Whole-tumor histogram analysis of synthetic magnetic resonance imaging predicts isocitrate dehydrogenase mutation status in gliomas
Introduction
Gliomas are the most widespread form of malignant primary brain tumor in the central nervous system (CNS) (1). The 2021 World Health Organization (WHO) CNS tumor classification recognizes glioma into astrocytoma isocitrate dehydrogenase (IDH) mutant (IDH-M) WHO grade 2–4 and glioblastoma IDH-wildtype (IDH-W) WHO grade 4, while the genotype for oligodendroglioma (WHO grade 2–3) is defined both by IDH-M and 1p/19q (2). Gliomas with IDH-M have a better prognosis and overall survival rate than IDH-W (3-5). IDH-M glioma patients can benefit more from standard chemotherapy due to the association of IDH mutation with DNA hypermethylation. Additionally, molecular therapies with IDH inhibitors can also be considered (6-8). Therefore, a precise assessment of IDH mutation status is critical for the diagnosis and appropriate treatment of gliomas. Despite the gold standard of IDH mutation status detection relies solely on immunohistochemistry (IHC) staining or genetic sequencing of surgical specimens, these methods are invasive, unable to reflect tumor heterogeneities, and costly for examinations (4). Therefore, an urgent requirement exists for identifying an economical and non-invasive method to determine IDH mutation status in gliomas.
Magnetic resonance imaging (MRI) is an essential preoperative examination for glioma patients. Conventional radiological features, including tumor location, signal characteristics, and tumor border are associated with IDH mutation status in gliomas (9). However, the judgments of diagnostic radiologists impact the accuracy of using these features to identify IDH mutation status. With an increasing rise in clinical demand, advanced MRI techniques for predicting IDH mutation status are rapidly evolving. Quantitative T1, T2, and proton density (PD) values, as fundamental intrinsic properties of MRI physics, can partly reflect tissue pathophysiological changes (10,11). A previous study demonstrated the T2 values derived from T2-mapping method could distinguish IDH mutation status in grade II-III gliomas (12). Nevertheless, conventional mapping methods (including T1-mapping, T2-mapping, etc.) involve acquiring all three relaxation quantitative values separately using different sequences. These methods lead to an increase in scan time and spatial mis-registration among various relaxation quantitative maps, which is a significant barrier to the widespread clinical utilization of conventional mapping methods.
Recently, synthetic MRI, a novel quantitative imaging method equipped with a multi-dynamic multi-echo (MDME) sequence, has been recommended for clinical use (13,14). Synthetic MRI can simultaneously quantify T1, T2, and PD values to generate relaxation quantitative maps (T1, T2, and PD maps) and contrast-weighted maps (including synthetic T1-weighted (T1W) image, synthetic T2-weighted (T2W) image, synthetic PD-weighted image, synthetic T2W fluid-attenuated inversion-recovery (FLAIR) image, etc.) in a single scan within a short examination time (15,16). Compared to conventional mapping methods, synthetic MRI is a more efficient and less time-consuming process. Synthetic MRI has successfully differentiated benign and malignant lesions in rectal and bladder cancer (17,18). In the study conducted by Ge et al., the T1 and PD values were crucial for preoperative gliomas grading (19). Kikuchi et al. reported that the use of quantitative relaxometry with synthetic MRI has the potential to differentiate between astrocytoma and oligodendroglioma, achieving increased sensitivity and objectivity compared to relying on the qualitative T2-FLAIR mismatch sign (20). However, the clinical implications of synthetic MRI for predicting IDH mutation status are still unclear. Histogram analysis is an intuitive texture analysis that is mostly based on statistics (21). This analysis can provide additional quantitative information about the tumor’s microstructure, allowing a more comprehensive assessment of tumor heterogeneity.
The purpose of our study was to investigate the potential value of synthetic MRI metrics combined with whole-tumor histogram analysis for distinguishing IDH mutation status between astrocytoma and glioblastoma, and compare its predictive performance with clinical and radiological features. We present this article in accordance with the STARD reporting checklist (available at https://qims.amegroups.com/article/view/10.21037/qims-23-1288/rc).
Methods
Patients
The study was conducted in accordance with the Declaration of Helsinki (as revised in 2013). The study was approved by the Bioethics Committee of the Lanzhou University Second Hospital (No. 2017A-005) and informed consent was taken from all individual participants. The study was registered under Chinese Clinical Trial Registry (No. ChiCTR2100049967). Between September 2019 and June 2022, a total of 105 continuous patients with primary gliomas from Lanzhou University Second Hospital were evaluated for study eligibility. Inclusion criteria were as follows: (I) confirmation of gliomas via surgical resection or biopsy; (II) acquisition of synthetic MRI scan protocol; (III) availability of basic information and clinicopathological data. Exclusion criteria: (I) confirmed oligodendroglioma based on pathology examination (where the genotype of the tumor was definitively defined by IDH and 1p/19q); (II) prior treatment with radiotherapy, chemotherapy, or chemoradiotherapy before surgical resection; (III) cases involving recurrent gliomas; (IV) poor MR image quality with artifacts affecting the post-processing. An overview of the case selection process is in Figure 1. The flowchart of this study is shown in Figure 2.
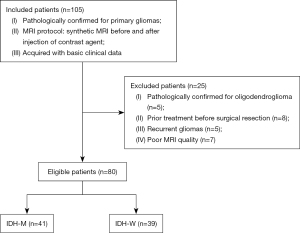
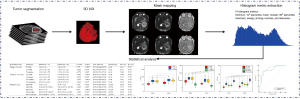
MRI protocol
Patients underwent MRI examinations on a 3.0 T MRI system (SIGNATM Premier, GE Healthcare, Milwaukee, WI, USA) equipped with a 48-channel head-neck coil. Synthetic MRI was performed by using an axial MDME sequence with the following parameters: TR =4,214 ms, TE1 =21 ms, TE2 =108 ms, FOV =24×18 cm2, matrix =320×256, slice thickness =5 mm, spacing =1 mm, slice =20, echo train length =16, bandwidth =22.73 kHz, number of excitations =1, scan time =3 min 39 s. After the first MDME scan, a standard dose of 0.1 mmol/kg contrast agent (Gadodiamide, GE Healthcare, Ireland) was injected at a rate of 4.0 mL/s and then flushed with 20 mL of saline, and the contrast-enhanced MDME acquisition was performed closely after the injection. The scanning parameters of MDME pre- and post-contrast injection were identical.
