The agreement of different techniques for muscle measurement in diagnosing sarcopenia: a systematic review and meta-analysis
Introduction
Driven by falling fertility rates and remarkable increases in life expectancy, population aging is accelerating globally (1). Sarcopenia progressively results in negative consequences such as falls, fractures, social isolation, hospitalization, and an overall decrease in the quality of life (2). The estimated prevalence of sarcopenia ranged from 8% to 36% in individuals aged <60 years and from 10% to 27% in those ≥60 years when using different classifications and cut-off points (3). Identified as the age-related loss of skeletal muscle mass plus loss of muscle strength and/or reduced physical performance (4,5), sarcopenia is now formally recognized as a muscle disease with an International Classification of Diseases, 10th Revision, Clinical Modification (ICD-10-CM) diagnosis code to support timely prevention and improvement of muscle health worldwide (6).
An expert consensus has been established by European, North American, and Asian working groups on putting forward plans and recommendations for the screening, diagnosis, prevention, and treatment of sarcopenia (7). Currently, the main parameters available for the diagnosis of sarcopenia are muscle mass, muscle strength, and physical function (8). Among these, the identification of muscle mass loss plays a key role. Different kinds of instruments measure the muscle mass in terms of the total body skeletal muscle mass, the appendicular skeletal muscle mass (ASM), or the muscle cross-sectional area of specific muscle groups or body locations (4).
Dual-energy X-ray absorptiometry (DXA) measures fat and bone mineral content and subsequently determines lean tissue quantity (9). Both the European Working Group on Sarcopenia in Older People (EWGSOP) and the Asian Working Group for Sarcopenia (AWGS) recommend the clinical use of DXA for muscle mass measurement due to its ease of operation, non-invasive nature, and relatively low radiation (4,5). However, its relative high cost and non-mobile nature restrict its widespread use in the community (10), especially in those regions with limited medical resources. In addition, the DXA measurements can also be influenced by the hydration and obesity status of those examined. Other imaging or electrical assessments may help to support the measurement of muscle mass. Recently, bioelectrical impedance analysis (BIA), with the advantages of no radiation, cost-effectiveness, and portability, has been recommended to assess muscle mass by the EWGSOP and the AWGS (4,5). Although neither EWGSOP nor AWGS recommends ultrasound (US) as a valid assessment for total body skeletal muscle mass or ASM (4,5), it can measure muscle thickness (MT) and cross-sectional area (11); it shows potential as a convenient and fast method for muscle mass assessment in the community and has clinical application prospects (12). The reliability and validity of US to quantify muscles has been confirmed in older adults, and it has been recommended to be used for muscle assessment in sarcopenia (13,14). Moreover, computed tomography (CT) and magnetic resonance imaging (MRI), which could offer assessments of the structure and morphology of skeletal muscle tissue, are more accurate measurement tools for skeletal muscle quality. In particular, CT is widely used in routine clinical examinations. Retrospective studies of sarcopenia based on previous CT findings have been conducted with increasing interest. The value of CT has also been reported in sarcopenia patients with cancer (15), hip fractures, and refractures (16-18).
Although the performance of these assessments for sarcopenia identification have been studied, the understanding of how they are correlated with the value of DXA and validated evidence for their clinical uses to assess muscle mass in sarcopenia remains limited. Thus, this study was aimed to conduct a systematic review and meta-analysis to evaluate the diagnostic value of the BIA, US, MRI, and CT, comparing with that of DXA, for muscle mass in sarcopenia. We present this article in accordance with the PRISMA-DTA reporting checklist (19) (available at https://qims.amegroups.com/article/view/10.21037/qims-23-1089/rc).
Methods
This study was registered at the International Prospective Register of Systematic Reviews (PROSPERO) (No. CRD42022374959). L.L. and Z.X. independently reviewed and extracted data from the qualified articles, and assessed the risk of bias to evaluate the methodological quality. Any discrepancies were resolved by discussion or further consultation with Y.S.
Search strategy
A systematic search on PubMed, Web of Science, Cochrane Library, and Embase Database was conducted from inception of each database to 12 October, 2023 by using the following search terms with relevant Medical Subject Headings (MeSH) terms: sarcopenia, muscle mass, body composition, DXA, BIA, US, MRI, and CT. Manual retrieval and cross-referencing from reference lists were also conducted. A full description of the search strategy on the 4 databases is provided in Tables S1-S4.
Eligibility criteria
The inclusion criteria were as follows: (I) study design: cohorts, case-control studies; (II) Study subject: patients using BIA, US, MRI, or CT to estimate muscle mass alone or to support the diagnosis of sarcopenia while using DXA as reference, regardless of race, nationality, sex, and so on; (III) diagnostic method: The diagnostic method to be evaluated was BIA, CT, MRI, and US, whereas DXA was the gold standard; (IV) diagnostic parameter: ASM, appendicular skeletal muscle mass/height2 (ASMI), or other equivalent parameters for DXA and BIA, appendicular lean mass (ALM) or MT for US, and skeletal muscle mass for CT and MRI; (V) outcome: sensitivity, specificity, area under the receiver operating characteristic curve (ROC) curve (AUC), Cohen’s kappa coefficient (κ) and Pearson correlation coefficient for all cases; (VI) age of participant: ≥18 years; (VII) publication time of original study: from inception to 12 October 2023. The exclusion criteria were as follows: (I) non-English studies; (II) for repeated publications, the newly published studies with complete data should be selected; (III) studies with incomplete or unretrievable data; (IV) studies focused on “sarcopenia/muscle mass” assessment in specific clinical populations, in which the participants have any specific condition or disease that would impact on muscle mass; (V) abstract, review, comment, lecture, case report, conference paper, and so on; (VI) non-human research.
Data extraction
The extracted data included the following: (I) basic information of the original studies including the first authors, publication year, publication district, population, ethnicity, setting, sample size, age, sex, body mass index (BMI); (II) image omics features for differential diagnosis: the definition of sarcopenia, reference methods, new methods, measured parameters, instrument type, instrument frequency, measurement position; (III) relevant outcome obtained by extracting or calculating: sensitivity, specificity, positive likelihood ratio (PLR), negative likelihood ratio (NLR), positive predictive value (PPV), negative predictive value (NPV), AUC, Cohen’s κ, and Pearson correlation coefficient; (IV) statistical findings and overall conclusions.
Quality assessment
Quality assessments were conducted using the Quality Assessment of Diagnostic Accuracy Studies 2 (QUADAS-2) by Review Manager Software version 5.4 (Cochrane Collaboration, Copenhagen, Denmark). The QUADAS-2 tool involves 4 key domains: patient selection, index test, reference standard, and flow and timing. Assessment of the risk of bias and concerns about applicability are regarded as distinct components of quality assessment and classified as “Low”, “High”, or “Unclear” (20).
