The impact of paramagnetic rim lesions on cortical thickness and gray to white matter contrast in relapsing-remitting multiple sclerosis
Introduction
Multiple sclerosis (MS) is a disease characterized by inflammatory demyelinating lesions in the central nervous system (CNS) that cause structural and functional changes in the brain, including extensive cortical thinning, deep gray matter (DGM) atrophy, and abnormal functional connectivity (1-3). The cascade pathology of MS lesions leads to changes in neurons and microenvironment causing changes in gray matter (GM) and white matter (WM). Retrograde and anterograde degeneration resulting from focal axon transection and tissue damage caused by lesions may be the main contributors to morphological changes in the brain.
The pathophysiological mechanisms described above lead to structural changes in the brain that could be demonstrated by structural magnetic resonance imaging (MRI). Several previous studies have analyzed cortical thickness in MS patients and found cortical thinning in multiple regions, which could indicate the extent of neurodegenerative damage in patients with MS (4-7). However, detectable cortical thinning indicates that the damage is already in an irreversible state, so we hoped to find imaging metrics that are more sensitive to reflect structural brain damage.
In recent years, a relatively new method of measuring structural brain damage, which quantifies disease severity by focusing on the GM to WM signal intensity ratio (GWR), has been investigated in many diseases (8-10). The T1 relaxation time is influenced by the microstructural properties of the tissue and the cellular water/lipid content, thus exhibiting differences in T1 signal intensity between tissues, namely, low signal in GM and high signal in WM (11). Pathological processes in MS such as myelin degradation, axonal loss, and iron deposition affect tissue microstructure or cellular content and therefore lead to alterations in local or global T1 relaxation time (12,13). Contrast changes at the gray and WM borderline may be consistent with the pathological processes described above (14). Thus, GWR appears to sensitively reflect the pathophysiological changes of brain tissue in the early stages of disease progression and may reflect pathological mechanisms that precede the onset of cortical atrophy (15).
Relapsing-remitting MS (RRMS) is the most common MS subtype, but due to pathological heterogeneity, RRMS patients showed significant differences in imaging manifestations, treatment response, and prognosis (16,17). The accurate classification of RRMS is helpful for clinicians to develop treatment options, which meets the current clinical needs. A subset of MS lesions is defined as chronic active lesions surrounded by activated macrophages and iron-rich microglia (18). Such lesions, which are confirmed by combined MRI/histopathologic studies to exhibit paramagnetic rim lesions (PRLs) on susceptibility magnetic resonance (MR) sequences, are associated with persistent neuroinflammation and tissue damage, leading to more severe disability (19). Therefore, investigating the impact of PRL on structural brain damage in RRMS patients could help to classify RRMS and assess the impact of persistent damage on pathophysiological changes in the brain.
In this study, we hypothesized that RRMS patients with PRL would have different degrees of structural brain alterations compared to patients without PRL. By comparing GWR and cortical thickness in patients with and without PRL, we hoped to find imaging metrics that would be sensitive to cortical damage in RRMS patients. Furthermore, we aimed to investigate whether the presence of PRL implies more severe cortical damage,which could contribute to the classification and precise treatment of RRMS patients. We present this article in accordance with STROBE reporting checklist (available at https://qims.amegroups.com/article/view/10.21037/qims-23-1395/rc).
Methods
Patient population
This retrospective study was conducted in accordance with the Declaration of Helsinki (as revised in 2013). This study was approved by the Institutional Review Board of Tongji Hospital affiliated with Tongji Medical College of Huazhong University of Science and Technology (ethical approval number: TJ-IRB202303157) and written informed consent was provided by all patients.
The inclusion criteria were patients who: (I) were diagnosed as RRMS, (II) had undergone MRI examinations that included T2 fluid-attenuated inversion recovery (FLAIR) images, T1-weighted images (T1WI), and 3-dimensional (3D) multi-echo gradient echo (mGRE) images, and (III) had not received steroid therapy within 4 weeks before the MRI scan. The Expanded Disability Status Scale (EDSS) scores were used clinically to assess the degree of disability. From May 2014 to May 2023, a total of 102 RRMS patients met the inclusion criteria. We excluded 20 patients according to the following exclusion criteria: (I) younger than 18 years old (n=6), (II) MR images with motion artifacts (n=5), and (III) poor image reconstruction quality (n=9). Ultimately, 82 RRMS patients were included in the study. Healthy volunteers who underwent the physical examination at the hospital during the same period were recruited as the control group. Based on conventional MRI, healthy controls (HC) had no CNS diseases such as cerebrovascular disease, demyelinating and neurodegenerative diseases, brain tumor, or trauma. A total of 43 age- and gender-matched HC were included in the study. The flowchart for participant selection criteria is shown in Figure 1.