Histopathologic assessment
The tissue samples of glioma were initially preserved in a 4% formalin solution, prior to paraffin embedding at the hospital’s pathology department. Hematoxylin and eosin were then used to stain 5-µm sections of the paraffin blocks. Identification of IDH mutation status was conducted through IHC staining using a mutation-specific antibody (1:50; internal clone H09; Dianova, Hamburg, Germany) that targets the most common IDH mutation (IDH1 R132H). Gliomas that tested negative for IDH-R132H were subjected to further genetic sequencing, primarily for noncanonical mutations located at the R132 codon in IDH1 and the R172 codon in IDH2. In accordance with the 2021 WHO classification (2), glioma samples that demonstrated evidence of IDH1/2 mutations (1p/19q retained) by means of IHC staining and gene sequencing were classified as IDH-M astrocytoma, while remaining samples lacking such mutations were deemed IDH-W glioblastoma. All histopathological examinations were performed by a senior neuropathologist who remained blind to other results.
Imaging processing
The raw image data of synthetic MRI underwent post-processing using SyMRI 8.0 software (SyntheticMR, Linköping, Sweden), which led to the generation of quantitative maps [T1map, T2map, and PDmap, as well as post-contrast T1map (T1map+C), T2map (T2map+C), and PDmap (PDmap+C)] and contrast-weighted maps [synthetic T1W FLAIR, synthetic T2W, synthetic T2W FLAIR, and post-contrast synthetic T1W FLAIR (synthetic T1FLAIR+C) image]. The images were subjected to registration using the SPM12 toolbox (http://www.fil.ion.ucl.ac.uk/spm/) implemented in MATLAB R2014b software (Math Woks, Natick, Massachusetts, USA), thereby aligning the T1map+C, T2map+C, PDmap+C, and synthetic T1FLAIR+C image to the synthetic T2W FLAIR image on a rigid basis. Next, a neuroradiologist (X.G., with 4 years of experience) manually segmented every tumor slice to obtain volume of interests (VOIs) using ITK-SNAP software (v. 3.8.0, http://www.itksnap.org). To delimit the VOIs, we established the tumor boundaries of no enhancement in synthetic T2W FLAIR images (Figure 3), while tumor boundaries with enhancement were determined in synthetic T1FLAIR+C images (Figure 4). Subsequently, we applied the VOIs of the tumor core (combined non-enhancing and enhancing tumor components) to all quantitative maps. From these maps, we extracted histogram metrics s including minimum, 10th, mean, median, 90th, maximum, energy, entropy, kurtosis, and skewness using Pyradiomics, an open-source Python package (https://github.com/Radiomics/pyradiomics). The quantitative metrics derived from synthetic MRI after injection of contrast agent were defined as post-contrast T1 (cT1), post-contrast T2 (cT2), and post-contrast PD (cPD). To evaluate the interobserver reliability of histogram metrics, another neuroradiologist (W.H., with 7 years of experience) undertook the VOIs segmentation again independently. Two neuroradiologists who were blinded to the pathological results measured each metric and the average of each metric they measured was taken for further analysis. The histogram metrics that extracted from these VOIs were assessed with the interclass correlation coefficient (ICC; ICC <0.40, poor; 0.40≤ ICC <0.60, moderate; 0.60≤ ICC <0.80, good; ICC ≥0.80, excellent).
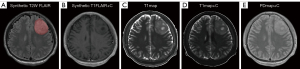
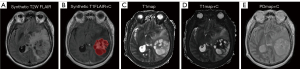
Development of the clinicoradiological and combined model
For each patient, the basic clinical features on age and gender were collected. The radiological features were retrospectively evaluated by a neuroradiologist (Y.B.) with over 10 years of experience. The neuroradiologist was blinded to the pathological results throughout this process. The features evaluated included tumor location (frontal lobe, parietal lobe, temporal lobe, occipital lobe, insular lobe, multi-lobe, others), tumor size (longest diameter), tumor border (sharp/indistinct), enhancement style (no, ring, nodular, irregular), enhancement degree (no, slight, obvious), edema degree (longest diameter, defined as a non-enhancing area on synthetic T1FLAIR+C images and hyperintense signal outside the tumoral solid portion on synthetic T2W or synthetic T2W FLAIR images. Typically, the edema region in non-enhanced IDH-W glioblastoma is determined using synthetic T2W FLAIR images (Figure S1), hemorrhage (no/yes, defined as the presence of a hyperintense signal within the tumor area on synthetic T1W FLAIR images), cystic and necrosis (no, ≤25%, 25–50%, ≥50%), signal characteristics (homogeneous/heterogeneous), as well as cross midline growth (no/yes) (22). The tumors in all of the included patients were solitary. To assess the interobserver reliability of radiological features, another neuroradiologist (G.L., with 15 years of experience) conducted an independent evaluation. The agreement between observers was determined using the ICC.
Univariate analysis was used to compare clinical, radiological, and histogram metrics between IDH-M and IDH-W groups. The clinicoradiological model was developed by multivariate logistic regression analysis. We also created the combined model that integrated independent risk factors from clinical, radiological, and histogram metrics to distinguish IDH mutation status.