Statistical analysis
The representative muscle index derived from DXA and BIA according to AWGS (4) and EWGSOP (5), and the MT and ALM from US according to the published studies (21-23), were selected as the main outcomes for comparison. The diagnostic value was compared based on the estimated sensitivity, specificity, AUC, Cohen’s κ, and Pearson correlation coefficient. When multiple outcomes were reported in the same original study due to the different cut-off values, muscular parameters, and predictive equations, the symptomatic 1 or 2 with the largest sensitivity, specificity, or Pearson correlation coefficient and with the most significant level were used in the final analysis. For Cohen’s κ scores, the values of 0 to 0.20, 0.21 to 0.40, 0.41 to 0.60, 0.61 to 0.80, and 0.81 to 1.00 were regarded as poor, fair, moderate, good, and excellent agreement, respectively (24). For AUC, the values of 0 to 0.50, 0.51 to 0.70, 0.71 to 0.90, 0.91 to 1.00, and 1.00 were treated as none, low, moderate, high, and perfect predictive power, respectively (25). For the correlation coefficient, the values of 0 to 0.25, 0.26 to 0.49, 0.50 to 0.69, 0.70 to 0.89, and 0.90 to 1.00 were classified as little, low, moderate, high, and very high correlation, respectively (26).
The pooled sensitivity and specificity, Cohen’s κ and their 95% confidence intervals (CIs) were computed if there was no heterogeneity according to the Spearman threshold effect analysis. Otherwise, the pooled estimates would be provided for each effect size. The pooled Pearson correlation coefficient and its 95% CI were also computed. An inconsistency index (I2) was used to evaluate the statistical heterogeneity (27) across the original studies for each outcome. Random effects models were used when heterogeneity was moderate or high (I2 ≥50%). A sensitivity analysis was performed by removing each study to test the robustness of the summarized outcomes. Meta-regression analysis and subgroup analysis (by the group of sample size, population source, diagnostic criteria, publication year, publication region, the type of DXA or BIA, the average age of the cases and other information if sufficient) were further performed to explore the sources of heterogeneity for the pooled sensitivity and specificity and pooled Pearson correlation coefficient. A summary receiver operating characteristic (sROC) was also drawn to estimate the pooled AUC and its 95% CI.
Publication bias was evaluated by Deek’s funnel plot asymmetry test for the pooled sensitivity and specificity, and Egger’s linear regression test (28) and Begg’s rank correlation test (29) with funnel plots for the pooled Pearson correlation coefficient. Sensitivity analysis was carried out by removing each study to explore the potential impact of a single study on the summarized estimates. The statistical analyses were conducted by using Stata software version 15.0 (Stata Corp, College Station, Texas, USA), MetaDisc software version 1.4 (Unit of Clinical Biostatistics Team of the Ramony Cajal Hospital, Madrid, Spain), or RStudio Software version 4.2.3 (RStudio, Boston, Massachusetts, USA). A P value <0.05 (2-sides) was considered the level of statistical significance.
Results
Search results and study characteristics
A total of 10,968 records were retrieved (675 from PubMed, 3,922 from Web of Science, 1,816 from Cochrane library, 4,554 from Embase Database, and 1 from manual search). Duplicates (n=4,353) were excluded, and the others (n=6,615) were screened by review of the titles and abstracts. After that, 166 were selected for a full-text review for the eligibility assessment. Of these, 138 studies were removed according to the inclusion and exclusion criteria. Finally, 28 studies were included in this systematic review for narrative synthesis (21-23) and (30-54). The flow chart for the selection process according to the PRISMA guidelines is displayed in Figure 1.
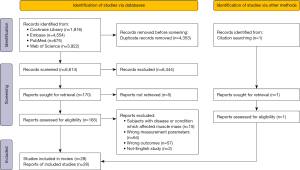
In the current meta-analysis, we included 28 articles (16 for BIA, 8 for US, and 4 for MRI), which involved 4,926 participants. Among these, 13 were conducted in Asia, 3 in Europe, 7 in North America, 4 in South America, and 1 in Oceania. The sample size ranged from 18 to 551. The mean age of the cases ranged from 22.1 [standard deviation (SD) =1.4] to 83.3 (SD =3.0) years, and the mean BMI (kg/cm2) ranged from 21.9 (SD =2.8) to 28.9 (SD =4.7). The characteristics of the 28 studies, including the publication year, publication region, sample size, gender, age, BMI, gold standard, and new method, are shown in Table 1.
Table 1
Number | Study | Country | Sample size (total/male/female) | Age (mean ± SD, year) | BMI (mean ± SD, kg/m2) | Gold standard | New method |
---|---|---|---|---|---|---|---|
1 | Ashley A. Herda 2022 (31) | USA | 73/19/54 | 69.4±6.3/66.1±7.2 | 27.1±4.3 | DXA | BIA |
2 | Hong-Qi Xu 2021 (30) | China | 301/129/172 | 46.7±25.2/52.5±23.5 | 23.6±3.8/23.1±3.9 | DXA | BIA |
3 | Hyeoijin Kim 2022 (32) | Korea | Development group: 131/63/68 | 77.5±4.1/76.9±4.4 | 23.5±2.4/23.6±2.5 | DXA | BIA |
Validation group: 64/31/33 | 76.5±4.4/76.9±3.7 | 23.7±2.4/23.8±2.1 | |||||
4 | Jantine van den Helder 2022 (33) | Netherlands | 202/58/144 | 72.0±6.0/72.1±6.6/72.1±6.4 | 25.2±3.0/25.6±3.8/25.4±3.6 | DXA | BIA |
5 | Kwon Chan Jeon 2020 (34) | Korea | 199/94/105 | Development group: 76.4±4.2/76.1±4.1 | 23.7±2.3/23.8±2.2 | DXA | BIA |
Validation group: 75.9±4.1/75.6±4.3 | 23.5±2.3/23.3±2.7 | ||||||
6 | M. Lane Moore 2020 (35) | USA | 179/76/103 | 33.8±14.5/33.4±15.9/33.6±15.3 | 26.2±4.3/24.2±4.0/25.1±4.2 | DXA | BIA |
7 | Maria Aquimara Zambone 2020 (36) | Brazil | 92/92/0 | >60 | NA | DXA | BIA |
8 | Purwita W. Laksmi 2019 (37) | Indonesia | 120/46/74 | 71.9±6.1 | 21.9±3.8/22.9±5.0 | DXA | BIA |
