Automatic detection of cerebral microbleeds using susceptibility weighted imaging and artificial intelligence
Introduction
Cerebral microbleeds (CMBs) represent small accumulations of chronic blood products within the brain. They can be detected as small regions of signal loss in susceptibility weighted imaging (SWI) data (1), while their iron content can be assessed through quantitative susceptibility mapping (QSM) (2). The formation of CMBs is influenced by various factors such as age, hypertension, and the use of antidepressant drugs (3,4). Notably, the prevalence of CMBs naturally increases with age due to the gradual degeneration of the vascular system (5). CMBs have been associated with a myriad of neurovascular diseases including cerebral amyloid angiopathy (CAA) (6,7), Alzheimer’s disease (AD) and other forms of dementia (8), hypertensive arteriosclerosis, small vessel disease (9,10), stroke (11,12), and traumatic brain injury (TBI) (13). The distribution of CMBs within the brain appears to be contingent on the specific pathology, with hypertension primarily affecting basal ganglia and thalamus, vascular dementia impacting the temporal and visual cortex, and TBI leading to widespread CMB occurrence (14-16).
An increase in both the quantity and size of CMBs over time, especially concerning the spatial distribution range of lesions across the brain, has been associated with a decline in cognitive function and an increased risk of stroke (17,18), as well as the onset of dementia (19). Certain studies have explored the utility of CMB detection as a predictive tool for CAA-associated dementia (20,21), and radiation-induced brain damage in tumor patients (22,23). Additionally, SWI has been used for tumor grading based on the presence of CMBs (24). Research also indicates an association between the presence of CMBs and diffuse axonal injury in TBI (25). A recent study established a link between the detection of CMBs on SWI and the histological presence of iron-rich macrophages in the perivascular space, suggesting that the presence of CBMs is an independent predictor of disability following TBI, irrespective of CMB shape (26). The need for patient follow-up post-TBI is partly determined by the detection of CBMs (27). In summary, there are compelling indications that CMBs can serve as predictors of cognitive decline, intracerebral hemorrhage (ICH), and ischemic infarction. Thus, the quantity and distribution of CMBs are crucial in interpreting their clinical implications (16).
In clinical practice, the manual detection of CMBs can be both time consuming and error-prone. Over the past few years, numerous studies have reported varying degrees of success with artificial intelligence (AI)-based automatic CMB detection methodologies (1,28,29), however, their practical application in clinical settings remains largely untested. The main drawback of traditional machine learning models is their reliance on feature engineering. Due to the variations in shape and intensity of CMBs on SWI images, designing effective and robust features can be quite challenging. In addition, the contrast of SWI data may depend on imaging parameters, especially the field strength, echo time and image resolution.
In this study, our method employs a two-pronged AI strategy, initially leveraging a 3D fast radial symmetry algorithm, which is subsequently followed by a convolutional neural network (CNN) model for the elimination of false positives. The pipeline has outperformed single-channel models (28,30,31). Furthermore, using QSM reconstructed from SWI phase images, diamagnetic substances such as calcifications can be separated from paramagnetic blood products based on the sign of SWI phase or susceptibility. As the susceptibility of CMBs is much higher than the surrounding tissue or veins, QSM could provide valuable features for distinguishing CMBs from their mimics (28). Consequently, the primary objective of this study was to deploy a recently proposed automatic CMB detection software in a clinical environment, comparing its results with those of two experienced radiologists to verify its clinical viability (28). We present this article in accordance with the STARD reporting checklist (available at https://qims.amegroups.com/article/view/10.21037/qims-23-1319/rc).
Methods
Subject
This retrospective study was conducted in accordance with the Declaration of Helsinki (as revised in 2013). This study was approved by the Institutional Review Board of Shanghai Fourth People’s Hospital and individual consent for this retrospective analysis was waived. All data used were obtained from previous clinical consultations. This research project did not involve personal interests and was run under the guidance of a faculty at Shanghai Fourth People’s Hospital. The facility is a specialized acute stroke center at which extensive and time-conscious magnetic resonance imaging (MRI) data are collected in addition to computed tomography (CT) data as standard of care. All patients underwent a further stroke specific workup (not related to this paper).
A total of 265 patients were selected from the data center of our institution within the time frame of January 2015 to December 2018 (Figure 1). The inclusion criteria were: (I) all patients underwent both CT and magnetic resonance (MR) scans; and (II) the time interval between CT and MR scans was no more than 48 hours. The exclusion criteria were: (I) poor image quality due to the presence of motion artifacts that significantly compromised diagnostic integrity of either CT or MRI scans; and (II) lack of necessary clinical information. Eighty-five females (47–96 years old) and 180 males (53–91 years old) were finally enrolled in this study, with various medical histories including hypertension, diabetes, hyperlipidemia, ischemic stroke, ICH, intracranial vascular malformations, tumor, and inflammation. A total of 206 of these patients had a history of stroke, but they were not experiencing acute stroke at the time of the study (Table 1).