Statistical analysis
All statistical analyses were conducted using SPSS (v.26, SPSS Inc., Chicago, IL, USA), MedCalc (v.20.009, MedCalc Inc., Ostend, Belgium), and OriginPro 2018C software (v.b9.5.1.195, OriginLab Corporation, Northampton, MA, USA). Levene test was utilized to test for homogeneity of variance, while Shapiro-Wilk test was conducted to assess the normality of data distribution. The mean ± standard deviation was reported for normally distributed continuous data, while the median (interquartile range) was used for non-normal continuous data. Categorical data were presented as percentages [n (%)]. Continuous data analysis was performed using Student t-test, while Mann-Whitney U test was employed for variables with unequal variance or skewed distributions. Chi-squared test and Fisher’s exact test were used to compare categorical variables. Multivariate logistic regression analysis using forward LR was employed to determine the risk factors between IDH-M and IDH-W groups. Subsequently, receiver operating characteristic (ROC) curves were plotted to ascertain area under the curve (AUC), cutoff values, sensitivity (Sen.), specificity (Spe.), positive predictive value (PPV), and negative predictive value (NPV) for significant results. Finally, Delong test was utilized to compare the AUCs. Statistical tests were two-tailed with P<0.05.
Results
Patients’ clinical and radiological features
Table 1 illustrates the clinical and radiological features of the 80 patients enrolled in this study, including 41 cases of IDH-M astrocytoma (WHO grade 2–4) and 39 cases of IDH-W glioblastoma (WHO grade 4). The cohort comprised of 49 males and 31 females with a mean age of 50.88±12.39 years (ranging from 26 to 76 years). Remarkably, patients with IDH mutation were comparatively younger (P<0.001) and demonstrated less edema degree (P=0.005) than those without IDH mutation. Furthermore, statistically significant differences in tumor border, enhancement style, enhancement degree, cystic and necrosis, as well as signal characteristics were observed between IDH-M and IDH-W groups (all P<0.05). Nonetheless, IDH mutation status exhibited no correlation with oxygen 6-methylguanine-DNA methyltransferase (MGMT) and other radiological features (all P>0.05).
Table 1
Features | Univariate analysis | Multivariable logistic regression analysis | |||||||
---|---|---|---|---|---|---|---|---|---|
IDH-M (N=41) | IDH-W (N=39) | χ2/Z value | P value | β coefficient | OR (95% CI) | P value | |||
Gender, n (%) | 0.941 | 0.366# | – | – | – | ||||
Male | 23 (56.10) | 26 (66.67) | |||||||
Female | 18 (43.90) | 13 (33.33) | |||||||
MGMT promoter, n (%) | −0.821 | 0.389# | |||||||
Methylation | 14 (34.15) | 17 (43.59) | |||||||
Unmethylation | 27 (65.85) | 22 (56.41) | |||||||
Age (years) | 47.00 (37.00, 54.00) | 56.00 (51.00, 66.00) | −4.320 | <0.001* | −0.110 | 0.896 (0.848–0.946) | 0.003 | ||
Tumor location, n (%) | 7.045 | 0.296# | – | – | – | ||||
Frontal lobe | 20 (48.78) | 12 (30.77) | |||||||
Parietal lobe | 2 (4.88) | 4 (10.26) | |||||||
Temporal lobe | 4 (9.75) | 8 (20.51) | |||||||
Occipital lobe | 2 (4.88) | 4 (10.26) | |||||||
Insular lobe | 2 (4.88) | 1 (2.56) | |||||||
Multi-lobe | 11 (26.83) | 8 (20.51) | |||||||
Others | 0 (0.00) | 2 (5.13) | |||||||
Tumor size (cm) | 5.23 (4.15, 6.53) | 4.82 (3.58, 6.01) | −1.035 | 0.301* | – | – | – | ||
Tumor border, n (%) | 5.070 | 0.038# | – | – | 0.224 | ||||
Sharp | 30 (73.17) | 36 (92.31) | |||||||
Indistinct | 11 (26.83) | 3 (7.69) | |||||||
Enhancement style, n (%) | 17.236 | <0.001# | |||||||
No | 20 (48.78) | 5 (12.82) | – | – (Ref.) | 0.295 | ||||
Ring enhancement | 3 (7.32) | 15 (38.46) | – | – | 0.133 | ||||
Nodular enhancement | 10 (24.39) | 8 (20.51) | – | – | 0.765 | ||||
Irregular reinforcement | 8 (19.51) | 11 (28.21) | |||||||
Enhancement degree, n (%) | 19.764 | <0.001# | |||||||
No | 20 (48.78) | 5 (12.82) | – | – (Ref.) | 0.033 | ||||
Slight | 7 (17.07) | 2 (5.13) | 1.503 | 4.496 (1.242–16.278) | 0.022 | ||||
Obvious | 14 (34.15) | 32 (82.05) | 1.610 | 5.003 (0.794–31.534) | 0.087 | ||||
Edema degree (cm) | 0.00 (0.00, 2.10) | 1.75 (0.73, 3.24) | −2.819 | 0.005* | – | – | 0.312 | ||
Hemorrhage, n (%) | 1.208 | 0.272# | – | – | – | ||||
No | 38 (92.68) | 32 (82.05) | |||||||
Yes | 3 (7.32) | 7 (17.95) | |||||||
Cystic and necrosis, n (%) | 10.616 | 0.013# | |||||||
No | 10 (24.39) | 3 (7.69) | – | – | 0.753 | ||||
<25% | 17 (41.47) | 13 (33.33) | – | – (Ref.) | 0.875 | ||||
25–50% | 7 (17.07) | 4 (10.26) | – | – | 0.460 | ||||
>50% | 7 (17.07) | 19 (48.72) | – | – | 0.668 | ||||
Signal characteristics, n (%) | 5.070 | 0.038# | – | – | 0.676 | ||||
Homogeneous | 11 (26.83) | 3 (7.69) | |||||||
Heterogeneous | 30 (73.17) | 36 (92.31) | |||||||
Cross midline growth, n (%) | 0.130 | 0.718# | – | – | – | ||||
No | 37 (90.24) | 37 (94.87) | |||||||
Yes | 4 (9.76) | 2 (5.13) |
Continuous quantitative variables as median (first quartile and third quartile). *, P value represents the comparison results of IDH-M and IDH-W gliomas using the t-test analysis; #, P value represents the comparison results of IDH-M and IDH-W gliomas using the non-parametric Mann-Whitney U-test. IDH-M, isocitrate dehydrogenase mutant; IDH-W, IDH-wildtype; OR, odds ratio; CI, confidence interval; MGMT, Oxygen 6-methylguanine-DNA methyltransferase; Ref., reference.
