 [3.80, 5.70] | 0.798 |
HDL-cholesterol (mmol/L) | 0.96 [0.88, 1.19] | 1.05 [0.90, 1.26] | 0.95 [0.83, 1.10] | 0.483 |
LDL-cholesterol (mmol/L) | 2.82±0.76 | 2.69±0.77 | 2.55±0.78 | 0.126 |
Hyperlipidemia | 25 (64.1) | 19 (48.7) | 22 (56.4) | 0.494 |
Statin | 17 (43.6) | 15 (38.5) | 14 (35.9) | 0.487 |
Oral drug | 29 (74.4) | 26 (66.7) | 32 (82.1) | 0.438 |
Insulin | 11 (28.2) | 9 (23.1) | 11 (28.2) | >0.999 |
LVSD (%) | 3 (7.7) | 11 (28.2) | 18 (46.2) | <0.001* |
Data are given as mean ± standard deviation (normal distribution), median [Q1 to Q3] (non-normal distribution), or number (%) for categorical variables. *, P<0.05. EFV, epicardial fat volume; DM, diabetes mellitus; FBG, fasting blood glucose; HbA1c, glycosylated hemoglobin; TG, triglycerides; TC, total cholesterol; HDL, high-density lipoprotein; LDL, low-density lipoprotein; LVSD, left ventricular systolic dyssynchrony.
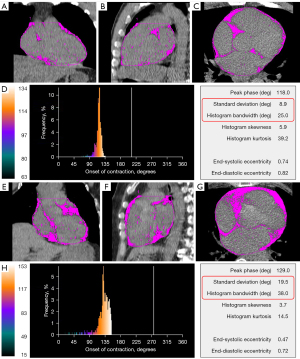
Univariate and multivariate logistic regression analysis
In univariate logistic regression, BMI and EFV were associated with LVSD [odds ratio (OR) =1.22, 95% confidence interval (CI): 1.06–1.41, P=0.005; OR =1.22, 95% CI: 1.10–1.37, P<0.001, respectively]. In multivariate logistic regression, EFV was the only independent risk factor of LVSD (OR =1.19; 95% CI: 1.06–1.34; P=0.003) (Table 3). Table 4 shows the results of the multivariable logistic regression analysis. In multivariable-adjusted model 1, the probability of LVSD increased by 24% for every 10 cm3 increase in EFV. In multivariable-adjusted model 2, the probability of LVSD increased by 20% for every 10 cm3 increase in EFV.
Table 3
Characteristics | Univariate model | Multivariate model | |||||
---|---|---|---|---|---|---|---|
OR | 95% CI | P value | OR | 95% CI | P value | ||
Age | 1.01 | 0.97–1.06 | 0.546 | – | – | – | |
Male | 2.27 | 0.94–5.48 | 0.066 | – | – | – | |
DM duration | 1.01 | 0.95–1.08 | 0.665 | – | – | – | |
BMI | 1.22 | 1.06–1.41 | 0.005* | 1.14 | 0.98–1.33 | 0.085 | |
Hypertension | 1.50 | 0.62–3.60 | 0.364 | – | – | – | |
Active smoking | 1.48 | 0.60–3.63 | 0.395 | – | – | – | |
Active drinking | 2.31 | 0.90–5.91 | 0.082 | – | – | – | |
FBG | 1.07 | 0.97–1.19 | 0.168 | – | – | – | |
HbA1c | 1.06 | 0.90–1.24 | 0.491 | – | – | – | |
TG | 1.15 | 0.95–1.34 | 0.16 | – | – | – | |
Hyperlipidemia | 1.41 | 0.61–3.25 | 0.416 | – | – | – | |
EFV | 1.22 | 1.10–1.37 | <0.001* | 1.19 | 1.06–1.34 | 0.003* |
*, P<0.05. LVSD, left ventricular systolic dyssynchrony; OR, odds ratio; CI, confidence interval; DM, diabetes mellitus; BMI, body mass index; FBG, fasting blood glucose; HbA1c, glycosylated hemoglobin; TG, triglycerides; EFV, epicardial fat volume.