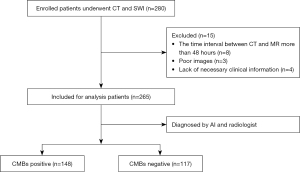
Table 1
Variables | All (n=265) | CMBs positive (n=148) | CMBs negative (n=117) | P value |
---|---|---|---|---|
Age (years) | 70.0±10.4 | 71.4±10.7 | 68.3±9.7 | 0.02 |
Female | 85 (32.1) | 48 (32.4) | 37 (31.6) | 0.98† |
Hypertension | 202 (76.2) | 120 (81.1) | 82 (70.1) | 0.10† |
Diabetes | 107 (40.4) | 64 (43.2) | 43 (36.8) | 0.39† |
Hyperlipidemia | 94 (35.5) | 51 (34.5) | 43 (36.8) | 0.57† |
Ischemic stroke | 206 (77.7) | 123 (83.1) | 83 (70.9) | 0.06† |
ICH | 27 (10.2) | 19 (12.8) | 8 (6.8) | 0.13† |
IVMs | 10 (3.8) | 8 (5.4) | 2 (1.7) | 0.13† |
Tumor | 2 (0.8) | 2 (1.4) | 0 | – |
Inflammation | 2 (0.8) | 1 (0.7) | 1 (0.9) | – |
Data are presented as mean ± standard deviation or n (%). P values are for the t-test unless otherwise indicated. †, P values are for the Chi-squared test. CMB, cerebral microbleed; ICH, intracerebral hemorrhage; IVM, intracranial vascular malformation.
MRI protocol
The MRI was performed on a 1.5-T MR system (Magnetom Avanto, Siemens Healthineers, Erlangen, Germany) for each patient with a commercial 16-channel head/neck coil. All patients took a head first supine position in the MRI scanner. The MRI sequences included: T1-weighted (T1W) sequence, T2-weighted (T2W) sequence, fluid attenuation inversion recovery (FLAIR) imaging, diffusion-weighted imaging (DWI), and SWI. The parameters used for the different sequences were: T1W: repetition time =2,000 ms, echo time =9 ms, flip angle =150°, slice thickness =4 mm, slice number =24, field of view =220 mm × 220 mm, matrix size =256×256; T2W: repetition time =3,000 ms, echo time =105 ms, flip angle =150°, slice thickness =4 mm, slice number =24, field of view =220 mm × 220 mm, matrix size =256×256, T2W-flair: repetition time =8,000 ms, echo time =80 ms, flip angle =150°, slice thickness =4 mm, slice number =30, field of view =240 mm × 240 mm, matrix size =320×320; DWI: repetition time =3,200 ms, echo time =54 ms, flip angle =180°, slice thickness =4 mm, slice number =24, field of view =220 mm × 220 mm, matrix size =256×256; SWI: repetition time =49 ms, echo time =40 ms, flip angle =15°, slice thickness =1.6 mm, field of view =220 mm × 220 mm, matrix size =256×256; and number of excitations =2. The total scan time for all MR sequences was 12 minutes and 43 seconds. Magnitude images, SWI phase images, QSM images, and maximum intensity projection (MIP) SWI images were obtained from the SWI data. QSM images were automatically processed by the built-in algorithms (version 3.2.5 of STAGE imaging software, SpinTech, Inc., Bingham Farms, MI, USA) on the vendor-provided MR workstation.
CT acquisition
The CT scan was performed on a SOMATOM Definition AS system (Siemens Healthineers) for each patient within 48 hours following the MRI scan. All patients were scanned axially in the supine position using the following imaging parameters: tube voltage =100 kV; tube current was determined by using Care Dose4D technology automatically; slice thickness =4.8 mm; field of view =220 mm × 220 mm; matrix size =512×512; window level =35 Hounsfield units (HU) and window width =80 HU.
Manual microbleed detection
A collection of multi-sequence images, including MRI (SWI, both magnitude and phase images, and QSM) and CT, were manually reviewed by two radiologists (observer 1 and observer 2, with 10 and 12 years of MRI experience, respectively). The lesions were considered as potential CMBs if they were surrounded by normal parenchyma, with clear margins, ranging from 1 to 10 mm in size on SWI. CMBs were categorized as small, round, well-defined hypointense lesions in the magnitude image and hyperintense lesions in SWI phase and QSM images, which showed a paramagnetic dipole effect (2) (Figure 2). The latter made it possible to differentiate CMBs from calcifications, which are diamagnetic, and were confirmed using the accompanying CT data. Both radiologists reviewed all the images and counted the number of CMBs. If they disagreed with the presence or absence of CMBs, discrepancies were resolved by consensus.