9 | S. Toselli 2021 (38) | Brazil | 184/44/140 | 71.5±7.3 | 27.9±5.3 | DXA | BIA |
10 | S. Vermeiren 2019 (39) | Belgium | 174/91/83 | 83.3±3.0/83.3±2.9/83.3±3.0 | 26.8±3.5/27.0±3.2/26.4±3.9 | DXA | BIA |
11 | Shaea A. Alkahtani 2017 (40) | Saudi Arabia | 232/232/0 | 27.1±4.2 | 28.1±5.5 | DXA | BIA |
12 | Solomon C. Y. Yu 2016 (41) | Australia | 195/78/117 | 48.0±17.0/52.7±14.7 | 27.4±4.5/26.4±5.7 | DXA | BIA |
13 | Thiago G. Barbosa-Silva, MD, PhD 2020 (42) | Brazil | Single frequency BIA: 181/70/111 | ≥60 | 27.0±3.7/28.9±4.7 | DXA | BIA |
Multi-frequency BIA: 178/67/111 | ≥60 | 26.9±3.6/28.9±4.7 | |||||
14 | Wen-Hui Fang, MD 2020 (43) | China | 438/179/259 | 73.3±6.8 | 24.09±3.32 | DXA | BIA |
15 | Ling-Chun Lee 2014 (44) | China | 77/42/35 | 62.5±5.3/62.6±6.0/62.5±5.6 | 26.4±3.6/25.6±3.8/26.0±3.7 | DXA | BIA |
16 | Miji Kim 2015 (45) | Japan | 551/241/310 | 74.3±5.1/73.0±5.3/73.6±2.4 | NA | DXA | BIA |
17 | Xinyu Zhao 2013 (46) | China | 66/52/14 | 52.0±13.3/46.6±11.8 | 24.2±3.1/22.7±2.7 | DXA | MRI |
18 | Richard V. Clark 2014 (47) | USA | 35/20/15 | 51.0±23.0 | 27.0±3.2 | DXA | MRI |
19 | Zhao Chen 2007 (48) | USA | 104/0/104 | 70.7±6.4 | 27.4±5.1 | DXA | MRI |
20 | Richard V. Clark 2018 (49) | USA | 18/14/4 | 73.3±5.8 | NA | DXA | MRI |
21 | M. Neira Álvarez 2021 (50) | Spain | 57/24/33 | Median (IQR): 78.9 (74.9–81.9) | Median (IQR): 27.1 (25.1–29.2) | DXA | US |
22 | Takashi Abe, PhD 2018 (51) | USA | 311/133/178 | Development group: 71.0±5.0 | 23.5±2.9 | DXA | US |
Validation group: 69.0±5.0 | 23.5±3.1 | ||||||
23 | Takashi Abe 2015 (22) | USA | 102/59/43 | Development group: 59.3±6.5/58.3±6.4 | 26.1±3.5/23.9±5.7 | DXA | US |
Validation group: 60.6±6.9/57.1±5.4 | 25.5±2.1/26.0±6.3 | ||||||
24 | Takashi Abe 2016 (23) | Japan | 158/72/86 | 64.0±8.0 | 24.0±3.4 | DXA | US |
25 | Thiago G. Barbosa-Silva, MD, PhD 2021 (21) | Brazil | 190/72/118 | 69.8±7.5 | 27.0±3.6/28.9±4.9/28.2±4.5 | DXA | US |
26 | Yen-Lung Chen 2022 (52) | China | 91/35/56 | 76.3±11.3/63.0±6.8/68.3±11.0 | 24.9±3.5/22.3±3.0/23.3±3.5 | DXA | US |
27 | Akio Morimoto 2017 (53) | Japan | 30/30/0 | 22.1±1.4 | NA | DXA | US |
28 | Satoshi Yuguchi 2022 (54) | Japan | 193/72/121 | 73.2±4.3/71.9±4.2/72.4±4.3 | 23.2±3.0/21.9±2.8/22.4±2.9 | DXA | US |
SD, standard deviation; BMI, body mass index; USA, the United States of America; DXA, dual-energy X-ray absorptiometry; BIA, bioelectrical impedance analysis; NA, not applicable; MRI, magnetic resonance imaging; IQR, interquartile range; US, ultrasound.
Quality assessment
Among the selected studies, 6 studies were considered to have a high risk of participant selection bias, 1 study was unclear, 1 study was noted to have high risk of index test bias, and 1 study was noted to have a high risk of flow and timing bias. Beyond the above-mentioned, there were no unclear or high-risk observations for the bias of index test and reference standard. Details about the risk of bias in the included studies are shown in the Figure S1.
Sensitivity and specificity for BIA
A total of 7 original studies, comprising 1,478 participants, on the diagnostic value of BIA compared to DXA in diagnosing sarcopenia were included. Among these, 4 used the parameter of ASMI as the outcome, and 3 used appendicular lean mass/height2 (ALMI) (Table S5). The Spearman rank correlation test suggested that there was no threshold effect in the above studies (coefficient =0.36, P=0.43). The heterogeneity was substantial for sensitivity [I2=75.60% (57.36–93.85%), P<0.001] and specificity [I2=96.19% (94.49–97.88%), P<0.001]. Thus, the pooled sensitivity, pooled specificity, sROC, and their 95% CI were 0.79 (0.71–0.86), 0.95 (0.82–0.99), and 0.88 (0.85–0.90), respectively (Figures 2,3). DXA and BIA had a good agreement with an estimated pooled Cohen’s κ statistic of 0.61 (0.51–0.72) (Figure S2). Deeks’ funnel plot asymmetry test showed a significant publication bias (P<0.001, Figure S3). Sensitivity analysis revealed that the study conducted by Fang et al. (43) may have largely contributed to the publication bias due to its large sample size compared with the other 6 studies (Figure S4). The results from meta-regression analysis indicated that population source, publication region, and diagnostic criteria were significantly related with the heterogeneity of sensitivity and specificity (Figure 4).
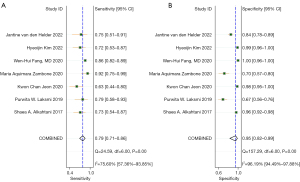
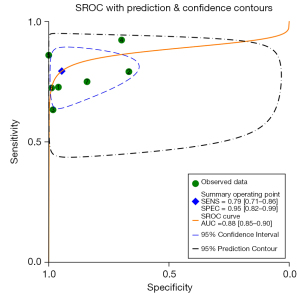
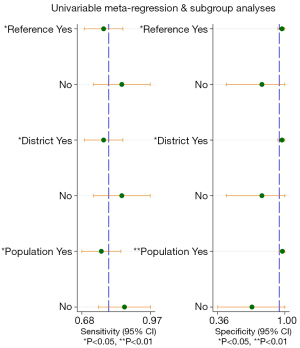
Sensitivity and specificity for US, MRI, and CT
There were inadequate studies available to compute the pooled sensitivity and specificity for US (none), MRI (none), and CT (none).
Pearson correlation coefficient for BIA and DXA
A total of 10 studies reported the Pearson correlation coefficient of muscle-related measures between BIA and DXA. Among them, ASM was used in 2 studies, ALM was used in 3 studies, appendicular lean soft tissue (ALST) was used in 4 studies, and ALMI was used in 1 study as the diagnostic parameters for DXA and BIA (Table S6). The correlation coefficient between muscle-related measures between BIA and DXA was very high [pooled r (95% CI) =0.94 (0.92–0.96), I2=89.00% (P<0.001)] (Figure 5). There was no obvious asymmetry for the pooled Pearson correlation coefficient from visual inspection of funnel plot (Figure S5). The Egger linear regression test and Begg’s rank correlation test indicated that there was no significant publication bias among the original studies (P>0.05). The results were robust in the leave-one-out sensitivity analysis, and the direction of the estimates were not essentially changed with the removal of each study (Figure S6). The results of subgroup analyses showed that the population source, publication region, sample size, publication year, BIA type, and average age of subjects were not the source of heterogeneity.