Interobserver reproducibility
In this study, the ICC for synthetic MRI histogram metrics ranged from 0.88 to 0.95, reflecting excellent interobserver agreement. The ICC ranges for the radiological features were all greater than 0.95, demonstrating a strong consistency among observers.
Histogram analysis for differentiating IDH-M and IDH-W gliomas
Figures 3 and 4 depict typical images of IDH-M and IDH-W gliomas, respectively. Table 2 and Table S1 show the histogram metrics with statistically significant differences between the two groups along with their ROC analysis results. Among the T1map-derived metrics, IDH-M astrocytoma exhibited significantly lower values of T1-10th, T1-mean, and T1-median compared to those in IDH-W glioblastoma (P<0.001, P=0.043, and P=0.048, respectively). T1-10th had the AUC of 0.735 for identifying IDH-M astrocytoma, followed by T1-mean (AUC =0.632) and T1-median (AUC =0.629). Regarding the T1map+C-derived metrics, IDH-M astrocytoma presented higher values of cT1-10th, cT1-mean, cT1-median, and cT1-minium than those IDH1-W glioblastoma (P<0.001, P=0.002, P<0.001, and P=0.001, respectively). cT1-10th had the largest AUC of 0.819 for identifying IDH-M astrocytoma, followed by cT1-median (AUC =0.730), cT1-minimum (AUC =0.719), and cT1-mean (AUC =0.699). In PDmap+C-derived metrics, cPD-10th, cPD-90th, cPD-mean, cPD-median, and cPD-maximum were significantly lower in IDH-M astrocytoma than in IDH-W glioblastoma (P=0.002, P=0.002, P<0.001, P=0.002, and P=0.002, respectively). The cPD-mean had the largest AUC of 0.792 for identifying IDH-M astrocytoma, followed by cPD-10th (AUC =0.704), cPD-maximum (AUC =0.703), cPD-median (AUC =0.701), and cPD-minimum (AUC =0.700). Moreover, the values of energy, entropy, kurtosis, and skewness derived from the aforementioned three quantitative maps were not significantly different between groups (all P>0.05). Overall, other quantitative maps such as T2map, PDmap, and T2map+C did not exhibit any statistically significant differences in their histogram metrics between IDH-M and IDH-W groups (all P>0.05).
Table 2
Quantitative maps | Parameters | IDH-M (N=41) | IDH-W (N=39) | t/Z value | P value | AUC (95% CI) | Cut-off value | Sen. (%) | Spe. (%) |
---|---|---|---|---|---|---|---|---|---|
T1map (×103 ms) | T1-10th | 1.06±0.14 | 1.20±0.20 | 3.375 | <0.001* | 0.735 (0.624–0.827) | ≤1.09 | 63.41 | 79.49 |
T1-mean | 1.45 (1.32, 1.60) | 1.58 (1.41, 1.71) | −2.026 | 0.043# | 0.632 (0.516–0.737) | ≤1.52 | 63.41 | 64.10 | |
T1-median | 1.38 (1.30, 1.55) | 1.52 (1.39, 1.60) | −1.978 | 0.048# | 0.629 (0.513–0.734) | ≤1.40 | 53.66 | 76.92 | |
T1map+C (×103 ms) | cT1-10th | 0.87 (0.73, 1.04) | 0.54 (0.48, 0.64) | −4.845 | <0.001# | 0.819 (0.717–0.896) | >0.68 | 78.05 | 84.62 |
cT1-mean | 1.31 (1.11, 1.47) | 0.98 (0.85, 1.20) | −3.056 | 0.002# | 0.699 (0.586–0.796) | >1.16 | 73.17 | 71.79 | |
cT1-median | 1.28 (1.07, 1.46) | 0.81 (0.76, 1.16) | −3.547 | <0.001# | 0.730 (0.620–0.824) | >1.06 | 75.61 | 71.79 | |
cT1-minimum | 0.43 (0.31, 0.61) | 0.29 (0.17, 0.37) | −3.364 | 0.001# | 0.719 (0.607–0.813) | >0.38 | 58.54 | 84.62 | |
PDmap+C (pu) | cPD-10th | 75.29±5.91 | 79.28±5.35 | 3.157 | 0.002* | 0.704 (0.591–0.801) | ≤76.90 | 68.29 | 66.67 |
cPD-90th | 93.60 (89.25, 99.90) |
101.20 (95.18, 103.58) |
−3.085 | 0.002# | 0.700 (0.588–0.798) | ≤97.50 | 73.17 | 71.79 | |
cPD-mean | 84.79±5.45 | 90.56±5.04 | 4.914 | <0.001* | 0.792 (0.687–0.875) | ≤87.28 | 78.05 | 76.92 | |
cPD-median | 84.90 (83.05±88.65) | 89.93 (87.10±94.40) | −3.090 | 0.002# | 0.701 (0.588–0.798) | ≤86.90 | 68.29 | 76.92 | |
cPD-maximum | 109.20 (106.40, 117.95) |
118.60 (112.25, 124.33) |
−3.124 | 0.002# | 0.703 (0.590–0.800) | ≤109.30 | 58.54 | 82.05 |
Continuous quantitative variables as mean ± standard deviation or median (first quartile and third quartile). *, P value represents the comparison results of IDH-M and IDH-W gliomas using the t-test analysis; #, P value represents the comparison results of IDH-M and IDH-W gliomas using the non-parametric Mann-Whitney U-test. IDH-M, isocitrate dehydrogenase mutant; IDH-W, isocitrate dehydrogenase wildtype; ROC, receiver operating characteristic; T1map+C, post-contrast T1map; PDmap+C, post-contrast PDmap; AUC, area under the curve; CI, confidence interval; Sen., sensitivity; Spe., specificity; 10th, 10th percentile; 90th, 90th percentile; cT1, post-contrast T1; cPD, post-contrast PD.
Development of the clinicoradiological and combined model
The study observed statistically significant differences in age, tumor border, enhancement style, enhancement degree, edema degree, cystic and necrosis, as well as signal characteristics between groups. The multivariate logistic analysis selected age and enhancement style to develop the clinicoradiological model. Table 1 summarizes the results of the univariate and multivariate analyses of clinical and radiological features between two IDH genotypes in gliomas.