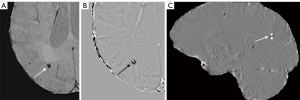
AI-based microbleed detection
The same MR images reviewed by the radiologists were then uploaded to the automatic CMB detection software (28) (SpinTech MRI), which returned a report providing the number, location based on the microbleed anatomical rating scale (MARS) (32), and volume of the CMBs. The automatic detection process included preprocessing and two major CMB detection steps (28). The preprocessing provided a set of normalized bias field corrected SWI and QSM data. CMB candidate maps were generated through the following steps. First, an initial candidate map was generated by thresholding the SWI image transformed by a 3D fast radial symmetry transform (FRST). Next, for each connected area in the candidate map, one or two candidates were generated based on the shape of that area. Then, the images underwent a 3D FRST followed by a deep residual neural network model using SWI and high pass filtered SWI phase images. Specifically, each CMB candidate was rotated around the Z-axis by 0° (i.e., the original sample), 90°, 180°, and 270°, respectively. With each rotation, the three-dimensional volume was also flipped in the Z-direction. Using each model, a total of eight predictions were made for each CMB candidate, and the average of these predictions was used as the final prediction of that model. This model was trained using SWI data from multiple sites and diseases and achieved an overall sensitivity of 95.8% and 1.3 false positives per patient (33). A report regarding the number, location and volume of CMBs was generated for every patient (Figure 3).
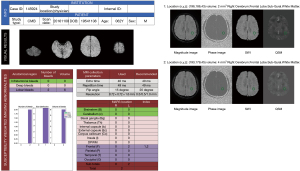
Decision of ground truth
After both manual and automatic CMB detection software were used, the reports from the latter were reviewed by the radiologists. The disagreements between the automatic CMB detection software and manual results were picked out as a checklist. Finally, a second-round manual review of the images was performed with the presence of the check-list, and the consensus of the two radiologists at this round were decided as the ground truth (Figure 4).
Statistical analysis
The difference of age between CMB positive and negative groups was reported as mean ± standard deviation and a t-test was used for comparison purposes. The difference of both sex and disease between the two groups were reported as frequency and percentage, and a Pearson chi-squared test was used for significance. We compared the differences of detection sensitivity and precision for patients with or without CMB and for individual CMBs between the radiologist and the automatic CMB detection software using Pearson chi-squared tests. All statistical analyses were performed with IBM SPSS statistical software version 25. A two-tailed P value <0.05 was considered to indicate a significant difference.
Results
A total of 265 patients (70.0±10.4 years) were enrolled in this study of which 148 presented with CMBs based on the second review of the images (Figure 4). There was a significant difference between the age of those with CMBs (71.4±10.7 years) versus those without (68.3±9.7 years, P=0.02) (Table 1). The automatic CMB detection software correctly detected 145 cases with CMBs and missed three cases with CMBs while it misjudged 27 patients as having CMBs but who did not. The two radiologists correctly found 139 cases with CMBs and missed nine cases with CMBs. They also misjudged five patients as having CMBs but who did not. Compared to the radiologists, there was no statistical difference in the sensitivity and specificity of the automatic CMB detection software in determining patients with CMBs (P=0.656 and P=0.212, Table 2). Based on the results of radiologist and the automatic CMB detection software, 90 patients did not have CMBs. Specifically, for the automatic CMB detection software 93 patients did not have CMBs, while the radiologists concluded that 121 patients did not have CMBs, which was statistically different (P=0.016, Table 2).
Table 2
Variables | Automatic CMB detection software | Radiologist | P value |
---|---|---|---|
Total, n | 265 | 265 | – |
True positive, n | 145 | 139 | – |
False positive, n | 27 | 5 | – |
False negative, n | 3 | 9 | – |
True negative, n | 90 | 112 | – |
Sensitivity (95% CI) (%) | 98.0 (95.7–100.0) | 93.9 (90.0–97.8) | 0.656 |
Specificity (95% CI) (%) | 76.9 (69.2–84.7) | 95.7 (92.0–99.4) | 0.212 |
Rule out patients without CMBs, n | 93 | 117 | 0.016 |
P values are for the chi-squared test. CMB, cerebral microbleed; CI, confidence interval.
A total of 1,738 CMBs were detected from all cases with CMBs. The automatic CMB detection software detected 1,677 lesions, missing 61. There were 273 hypointense foci on SWI that were misjudged as CMBs (i.e., false positives) by the software, which included calcifications in the pineal gland and choroid plexus. Most other false positives were either edges or mineralization near the basal ganglia or veins appearing prominently on one axial slice. Meanwhile, two radiologists found 1,393 putative CMBs of which 1,284 CMBs were determined to be actual CMBs with 109 misjudged as CMBs but which were not and 426 CMBs were missed. The sensitivity and precision of the automatic CMB detection software in detecting CMBs lesions were 96.5% and 86.0%, respectively, while those for the radiologists were 75.5% and 92.2%, respectively. Both the differences were statistically significant (P<0.001, Table 3). The false positive rate was 1.84 per case with CMBs (273/148) for the automatic CMB detection software, whereas the radiologists presented a lower rate of 0.74 per case with CMBs (109/148) but missed many CMBs. The automatic CMB detection software missed some of the CMBs in 26 cases in which were actually presence, while the radiologists missed some of the CMBs in 46 cases. Overall, the automatic CMB detection software was more sensitive than the radiologists in detecting CMBs. The automatic CMB detection software missed 61 CMBs, while the radiologists missed 426 CMBs, especially in cases with many microbleeds (more than 30 CMBs) (Table 4). More specifically, in cases with less than 30 CMBs, the radiologists missed a total of 74 lesions, while for cases with 30 or more CMBs, the raters missed 352 lesions. The small CMB lesions that were undetected by radiologists but identified by the automatic CMB detection software were generally less than 5 mm3 (cube of a micrometer) in volume, and in these patients, the automatic CMB detection software outperformed the radiologists. For example, the small CMB shown in Figure 5 was missed. However, the precision of the software was lower than that of the radiologists (Table 2). Further, the automatic CMB detection software incorrectly counted nine thromboses (Figure 6), 55 veins (Figure 7), and 29 calcifications (Figure 8) as CMBs. There were 24 CMBs at the gray-white matter junction area (Figure 9A,9B) and 14 CMBs at the edge of the brain (Figure 9C,9D) that were missed by the software.