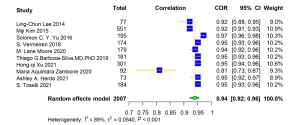
Pearson correlation coefficient for US and DXA
A total of 9 studies were included for the Pearson correlation coefficient between US and DXA. Among them, 6 studies used ALM and 3 used ASMI as the outcome for DXA. Regarding the outcome of US, 4 studies used upper limb MT, and the other 5 used lower limb MT (Table S7). The correlation coefficient between muscle-related measures between US and DXA was high [pooled r (95% CI) =0.69 (0.54–0.80), I2=93.00% (P<0.001)] (Figure 6). Obvious asymmetry for the pooled index was not observed from visual inspection of funnel plots (Figure S7). The results of Egger linear regression test and Begg’s rank correlation test suggested that there was no significant publication bias among the original studies (P>0.05). In sensitivity analysis, the estimations were robust after removing each of the studies (Figure S8). The results of subgroup analyses showed strong heterogeneities between the groups of different publication region (I2=95.00%, P<0.001; I2=0.00%, P=0.42) and MT parameter (I2=95.00%, P<0.001; I2=18.00%, P=0.30) (Figures 7,8).
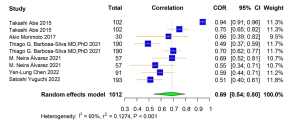
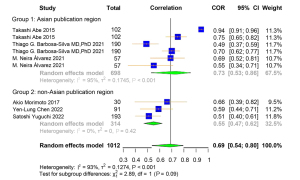
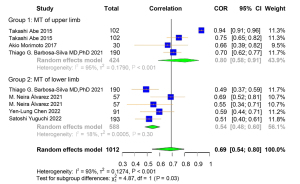
There were 3 original studies (659 participants) which used ALM as the outcome, and these were included to compute the pooled estimates for the diagnostic value of US (Table S8). The pooled r (95% CI) was 0.93 (0.91–0.95), with a high heterogeneity (I2=83.00%, P=0.003) (Figure S9). Sensitivity analysis suggested that the results were robust after removing each of the studies (Figure S10). Publication bias analysis and subgroup analysis were not conducted owing to the insufficient number of the original studies.
Pearson correlation coefficient for MRI and DXA
A total of 4 studies were included for the Pearson correlation coefficient between MRI and DXA. Among them, 3 studies used ALM and 1 used ALST as the outcome for DXA. All 4 studies used total body skeletal muscle mass as the outcome for MRI (Table S9). The correlation coefficient of muscle-related measures between MRI and DXA was very high [pooled r (95% CI) =0.96 (0.95–0.97), I2=33.00% (P=0.21)] (Figure 9). Obvious asymmetry for the pooled index was not detected from visual inspection of funnel plots (Figure S11). The results of Egger linear regression test and Begg’s rank correlation test suggested that there was no significant publication bias among the original studies (P>0.05). In sensitivity analysis, the estimations were robust after removing each of the studies (Figure S12). The results of subgroup analyses showed that there was no significant heterogeneity between the groups with different average age of participants (I2=0.00%, P=0.50; I2=65.00%, P=0.09) (Figure 10). The original or calculated data for the meta-analyses are presented in Tables S10-S14.
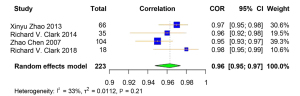
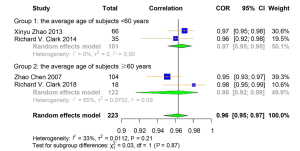
Discussion
This study evaluated the diagnostic agreement of BIA, US, CT, and MRI compared with that of DXA in measuring muscle mass to diagnose sarcopenia by meta-analyses. Overall, the findings suggested that BIA, US, and MRI may have a good value in quantifying muscle mass to diagnose sarcopenia when compared with DXA. More evidence is needed for the application of CT in helping diagnose sarcopenia.
In the current study, a relative high sensitivity and specificity for using BIA-measured muscle mass in diagnosing sarcopenia were estimated. Especially in specificity, the performance was better than in sensitivity. BIA has been reported as a mathematical method for detecting sarcopenia in adults with cancer prior to treatment, and a viable alternative to DXA, CT, and MRI in oncology clinical practice (55). Furthermore, this meta-analysis confirmed a high positive correlation between muscle mass measured by BIA and DXA. Even so, when compared to DXA, anthropometry and calf circumferences presented better agreements than BIA in 2 studies (36,56). Moreover, following the EWGSOP case finding algorithm, the BIA-based approach resulted in a higher rate of misclassification of sarcopenic/non-sarcopenic cases, when compared to the DXA-based approach (57). Previous studies also reported that BIA significantly overestimated (58,59) or underestimated (45,60) muscle mass in absolute terms. Besides, we found that differences in population source, diagnostic criteria, and publication region among the original studies may introduce substantial heterogeneity for the diagnostic value, which is consistent with the previous studies (61). Similarly, a study reported that when a prediction equation of BIA based on Caucasian data had a good application to Hispanics with minor differences in the muscle mass measured by BIA and DXA, it failed to have a good agreement with Afro-Americans (62). This also suggested that the measurement by BIA, and its agreement with DXA may be influenced by population characteristics (63,64). In addition, a previous study showed that BIA devices with multi-frequency and the ASM estimation equation resulted in poor agreements and significant differences from the reference method of DXA (31).
Although the small number of original studies limited the estimation for the diagnostic value of US-measured muscle mass in diagnosing sarcopenia, the relationship between muscle mass measured by DXA and US was explored. We found that US might also be a reliable imaging method to quantify muscle mass for its relatively high correlation with DXA, and it showed a better correlation when using ALM as outcome for DXA and US. There were 2 studies that evaluated the agreement of US with DXA (50,65), 1 of which assessed the agreement between ALM acquired by DXA, and MT of gastrocnemius medialis and longitudinal plane obtained by US (50). MT on gastrocnemius medialis measured by US was not merely correlated well with DXA, but also with calf circumferences values and muscle performance. The sensitivities by MT of the gastrocnemius muscle in transverse and longitudinal plane was 77.80% and 77.10%, respectively, and the specificity was 100.00% and 68.80%, respectively. The other study found that the sensitivity and specificity by MT on gastrocnemius was 70.00% and 76.00%, respectively (65). According to the published studies, the most frequently used parameter for US was MT, following by cross-sectional area, echo intensity, and pennation angle, and the most frequently used positions were muscles in the gastrocnemius and rectus femoris (66). A previous study suggested that gastrocnemius MT and fascicle length values can serve as alternative measurements for predicting/quantifying sarcopenia (67). Besides gastrocnemius medialis, position of forearm-ulna (22) and rectus femoris (52), were also used. A study carried out ultrasonographic examinations for the non-dominant thenar musculature (68), which provided evidence for researchers to perform measurement on such a small muscle. However, US-derived ALM may be overestimated when compared with DXA-derived ALM (22), and the related ultrasonic measurements to anthropometric assessments, such as height (22) and calf circumferences (21), may help improve the accuracy. However, the clinical use of US is narrow due to the lack of a standardized protocol and validated cutoff points. Several new ultrasonic techniques for diagnosing muscle diseases have emerged in recent years, such as elastosonography and artificial intelligence (69).