We included only the clinical, radiological, and histogram metrics that had a P value lower than 0.05 in the univariate analysis between IDH-M and IDH-W groups in the multivariate logistic analysis. The multivariate logistic regression identified T1-10th [odds ratio (OR) =0.996], cT1-10th (OR =1.004), and age (OR =0.936) as significant predictors of IDH-M astrocytoma, as shown in Table 3. The combination of the above independent predictors established the combined model.
Table 3
Variables | β coefficient | OR (95% CI) | P value |
---|---|---|---|
T1-10th (pre 1 ms increase) | −0.004 | 0.996 (0.992–0.999) | 0.042 |
T1-mean | – | – | 0.264 |
T1-median | – | – | 0.391 |
cT1-10th (pre 1 ms increase) | 0.004 | 1.004 (1.002–1.007) | 0.002 |
cT1-mean | – | – | 0.476 |
cT1-median | – | – | 0.822 |
cT1-minimum | – | – | 0.093 |
cPD-10th | – | – | 0.716 |
cPD-90th | – | – | 0.136 |
cPD-mean | – | – | 0.781 |
cPD-median | – | – | 0.238 |
cPD-maximum | – | – | 0.392 |
Age (pre 1 year) | −0.066 | 0.936 (0.878–0.998) | 0.044 |
Edema degree | – | – | 0.073 |
Tumor border | – | – | 0.353 |
Sharp | |||
Indistinct | |||
Enhancement style | |||
No | – | – | 0.251 |
Ring enhancement | – | – | 0.233 |
Nodular enhancement | – | – | 0.457 |
Irregular reinforcement | – | – (Ref.) | 0.163 |
Enhancement degree | |||
No | – | – | 0.219 |
Slight | – | – | 0.17 |
Obvious | – | – (Ref.) | 0.926 |
Cystic and necrosis | |||
No | – | – | 0.949 |
<25% | – | – (Ref.) | 0.919 |
25–50% | – | – | 0.769 |
>50% | – | – | 0.501 |
Signal characteristics | – | – | 0.795 |
Homogeneous | |||
Heterogeneous |
OR, odds ratio; CI, confidence interval; 10th, 10th percentile; 90th, 90th percentile; cT1, post-contrast T1; cPD, post-contrast PD; Ref., reference.
Diagnostic performance of models for predicting IDH mutation status in gliomas
Table 4 presents the diagnostic performance for age, enhancement degree, and prediction models. Of the predictors, the clinicoradiological model for IDH mutation status prediction achieved an AUC of 0.822, which is superior to enhancement degree (AUC =0.741, P=0.017 by DeLong test), but not significantly better than age (AUC =0.780, P=0.158 by DeLong test). Table 4 and Figure 5 show that the combined model has the best diagnostic performance (AUC =0.872) which can achieve 75.61% Sen., 89.74% Spe., 88.57% PPV, and 77.78% NPV among all models and variables, followed by the clinicoradiological model (AUC =0.822, P=0.035 by DeLong test).
Table 4
Models | Variables | AUC (95% CI) | Cutoff value | Sen. (%) | Spe. (%) | PPV (%) | NPV (%) |
---|---|---|---|---|---|---|---|
– | Age | 0.780 (0.674–0.865) | ≤49.00 (years) | 63.41 (26/41) | 82.05 (32/39) | 78.79 (26/33) | 68.09 (32/47) |
– | Enhancement degree | 0.741 (0.631–0.833) | ≤1.00 | 58.54 (24/41) | 82.05 (32/39) | 81.82 (27/33) | 70.21 (33/47) |
Clinicoradiological | Age + enhancement degree | 0.822 (0.870–0.898) | >0.47 | 75.61 (31/41) | 84.62 (33/39) | 83.78 (31/37) | 76.74 (33/43) |
Combined | T1-10th + cT1-10th + age | 0.872 (0.778–0.936) | >0.57 | 75.61 (31/41) | 89.74 (35/39) | 88.57 (31/35) | 77.78 (35/45) |
AUC, area under the curve; CI, confidence interval; Sen., sensitivity; Spe., specificity; PPV, positive predictive value; NPV, negative predictive value; Enhancement degree, enhancement degree of tumor (no, slight, obvious); 10th, 10th percentile; cT1, post-contrast T1.
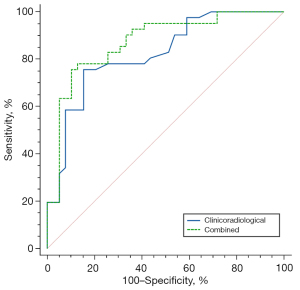
Discussion
Investigating an effective and noninvasive technique for distinguishing IDH mutation status between astrocytoma and glioblastoma is crucial for tailoring treatment and prognosis evaluation. Our study demonstrated that the histogram analysis of synthetic MRI can be used as a useful means to differentiate IDH mutation status, with T1 (10th, mean, and median), cT1 (10th, mean, median, and minimum), and cPD (10th, 90th, mean, median, and maximum) derived from the synthetic MRI histogram metrics having moderate discrimination. Our results showed that the combined model (T1-10th + cT1-10th + age) had the best performance compared to the clinicoradiological model (age + enhancement style).
Various IDH genotypes of glioma have unique microstructures, such as tissue water and fat content, macromolecule concentration, and hydration state, which affect differences in relaxometry (T1 & T2) and PD. In this study, we identified that the T1 histogram metrics (T1-10th, T1-mean, and T1-median) of IDH-W glioblastoma were significantly higher than those of IDH-M astrocytoma. Our findings are consistent with prior research on glioma grading indicating that increased malignancy of higher-grade tumors generally correlates with higher T1 values (19). The higher T1 values in IDH-W tumors could be due to tumor growth exceeding blood supply capacity, which causes intra-tumoral necrosis or cystic degeneration (23). Kern et al. reported that the T2 values in the central region of IDH-M gliomas were significantly different from that of IDH-W (P=0.0037), which is different from us study (12). This is because Kern et al.’s study only included solid enhancing portions that had been analyzed. However, our segmentation also included tumoral cysts and necrosis, which may have an impact on the T2 measurements.