Table 3
Variables | Automatic CMB detection software | Radiologist | P value |
---|---|---|---|
Total, n | 1,738 | 1,738 | – |
True positives, n | 1,677 | 1,284 | – |
False positives, n | 273 | 109 | – |
False negatives, n | 61 | 426 | – |
Sensitivity (95% CI) (%) | 96.5 (95.6–97.4) | 75.5 (73.0–77.1) | <0.001 |
Precision (95% CI) (%) | 86.0 (84.5–87.5) | 92.2 (90.8–93.6) | <0.001 |
P values are for the chi-squared test. CMB, cerebral microbleed; CI, confidence interval.
Table 4
Variables | Total CMBs (%)/cases with CMBs (%) | Automatic CMB detection software missed CMBs, n | Radiologists missed CMBs, n | P value |
---|---|---|---|---|
1–4 CMBs | 166 (9.55)/93 (62.84) | 4 | 10 | 0.101 |
5–9 CMBs | 76 (4.37)/11 (7.43) | 8 | 6 | 0.576 |
10–19 CMBs | 200 (11.51)/15 (10.14) | 14 | 10 | 0.400 |
20 or more CMBs | 1,296 (74.57)/29 (19.59) | 35 | 400 | <0.001 |
<30 CMBs per case with CMBs | 700 (40.28)/132 (89.19) | – | 74 | <0.001 |
≥30 CMBs per case with CMBs | 1,038 (59.72)/16 (10.81) | – | 352 |
P values are for the chi-squared test. CMB, cerebral microbleed.
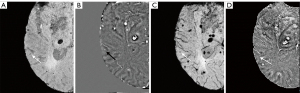
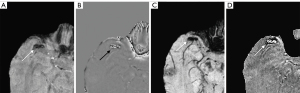
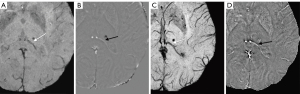
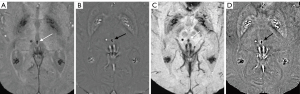
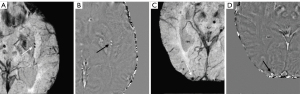
Discussion
The results presented in this study confirm the practicality of an AI-driven CMB detection algorithm that operates with increased sensitivity in a clinical environment when compared to a radiologist. A detailed examination demonstrated that the sensitivity of the automated CMB detection software could match or even exceed that of human readers, supporting findings from previously published literature (28). The results of this study provide evidence supporting the claimed effectiveness of the automated CMB detection software when applied to clinical data. As anticipated, a greater prevalence of CMBs was observed in elderly patients. While previous studies have indicated that both age and hypertension are independent risk factors for CMBs (5). However, our study found that hypertension did not notably amplify the CMB risk, potentially due to the dominating role of aging and ischemic stroke as covariates.
Despite the promise shown by the automatic CMB detection software, it was not flawless. Our data revealed that the accuracy of the automatic CMB detection software in detecting microbleeds was marginally lower than that of human raters, albeit the disparity in specificity between the two methods was not statistically significant. However, it was particularly intriguing to note that the false positives identified by the radiologists and the automatic CMB detection software did not overlap, which suggests the automatic CMB detection software could provide findings compensatory to those found by a human rater. A few instances occurred in which the automatic CMB detection software inaccurately classified thrombosis and veins as CMBs. In contrast, the radiologists identified 14 CMBs in the superficial lobes of the brain and 24 in the gray-white matter junction zone, which the automatic CMB detection software overlooked. Despite these oversights, the automatic CMB detection software outperformed the radiologists in detecting an increased number of CMBs and identifying smaller CMBs, highlighting its significant potential in advancing CMB detection technology.
Additionally, some CMB candidate lesions turned out to be shown as calcifications on QSM or SWI phase images, but not on CT images. It is possible that these lesions may undergo temporal changes, and the timing of imaging studies might have influenced the detection on CT images. This might explain why some of these small immature calcifications fail to show up as hyperdense foci on CT images but could be found on QSM. The application of QSM could eliminate the need for reviewing images in the sagittal plane to identify dipole effects. QSM has been utilized in numerous studies for quantifying in vivo iron content, calcifications, and alterations in venous oxygen saturation (2,28,34). However, it is important to note that QSM may fail to construct high-quality susceptibility maps if the calcium content is significantly high, leading to excessive SWI phase aliasing, particularly with longer echo times which are common in susceptibility-sensitive sequences. This issue can be mitigated using newer sequences that offer multi-echo versions of SWI employing shorter echoes (35).