Table 4
Groups | Crude model | Multivariable-adjusted model 1 | Multivariable-adjusted model 2 | |||||
---|---|---|---|---|---|---|---|---|
OR (95% CI) | P value | OR (95% CI) | P value | OR (95% CI) | P value | |||
EFV (per 10 cm3) | 1.22 (1.10, 1.37) | <0.001* | 1.24 (1.09, 1.41) | <0.001* | 1.20 (1.05, 1.37) | <0.001* | ||
Tertiles | ||||||||
Bottom tertile (events/N =3/39) | 1 | – | 1 | – | 1 | – | ||
Middle tertile (events/N =11/39) | 4.71 (1.20, 18.53) | 0.026* | 4.51 (1.13, 17.93) | 0.032* | 4.71 (1.07, 20.67) | 0.040* | ||
Top tertile (events/N =18/39) | 10.29 (2.71, 39.11) | <0.001* | 9.69 (2.42, 38.83) | 0.001* | 5.60 (1.26, 24.94) | 0.024* |
Multivariable model 1 was adjusted for age and sex; multivariable model 2 (confounder model) was adjusted for age, sex, BMI, DM duration, presence of hypertension, active smoking, FBG, HbA1c and TG. *, P<0.05. EFV, epicardial fat volume; LVSD, left ventricular systolic dyssynchrony; OR, odds ratio; CI, confidence interval; BMI, body mass index; DM, diabetes mellitus; FBG, fasting blood glucose; HbA1c, glycosylated hemoglobin; TG, triglycerides.
Smooth curve fitting
GAM (Figure 3) was performed to visually assess the association between EFV and LVSD after adjusting for age, sex, BMI, DM duration, presence of hypertension, active smoking, FBG, HbA1c, and TG; it turned out that EFV tended to be linearly associated with LVSD.
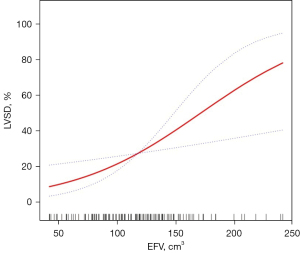
The ROC curve analysis
To assess the predictive value of EFV for LVSD, we performed ROC curve analysis, the area under the curve (AUC) was 0.732 (95% CI: 0.633–0.831, P<0.001) with the optimal cut-off values of EFV (122.26 cm3) (Figure 4). The sensitivity, specificity, positive predictive value (PPV), and negative predictive value (NPV) were 71.9%, 69.4%, 45.8%, and 85.5%, respectively.
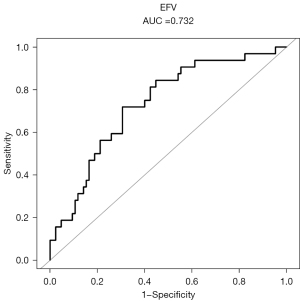
Discussion
In this study, we evaluated the relationship between EFV and LVSD in T2DM patients with normal LVEF and normal myocardial perfusion. The main findings were as follows. Firstly, the LVSD group had significantly higher EFV than the non-LVSD group, and multivariate logistic regression analysis showed EFV was the independent risk factor for LVSD. Secondly, a linear relationship was observed between EFV and LVSD, which remained significant after adjusting for traditional cardiovascular risk factors. For each 10 cm3 increased in EFV, the risk of LVSD increased by 20.0%. Thirdly, age, BMI, incidence of hypertension, and LVSD were closely associated with EFV and increased with tertiles of EFV. Finally, EFV could effectively predict LVSD at the cutoff value of 122.26 cm3, with the sensitivity of 71.9% and NPV of 85.5%.
LVSD existed in T2DM patients with normal myocardial perfusion and normal LVEF, which is also known as subclinical myocardial damage and challenging to diagnose early (23,24). Previous studies based on SPECT-MPI have demonstrated that 23–28% of T2DM patients with normal LVEF and normal myocardial perfusion had the presence of LVSD (19,22), which was similar to our findings. A large community-based cohort study found that patients with subclinical myocardial damage have a significantly increased risk of HF and all-cause mortality than those without subclinical myocardial damage (25). Therefore, it is crucial to investigate the factors contributing to early myocardial damage, which may help in diagnostic and treatment decisions, risk stratification, and improve poor prognosis.