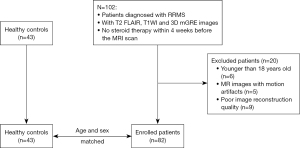
Image acquisition
MR images were obtained on a 3T MR scanner (Discovery MR750; GE Medical Systems, Waukesha, WI, USA) with a 32-channel head coil. The 3D T1WI before gadolinium-based contrast injection was acquired using the BRAin VOlume (BRAVO) sequence with the following parameters: repetition time (TR)/echo time (TE) =8.16/3.18 ms, number of excitation (NEX) =1, matrix =256×256, slice thickness =1 mm, slice spacing =1 mm, and field of view (FOV) =256×256 mm2. The acquisition parameters for 3D T2 FLAIR were as follows: TR/TE =5,000/117 ms, NEX =1, matrix =256×256, slice thickness =1 mm, slice spacing =1 mm, and FOV =256×256 mm2. The 3D mGRE was obtained with the following parameters: FOV =240×240 mm2, TR =42.8 ms, TE1/ΔTE =4.5/4.9 ms, number of TEs =8, matrix =416×320, readout bandwidth =244 Hz/pixel, slice thickness =2 mm, and flip angle =20°.
Data processing
The reconstruction of quantitative susceptibility mapping (QSM) involved several steps. Firstly, nonlinear field map estimation was employed, followed by graph-cut-based phase unwrapping to estimate the total field (20). Subsequently, background field removal was performed using projection onto dipole fields (PDF) to obtain the local tissue field (21). The local field was then inverted using the morphology-enabled dipole inversion with automatic uniform cerebrospinal fluid (CSF) zero reference (MEDI+0) algorithm, which allowed for the determination of susceptibility distribution. To achieve this, the tissue field was deconvolved with the dipole kernel using Bayesian machine learning techniques (22). In this algorithm, the susceptibility value for the average ventricular CSF was set to zero (23).
The presence of PRL was determined by visual consensus between 2 neuroradiologists (with 5 and 13 years of experience, respectively) regarding the presence of a hyperintense rim completely or partially surrounding the edge of the chronic lesion on the QSM, visible on at least 2 contiguous sections. When there was a disagreement, a third neuroradiologist (with 10 years of experience) who was unaware of previous choice was called on to determine the lesion type. To exclude the influence of partial volume effects, only lesions ≥5 mm were included in the study (24). Then RRMS patients were divided into the PRL group and the non-PRL group based on the presence or absence of PRL.
T2 FLAIR images were co-registered to the 3D T1WI. Lesions on T2 FLAIR and 3D T1WI were segmented by the Lesion Growth Algorithm (LGA) in the Lesion Segmentation Toolbox (LST) in SPM12 (https://www.fil.ion.ucl.ac.uk/spm/), with the Kappa value set to 0.3 (7,25,26). The segmented probability map was checked by a neuroradiologist (with 5 years of experience) and manual corrections were made for any inaccuracies. Then the segmented probability map of the lesion was used for lesion filling of T1WI by the “lesion filling” function of LST. The images were corrected for intensity nonuniformity prior to reconstruction of the cortical surface to reduce the effect of B1 and coil profile-induced nonuniformity (15). The T1WI were processed using the FreeSurfer (package 7.1.1; http://surfer.nmr.mgh.harvard.edu), to automatically reconstruct the cortical surface and measure the cortical thickness of the entire brain. We visually examined the cortical surface reconstructions of each participant without knowledge of any clinical outcomes and made manual corrections where necessary. Cortical thickness was defined as the closest distance from the gray/white boundary to the gray/CSF boundary at each vertex. We also recorded the volumes of cortex, WM, and DGM segmented by FreeSurfer. To correct for the difference in head size, the brain region volume of the participant was divided by the total intracranial volume (TIV) of that participant as a normalization process. Therefore, the normalized brain structure volumes were calculated in patients with PRL, patients without PRL, and HC, respectively.
GWR was calculated from the signal intensity of T1WI as another measure to assess pathophysiological changes in RRMS patients. The signal intensity of the GM was sampled from 35% of the total cortical thickness near the GM/WM boundary, and the signal intensity of the WM was sampled at a constant distance of 1 mm subjacent to gray/white boundary of the surface (Figure 2) (27). The GWR for each vertex was obtained by dividing the signal intensity of the GM by the signal intensity of the WM. Similar to previous studies (8,10,14), whole-brain surface maps of cortical thickness and GWR were registered to the surface of the template and smoothed using a kernel with full-width-half-maximum (FWHM) of 10 mm.
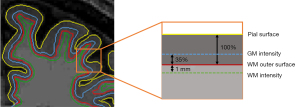
Statistical analyses
The Chi-square test, one-way analysis of variance (ANOVA) test, and Mann-Whitney U test were used to compare the differences in clinical characteristics between groups. General linear model analysis with gender and age as covariates was used to compare differences in brain morphometric metrics (mean cortical thickness, mean GWR, normalized cortex volume, normalized WM volume, and normalized DGM volume) between the PRL group, the non-PRL group, and the HC group. Statistical difference maps were generated between the PRL group, the non-PRL group, and the HC group by comparing cortical thickness and GWR based on vertex-by-vertex general linear model analysis in FreeSurfer, using gender and age as covariates. Monte Carlo permutation cluster analysis (P<0.05) with 1,000 permutations was applied for multiple comparisons correction. Finally, partial correlation analysis controlling for gender and age was used to analyze the relationship between MRI metrics (mean cortical thickness and GWR) and clinical characteristics in the PRL group. P<0.05 was considered statistically significant.