Although the automatic CMB detection software in this study validated its potential as a valuable clinical tool, its efficiency could be further elevated if some of the false positives could be mitigated. False positives may arise from several factors unrelated to the disease, such as calcification, normal veins with high levels of deoxyhemoglobin such as in the circumstances of strokes, arteries with inadequate flow compensation, and susceptibility artifacts induced by air-tissue interfaces. In this current version of the software, the diagnosis of whether a lesion is a CMB was still based on signal changes. In our study, false positive lesions were mainly caused by three problems. Firstly, high levels of calcification could lead to the potential loss of phase information, limiting the accuracy of QSM reconstruction, and leading to strong streaking artifacts. Secondly, veins were misidentified as CMBs in some cases because of high levels of deoxyhemoglobin in the veins as occurs in stroke. In principle, with high enough resolution, these errors could be eliminated by using a vessel tracking algorithm. Radiologists, on the other hand, have knowledge of anatomy and past experience to draw on. Thirdly, iron deposition in the basal ganglia could mimic CMBs, which could be addressed by using a template approach to segment the basal ganglia and treat separately, reducing false positives.
Following the original training of this model, several automated CMB detection tools have been introduced. One such tool, rooted in AI, displayed tradeoffs in certain performance metrics, resulting in fewer false positives but at the cost of reduced sensitivity (1). A different approach employed an entirely geometric technique on SWI data, which however, produced a higher number of false positives (35). While it was not feasible to test every one of these pipelines in our dataset, the current tool has demonstrated consistency with the test data provided in the original seminal study (28). This suggests its potential reliability and applicability in real-world clinical settings.
While human raters overlooked 426 CMBs, it is unrealistic to expect radiologists to count beyond 30 CMBs, and an extensive number of CMBs is unlikely to affect the diagnosis substantially. However, knowing the accurate number of CMBs could still be useful in situations like long-term monitoring or post-treatment evaluation. Basically, no change in clinical diagnosis is likely to be observed if there are 35 or 40 CMBs or in differentiating between two patients with slightly different numbers of CMBS. However, it would be informative if 35 CMBs changed to 40 in a longitudinal follow-up. The assessment of CMBs in patients suffering from ischemic stroke carries significant importance as the presence of CMBs could potentially act as a biomarker indicating an elevated risk of bleeding and stroke recurrence. Research conducted by Shoamanesh et al. correlated the existence of CMBs with a 1.5 times higher risk of recurrent stroke, a four times greater risk of ICH, and a doubling in the risk of all-cause mortality. Furthermore, strictly lobar CMBs were associated with approximately a 2.5 times higher risk of ischemic stroke (33). Additional studies suggest that CMBs are indicative of small asymptomatic local hemorrhages and are associated with an increased risk for symptomatic ICH (36). A higher number of microbleeds was associated with lower Mini-Mental State Examination (MMSE) score and worse performance on tests of information processing speed and motor speed (37). Risk and mortality of ICH increased with quantity of CMBs. For larger numbers of CMBs in stroke patients, extra precautions should be taken to minimize risk of ICH during anticoagulation (38).
A noteworthy advantage of the automated CMB detection program, especially in cases with CMBs with a high prevalence of bleeds, is its ability to provide comprehensive data on lesion load (number and volume). The vast majority of lesions missed by the automatic CMB detection software were usually clustered together or at the edge of the cortex. The automatic CMB detection software would recognize multiple lesions clustered together as one CMB or ignored lesions that were very close to veins. These lesions can be detected by the radiologist. The lesions that are missed manually are mainly due to the size of the lesions, the vast majority of which are tiny and have no relation to the distribution of the lesions. There was no overlap between them. Ideally, integrating the anatomical position into the detection process could significantly reduce the number of false positives.
While the central aim of this paper was to evaluate the automated detection of CMBs in a clinical setting, rather than their specific etiology, a detailed statistical analysis for these clinical implications extends beyond the scope of current study. Regardless, accurate identification of patients featuring CMBs holds considerable value in a stroke center. CMBs also play a crucial role in evaluating other conditions, such as TBI. A study by Griffin et al. identified the presence of traumatic microbleeds as an independent predictor of disability, with an odds ratio of 2.5 (26). This finding was grounded in the observation of either punctate or linear appearing bleeds. Other research has indicated that the prevalence of TBI-induced CMBs may be similar to CAA-related CMBs, even though the causative factors differ (39). Considering their relative rarity in large 3D datasets and the challenge they pose for identification, the detection of CMBs exemplifies the potential benefits of incorporating AI into clinical practice.