Previous studies have found that overweight was independently associated with subclinical myocardial injury quantified by radionuclide MPI and 3-dimensional echocardiography (5,19,26). In addition to measuring systemic fat, we also assessed regional fat distribution by measuring EFV, which is a special visceral adipose tissue located between the myocardium around the heart and the visceral pericardium, and may be a superior marker compared to BMI. We found that LVSD tended to be linearly associated with EFV and the probability of LVSD increased by 20% for every 10 cm3 increase in EFV after adjusting for confounding factors. Multiple studies have assessed the impact of EAT on subclinical myocardial damage in T2DM patients. Zhu et al. observed that EFV measured by MRI was independently linked to left ventricular global peak systolic longitudinal strain and left ventricular peak diastolic longitudinal strain rate (27). However, the measurement of EFV by MRI is limited in clinical application. In another study utilizing ultrasound, Christensen et al. found that the thickness of pericardial adipose tissue was associated with impaired global longitudinal strain (28). However, ultrasound has the limitation of reduced objectivity and reproducibility. The results of our study are similar to those measured by other imaging technologies. SPECT-CT allows for the concurrent assessment of myocardial perfusion and left ventricular systolic synchrony, demonstrating favorable test-retest reliability. In addition to the limitations already discussed, it is important to note that the SPECT-CT utilized in this study does involve exposure to ionizing radiation. However, it does not increase the risk of cancer when the radiation dose is less than 100 mSv. The effective radiation dose for a standard 99mTc-MIBI imaging (including both rest and stress imaging) is 9–12 mSv, and the dose for a non-contrast CT chest scan (voltage, 130 kV; tube current, 100 mA) to assess CACS and EFV is 1–2 mSv (29).
The pathophysiology connecting EAT to myocardial damage may involve several mechanisms. Firstly, accumulation of EAT may lead to disturbances in glucose and lipid metabolism. EAT has been found to be associated with insulin resistance and metabolic syndrome (30,31). Hyperglycemia damages the myocardium and aggravates mitochondrial dysfunction, and the release of inflammatory factors and oxidative stress (32). The microenvironment of diabetes triggers cellular senescence, leading to excessive release of free fatty acids by adipose tissue, causing lipotoxicity (33). Secondly, as an endocrine organ, EAT secretes various cytokines and pro-inflammatory chemokines, such as tumor necrosis factor-alpha (TNF-α), interleukins (ILs), and adiponectin, directly or indirectly acting on the myocardium and cardiac blood vessels (34). Thirdly, EAT is closely related to coronary microcirculation. The study by Nakanishi et al. found the association of periventricular EAT with impaired coronary flow reserve (CFR) and deteriorated left ventricular diastolic function (35). Numerous observational investigations have documented that individuals with T2DM manifest an aberrantly augmented and biologically altered EAT in comparison to their non-diabetic counterparts. This enlarged EAT exerts not only a mechanical constraint on diastolic filling but also serves as a reservoir for pro-inflammatory mediators, which have the potential to induce inflammation, microcirculatory dysfunction, and fibrosis within the subjacent myocardium. Consequently, these pathological processes impede the relaxability of the left ventricle and escalate its filling pressure (36).