The forest plot showed that DXA-derived muscle mass had a strong correlation with MRI-derived muscle mass with a pooled r (95% CI) =0.96 (0.95–0.97). Similarly, even if different diagnostic parameters were used, 1 study reported a high correlation between the total body lean mass assessed by DXA and the thigh skeletal muscle size assessed by MRI (r=0.89) (70). The other Chinese study showed significant high correlations between total-body bone-free lean mass measured by DXA and skeletal muscle mass measured by MRI in both women (r=0.78) and men (r=0.83) (71). However, a weak association between ALMI gained by DXA and cross-sectional area measured by MRI (r=0.18), and a modest association between ALMI and muscle volume (r=0.58) were reported in South Korea (72).
A paper reported the correlation between the measurements of muscle mass by CT and DXA (r=0.81–0.98), and provided the prediction equations between lean mass by DXA and lean mass volume by CT (73), which made it possible to convert DXA-derived lean mass to the CT-derived skeletal muscle volume with a higher accuracy in estimation. Another paper reported the correlation between DXA-derived ASMI and CT-derived cross-sectional area for men (r=0.75–0.80) and women (r=0.63–0.71), respectively (74).
Sarcopenia is identified as the gradual loss of skeletal muscle mass and strength (75), therefore, early diagnosis and management are very important. Muscle mass is one of the main parameters in the diagnosis and evaluation of sarcopenia. ASM is a key index to evaluate muscle mass, so a precise quantitative estimate of ASM is fundamental for diagnosing sarcopenia (76). The current meta-analysis showed that BIA is a more attractive method for assessing muscle mass due to its higher correlation with DXA. Although there was also a good correlation between MRI and DXA, MRI is mostly used in the research, especially in populations with a specific condition, for example, patients with cancer (77). BIA would be more available because it provides an affordable, noninvasive test that can be completed within a few minutes during a clinic visit, it does not require highly skilled personnel, and the results are immediately available (55). However, all of the muscle mass parameters such as fat-free mass, skeletal muscle, or ASM measured by BIA depend on an equation, first generated from a validation study against a reference method, usually including MRI or DXA, and the equations or algorithm are device-specific (63). More research should be conducted to standardize the terminology employed to describe muscularity and provide precise cut-off values for specific populations using this method (78).
As far as we know, this is the first meta-analysis to evaluate the diagnostic agreement in sarcopenia of BIA, US, CT, and MRI to assess muscle mass. Eventually, 28 articles met the inclusion and exclusion criteria, and most of the studies in this review have high methodological quality. We selected 2 indicators including diagnostic tests and correlation to examine the validity between DXA and 4 techniques. However, this study had some limitations. Firstly, we did not conduct meta-analysis for CT on account of the limited number of relevant original studies and the discrepancies in measurement parameters. Secondly, the comparison between males and females was not implemented because of inadequate data. Thirdly, there was high heterogeneity in different studies, such as the definition of sarcopenia, publication year, equipment type, and others. Fourthly, the unit disparities within the original studies may be one of the sources of heterogeneity. Additionally, as muscle mass is only one of the parameters in diagnosing sarcopenia, the accuracy of the remaining parameters (muscle strength and/or physical performance) would probably also affect the accuracy of diagnosis. Moreover, the variance in the adopted predictive equations, cut-offs, and their adequacy to the evaluated population may also have compromised the validity of the findings.
Conclusions
BIA, US, and MRI would provide acceptable diagnostic accuracy for sarcopenia by evaluating muscle mass in terms of sensitivity, specificity, accuracy, and their higher correlations with DXA. More studies are needed for the value of evaluating muscle mass by CT in diagnosing sarcopenia.
Acknowledgments
The authors wish to thank all participants dedicated to contributing to the study.
Funding: This work was supported by
Footnote
Reporting Checklist: The authors have completed the PRISMA-DTA reporting checklist. Available at https://qims.amegroups.com/article/view/10.21037/qims-23-1089/rc
Conflicts of Interest: All authors have completed the ICMJE uniform disclosure form (available at https://qims.amegroups.com/article/view/10.21037/qims-23-1089/coif). The authors have no conflicts of interest to declare.
Ethical Statement: The authors are accountable for all aspects of the work in ensuring that questions related to the accuracy or integrity of any part of the work are appropriately investigated and resolved.
Open Access Statement: This is an Open Access article distributed in accordance with the Creative Commons Attribution-NonCommercial-NoDerivs 4.0 International License (CC BY-NC-ND 4.0), which permits the non-commercial replication and distribution of the article with the strict proviso that no changes or edits are made and the original work is properly cited (including links to both the formal publication through the relevant DOI and the license). See: https://creativecommons.org/licenses/by-nc-nd/4.0/.
References
- Beard JR, Officer A, de Carvalho IA, Sadana R, Pot AM, Michel JP, Lloyd-Sherlock P, Epping-Jordan JE, Peeters GMEEG, Mahanani WR, Thiyagarajan JA, Chatterji S. The World report on ageing and health: a policy framework for healthy ageing. Lancet 2016;387:2145-54. [Crossref] [PubMed]
- Öztürk ZA, Türkbeyler İH, Abiyev A, Kul S, Edizer B, Yakaryılmaz FD, Soylu G. Health-related quality of life and fall risk associated with age-related body composition changes; sarcopenia, obesity and sarcopenic obesity. Intern Med J 2018;48:973-81. [Crossref] [PubMed]
- Petermann-Rocha F, Balntzi V, Gray SR, Lara J, Ho FK, Pell JP, Celis-Morales C. Global prevalence of sarcopenia and severe sarcopenia: a systematic review and meta-analysis. J Cachexia Sarcopenia Muscle 2022;13:86-99. [Crossref] [PubMed]
- Cruz-Jentoft AJ, Bahat G, Bauer J, Boirie Y, Bruyère O, Cederholm T, Cooper C, Landi F, Rolland Y, Sayer AA, Schneider SM, Sieber CC, Topinkova E, Vandewoude M, Visser M, Zamboni M. Writing Group for the European Working Group on Sarcopenia in Older People 2 (EWGSOP2), and the Extended Group for EWGSOP2. Sarcopenia: revised European consensus on definition and diagnosis. Age Ageing 2019;48:16-31. Erratum in: Age Ageing 2019;48:601.