Results
Demographic and clinical characteristics
Table 1 showes the general characteristics of the participants. There were no participants with missing data for each variable of interest. There were 40 patients in the PRL group [PRL volume, median: 1.461 mL, interquartile range (IQR): 0.664–5.029 mL; non-PRL volume, median: 7.416 mL, IQR: 2.479–14.358 mL] and 42 patients in the non-PRL group (non-PRL volume, median: 1.645 mL, IQR: 0.416–6.012 mL). The PRL group had a larger total lesion volume and non-PRL volume compared to the non-PRL group (P<0.001, P<0.001, respectively). There was no statistically significant difference in gender and age among the PRL group, the non-PRL group, and the HC group (P=0.525, P=0.089, respectively). Moreover, there was no significant difference in disease duration and EDSS between the PRL group and the non-PRL group (P=0.138, P=0.155, respectively).
Table 1
Metrics | PRL group (n=40) | Non-PRL group (n=42) | HC group (n=43) | P value |
---|---|---|---|---|
Gender, female | 24 (60.0) | 30 (71.4) | 27 (62.8) | 0.525a |
Age, years | 38.35±10.72 | 33.43±10.91 | 34.26±10.47 | 0.089b |
Disease duration, years | 4.00 (0.35, 8.00) | 2.00 (0.23, 5.19) | – | 0.138c |
EDSS | 3.25 (2.00, 5.00) | 3.00 (2.00, 3.50) | – | 0.155c |
Total lesion volume, mL | 13.162 (3.817, 19.949) | 1.645 (0.416, 6.012) | – | <0.001c |
PRL count | 3 (2, 10) | – | – | – |
PRL volume, mL | 1.461 (0.664, 5.029) | – | – | – |
Non-PRL count | 34 (16, 58) | 9 (3, 14) | – | <0.001c |
Non-PRL volume, mL | 7.416 (2.479, 14.358) | 1.645 (0.416, 6.012) | – | <0.001c |
Data are presented as n (%), mean ± SD or median (IQR). a, the P value was obtained by the Chi-square test; b, the P value was obtained by one-way analysis of variance test; c, the P value was obtained by Mann-Whitney U test. PRL, paramagnetic rim lesion; HC, healthy controls; EDSS, Expanded Disability Status Scale; SD, standard deviation; IQR, interquartile range.
Mean cortical thickness and mean GWR and brain structure volume analysis
Compared to HC, the PRL group exhibited thinner mean cortical thickness and higher mean GWR (P<0.001, P=0.001). Furthermore, patients with PRL showed significant reductions in normalized cortex volume, WM volume, and DGM volume (P=0.001, P<0.001, P<0.001, respectively). Compared to HC, the non-PRL group had higher GWR and lower normalized DGM volume (P=0.019, P=0.016). In addition, significant differences in normalized WM volume and DGM volume were observed between patients with and without PRL (P=0.005, P=0.001) (Figure 3).
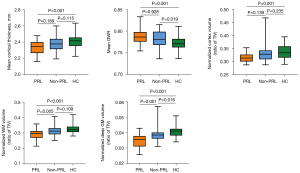
Cortical thickness analysis
RRMS patients with PRL showed multiple regional cortical thinning in the left and right hemispheres compared to HC (Figure 4). Table 2 summarizes the regions with significant cortical thinning. Certain regions, such as the parahippocampal, superior temporal, and fusiform, demonstrated symmetrical cortical thinning in the bilateral hemispheres, whereas other regions exhibited unilateral cortical thinning in each hemisphere. For example, the superior frontal was more affected in the left hemisphere, and the entorhinal in the right hemisphere showed regional cortical thinning. When comparing the non-PRL group to the HC group, significantly thinner cortical thickness was observed in the left superior frontal [cluster-wise P-value (CWP) =0.038, cluster size of 221.19 mm2] and rostral middle frontal (CWP =0.047, cluster size of 197.91 mm2) regions (Table S1). Only right parahippocampal showed significant cortical thinning in the PRL group compared to the non-PRL group (CWP =0.020, cluster size of 282.55 mm2) (Table S2).
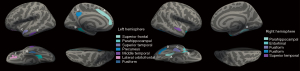
Table 2
PRL group vs. HC group | Size (mm2) | Talairach coordinates | CWP | ||
---|---|---|---|---|---|
x | y | z | |||
Left superior frontal | 1,030.00 | −12.1 | 20.1 | 35.8 | 0.002 |
Left parahippocampal | 517.18 | −33.9 | −38.2 | −11.9 | 0.002 |
Left superior temporal | 423.17 | −50.0 | −5.9 | −18.1 | 0.002 |
Left precuneus | 403.18 | −14.8 | −50.1 | 31.6 | 0.004 |
Left middle temporal | 346.92 | −55.0 | −21.0 | −24.2 | 0.006 |
Left lateral orbitofrontal | 333.77 | −20.3 | 22.9 | −16.6 | 0.006 |
Left fusiform | 214.68 | −40.2 | −23.5 | −21.6 | 0.042 |
Right parahippocampal | 593.00 | 34.4 | −35.9 | −13.0 | 0.002 |
Right entorhinal | 488.18 | 27.7 | 3.0 | −33.7 | 0.006 |
Right fusiform | 466.03 | 41.9 | −17.6 | −22.8 | 0.006 |
Right fusiform | 450.45 | 40.3 | −64.4 | −12.5 | 0.008 |
Right superior temporal | 373.70 | 55.4 | −6.6 | −9.9 | 0.014 |
PRL, paramagnetic rim lesion; HC, healthy controls; CWP, cluster-wise P-value.