Falsely categorizing patients without CMBs as CMB-positive can lead to unnecessary patient anxiety, inappropriate treatments, and increased healthcare costs. High false positive rates in the automatic CMB detection software stem from sensitivity-specificity trade-offs, data quality issues, algorithm complexity, and training dataset biases. Addressing these issues will require collaboration between clinicians and software developers, implementing feedback loops for continuous improvement, and promoting the use of AI as a decision support tool rather than a standalone diagnostic tool. This approach would ensure better alignment with clinical reality, reduces false positives, and enhances overall patient care. Significantly, while the human review process is lengthy, averaging 15 minutes per patient, the automatic CMB detection software currently requires only 5 minutes and simultaneously produces a detailed report outlining the location and volumes of the lesions.
There are several limitations to this study. Firstly, it is a single-center investigation, with varying resolutions and echo times employed by different manufacturers for SWI. Practically, this should not pose an issue as this algorithm was originally tested on a range of long echo time data with varying resolutions (28). However, a multi-site study could further validate its effectiveness. Secondly, the absence of a definitive gold standard for detecting CMBs poses a challenge. It is easy for radiologists to overlook small lesions during manually counting, hence we adopted the second round of consensus data as the gold standard. There is a certain probability that the radiologist and the automatic CMB detection software may miss the same lesion, which suggests the need for further improvement and training for the automatic CMB detection software to avoid this. Thirdly, the data for this study were exclusively acquired from a 1.5-T MR scanner, and the count of detected CMBs could potentially have been higher with the use of a 3-T MR scanner. This variation might have influenced certain outcomes. Nevertheless, the use of this software would alleviate the radiologist’s workload, highlighting the increased utility of AI software assistance. Despite these limitations, the findings from this study are promising, particularly for the potential of AI in automating the detection of CMBs in clinical settings.
Conclusions
The automated detection of CMBs presented in this study offers a robust method for their identification and quantification. The automatic CMB detection software proved more sensitive than the radiologists, particularly in detecting smaller CMBs and in cases with a high prevalence of CMBs. The positions of the lesions and overall lesion load were consolidated into an exhaustive report, allowing for post-analysis data review. This study underscores the potential of the automatic CMB detection software based on AI in equipping radiologists with crucial quantitative and spatial information regarding CMBs, thereby optimizing the time-efficiency of data review processes. The demonstrated efficacy of this automatic CMB detection software approach heralds a significant advancement in the clinical handling of CMBs.
Acknowledgments
Funding: This work was supported by
Footnote
Reporting Checklist: The authors have completed the STARD reporting checklist. Available at https://qims.amegroups.com/article/view/10.21037/qims-23-1319/rc
Conflicts of Interest: All authors have completed the ICMJE uniform disclosure form (available at https://qims.amegroups.com/article/view/10.21037/qims-23-1319/coif). Yi Zhong reports that he is a former employee of SpinTech MRI. Miller Fawaz reports that he is a current employee of SpinTech MRI. E.M.H. reports that he is a shareholder of SpinTech MRI. B.W. reports that he is a current employee of Shanghai Zhuyan, Inc. Y.L. reports that this work was supported by the National Natural Science Foundation (81971590) and the Research Fund of Shanghai Fourth People’s Hospital, School of Medicine, Tongji University (SY-XKZT-2019-1003). All authors report that the software used in this study was provided by SpinTech MRI. The authors have no other conflicts of interest to declare.
Ethical Statement: The authors are accountable for all aspects of the work in ensuring that questions related to the accuracy or integrity of any part of the work are appropriately investigated and resolved. The study was conducted in accordance with the Declaration of Helsinki (as revised in 2013). The study was approved by the Institutional Review Board of Shanghai Fourth People’s Hospital institute and individual consent for this retrospective analysis was waived.
Open Access Statement: This is an Open Access article distributed in accordance with the Creative Commons Attribution-NonCommercial-NoDerivs 4.0 International License (CC BY-NC-ND 4.0), which permits the non-commercial replication and distribution of the article with the strict proviso that no changes or edits are made and the original work is properly cited (including links to both the formal publication through the relevant DOI and the license). See: https://creativecommons.org/licenses/by-nc-nd/4.0/.
References
- Li T, Zou Y, Bai P, Li S, Wang H, Chen X, Meng Z, Kang Z, Zhou G. Detecting cerebral microbleeds via deep learning with features enhancement by reusing ground truth. Comput Methods Programs Biomed 2021;204:106051. [Crossref] [PubMed]
- Subramanian K, Utriainen D, Ramasamy DP, Sethi SK, Schweser F, Beaver J, Hagemeier J, Weinstock-Guttman B, Rajagovindan R, Zivadinov R, Haacke EM. Longitudinal Magnetic Resonance Imaging of Cerebral Microbleeds in Multiple Sclerosis Patients. Diagnostics (Basel) 2020;10:942. [Crossref] [PubMed]
- Akoudad S, Aarts N, Noordam R, Ikram MA, Tiemeier H, Hofman A, Stricker BH, Vernooij MW, Visser LE. Antidepressant Use Is Associated With an Increased Risk of Developing Microbleeds. Stroke 2016;47:251-4. [Crossref] [PubMed]
- Wobith M, Mayer C, Belke M, Haag A, Gerstner A, Teepker M, Strzelczyk A, Werner R, Hamer HM, Rosenow F, Menzler K, Knake S. Predictors of New Cerebral Microbleeds in Patients with Antiplatelet Drug Therapy. J Stroke Cerebrovasc Dis 2016;25:1671-7.