Furthermore, we investigated the potential of synthetic MRI with contrast agent for distinguishing between different IDH subtypes of glioma. This method enables the quantification of tumor enhancement and provides additional information, exceeding the capabilities of conventional scanning. Analysis of The Cancer Genome Atlas database revealed that IDH-W glioblastoma express significantly higher level of vascular endothelial growth factor (VEGF) in comparison to their IDH-M counterparts (24). The production of VEGF by IDH-W glioblastoma leads to the development of malformed, tangled capillary networks with high permeability, facilitating a massive contrast material influx through a disrupted blood-brain barrier and into the tumor. As such, IDH-W glioblastoma present lower post-contrast T1 values (cT1-10th, cT1-mean, cT1-median and cT1-minimum). PD is a measure of the water content in tissues and is commonly utilized in the bone and brain imaging (25). Ge et al. (19) found significant differences in PD values derived from synthetic MRI between low- and high-grade gliomas [82.96±2.46 vs. 86.19±2.33 (pu), P<0.001]. Our results indicated that IDH-W glioblastoma display elevated cPD histogram metrics, including 10th, 90th, mean, median and maximum values, when compared to IDH-M astrocytoma—this observation is noteworthy as it remains largely unexplored in the literature. This finding suggests that the cPD could identify gadolinium-based contrast agents’ aggregation in IDH-W glioblastoma, contradicting the T1-shortening effect. Nonetheless, further investigations are warranted to examine the alterations in PD values obtained through pre- and post-contrast synthetic MRI.
Recent studies have demonstrated that the quality of synthetic contrast-weighted maps was adequate for routine neuroimaging protocol (26-28). In this study, the MDME sequence synthetically generated multiple contrast-weighted maps to evaluate radiological features of gliomas. The multivariate analysis in our study revealed that enhancement degree was an independent risk factor for IDH mutation in the radiological assessment, confirming the significantly enhanced features of most IDH-W glioblastoma which was mutually corroborated by their lower cT1 histogram metrics. We also observed that tumor border, enhancement style, edema degree, cystic and necrosis, as well as signal characteristics were related to IDH mutation status, without being independent risk factors of IDH mutation. In clinical practice, conventional contrast-weighted maps need to be acquired separately, whereas multiple contrast-weighted maps derived from synthetic MRI meet the preliminary diagnosis needs of glioma patients within a feasible timeframe.
In the multiple logistic regression analysis of all variates, T1-10th and cT1-10th were identified as independent risk factors for IDH mutation. These variables, in turn, revealed that various histogram metrics derived from quantitative maps could distinguish IDH mutation status. The combined model, which incorporated T1-10th, cT1-10th, and age, outperformed the clinicoradiological model. This result indicated that the histogram metrics derived from synthetic MRI were better indicators of potential tumor heterogeneity and aggressive tumor biology, confirming previous findings in cancers outside the brain (29,30). Notably, age demonstrated significant predictive strength and can be easily obtained preoperatively (31), making its inclusion in the combined model a common strategy.
Ohmura et al. observed that integrating the T2-FLAIR mismatch sign with 11C-methionine (MET)-PET enhances the diagnostic accuracy for identifying the IDH mutation status (32). However, MET-PET scans are cost-prohibitive, and not all patients are subjected to this diagnostic procedure. Amide proton transfer (APT) imaging, a specialized form of chemical exchange saturation transfer (CEST) imaging, is an advanced non-invasive imaging technique. In a study conducted by Joo et al., it was found that the CEST-APT signals of IDH-W were significantly higher in comparison to IDH-M (33). Considering the constrained spatial resolution of APT imaging, the authors utilized the “hotspot” method for joint registration. Synthetic MRI is an advanced quantitative imaging technique widely used for various tumor-related analyses, including grading, differential diagnosis, and prognostic evaluation, due to its rapid acquisition and data quantification (18,19,34-36). Unlike CEST-APT and MET-PET imaging techniques, synthetic MRI offers cost-effectiveness and eliminates the need to address potential misregistration between contrast-weighted images and quantification maps due to motion during acquisition. These advantages arise from obtaining all the required images and maps from a single scan, using the same raw data. Furthermore, “hotspot” analysis alone fails to provide a comprehensive assessment of the spatial heterogeneity of tumor histological features. To better reflect the heterogeneity of lesion, the histogram analysis of whole-tumor may provide a more objective measure. Our study concluded that T1 and cT1 10th percentile values were independent risk factors for IDH mutation. This type of histogram metric can sometimes be more effective than the maximum or mean values, as it is less influenced by random statistical fluctuations (21).
This study has several limitations. Firstly, it was a single-center study on a small sample of gliomas and did not undergo multicenter data verification. Secondly, the focus of this study was solely on determining the IDH mutation status differences between astrocytoma and glioblastoma. However, future investigations will be expanded to include the analysis of oligodendroglioma as well. As the number of cases increase, further investigation should explore the relationship between imaging features and meta-gene abnormal expressions, such as MGMT, TERT, and ATRX. Lastly, the study relied only on histogram metrics with good repeatability, and it would be beneficial to explore artificial intelligence techniques such as radiomics or deep learning in future studies to further explore the full potential of synthetic MRI.
Conclusions
The histogram metrics derived from synthetic MRI are capable of quantifying the distribution of whole-tumor relaxometry and PD, which associated with IDH mutation in gliomas. Combining T1-10th, cT1-10th, and age yields better predictive performance in distinguishing IDH mutation status between astrocytoma and glioblastoma.
Acknowledgments
Funding: This work was supported by
Footnote
Reporting Checklist: The authors have completed the STARD reporting checklist. Available at https://qims.amegroups.com/article/view/10.21037/qims-23-1288/rc
Conflicts of Interest: All authors have completed the ICMJE uniform disclosure form (available at https://qims.amegroups.com/article/view/10.21037/qims-23-1288/coif). Y.X. and M.L., employees of GE healthcare, provided MRI technical guidance and writing guidance for this paper. The other authors have no conflicts of interest to declare.