GWR analysis
The PRL group exhibited extensive regions with increased GWR in the bilateral hemispheres when compared to the HC group (Figure 5). Table 3 summarizes the regions displaying significant increase in GWR. In the left hemisphere, clusters of increased GWR were located in the fusiform, isthmus of cingulate, superior frontal, and pericalcarine. In the right hemisphere, clusters of increased GWR were located in the parahippocampal, isthmus of cingulate, superior frontal, and pars opercularis. In the comparison of the non-PRL group with the HC group, no significant region of increased GWR was found in either hemisphere. It is worth noting that some regions in the PRL group showed higher GWR compared to the non-PRL group, including left parahippocampal, right lingual, and entorhinal (Figure S1, Table 4).
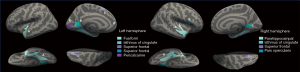
Table 3
PRL group vs. HC group | Size (mm2) | Talairach coordinates | CWP | ||
---|---|---|---|---|---|
x | y | z | |||
Left fusiform | 3,216.89 | −35.1 | −43.2 | −9.8 | 0.002 |
Left isthmus of cingulate | 859.63 | −19.2 | −49.8 | 0.2 | 0.002 |
Left superior frontal | 669.82 | −17.0 | 33.4 | 49.3 | 0.004 |
Left superior frontal | 388.54 | −16.6 | 60.8 | 4.9 | 0.020 |
Left pericalcarine | 368.86 | −12.0 | −83.4 | 2.4 | 0.024 |
Right parahippocampal | 3,527.08 | 34.0 | −43.9 | −7.6 | 0.002 |
Right isthmus of cingulate | 412.90 | 20.0 | −47.8 | 0.0 | 0.016 |
Right superior frontal | 338.44 | 16.8 | 22.5 | 54.1 | 0.022 |
Right pars opercularis | 213.13 | 52.1 | 10.2 | 2.4 | 0.047 |
GWR, GM to WM signal intensity ratio; PRL, paramagnetic rim lesion; HC, healthy controls; CWP, cluster-wise P-value; GM, gray matter; WM, white matter.
Table 4
PRL group vs. non-PRL group | Size (mm2) | Talairach coordinates | CWP | ||
---|---|---|---|---|---|
x | y | z | |||
Left parahippocampal | 254.82 | −34.5 | −41.2 | −10.1 | 0.026 |
Right lingual | 516.09 | 32.6 | −45.6 | −6.4 | 0.012 |
Right entorhinal | 422.65 | 24.9 | −10.6 | −32.9 | 0.018 |
GWR, GM to WM signal intensity ratio; PRL, paramagnetic rim lesion; CWP, cluster-wise P-value; GM, gray matter; WM, white matter.
Combined cortical thickness and GWR analysis
In order to visually assess the co-localization of cortical thickness changes and GWR changes, we put the clusters with thinner cortical thickness or higher GWR in the PRL group compared to the HC group in the same inflated brain (Figure 6). The regions displaying increased GWR were more extensive than those exhibiting cortical thinning. Notably, there were regions in both hemispheres where cortical thinning and increased GWR overlapped. Furthermore, certain regions demonstrated either cortical thinning or increased GWR exclusively.
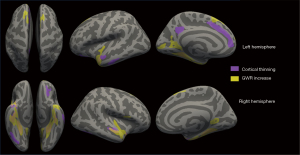
Correlation analysis between MRI metrics and clinical characteristics
Table 5 shows the results of the correlation analysis between mean cortical thickness and mean GWR and clinical characteristics in the PRL group. After controlling for gender and age, only PRL volume showed a significant negative correlation with mean cortical thickness (r=−0.323, P=0.048). There was a marginally negative correlation between PRL count and mean cortical thickness (r=−0.317, P=0.052). PRL volume, PRL count, non-PRL count, and total lesion volume exhibited a significant positive correlation with mean GWR (r=0.347, P=0.033; r=0.514, P=0.001; r=0.570, P<0.001; r=0.431, and P=0.007, respectively).