- Poels MM, Vernooij MW, Ikram MA, Hofman A, Krestin GP, van der Lugt A, Breteler MM. Prevalence and risk factors of cerebral microbleeds: an update of the Rotterdam scan study. Stroke 2010;41:S103-6. [Crossref] [PubMed]
- Yamada M. Cerebral amyloid angiopathy: emerging concepts. J Stroke 2015;17:17-30. [Crossref] [PubMed]
- Viswanathan A, Greenberg SM. Cerebral amyloid angiopathy in the elderly. Ann Neurol 2011;70:871-80. [Crossref] [PubMed]
- Miwa K, Tanaka M, Okazaki S, Yagita Y, Sakaguchi M, Mochizuki H, Kitagawa K. Multiple or mixed cerebral microbleeds and dementia in patients with vascular risk factors. Neurology 2014;83:646-53. [Crossref] [PubMed]
- Braun H, Schreiber S. Microbleeds in cerebral small vessel disease. Lancet Neurol 2013;12:735-6. [Crossref] [PubMed]
- Pantoni L. Cerebral small vessel disease: from pathogenesis and clinical characteristics to therapeutic challenges. Lancet Neurol 2010;9:689-701. [Crossref] [PubMed]
- Yan S, Jin X, Zhang X, Zhang S, Liebeskind DS, Lou M. Extensive cerebral microbleeds predict parenchymal haemorrhage and poor outcome after intravenous thrombolysis. J Neurol Neurosurg Psychiatry 2015;86:1267-72. [Crossref] [PubMed]
- Gratz PP, El-Koussy M, Hsieh K, von Arx S, Mono ML, Heldner MR, Fischer U, Mattle HP, Zubler C, Schroth G, Gralla J, Arnold M, Jung S. Preexisting cerebral microbleeds on susceptibility-weighted magnetic resonance imaging and post-thrombolysis bleeding risk in 392 patients. Stroke 2014;45:1684-8. [Crossref] [PubMed]
- Wang X, Wei XE, Li MH, Li WB, Zhou YJ, Zhang B, Li YH. Microbleeds on susceptibility-weighted MRI in depressive and non-depressive patients after mild traumatic brain injury. Neurol Sci 2014;35:1533-9. [Crossref] [PubMed]
- Greenberg SM, Vernooij MW, Cordonnier C, Viswanathan A, Al-Shahi Salman R, Warach S, Launer LJ, Van Buchem MA, Breteler MMMicrobleed Study Group. Cerebral microbleeds: a guide to detection and interpretation. Lancet Neurol 2009;8:165-74. [Crossref] [PubMed]
- Romero JR, Preis SR, Beiser A, DeCarli C, Viswanathan A, Martinez-Ramirez S, Kase CS, Wolf PA, Seshadri S. Risk factors, stroke prevention treatments, and prevalence of cerebral microbleeds in the Framingham Heart Study. Stroke 2014;45:1492-4. [Crossref] [PubMed]
- Huang YL, Kuo YS, Tseng YC, Chen DY, Chiu WT, Chen CJ. Susceptibility-weighted MRI in mild traumatic brain injury. Neurology 2015;84:580-5. [Crossref] [PubMed]
- Takashima Y, Mori T, Hashimoto M, Kinukawa N, Uchino A, Yuzuriha T, Yao H. Clinical correlating factors and cognitive function in community-dwelling healthy subjects with cerebral microbleeds. J Stroke Cerebrovasc Dis 2011;20:105-10. [Crossref] [PubMed]
- Werring DJ, Gregoire SM, Cipolotti L. Cerebral microbleeds and vascular cognitive impairment. J Neurol Sci 2010;299:131-5. [Crossref] [PubMed]
- Ayaz M, Boikov AS, Haacke EM, Kido DK, Kirsch WM. Imaging cerebral microbleeds using susceptibility weighted imaging: one step toward detecting vascular dementia. J Magn Reson Imaging 2010;31:142-8. [Crossref] [PubMed]
- Khan MA, Viswanathan A, Greenberg MS. Cerebral microbleeds in relation to cerebral amyloid angiopathy. In: Werring DJ, editor. Cerebral Microbleeds: Pathophysiology to Clinical Practice. Cambridge: Cambridge University Press; 2011:109-16.