Using a threshold value of 122.26 cm3, EFV could effectively predict LVSD with the AUC, sensitivity, and specificity of 0.73, 71.9%, and 69.4% respectively. Similarly, Maimaituxun et al. found that in patients with normal LVEF, EFV showed a significant power for predicting myocardial dysfunction using GLS ≤18 by ultrasound as the reference with the cutoff value of 116 cm3 and the AUC, sensitivity, and specificity were 0.60, 62.3%, and 60.6%, respectively (37). Therefore, T2DM patients with excess EFV may need appropriate intervention to prevent subclinical myocardial damage and further deterioration of left ventricular function. Since EAT is a modifiable factor, therapeutic strategies are targeted to reduce EAT. For example, both glucagon-like-peptide-1 receptor agonists (GLP-1) and inhibitors of sodium glucose cotransporter 2 (SGLT2i) exhibit the potential to decrease the accumulation of EAT and provide further cardiovascular benefits (38,39). EAT may be a potential therapeutic target for subclinical myocardial damage and whether the LVSD could be improved after reducing EFV accumulation needs further verification. Besides, it has been confirmed that SGLT2i can significantly improve the longitudinal strain of T2DM in the early stage (40), whether the efficacy can be ascribed to the direct effect on EAT still requires further study.
Our study has several limitations. Firstly, it is a single-center retrospective observational study with a small sample size. Future detailed prospective studies with larger samples are necessary to further investigate the potential causal relationship between EFV and subclinical myocardial damage in people with diabetes. Secondly, due to limitations in laboratory conditions, various adipokines and pro-inflammatory factors related to EAT were not detected. Thirdly, we did not conduct oral glucose tolerance tests or glucose clamp studies to measure insulin resistance. As insulin resistance plays a central pathophysiological role in the possible correlation between epicardial fat and subclinical myocardial damage in patients with T2DM, the relationship between the accumulation of epicardial fat, insulin resistance, and subclinical myocardial damage deserves further research. Fourthly, there is a lack of measurements of waist or hip circumference. Finally, due to a lack of follow-up assessments, the prognostic value of EFV remains unknown.
Conclusions
EFV is an independent risk factor for LVSD in T2DM patients with normal LVEF and normal MPI, which could serve as a novel imaging marker and a potential therapeutic target for subclinical myocardial damage.
New knowledge gained
In the present study, we elucidated the relationship between EFV and LVSD in T2DM patients with normal LVEF and normal myocardial perfusion. We concluded that EFV was the independent risk factor for LVSD in T2DM patients with normal LVEF and normal MPI, which might serve as a novel imaging marker and a potential therapeutic target for subclinical myocardial damage.
Acknowledgments
Funding: This research was supported by
Footnote
Reporting Checklist: The authors have completed the STROBE reporting checklist. Available at https://qims.amegroups.com/article/view/10.21037/qims-23-1413/rc
Conflicts of Interest: All authors have completed the ICMJE uniform disclosure form (available at https://qims.amegroups.com/article/view/10.21037/qims-23-1413/coif). The authors have no conflicts of interest to declare.
Ethical Statement: The authors are accountable for all aspects of the work in ensuring that questions related to the accuracy or integrity of any part of the work are appropriately investigated and resolved. The study was conducted in accordance with the Declaration of Helsinki (as revised in 2013) and approved by the Ethics Committee of The Third Affiliated Hospital of Soochow University (No. 2023-062). The requirement for informed consent was waived due to the retrospective nature of the study.
Open Access Statement: This is an Open Access article distributed in accordance with the Creative Commons Attribution-NonCommercial-NoDerivs 4.0 International License (CC BY-NC-ND 4.0), which permits the non-commercial replication and distribution of the article with the strict proviso that no changes or edits are made and the original work is properly cited (including links to both the formal publication through the relevant DOI and the license). See: https://creativecommons.org/licenses/by-nc-nd/4.0/.