Table 5
Metrics | Mean cortical thickness | Mean GWR | |||
---|---|---|---|---|---|
r | P value | r | P value | ||
PRL volume | −0.323 | 0.048 | 0.347 | 0.033 | |
PRL count | −0.317 | 0.052 | 0.514 | 0.001 | |
Non-PRL volume | 0.011 | 0.948 | 0.291 | 0.076 | |
Non-PRL count | −0.081 | 0.630 | 0.570 | <0.001 | |
Total lesion volume | −0.172 | 0.302 | 0.431 | 0.007 | |
EDSS | −0.077 | 0.648 | 0.093 | 0.580 | |
Disease duration | −0.145 | 0.384 | 0.034 | 0.841 |
r represents the partial correlation coefficient. GWR, GM to WM signal intensity ratio; PRL, paramagnetic rim lesion; EDSS, Expanded Disability Status Scale; GM, gray matter; WM, white matter.
Discussion
In this study, we found that patients with PRL exhibited more significant cortical thinning, increased GWR, and structural brain atrophy. Vertex-based analysis revealed that RRMS patients with PRL showed significant cortical thinning in several regions and GWR increase in a wider range of regions. These two types of clusters displayed both overlapping and independent regions. In contrast, patients without PRL only showed some regions with cortical thinning. Correlation analysis further showed that only PRL volume was associated with mean cortical thickness in patients with PRL, whereas PRL volume, PRL count, non-PRL count, and total lesion volume were all positively correlated with mean GWR.
GWR, a relatively new MRI-based biomarker of tissue properties, shows potential in providing information about tissue damage caused by demyelinating lesions in the brain, complementing volumetric measurements. It has been used to reflect some of the pathologic mechanisms of Alzheimer’s disease, and manifested abnormality in some non-atrophic regions, which may reflect pathologic mechanisms that occur prior to cortical atrophy (8,15). In the present study, we observed several independent and co-localized regions of GWR abnormality and cortical thinning in RRMS patients with PRL. Notably, the extent of GWR abnormality appeared to be larger compared to cortical thickness abnormality. These findings suggest that GWR might serve as a more sensitive indicator of structural brain damage resulting from demyelination in the CNS. This metric has the potential to detect changes in microstructural properties that conventional cortical morphometry fails to capture. GWR reflects both pathological changes in the GM and subcortical WM. For example, demyelination, oligodendrocyte reduction, and axonal loss result in reduced WM signal intensity, whereas neuronal shrinkage and progressive neuronal loss lead to increased GM signal intensity, thus further resulting in higher GWR (28).
Numerous studies have investigated cortical changes in MS, but the underlying mechanisms of cortical atrophy are complex (29-31). Some studies have suggested that local cortical demyelination is the driving factor causing the loss of cortical tissue (32,33), whereas others have shown that degenerative damage caused by WM lesions plays a larger role in cortical atrophy (34-36). In contrast to previous research focusing on the impact of lesion location on the cortex, our study placed more emphasis on the damage to brain tissue caused by different lesion types. The PRLs were defined as chronic active MS lesions with iron-deposited microglia at the edge, and the release of toxins from these microglia to surrounding oligodendrocytes could limit myelin regeneration in the lesion and further promote the demyelination process (37). Some studies have suggested that the presence of these lesions may lead to disease progression as well as functional impairment (24,38). Excess iron exacerbates oxidative stress, which, in turn, increases the release of inflammatory mediators by activating microglia and macrophages, resulting in demyelination and axonal damage (39,40). The above pathological alterations may be reflected in imaging indicators such as cortical thickness and GWR in RRMS patients with PRL.
There was also significant DGM atrophy and mean GWR increase as well as significant cortical thinning in a few regions in the non-PRL group compared to HC. However, patients without PRL appear to be the subtype of RRMS with relatively milder brain tissue damage compared to patients with PRL. A previous study reported greater lesion load and more severe brain atrophy in the PRL group than in the non-PRL group (38). MS patients with PRL had higher EDSS and pyramidal function system scores than MS patients without PRL (24). Consequently, it may be possible to classify RRMS patients based on the presence or absence of PRL, with special attention given to the patients with PRL who may exhibit more severe clinical disability.
According to the correlation analysis output, the PRL load played an important role in the cortical thickness development. Moreover, the increase of PRL load could also cause the increase of GWR. The changes observed in GWR may be attributed to Wallerian degeneration, retrograde neuroaxonal degeneration, and direct inflammatory damage to the lesion. The PRL which causes chronic progressive damage to brain tissue exacerbates these pathological processes, leading to altered signal intensity in GM and WM.
There were still some limitations in our study and we hope that this will stimulate future research endeavors to better understand and utilize GWR in the study of CNS demyelinating diseases. Firstly, our sample size was small, which limited our study to exploratory purposes only. Secondly, we only conducted cross-sectional studies to explore the impact of PRL on cortical thickness and GM to WM contrast in RRMS patients, and considered that GWR was a more sensitive indicator of pathological changes in the cortex and subcortical WM, which needs to be confirmed by longitudinal studies as well as pathological findings. In addition, there was a difference in the non-PRL volume between the PRL group and the non-PRL group, which may have impacted the outcome measures. Finally, our cohort included only RRMS, so conclusions about cortical thickness and GWR may not be generalizable to patients with other types of MS.