- Chen LK, Woo J, Assantachai P, Auyeung TW, Chou MY, Iijima K, et al. Asian Working Group for Sarcopenia: 2019 Consensus Update on Sarcopenia Diagnosis and Treatment. J Am Med Dir Assoc 2020;21:300-307.e2. [Crossref] [PubMed]
- Vellas B, Fielding RA, Bens C, Bernabei R, Cawthon PM, Cederholm T, Cruz-Jentoft AJ, Del Signore S, Donahue S, Morley J, Pahor M, Reginster JY, Rodriguez Mañas L, Rolland Y, Roubenoff R, Sinclair A, Cesari M. Implications of ICD-10 for Sarcopenia Clinical Practice and Clinical Trials: Report by the International Conference on Frailty and Sarcopenia Research Task Force. J Frailty Aging 2018;7:2-9. [Crossref] [PubMed]
- Fielding RA, Vellas B, Evans WJ, Bhasin S, Morley JE, Newman AB, et al. Sarcopenia: an undiagnosed condition in older adults. Current consensus definition: prevalence, etiology, and consequences. International working group on sarcopenia. J Am Med Dir Assoc 2011;12:249-56. [Crossref] [PubMed]
- Kim JW, Kim R, Choi H, Lee SJ, Bae GU. Understanding of sarcopenia: from definition to therapeutic strategies. Arch Pharm Res 2021;44:876-89. [Crossref] [PubMed]
- Bazzocchi A, Ponti F, Albisinni U, Battista G, Guglielmi G. DXA: Technical aspects and application. Eur J Radiol 2016;85:1481-92. [Crossref] [PubMed]
- Albano D, Messina C, Vitale J, Sconfienza LM. Imaging of sarcopenia: old evidence and new insights. Eur Radiol 2020;30:2199-208. [Crossref] [PubMed]
- Li S, Li H, Hu Y, Zhu S, Xu Z, Zhang Q, Yang Y, Wang Z, Xu J. Ultrasound for Measuring the Cross-Sectional Area of Biceps Brachii Muscle in Sarcopenia. Int J Med Sci 2020;17:2947-53. [Crossref] [PubMed]
- Zhu S, Lin W, Chen S, Qi H, Wang S, Zhang A, Cai J, Lai B, Sheng Y, Ding G. The correlation of muscle thickness and pennation angle assessed by ultrasound with sarcopenia in elderly Chinese community dwellers. Clin Interv Aging 2019;14:987-96. [Crossref] [PubMed]
- Nijholt W, Scafoglieri A, Jager-Wittenaar H, Hobbelen JSM, van der Schans CP. The reliability and validity of ultrasound to quantify muscles in older adults: a systematic review. J Cachexia Sarcopenia Muscle 2017;8:702-12. [Crossref] [PubMed]
- Perkisas S, Baudry S, Bauer J, Beckwée D, De Cock AM, Hobbelen H, Jager-Wittenaar H, Kasiukiewicz A, Landi F, Marco E, Merello A, Piotrowicz K, Sanchez E, Sanchez-Rodriguez D, Scafoglieri A, Cruz-Jentoft A, Vandewoude M. Application of ultrasound for muscle assessment in sarcopenia: towards standardized measurements. Eur Geriatr Med 2018;9:739-57.
- Vangelov B, Bauer J, Kotevski D, Smee RI. The use of alternate vertebral levels to L3 in computed tomography scans for skeletal muscle mass evaluation and sarcopenia assessment in patients with cancer: a systematic review. Br J Nutr 2022;127:722-35. [Crossref] [PubMed]
- Wang L, Yin L, Zhao Y, Su Y, Sun W, Liu Y, Yang M, Yu A, Blake GM, Cheng X, Wu X, Veldhuis A, Engelke K. Muscle density discriminates hip fracture better than computed tomography X-ray absorptiometry hip areal bone mineral density. J Cachexia Sarcopenia Muscle 2020;11:1799-812. [Crossref] [PubMed]
- Wang L, Yin L, Yang M, Ge Y, Liu Y, Su Y, et al. Muscle density is an independent risk factor of second hip fracture: a prospective cohort study. J Cachexia Sarcopenia Muscle 2022;13:1927-37. [Crossref] [PubMed]
- Wang L, Yang M, Ge Y, Liu Y, Su Y, Guo Z, Huang P, Geng J, Wang G, Blake GM, He B, Yin L, Cheng X, Wu X, Engelke K, Vlug AG. Muscle size and density are independently associated with death after hip fracture: A prospective cohort study. J Cachexia Sarcopenia Muscle 2023;14:1824-35. [Crossref] [PubMed]
- McInnes MDF, Moher D, Thombs BD, McGrath TA, Bossuyt PM, et al. Preferred Reporting Items for a Systematic Review and Meta-analysis of Diagnostic Test Accuracy Studies: The PRISMA-DTA Statement. JAMA 2018;319:388-96. [Crossref] [PubMed]
- Wade R, Corbett M, Eastwood A. Quality assessment of comparative diagnostic accuracy studies: our experience using a modified version of the QUADAS-2 tool. Res Synth Methods 2013;4:280-6. [Crossref] [PubMed]
- Barbosa-Silva TG, Gonzalez MC, Bielemann RM, Santos LP, Costa CDS, Menezes AMBCOCONUT Study Group, Brazil. 2 + 2 (+ 2) = 4: A new approach for appendicular muscle mass assessment by ultrasound. Nutrition 2021;83:111056. [Crossref] [PubMed]
- Abe T, Thiebaud RS, Loenneke JP, Young KC. Prediction and validation of DXA-derived appendicular lean soft tissue mass by ultrasound in older adults. Age (Dordr) 2015;37:114. [Crossref] [PubMed]
- Abe T, Fujita E, Thiebaud RS, Loenneke JP, Akamine T. Ultrasound-Derived Forearm Muscle Thickness Is a Powerful Predictor for Estimating DXA-Derived Appendicular Lean Mass in Japanese Older Adults. Ultrasound Med Biol 2016;42:2341-4. [Crossref] [PubMed]
- Sun S. Meta-analysis of Cohen’s kappa. Health Serv Outcomes Res Method 2011;11:145-63.
- Park SH, Goo JM, Jo CH. Receiver operating characteristic (ROC) curve: practical review for radiologists. Korean J Radiol 2004;5:11-8. [Crossref] [PubMed]
- Selistre LFA, Melo CS, Noronha MA. Reliability and Validity of Clinical Tests for Measuring Strength or Endurance of Cervical Muscles: A Systematic Review and Meta-analysis. Arch Phys Med Rehabil 2021;102:1210-27. [Crossref] [PubMed]
- Higgins JP, Thompson SG, Deeks JJ, Altman DG. Measuring inconsistency in meta-analyses. BMJ 2003;327:557-60. [Crossref] [PubMed]
- Egger M, Davey Smith G, Schneider M, Minder C. Bias in meta-analysis detected by a simple, graphical test. BMJ 1997;315:629-34. [Crossref] [PubMed]
- Begg CB, Mazumdar M. Operating characteristics of a rank correlation test for publication bias. Biometrics 1994;50:1088-101.