Ethical Statement: The authors are accountable for all aspects of the work in ensuring that questions related to the accuracy or integrity of any part of the work are appropriately investigated and resolved. The study was conducted in accordance with the Declaration of Helsinki (as revised in 2013). The study was approved by the Bioethics Committee of the Lanzhou University Second Hospital (No. 2017A-005) and informed consent was taken from all individual participants.
Open Access Statement: This is an Open Access article distributed in accordance with the Creative Commons Attribution-NonCommercial-NoDerivs 4.0 International License (CC BY-NC-ND 4.0), which permits the non-commercial replication and distribution of the article with the strict proviso that no changes or edits are made and the original work is properly cited (including links to both the formal publication through the relevant DOI and the license). See: https://creativecommons.org/licenses/by-nc-nd/4.0/.
References
- Gittleman H, Sloan AE, Barnholtz-Sloan JS. An independently validated survival nomogram for lower-grade glioma. Neuro Oncol 2020;22:665-74. [Crossref] [PubMed]
- Louis DN, Perry A, Wesseling P, Brat DJ, Cree IA, Figarella-Branger D, Hawkins C, Ng HK, Pfister SM, Reifenberger G, Soffietti R, von Deimling A, Ellison DW. The 2021 WHO Classification of Tumors of the Central Nervous System: a summary. Neuro Oncol 2021;23:1231-51. [Crossref] [PubMed]
- van den Bent MJ, Tesileanu CMS, Wick W, Sanson M, Brandes AA, Clement PM, et al. Adjuvant and concurrent temozolomide for 1p/19q non-co-deleted anaplastic glioma (CATNON; EORTC study 26053-22054): second interim analysis of a randomised, open-label, phase 3 study. Lancet Oncol 2021;22:813-23. [Crossref] [PubMed]
- Weller M, van den Bent M, Preusser M, Le Rhun E, Tonn JC, Minniti G, et al. EANO guidelines on the diagnosis and treatment of diffuse gliomas of adulthood. Nat Rev Clin Oncol 2021;18:170-86. [Crossref] [PubMed]
- Brat DJ, Verhaak RG, Aldape KD, Yung WK, Salama SR, et al. Comprehensive, Integrative Genomic Analysis of Diffuse Lower-Grade Gliomas. N Engl J Med 2015;372:2481-98. [Crossref] [PubMed]
- Kizilbash SH, Giannini C, Voss JS, Decker PA, Jenkins RB, Hardie J, Laack NN, Parney IF, Uhm JH, Buckner JC. The impact of concurrent temozolomide with adjuvant radiation and IDH mutation status among patients with anaplastic astrocytoma. J Neurooncol 2014;120:85-93. [Crossref] [PubMed]
- Grasso CS, Tang Y, Truffaux N, Berlow NE, Liu L, Debily MA, et al. Functionally defined therapeutic targets in diffuse intrinsic pontine glioma. Nat Med 2015;21:555-9. [Crossref] [PubMed]
- Rohle D, Popovici-Muller J, Palaskas N, Turcan S, Grommes C, Campos C, et al. An inhibitor of mutant IDH1 delays growth and promotes differentiation of glioma cells. Science 2013;340:626-30. [Crossref] [PubMed]
- Qi S, Yu L, Li H, Ou Y, Qiu X, Ding Y, Han H, Zhang X. Isocitrate dehydrogenase mutation is associated with tumor location and magnetic resonance imaging characteristics in astrocytic neoplasms. Oncol Lett 2014;7:1895-902. [Crossref] [PubMed]
- Jara H, Sakai O, Farrher E, Oros-Peusquens AM, Shah NJ, Alsop DC, Keenan KE. Primary Multiparametric Quantitative Brain MRI: State-of-the-Art Relaxometric and Proton Density Mapping Techniques. Radiology 2022;305:5-18. [Crossref] [PubMed]
- Hwang KP, Fujita S, Synthetic MR. Physical principles, clinical implementation, and new developments. Med Phys 2022;49:4861-74. [Crossref] [PubMed]
- Kern M, Auer TA, Picht T, Misch M, Wiener E. T2 mapping of molecular subtypes of WHO grade II/III gliomas. BMC Neurol 2020;20:8. [Crossref] [PubMed]
- Huo M, Ye J, Dong Z, Cai H, Wang M, Yin G, Qian L, Li ZP, Zhong B, Feng ST. Quantification of brown adipose tissue in vivo using synthetic magnetic resonance imaging: an experimental study with mice model. Quant Imaging Med Surg 2022;12:526-38. [Crossref] [PubMed]
- Ma L, Lian S, Liu H, Meng T, Zeng W, Zhong R, Zhong L, Xie C. Diagnostic performance of synthetic magnetic resonance imaging in the prognostic evaluation of rectal cancer. Quant Imaging Med Surg 2022;12:3580-91. [Crossref] [PubMed]
- Fujita S, Hagiwara A, Aoki S, Abe O. Synthetic MRI and MR fingerprinting in routine neuroimaging protocol: What’s the next step? J Neuroradiol. 2020;47:134-135. [Crossref] [PubMed]
- Liu S, Meng T, Russo C, Di Ieva A, Berkovsky S, Peng L, Dou W, Qian L. Brain volumetric and fractal analysis of synthetic MRI: A comparative study with conventional 3D T1-weighted images. Eur J Radiol 2021;141:109782. [Crossref] [PubMed]
- Zhao L, Liang M, Xie L, Yang Y, Zhang H, Zhao X. Prediction of pathological prognostic factors of rectal cancer by relaxation maps from synthetic magnetic resonance imaging. Eur J Radiol 2021;138:109658. [Crossref] [PubMed]
- Cai Q, Wen Z, Huang Y, Li M, Ouyang L, Ling J, Qian L, Guo Y, Wang H. Investigation of Synthetic Magnetic Resonance Imaging Applied in the Evaluation of the Tumor Grade of Bladder Cancer. J Magn Reson Imaging 2021;54:1989-97. [Crossref] [PubMed]
- Ge X, Wang M, Ma H, Zhu K, Wei X, Li M, Zhai X, Shen Y, Huang X, Hou M, Liu W, Wang M, Wang X. Investigated diagnostic value of synthetic relaxometry, three-dimensional pseudo-continuous arterial spin labelling and diffusion-weighted imaging in the grading of glioma. Magn Reson Imaging 2022;86:20-7. [Crossref] [PubMed]
- Kikuchi K, Togao O, Yamashita K, Momosaka D, Kikuchi Y, Kuga D, Hata N, Mizoguchi M, Yamamoto H, Iwaki T, Hiwatashi A, Ishigami K. Quantitative relaxometry using synthetic MRI could be better than T2-FLAIR mismatch sign for differentiation of IDH-mutant gliomas: a pilot study. Sci Rep 2022;12:9197. [Crossref] [PubMed]
- Park KJ, Kim HS, Park JE, Shim WH, Kim SJ, Smith SA. Added value of amide proton transfer imaging to conventional and perfusion MR imaging for evaluating the treatment response of newly diagnosed glioblastoma. Eur Radiol 2016;26:4390-403. [Crossref] [PubMed]
- Tan Y, Zhang ST, Wei JW, Dong D, Wang XC, Yang GQ, Tian J, Zhang H. A radiomics nomogram may improve the prediction of IDH genotype for astrocytoma before surgery. Eur Radiol 2019;29:3325-37. [Crossref] [PubMed]
- Vinay K, Abbas AK, Nelson F, Richard M. Robbins Basic Pathology. eighth ed., Philadelphia: Saunders Elsevier, 2007.