Conclusions
Our study revealed regionally selective changes in GWR and cortical thickness specifically in RRMS patients with PRL, with the changes in GWR being more extensive. This suggests that GWR could serve as a significant biomarker for potential pathological changes in RRMS and provide further insights into the mechanisms of cortical damage caused by PRL. Moreover, the presence of PRL could potentially serve as a criterion for classifying RRMS, aiding in early and precise determination of the patient’s brain injury pattern and facilitating personalized therapeutic interventions.
Acknowledgments
Funding: This study has received funding from
Footnote
Reporting Checklist: The authors have completed the STROBE reporting checklist. Available at https://qims.amegroups.com/article/view/10.21037/qims-23-1395/rc
Conflicts of Interest: All authors have completed the ICMJE uniform disclosure form (available at https://qims.amegroups.com/article/view/10.21037/qims-23-1395/coif). All authors report this study has received funding from the National Natural Science Foundation of China (Grant Nos. U22A20354 and 81730049). The authors have no other conflicts of interest to declare.
Ethical Statement: The authors are accountable for all aspects of the work in ensuring that questions related to the accuracy or integrity of any part of the work are appropriately investigated and resolved. The study was conducted in accordance with the Declaration of Helsinki (as revised in 2013). The study was approved by the Institutional Review Board of Tongji Hospital affiliated with Tongji Medical College of Huazhong University of Science and Technology (ethical approval number: TJ-IRB202303157) and informed consent was provided by all individual participants.
Open Access Statement: This is an Open Access article distributed in accordance with the Creative Commons Attribution-NonCommercial-NoDerivs 4.0 International License (CC BY-NC-ND 4.0), which permits the non-commercial replication and distribution of the article with the strict proviso that no changes or edits are made and the original work is properly cited (including links to both the formal publication through the relevant DOI and the license). See: https://creativecommons.org/licenses/by-nc-nd/4.0/.
References
- Lorefice L, Fenu G, Mammoliti R, Carta E, Sechi V, Frau J, Coghe G, Canalis L, Barracciu MA, Marrosu G, Marrosu MG, Cocco E. Event-related potentials and deep grey matter atrophy in multiple sclerosis: Exploring the possible associations with cognition. Mult Scler Relat Disord 2021;49:102785. [Crossref] [PubMed]
- Eshaghi A, Marinescu RV, Young AL, Firth NC, Prados F, Jorge Cardoso M, et al. Progression of regional grey matter atrophy in multiple sclerosis. Brain 2018;141:1665-77. [Crossref] [PubMed]
- Zheng F, Li Y, Zhuo Z, Duan Y, Cao G, Tian D, Zhang X, Li K, Zhou F, Huang M, Li H, Li Y, Zeng C, Zhang N, Sun J, Yu C, Han X, Hallar S, Barkhof F, Liu Y. Structural and functional hippocampal alterations in Multiple sclerosis and neuromyelitis optica spectrum disorder. Mult Scler 2022;28:707-17. [Crossref] [PubMed]
- Narayana PA, Govindarajan KA, Goel P, Datta S, Lincoln JA, Cofield SS, Cutter GR, Lublin FD, Wolinsky JS. Regional cortical thickness in relapsing remitting multiple sclerosis: A multi-center study. Neuroimage Clin 2012;2:120-31. [Crossref] [PubMed]
- Kim SH, Kwak K, Hyun JW, Jeong IH, Jo HJ, Joung A, Kim JH, Lee SH, Yun S, Joo J, Lee JM, Kim HJ. Widespread cortical thinning in patients with neuromyelitis optica spectrum disorder. Eur J Neurol 2016;23:1165-73. [Crossref] [PubMed]
- Pareto D, Sastre-Garriga J, Auger C, Vives-Gilabert Y, Delgado J, Tintoré M, Montalban X, Rovira A. Juxtacortical Lesions and Cortical Thinning in Multiple Sclerosis. AJNR Am J Neuroradiol 2015;36:2270-6. [Crossref] [PubMed]
- Genç B, Aslan K, Şen S, İncesu L. Cortical morphological changes in multiple sclerosis patients: a study of cortical thickness, sulcal depth, and local gyrification index. Neuroradiology 2023;65:1405-13. [Crossref] [PubMed]
- Putcha D, Katsumi Y, Brickhouse M, Flaherty R, Salat DH, Touroutoglou A, Dickerson BC. Gray to white matter signal ratio as a novel biomarker of neurodegeneration in Alzheimer's disease. Neuroimage Clin 2023;37:103303. [Crossref] [PubMed]
- Uribe C, Segura B, Baggio HC, Abos A, Garcia-Diaz AI, Campabadal A, Marti MJ, Valldeoriola F, Compta Y, Bargallo N, Junque C. Gray/White Matter Contrast in Parkinson's Disease. Front Aging Neurosci 2018;10:89. [Crossref] [PubMed]
- Godel M, Andrews DS, Amaral DG, Ozonoff S, Young GS, Lee JK, Wu Nordahl C, Schaer M. Altered Gray-White Matter Boundary Contrast in Toddlers at Risk for Autism Relates to Later Diagnosis of Autism Spectrum Disorder. Front Neurosci 2021;15:669194. [Crossref] [PubMed]
- Jefferson AL, Gifford KA, Damon S, Chapman GW 4th, Liu D, Sparling J, Dobromyslin V, Salat DAlzheimer’s Disease Neuroimaging Initiative. Gray & white matter tissue contrast differentiates Mild Cognitive Impairment converters from non-converters. Brain Imaging Behav 2015;9:141-8. [Crossref] [PubMed]
- Xu X, Jang I, Zhang J, Zhang M, Wang L, Ye G, Zhao A, Zhang Y, Li B, Liu J, Li BAlzheimer's Disease Neuroimaging Initiative. Cortical gray to white matter signal intensity ratio as a sign of neurodegeneration and cognition independent of β-amyloid in dementia. Hum Brain Mapp 2024;45:e26532.