References
- Sun H, Saeedi P, Karuranga S, Pinkepank M, Ogurtsova K, Duncan BB, Stein C, Basit A, Chan JCN, Mbanya JC, Pavkov ME, Ramachandaran A, Wild SH, James S, Herman WH, Zhang P, Bommer C, Kuo S, Boyko EJ, Magliano DJ. IDF Diabetes Atlas: Global, regional and country-level diabetes prevalence estimates for 2021 and projections for 2045. Diabetes Res Clin Pract 2022;183:109119. [Crossref] [PubMed]
- Ritchie RH, Abel ED. Basic Mechanisms of Diabetic Heart Disease. Circ Res 2020;126:1501-25. [Crossref] [PubMed]
- Murtaza G, Virk HUH, Khalid M, Lavie CJ, Ventura H, Mukherjee D, Ramu V, Bhogal S, Kumar G, Shanmugasundaram M, Paul TK. Diabetic cardiomyopathy - A comprehensive updated review. Prog Cardiovasc Dis 2019;62:315-26. [Crossref] [PubMed]
- Lorenzo-Almorós A, Tuñón J, Orejas M, Cortés M, Egido J, Lorenzo Ó. Diagnostic approaches for diabetic cardiomyopathy. Cardiovasc Diabetol 2017;16:28. [Crossref] [PubMed]
- Wang Y, Yu W, Yang X, Zhang F, Sun Y, Hu Y, Yang L, Jiang Q, Wang J, Shao X, Wang Y. Left ventricular systolic dyssynchrony: a novel imaging marker for early assessment of myocardial damage in Chinese type 2 diabetes mellitus patients with normal left ventricular ejection fraction and normal myocardial perfusion. J Nucl Cardiol 2023;30:1797-809. [Crossref] [PubMed]
- Nagueh SF. Mechanical dyssynchrony in congestive heart failure: diagnostic and therapeutic implications. J Am Coll Cardiol 2008;51:18-22. [Crossref] [PubMed]
- Fudim M, Dalgaard F, Fathallah M, Iskandrian AE, Borges-Neto S. Mechanical dyssynchrony: How do we measure it, what it means, and what we can do about it. J Nucl Cardiol 2021;28:2174-84. [Crossref] [PubMed]
- Hage FG. Left ventricular mechanical dyssynchrony by phase analysis as a prognostic indicator in heart failure. J Nucl Cardiol 2014;21:67-70. [Crossref] [PubMed]
- Iacobellis G. Epicardial adipose tissue in contemporary cardiology. Nat Rev Cardiol 2022;19:593-606.
- Yu W, Liu B, Zhang F, Wang J, Shao X, Yang X, Shi Y, Wang B, Xu Y, Wang Y. Association of Epicardial Fat Volume With Increased Risk of Obstructive Coronary Artery Disease in Chinese Patients With Suspected Coronary Artery Disease. J Am Heart Assoc 2021;10:e018080. [Crossref] [PubMed]
- Arshi B, Aliahmad HA, Ikram MA, Bos D, Kavousi M. Epicardial Fat Volume, Cardiac Function, and Incident Heart Failure: The Rotterdam Study. J Am Heart Assoc 2023;12:e026197. [Crossref] [PubMed]
- van Rosendael AR, Smit JM, El'Mahdiui M, van Rosendael PJ, Leung M, Delgado V, Bax JJ. Association between left atrial epicardial fat, left atrial volume, and the severity of atrial fibrillation. Europace 2022;24:1223-8. [Crossref] [PubMed]
- Liu J, Li J, Pu H, He W, Zhou X, Tong N, Peng L. Cardiac remodeling and subclinical left ventricular dysfunction in adults with uncomplicated obesity: a cardiovascular magnetic resonance study. Quant Imaging Med Surg 2022;12:2035-50. [Crossref] [PubMed]
- Muzurović EM, Vujošević S, Mikhailidis DP. Can We Decrease Epicardial and Pericardial Fat in Patients With Diabetes? J Cardiovasc Pharmacol Ther 2021;26:415-36. [Crossref] [PubMed]
- Jellis CL, Sacre JW, Wright J, Jenkins C, Haluska B, Jeffriess L, Martin J, Marwick TH. Biomarker and imaging responses to spironolactone in subclinical diabetic cardiomyopathy. Eur Heart J Cardiovasc Imaging 2014;15:776-86. [Crossref] [PubMed]