- Xu HQ, Liu JM, Zhang X, Xue YT, Shi JP, Chen W, Zheng XY. Estimation of skeletal muscle mass by bioimpedance and differences among skeletal muscle mass indices for assessing sarcopenia. Clin Nutr 2021;40:2308-18. [Crossref] [PubMed]
- Herda AA, Cleary CJ. Agreement between multifrequency BIA and DXA for assessing segmental appendicular skeletal muscle mass in older adults. Aging Clin Exp Res 2022;34:2789-95. [Crossref] [PubMed]
- Kim H, Song KH, Ambegaonkar JP, Chung S, Jeon K, Jiang FL, Eom JJ, Kim CH. Two-megahertz impedance index prediction equation for appendicular lean mass in Korean older people. BMC Geriatr 2022;22:385. [Crossref] [PubMed]
- van den Helder J, Verreijen AM, van Dronkelaar C, Memelink RG, Engberink MF, Engelbert RHH, Weijs PJM, Tieland M. Bio-Electrical Impedance Analysis: A Valid Assessment Tool for Diagnosis of Low Appendicular Lean Mass in Older Adults? Front Nutr 2022;9:874980. [Crossref] [PubMed]
- Jeon KC, Kim SY, Jiang FL, Chung S, Ambegaonkar JP, Park JH, Kim YJ, Kim CH. Prediction Equations of the Multifrequency Standing and Supine Bioimpedance for Appendicular Skeletal Muscle Mass in Korean Older People. Int J Environ Res Public Health 2020;17:5847. [Crossref] [PubMed]
- Moore ML, Benavides ML, Dellinger JR, Adamson BT, Tinsley GM. Segmental body composition evaluation by bioelectrical impedance analysis and dual-energy X-ray absorptiometry: Quantifying agreement between methods. Clin Nutr 2020;39:2802-10. [Crossref] [PubMed]
- Zambone MA, Liberman S, Garcia MLB. Anthropometry, bioimpedance and densitometry: Comparative methods for lean mass body analysis in elderly outpatients from a tertiary hospital. Exp Gerontol 2020;138:111020. [Crossref] [PubMed]
- Laksmi PW, Sukma FA, Setyohadi B, Nugroho P, Ariane A, Tirtarahardja G. The Need for a New Cut-off Value to Increase Diagnostic Performance of Bioelectrical Impedance Analysis Compared with Dual-Energy X-ray Absorptiometry to Measure Muscle Mass in Indonesian Elderly. Acta Med Indones 2019;51:95-101.
- Toselli S, Campa F, Matias CN, de Alencar Silva BS, Dos Santos VR, Maietta Latessa P, Gobbo LA. Predictive equation for assessing appendicular lean soft tissue mass using bioelectric impedance analysis in older adults: Effect of body fat distribution. Exp Gerontol 2021;150:111393. [Crossref] [PubMed]
- Vermeiren S, Beckwée D, Vella-Azzopardi R, Beyer I, Knoop V, Jansen B, Delaere A, Antoine A, Bautmans I, Scafoglieri AGerontopole Brussels Study group. Evaluation of appendicular lean mass using bio impedance in persons aged 80+: A new equation based on the BUTTERFLY-study. Clin Nutr 2019;38:1756-64. [Crossref] [PubMed]
- Alkahtani SA. A cross-sectional study on sarcopenia using different methods: reference values for healthy Saudi young men. BMC Musculoskelet Disord 2017;18:119. [Crossref] [PubMed]
- Yu SC, Powell A, Khow KS, Visvanathan R. The Performance of Five Bioelectrical Impedance Analysis Prediction Equations against Dual X-ray Absorptiometry in Estimating Appendicular Skeletal Muscle Mass in an Adult Australian Population. Nutrients 2016;8:189. [Crossref] [PubMed]
- Barbosa-Silva TG, Gonzalez MC, Bielemann RM, Santos LP, Menezes AMBCOCONUT Study Group, Brazil. Think Globally, Act Locally: The Importance of Population-Specific Bioelectrical Impedance Analysis Prediction Equations for Muscle Mass Assessment. JPEN J Parenter Enteral Nutr 2020;44:1338-46. [Crossref] [PubMed]
- Fang WH, Yang JR, Lin CY, Hsiao PJ, Tu MY, Chen CF, Tsai DJ, Su W, Huang GS, Chang H, Su SL. Accuracy augmentation of body composition measurement by bioelectrical impedance analyzer in elderly population. Medicine (Baltimore) 2020;99:e19103. [Crossref] [PubMed]
- Lee LC, Hsieh KC, Wu CS, Chen YJ, Chiang J, Chen YY. Validity of Standing Posture Eight-electrode Bioelectrical Impedance to Estimate Body Composition in Taiwanese Elderly. Int J Gerontol 2014;8:137-42.
- Kim M, Shinkai S, Murayama H, Mori S. Comparison of segmental multifrequency bioelectrical impedance analysis with dual-energy X-ray absorptiometry for the assessment of body composition in a community-dwelling older population. Geriatr Gerontol Int 2015;15:1013-22. [Crossref] [PubMed]
- Zhao X, Wang Z, Zhang J, Hua J, He W, Zhu S. Estimation of total body skeletal muscle mass in Chinese adults: prediction model by dual-energy X-ray absorptiometry. PLoS One 2013;8:e53561. [Crossref] [PubMed]
- Clark RV, Walker AC, O'Connor-Semmes RL, Leonard MS, Miller RR, Stimpson SA, Turner SM, Ravussin E, Cefalu WT, Hellerstein MK, Evans WJ. Total body skeletal muscle mass: estimation by creatine (methyl-d3) dilution in humans. J Appl Physiol (1985) 2014;116:1605-13. [Crossref] [PubMed]
- Chen Z, Wang Z, Lohman T, Heymsfield SB, Outwater E, Nicholas JS, Bassford T, LaCroix A, Sherrill D, Punyanitya M, Wu G, Going S. Dual-energy X-ray absorptiometry is a valid tool for assessing skeletal muscle mass in older women. J Nutr 2007;137:2775-80. [Crossref] [PubMed]
- Clark RV, Walker AC, Miller RR, O'Connor-Semmes RL, Ravussin E, Cefalu WT. Creatine (methyl-d(3)) dilution in urine for estimation of total body skeletal muscle mass: accuracy and variability vs. MRI and DXA. J Appl Physiol (1985) 2018;124:1-9. [Crossref] [PubMed]
- Neira Álvarez M, Vázquez Ronda MA, Soler Rangel L, Thuissard-Vasallo IJ, Andreu-Vazquez C, Martinez Martin P, Rábago Lorite I, Serralta San Martín G. Muscle Assessment by Ultrasonography: Agreement with Dual-Energy X-Ray Absorptiometry (DXA) and Relationship with Physical Performance. J Nutr Health Aging 2021;25:956-63. [Crossref] [PubMed]
- Abe T, Loenneke JP, Thiebaud RS, Fujita E, Akamine T, Loftin M. Prediction and Validation of DXA-Derived Appendicular Fat-Free Adipose Tissue by a Single Ultrasound Image of the Forearm in Japanese Older Adults. J Ultrasound Med 2018;37:347-53. [Crossref] [PubMed]
- Chen YL, Liu PT, Chiang HK, Lee SH, Lo YL, Yang YC, Chiou HJ. Ultrasound Measurement of Rectus Femoris Muscle Parameters for Discriminating Sarcopenia in Community-Dwelling Adults. J Ultrasound Med 2022;41:2269-77. [Crossref] [PubMed]
- Morimoto A, Suga T, Tottori N, Wachi M, Misaki J, Tsuchikane R, Isaka T. Association between hand muscle thickness and whole-body skeletal muscle mass in healthy adults: a pilot study. J Phys Ther Sci 2017;29:1644-8. [Crossref] [PubMed]
- Yuguchi S, Asahi R, Kamo T, Azami M, Ogihara H. Prediction Model including Gastrocnemius Thickness for the Skeletal Muscle Mass Index in Japanese Older Adults. Int J Environ Res Public Health 2022;19:4042. [Crossref] [PubMed]
- Aleixo GFP, Shachar SS, Nyrop KA, Muss HB, Battaglini CL, Williams GR. Bioelectrical Impedance Analysis for the Assessment of Sarcopenia in Patients with Cancer: A Systematic Review. Oncologist 2020;25:170-82. [Crossref] [PubMed]
- Sousa-Santos AR, Barros D, Montanha TL, Carvalho J, Amaral TF. Which is the best alternative to estimate muscle mass for sarcopenia diagnosis when DXA is unavailable? Arch Gerontol Geriatr 2021;97:104517. [Crossref] [PubMed]
- Reiss J, Iglseder B, Kreutzer M, Weilbuchner I, Treschnitzer W, Kässmann H, Pirich C, Reiter R. Case finding for sarcopenia in geriatric inpatients: performance of bioimpedance analysis in comparison to dual X-ray absorptiometry. BMC Geriatr 2016;16:52. [Crossref] [PubMed]
- Bosaeus I, Wilcox G, Rothenberg E, Strauss BJ. Skeletal muscle mass in hospitalized elderly patients: comparison of measurements by single-frequency BIA and DXA. Clin Nutr 2014;33:426-31. [Crossref] [PubMed]
- Lee SY, Ahn S, Kim YJ, Ji MJ, Kim KM, Choi SH, Jang HC, Lim S. Comparison between Dual-Energy X-ray Absorptiometry and Bioelectrical Impedance Analyses for Accuracy in Measuring Whole Body Muscle Mass and Appendicular Skeletal Muscle Mass. Nutrients 2018;10:738.