- Cao J, Xu X, Zhu J, Wu P, Pang H, Fan G, Cui L. Rapid quantification of global brain volumetry and relaxometry in patients with multiple sclerosis using synthetic magnetic resonance imaging. Quant Imaging Med Surg 2022;12:3104-14. [Crossref] [PubMed]
- Chwa WJ, Tishler TA, Raymond C, Tran C, Anwar F, Villablanca JP, Ventura J, Subotnik KL, Nuechterlein KH, Ellingson BM. Association between cortical volume and gray-white matter contrast with second generation antipsychotic medication exposure in first episode male schizophrenia patients. Schizophr Res 2020;222:397-410. [Crossref] [PubMed]
- Salat DH, Chen JJ, van der Kouwe AJ, Greve DN, Fischl B, Rosas HD. Hippocampal degeneration is associated with temporal and limbic gray matter/white matter tissue contrast in Alzheimer's disease. Neuroimage 2011;54:1795-802.
- Thompson AJ, Banwell BL, Barkhof F, Carroll WM, Coetzee T, Comi G, et al. Diagnosis of multiple sclerosis: 2017 revisions of the McDonald criteria. Lancet Neurol 2018;17:162-73. [Crossref] [PubMed]
- Huisman E, Papadimitropoulou K, Jarrett J, Bending M, Firth Z, Allen F, Adlard N. Systematic literature review and network meta-analysis in highly active relapsing-remitting multiple sclerosis and rapidly evolving severe multiple sclerosis. BMJ Open 2017;7:e013430. [Crossref] [PubMed]
- Dal-Bianco A, Grabner G, Kronnerwetter C, Weber M, Höftberger R, Berger T, Auff E, Leutmezer F, Trattnig S, Lassmann H, Bagnato F, Hametner S. Slow expansion of multiple sclerosis iron rim lesions: pathology and 7 T magnetic resonance imaging. Acta Neuropathol 2017;133:25-42. [Crossref] [PubMed]
- Rahmanzadeh R, Galbusera R, Lu PJ, Bahn E, Weigel M, Barakovic M, et al. A New Advanced MRI Biomarker for Remyelinated Lesions in Multiple Sclerosis. Ann Neurol 2022;92:486-502. [Crossref] [PubMed]
- Dong J, Liu T, Chen F, Zhou D, Dimov A, Raj A, Cheng Q, Spincemaille P, Wang Y. Simultaneous phase unwrapping and removal of chemical shift (SPURS) using graph cuts: application in quantitative susceptibility mapping. IEEE Trans Med Imaging 2015;34:531-40. [Crossref] [PubMed]
- Liu T, Khalidov I, de Rochefort L, Spincemaille P, Liu J, Tsiouris AJ, Wang Y. A novel background field removal method for MRI using projection onto dipole fields (PDF). NMR Biomed 2011;24:1129-36. [Crossref] [PubMed]
- Wang Y, Spincemaille P, Liu Z, Dimov A, Deh K, Li J, et al. Clinical quantitative susceptibility mapping (QSM): Biometal imaging and its emerging roles in patient care. J Magn Reson Imaging 2017;46:951-71.
- Liu Z, Spincemaille P, Yao Y, Zhang Y, Wang Y. MEDI+0: Morphology enabled dipole inversion with automatic uniform cerebrospinal fluid zero reference for quantitative susceptibility mapping. Magn Reson Med 2018;79:2795-803. [Crossref] [PubMed]
- Wittayer M, Weber CE, Platten M, Schirmer L, Gass A, Eisele P. Spatial distribution of multiple sclerosis iron rim lesions and their impact on disability. Mult Scler Relat Disord 2022;64:103967. [Crossref] [PubMed]
- van der Weijden CWJ, Pitombeira MS, Haveman YRA, Sanchez-Catasus CA, Campanholo KR, Kolinger GD, Rimkus CM, Buchpiguel CA, Dierckx RAJO, Renken RJ, Meilof JF, de Vries EFJ, de Paula Faria D. The effect of lesion filling on brain network analysis in multiple sclerosis using structural magnetic resonance imaging. Insights Imaging 2022;13:63. [Crossref] [PubMed]
- Schmidt P, Gaser C, Arsic M, Buck D, Förschler A, Berthele A, Hoshi M, Ilg R, Schmid VJ, Zimmer C, Hemmer B, Mühlau M. An automated tool for detection of FLAIR-hyperintense white-matter lesions in Multiple Sclerosis. Neuroimage 2012;59:3774-83.