- Henzlova MJ, Duvall WL, Einstein AJ, Travin MI, Verberne HJ. ASNC imaging guidelines for SPECT nuclear cardiology procedures: Stress, protocols, and tracers. J Nucl Cardiol 2016;23:606-39. [Crossref] [PubMed]
- Driessen RS, Raijmakers PG, Danad I, Stuijfzand WJ, Schumacher SP, Leipsic JA, Min JK, Knuuti J, Lammertsma AA, van Rossum AC, van Royen N, Underwood SR, Knaapen P. Automated SPECT analysis compared with expert visual scoring for the detection of FFR-defined coronary artery disease. Eur J Nucl Med Mol Imaging 2018;45:1091-100. [Crossref] [PubMed]
- Chen J, Garcia EV, Bax JJ, Iskandrian AE, Borges-Neto S, Soman P. SPECT myocardial perfusion imaging for the assessment of left ventricular mechanical dyssynchrony. J Nucl Cardiol 2011;18:685-94. [Crossref] [PubMed]
- Malik D, Mittal B, Sood A, Parmar M, Kaur G, Bahl A. Left ventricular mechanical dyssynchrony assessment in long-standing type II diabetes mellitus patients with normal gated SPECT-MPI. J Nucl Cardiol 2019;26:1650-8. [Crossref] [PubMed]
- Huang G, Wang D, Zeb I, Budoff MJ, Harman SM, Miller V, Brinton EA, El Khoudary SR, Manson JE, Sowers MR, Hodis HN, Merriam GR, Cedars MI, Taylor HS, Naftolin F, Lobo RA, Santoro N, Wildman RP. Intra-thoracic fat, cardiometabolic risk factors, and subclinical cardiovascular disease in healthy, recently menopausal women screened for the Kronos Early Estrogen Prevention Study (KEEPS). Atherosclerosis 2012;221:198-205. [Crossref] [PubMed]
- Song XT, Wang SK, Zhang PY, Fan L, Rui YF. Association between epicardial adipose tissue and left ventricular function in type 2 diabetes mellitus: Assessment using two-dimensional speckle tracking echocardiography. J Diabetes Complications 2022;36:108167. [Crossref] [PubMed]
- Hosseinzadeh E, Ghodsirad MA, Alirezaei T, Arfenia M. Pirayesh, Amoiee M, Norouzi GH. Comparing left ventricular mechanical dyssynchrony between diabetic and non-diabetic patients with normal gated SPECT MPI. Int J Cardiovasc Imaging 2022;38:249-56. [Crossref] [PubMed]
- Nappi C, Gaudieri V, Acampa W, Assante R, Zampella E, Mainolfi CG, Petretta M, Germano G, Cuocolo A. Comparison of left ventricular shape by gated SPECT imaging in diabetic and nondiabetic patients with normal myocardial perfusion: A propensity score analysis. J Nucl Cardiol 2018;25:394-403. [Crossref] [PubMed]
- Gaudieri V, Nappi C, Acampa W, Zampella E, Assante R, Mannarino T, Genova A, De Simini G, Klain M, Germano G, Petretta M, Cuocolo A. Added prognostic value of left ventricular shape by gated SPECT imaging in patients with suspected coronary artery disease and normal myocardial perfusion. J Nucl Cardiol 2019;26:1148-56. [Crossref] [PubMed]
- Selvin E, Lazo M, Chen Y, Shen L, Rubin J, McEvoy JW, Hoogeveen RC, Sharrett AR, Ballantyne CM, Coresh J. Diabetes mellitus, prediabetes, and incidence of subclinical myocardial damage. Circulation 2014;130:1374-82. [Crossref] [PubMed]
- Wu T, Gong L, Zhang C, Zhang D, Li X. Three-dimensional echocardiography and strain cardiac imaging in patients with prediabetes and type 2 diabetes mellitus. Quant Imaging Med Surg 2023;13:7753-64. [Crossref] [PubMed]
- Zhu J, Li W, Xie Z, Zhuo K. Relationship Between Epicardial Adipose Tissue and Biventricular Longitudinal Strain and Strain Rate in Patients with Type 2 Diabetes Mellitus. Acad Radiol 2023;30:833-40. [Crossref] [PubMed]