- Anderson LJ, Erceg DN, Schroeder ET. Utility of multifrequency bioelectrical impedance compared with dual-energy x-ray absorptiometry for assessment of total and regional body composition varies between men and women. Nutr Res 2012;32:479-85. [Crossref] [PubMed]
- Orsso CE, Silva MIB, Gonzalez MC, Rubin DA, Heymsfield SB, Prado CM, Haqq AM. Assessment of body composition in pediatric overweight and obesity: A systematic review of the reliability and validity of common techniques. Obes Rev 2020;21:e13041. [Crossref] [PubMed]
- Bosy-Westphal A, Jensen B, Braun W, Pourhassan M, Gallagher D, Müller MJ. Quantification of whole-body and segmental skeletal muscle mass using phase-sensitive 8-electrode medical bioelectrical impedance devices. Eur J Clin Nutr 2017;71:1061-7. [Crossref] [PubMed]
- Gonzalez MC, Barbosa-Silva TG, Heymsfield SB. Bioelectrical impedance analysis in the assessment of sarcopenia. Curr Opin Clin Nutr Metab Care 2018;21:366-74.
- Earthman CP. Body Composition Tools for Assessment of Adult Malnutrition at the Bedside: A Tutorial on Research Considerations and Clinical Applications. JPEN J Parenter Enteral Nutr 2015;39:787-822. [Crossref] [PubMed]
- Wang J, Hu Y, Tian G. Ultrasound measurements of gastrocnemius muscle thickness in older people with sarcopenia. Clin Interv Aging 2018;13:2193-9. [Crossref] [PubMed]
- Tagliafico AS, Bignotti B, Torri L, Rossi F. Sarcopenia: how to measure, when and why. Radiol Med 2022;127:228-37. [Crossref] [PubMed]
- Kuyumcu ME, Halil M, Kara Ö, Çuni B, Çağlayan G, Güven S, Yeşil Y, Arık G, Yavuz BB, Cankurtaran M, Özçakar L. Ultrasonographic evaluation of the calf muscle mass and architecture in elderly patients with and without sarcopenia. Arch Gerontol Geriatr 2016;65:218-24. [Crossref] [PubMed]
- Pedrianes-Martin PB, Hernanz-Rodriguez GM, Gonzalez-Martin JM, Perez-Valera M, De Pablos-Velasco PL. Ultrasonographic Size of the Thenar Muscles of the Nondominant Hand Correlates with Total Body Lean Mass in Healthy Subjects. Acad Radiol 2021;28:517-23. [Crossref] [PubMed]
- Zhao R, Li X, Jiang Y, Su N, Li J, Kang L, Zhang Y, Yang M. Evaluation of Appendicular Muscle Mass in Sarcopenia in Older Adults Using Ultrasonography: A Systematic Review and Meta-Analysis. Gerontology 2022;68:1174-98. [Crossref] [PubMed]
- Tavoian D, Ampomah K, Amano S, Law TD, Clark BC. Changes in DXA-derived lean mass and MRI-derived cross-sectional area of the thigh are modestly associated. Sci Rep 2019;9:10028. [Crossref] [PubMed]
- Xu L, Cheng X, Wang J, Cao Q, Sato T, Wang M, Zhao X, Liang W. Comparisons of body-composition prediction accuracy: a study of 2 bioelectric impedance consumer devices in healthy Chinese persons using DXA and MRI as criteria methods. J Clin Densitom 2011;14:458-64. [Crossref] [PubMed]
- Lee SY, Kim DH, Park SJ, Park J, Chung SG, Lim JY. Novel lateral whole-body dual-energy X-ray absorptiometry of lumbar paraspinal muscle mass: results from the SarcoSpine study. J Cachexia Sarcopenia Muscle 2021;12:913-20. [Crossref] [PubMed]
- Yoo HJ, Kim YJ, Hong H, Hong SH, Chae HD, Choi JY. Deep learning-based fully automated body composition analysis of thigh CT: comparison with DXA measurement. Eur Radiol 2022;32:7601-11. [Crossref] [PubMed]
- Tsukasaki K, Matsui Y, Arai H, Harada A, Tomida M, Takemura M, Otsuka R, Ando F, Shimokata H. Association of Muscle Strength and Gait Speed with Cross-Sectional Muscle Area Determined by Mid-Thigh Computed Tomography - A Comparison with Skeletal Muscle Mass Measured by Dual-Energy X-Ray Absorptiometry. J Frailty Aging 2020;9:82-9. [Crossref] [PubMed]
- Cruz-Jentoft AJ, Sayer AA. Sarcopenia. Lancet 2019;393:2636-46. [Crossref] [PubMed]
- Cruz-Jentoft AJ, Baeyens JP, Bauer JM, Boirie Y, Cederholm T, Landi F, Martin FC, Michel JP, Rolland Y, Schneider SM, Topinková E, Vandewoude M, Zamboni MEuropean Working Group on Sarcopenia in Older People. Sarcopenia: European consensus on definition and diagnosis: Report of the European Working Group on Sarcopenia in Older People. Age Ageing 2010;39:412-23. [Crossref] [PubMed]
- Guerri S, Mercatelli D, Aparisi Gómez MP, Napoli A, Battista G, Guglielmi G, Bazzocchi A. Quantitative imaging techniques for the assessment of osteoporosis and sarcopenia. Quant Imaging Med Surg 2018;8:60-85. [Crossref] [PubMed]
- Gonzalez MC, Heymsfield SB. Bioelectrical impedance analysis for diagnosing sarcopenia and cachexia: what are we really estimating? J Cachexia Sarcopenia Muscle 2017;8:187-9. [Crossref] [PubMed]