- Salat DH, Lee SY, van der Kouwe AJ, Greve DN, Fischl B, Rosas HD. Age-associated alterations in cortical gray and white matter signal intensity and gray to white matter contrast. Neuroimage 2009;48:21-8. [Crossref] [PubMed]
- Filippi M, Rocca MA, Barkhof F, Brück W, Chen JT, Comi G, DeLuca G, De Stefano N, Erickson BJ, Evangelou N, Fazekas F, Geurts JJ, Lucchinetti C, Miller DH, Pelletier D, Popescu BF, Lassmann H. Attendees of the Correlation between Pathological MRI findings in MS workshop. Association between pathological and MRI findings in multiple sclerosis. Lancet Neurol 2012;11:349-60. [Crossref] [PubMed]
- Steenwijk MD, Geurts JJ, Daams M, Tijms BM, Wink AM, Balk LJ, Tewarie PK, Uitdehaag BM, Barkhof F, Vrenken H, Pouwels PJ. Cortical atrophy patterns in multiple sclerosis are non-random and clinically relevant. Brain 2016;139:115-26. [Crossref] [PubMed]
- Fujimori J, Fujihara K, Wattjes M, Nakashima I. Patterns of cortical grey matter thickness reduction in multiple sclerosis. Brain Behav 2021;11:e02050. [Crossref] [PubMed]
- Lie IA, Kerklingh E, Wesnes K, van Nederpelt DR, Brouwer I, Torkildsen Ø, Myhr KM, Barkhof F, Bø L, Vrenken H. The effect of gadolinium-based contrast-agents on automated brain atrophy measurements by FreeSurfer in patients with multiple sclerosis. Eur Radiol 2022;32:3576-87. [Crossref] [PubMed]
- Geisseler O, Pflugshaupt T, Bezzola L, Reuter K, Weller D, Schuknecht B, Brugger P, Linnebank M. The relevance of cortical lesions in patients with multiple sclerosis. BMC Neurol 2016;16:204. [Crossref] [PubMed]
- Calabrese M, Reynolds R, Magliozzi R, Castellaro M, Morra A, Scalfari A, Farina G, Romualdi C, Gajofatto A, Pitteri M, Benedetti MD, Monaco S. Regional Distribution and Evolution of Gray Matter Damage in Different Populations of Multiple Sclerosis Patients. PLoS One 2015;10:e0135428. [Crossref] [PubMed]
- Jehna M, Pirpamer L, Khalil M, Fuchs S, Ropele S, Langkammer C, Pichler A, Stulnig F, Deutschmann H, Fazekas F, Enzinger C. Periventricular lesions correlate with cortical thinning in multiple sclerosis. Ann Neurol 2015;78:530-9. [Crossref] [PubMed]
- Steenwijk MD, Daams M, Pouwels PJ, Balk LJ, Tewarie PK, Killestein J, Uitdehaag BM, Geurts JJ, Barkhof F, Vrenken H. What explains gray matter atrophy in long-standing multiple sclerosis? Radiology 2014;272:832-42. [Crossref] [PubMed]
- Treaba CA, Herranz E, Barletta VT, Mehndiratta A, Ouellette R, Sloane JA, Klawiter EC, Kinkel RP, Mainero C. The relevance of multiple sclerosis cortical lesions on cortical thinning and their clinical impact as assessed by 7.0-T MRI. J Neurol 2021;268:2473-81. [Crossref] [PubMed]
- Yao Y, Nguyen TD, Pandya S, Zhang Y, Hurtado Rúa S, Kovanlikaya I, Kuceyeski A, Liu Z, Wang Y, Gauthier SA. Combining Quantitative Susceptibility Mapping with Automatic Zero Reference (QSM0) and Myelin Water Fraction Imaging to Quantify Iron-Related Myelin Damage in Chronic Active MS Lesions. AJNR Am J Neuroradiol 2018;39:303-10. [Crossref] [PubMed]
- Wittayer M, Weber CE, Krämer J, Platten M, Schirmer L, Gass A, Eisele P. Exploring (peri-) lesional and structural connectivity tissue damage through T1/T2-weighted ratio in iron rim multiple sclerosis lesions. Magn Reson Imaging 2023;95:12-8. [Crossref] [PubMed]
- Stephenson E, Nathoo N, Mahjoub Y, Dunn JF, Yong VW. Iron in multiple sclerosis: roles in neurodegeneration and repair. Nat Rev Neurol 2014;10:459-68. [Crossref] [PubMed]
- Padureanu R, Albu CV, Mititelu RR, Bacanoiu MV, Docea AO, Calina D, Padureanu V, Olaru G, Sandu RE, Malin RD, Buga AM. Oxidative Stress and Inflammation Interdependence in Multiple Sclerosis. J Clin Med 2019;8:1815. [Crossref] [PubMed]