- Christensen RH, Hansen CS, von Scholten BJ, Jensen MT, Pedersen BK, Schnohr P, Vilsbøll T, Rossing P, Jørgensen PG. Epicardial and pericardial adipose tissues are associated with reduced diastolic and systolic function in type 2 diabetes. Diabetes Obes Metab 2019;21:2006-11. [Crossref] [PubMed]
- Salvatori M, Rizzo A, Rovera G, Indovina L, Schillaci O. Radiation dose in nuclear medicine: the hybrid imaging. Radiol Med 2019;124:768-76. [Crossref] [PubMed]
- Cerit Z. Like two peas in a pod: Metabolic syndrome and epicardial obesity. Diabetes Metab Syndr 2018;12:477. [Crossref] [PubMed]
- Levelt E, Pavlides M, Banerjee R, Mahmod M, Kelly C, Sellwood J, Ariga R, Thomas S, Francis J, Rodgers C, Clarke W, Sabharwal N, Antoniades C, Schneider J, Robson M, Clarke K, Karamitsos T, Rider O, Neubauer S. Ectopic and Visceral Fat Deposition in Lean and Obese Patients With Type 2 Diabetes. J Am Coll Cardiol 2016;68:53-63. [Crossref] [PubMed]
- Gandoy-Fieiras N, Gonzalez-Juanatey JR, Eiras S. Myocardium Metabolism in Physiological and Pathophysiological States: Implications of Epicardial Adipose Tissue and Potential Therapeutic Targets. Int J Mol Sci 2020;21:2641. [Crossref] [PubMed]
- Molinaro C, Salerno L, Marino F, Scalise M, Salerno N, Pagano L, De Angelis A, Cianflone E, Torella D, Urbanek K. Unraveling and Targeting Myocardial Regeneration Deficit in Diabetes. Antioxidants (Basel) 2022;11:208. [Crossref] [PubMed]
- Gaborit B, Sengenes C, Ancel P, Jacquier A, Dutour A. Role of Epicardial Adipose Tissue in Health and Disease: A Matter of Fat? Compr Physiol 2017;7:1051-82. [Crossref] [PubMed]
- Nakanishi K, Fukuda S, Tanaka A, Otsuka K, Taguchi H, Shimada K. Relationships Between Periventricular Epicardial Adipose Tissue Accumulation, Coronary Microcirculation, and Left Ventricular Diastolic Dysfunction. Can J Cardiol 2017;33:1489-97. [Crossref] [PubMed]
- Salvatore T, Galiero R, Caturano A, Vetrano E, Rinaldi L, Coviello F, Di Martino A, Albanese G, Colantuoni S, Medicamento G, Marfella R, Sardu C, Sasso FC. Dysregulated Epicardial Adipose Tissue as a Risk Factor and Potential Therapeutic Target of Heart Failure with Preserved Ejection Fraction in Diabetes. Biomolecules 2022;12:176. [Crossref] [PubMed]
- Maimaituxun G, Kusunose K, Yamada H, Fukuda D, Yagi S, Torii Y, Yamada N, Soeki T, Masuzaki H, Sata M, Shimabukuro M. Deleterious Effects of Epicardial Adipose Tissue Volume on Global Longitudinal Strain in Patients With Preserved Left Ventricular Ejection Fraction. Front Cardiovasc Med 2020;7:607825. [Crossref] [PubMed]
- Iacobellis G, Baroni MG. Cardiovascular risk reduction throughout GLP-1 receptor agonist and SGLT2 inhibitor modulation of epicardial fat. J Endocrinol Invest 2022;45:489-95. [Crossref] [PubMed]
- Dozio E, Vianello E, Malavazos AE, Tacchini L, Schmitz G, Iacobellis G, Corsi Romanelli MM. Epicardial adipose tissue GLP-1 receptor is associated with genes involved in fatty acid oxidation and white-to-brown fat differentiation: A target to modulate cardiovascular risk? Int J Cardiol 2019;292:218-24. [Crossref] [PubMed]
- Oka S, Kai T, Hoshino K, Watanabe K, Nakamura J, Abe M, Watanabe A. Effects of empagliflozin in different phases of diabetes mellitus-related cardiomyopathy: a prospective observational study. BMC Cardiovasc Disord 2021;21:217. [Crossref] [PubMed]