- Sun C, Zhao Y, Shi J, Zhang J, Yuan Y, Gu Y, et al. Isocitrate dehydrogenase1 mutation reduces the pericyte coverage of microvessels in astrocytic tumours. J Neurooncol 2019;143:187-96.
- Gracien RM, Reitz SC, Hof SM, Fleischer V, Zimmermann H, Droby A, Steinmetz H, Zipp F, Deichmann R, Klein JC. Changes and variability of proton density and T1 relaxation times in early multiple sclerosis: MRI markers of neuronal damage in the cerebral cortex. Eur Radiol 2016;26:2578-86. [Crossref] [PubMed]
- Konar AS, Paudyal R, Shah AD, Fung M, Banerjee S, Dave A, Lee N, Hatzoglou V, Shukla-Dave A. Qualitative and Quantitative Performance of Magnetic Resonance Image Compilation (MAGiC) Method: An Exploratory Analysis for Head and Neck Imaging. Cancers (Basel) 2022.
- Ryu KH, Baek HJ, Moon JI, Choi BH, Park SE, Ha JY, Jeon KN, Bae K, Choi DS, Cho SB, Lee Y, Heo YJ. Initial clinical experience of synthetic MRI as a routine neuroimaging protocol in daily practice: A single-center study. J Neuroradiol 2020;47:151-60. [Crossref] [PubMed]
- Hagiwara A, Fujita S, Ohno Y, Aoki S. Variability and Standardization of Quantitative Imaging: Monoparametric to Multiparametric Quantification, Radiomics, and Artificial Intelligence. Invest Radiol 2020;55:601-16. [Crossref] [PubMed]
- Zhao L, Liang M, Shi Z, Xie L, Zhang H, Zhao X. Preoperative volumetric synthetic magnetic resonance imaging of the primary tumor for a more accurate prediction of lymph node metastasis in rectal cancer. Quant Imaging Med Surg 2021;11:1805-16. [Crossref] [PubMed]
- Li Q, Xiao Q, Yang M, Chai Q, Huang Y, Wu PY, Niu Q, Gu Y. Histogram analysis of quantitative parameters from synthetic MRI: Correlations with prognostic factors and molecular subtypes in invasive ductal breast cancer. Eur J Radiol 2021;139:109697. [Crossref] [PubMed]
- Reuss DE, Mamatjan Y, Schrimpf D, Capper D, Hovestadt V, Kratz A, Sahm F, Koelsche C, Korshunov A, Olar A, Hartmann C, Reijneveld JC, Wesseling P, Unterberg A, Platten M, Wick W, Herold-Mende C, Aldape K, von Deimling A. IDH mutant diffuse and anaplastic astrocytomas have similar age at presentation and little difference in survival: a grading problem for WHO. Acta Neuropathol 2015;129:867-73. [Crossref] [PubMed]
- Ohmura K, Kumagai N, Kumagai M, Ikegame Y, Shinoda J, Yano H, Muragaki Y, Iwama T. Combining methionine-PET and MRI fluid-attenuated inversion-recovery mismatch to determine glioma molecular subtype. J Neuroimaging 2023;33:652-60. [Crossref] [PubMed]
- Joo B, Han K, Ahn SS, Choi YS, Chang JH, Kang SG, Kim SH, Zhou J, Lee SK. Amide proton transfer imaging might predict survival and IDH mutation status in high-grade glioma. Eur Radiol 2019;29:6643-52. [Crossref] [PubMed]
- Cui Y, Han S, Liu M, Wu PY, Zhang W, Zhang J, Li C, Chen M. Diagnosis and Grading of Prostate Cancer by Relaxation Maps From Synthetic MRI. J Magn Reson Imaging 2020;52:552-64. [Crossref] [PubMed]
- Meng T, He H, Liu H, Lv X, Huang C, Zhong L, Liu K, Qian L, Ke L, Xie C. Investigation of the feasibility of synthetic MRI in the differential diagnosis of non-keratinising nasopharyngeal carcinoma and benign hyperplasia using different contoured methods for delineation of the region of interest. Clin Radiol 2021;76:238.e9-238.e15. [Crossref] [PubMed]
- Du S, Gao S, Zhao R, Liu H, Wang Y, Qi X, Li S, Cao J, Zhang L. Contrast-free MRI quantitative parameters for early prediction of pathological response to neoadjuvant chemotherapy in breast cancer. Eur Radiol 2022;32:5759-72. [Crossref] [PubMed]