- Park JH, Seo SW, Kim C, Kim GH, Noh HJ, Kim ST, et al. Pathogenesis of cerebral microbleeds: In vivo imaging of amyloid and subcortical ischemic small vessel disease in 226 individuals with cognitive impairment. Ann Neurol 2013;73:584-93. [Crossref] [PubMed]
- Mittal S, Wu Z, Neelavalli J, Haacke EM. Susceptibility-weighted imaging: technical aspects and clinical applications, part 2. AJNR Am J Neuroradiol 2009;30:232-52. [Crossref] [PubMed]
- Haacke EM, Mittal S, Wu Z, Neelavalli J, Cheng YC. Susceptibility-weighted imaging: technical aspects and clinical applications, part 1. AJNR Am J Neuroradiol 2009;30:19-30. [Crossref] [PubMed]
- Hsu CC, Watkins TW, Kwan GN, Haacke EM. Susceptibility-Weighted Imaging of Glioma: Update on Current Imaging Status and Future Directions. J Neuroimaging 2016;26:383-90. [Crossref] [PubMed]
- Tong KA, Ashwal S, Holshouser BA, Shutter LA, Herigault G, Haacke EM, Kido DK. Hemorrhagic shearing lesions in children and adolescents with posttraumatic diffuse axonal injury: improved detection and initial results. Radiology 2003;227:332-9. [Crossref] [PubMed]
- Griffin AD, Turtzo LC, Parikh GY, Tolpygo A, Lodato Z, Moses AD, Nair G, Perl DP, Edwards NA, Dardzinski BJ, Armstrong RC, Ray-Chaudhury A, Mitra PP, Latour LL. Traumatic microbleeds suggest vascular injury and predict disability in traumatic brain injury. Brain 2019;142:3550-64. [Crossref] [PubMed]
- Glushakova OY, Johnson D, Hayes RL. Delayed increases in microvascular pathology after experimental traumatic brain injury are associated with prolonged inflammation, blood-brain barrier disruption, and progressive white matter damage. J Neurotrauma 2014;31:1180-93. [Crossref] [PubMed]
- Liu S, Utriainen D, Chai C, Chen Y, Wang L, Sethi SK, Xia S, Haacke EM. Cerebral microbleed detection using Susceptibility Weighted Imaging and deep learning. Neuroimage 2019;198:271-82. [Crossref] [PubMed]
- Chen Y, Villanueva-Meyer JE, Morrison MA, Lupo JM. Toward Automatic Detection of Radiation-Induced Cerebral Microbleeds Using a 3D Deep Residual Network. J Digit Imaging 2019;32:766-72. [Crossref] [PubMed]
- He K, Zhang X, Ren S, Sun J. Deep residual learning for image recognition. Proceedings of the IEEE Conference on Computer Vision and Pattern Recognition; 2016:770-8.
- Loy G, Zelinsky A. A fast radial symmetry transform for detecting points of interest. Computer Vision-ECCV 2002: 7th European Conference on Computer Vision Copenhagen, Denmark, May 28-31, 2002 Proceedings, Part I 7. Springer Berlin Heidelberg; 2002:358-68.
- Gregoire SM, Chaudhary UJ, Brown MM, Yousry TA, Kallis C, Jäger HR, Werring DJ. The Microbleed Anatomical Rating Scale (MARS): reliability of a tool to map brain microbleeds. Neurology 2009;73:1759-66. [Crossref] [PubMed]
- Shoamanesh A, Hart RG, Connolly SJ, Kasner SE, Smith EE, Martí-Fàbregas J, Liu YY, Uchiyama S, Mikulik R, Veltkamp R, O'Donnell MJ, Ntaios G, Muir KW, Field TS, Santo GC, Olavarria V, Mundl H, Lutsep H, Berkowitz SD, Sharma M. Microbleeds and the Effect of Anticoagulation in Patients With Embolic Stroke of Undetermined Source: An Exploratory Analysis of the NAVIGATE ESUS Randomized Clinical Trial. JAMA Neurol 2021;78:11-20. [Crossref] [PubMed]
- Haacke EM, Liu S, Buch S, Zheng W, Wu D, Ye Y. Quantitative susceptibility mapping: current status and future directions. Magn Reson Imaging 2015;33:1-25. [Crossref] [PubMed]
- Chesebro AG, Amarante E, Lao PJ, Meier IB, Mayeux R, Brickman AM. Automated detection of cerebral microbleeds on T2*-weighted MRI. Sci Rep 2021;11:4004. [Crossref] [PubMed]
- Kwa VI, Algra A, Brundel M, Bouvy W, Kappelle LJMICRO Study Group. Microbleeds as a predictor of intracerebral haemorrhage and ischaemic stroke after a TIA or minor ischaemic stroke: a cohort study. BMJ Open 2013;3:e002575. [Crossref] [PubMed]
- Poels MM, Ikram MA, van der Lugt A, Hofman A, Niessen WJ, Krestin GP, Breteler MM, Vernooij MW. Cerebral microbleeds are associated with worse cognitive function: the Rotterdam Scan Study. Neurology 2012;78:326-33. [Crossref] [PubMed]
- Soo YO, Yang SR, Lam WW, Wong A, Fan YH, Leung HH, Chan AY, Leung C, Leung TW, Wong LK. Risk vs benefit of anti-thrombotic therapy in ischaemic stroke patients with cerebral microbleeds. J Neurol 2008;255:1679-86. [Crossref] [PubMed]
- Irimia A, Van Horn JD, Vespa PM. Cerebral microhemorrhages due to traumatic brain injury and their effects on the aging human brain. Neurobiol Aging 2018;66:158-64. [Crossref] [PubMed]