Comparison of deep-learning and radiomics-based machine-learning methods for the identification of chronic obstructive pulmonary disease on low-dose computed tomography images
Introduction
Chronic obstructive pulmonary disease (COPD) is a life-threatening incurable lung disease; however, medical and physical treatments can help to relieve symptoms and improve patients’ quality of life (1). As a complex and extremely heterogeneous disease, COPD is challenging to identify in the early stages. The pulmonary function test (PFT) is used to diagnose COPD. However, a considerable proportion of COPD patients are underdiagnosed due to the current diagnostic criteria (2).
Chest low-dose computed tomography (LDCT) is increasingly being used for lung cancer screening in high-risk populations; thus, there is an opportunity to use computed tomography (CT) scans to identify COPD. Quantitative computed tomography (QCT) has increasingly been used in the evaluation of COPD, as CT features can suggest the presence and severity of emphysema, airway disease, and pulmonary vessel disease (3-5). However, the use of QCT in COPD diagnosis is prone to variability between doctors and is time consuming. In addition, other imaging findings in COPD should also be considered, such as bronchiectasis and mucous plugging. The above-mentioned disadvantages limit the clinical application of QCT in the diagnosis of COPD.
Compared with specific quantification methods, the radiomics and artificial intelligence (AI) approaches aim to analyze all the information from CT imaging. The AI approach, which includes machine learning (ML) and deep learning (DL), refers to the simulation of human intelligence by computer systems. Convolutional neural networks (CNNs) are becoming a mainstream DL method and have made remarkable achievements in medical imaging (6,7). The CT radiomics approach could potentially quantify COPD and reveal the disease’s underlying mechanism. Thousands of quantitative radiomics features can be extracted from each image and further analyzed using ML tools to predict COPD and disease progress (8). In this context, several potential clinical applications for radiomics features and AI in COPD have been suggested. The diagnosis of COPD by AI or radiomics mainly relies on clinical information, CT imaging, or a combination of clinical and imaging characterization. Previous studies have shown that radiomics and AI models based on CT images can be used to distinguish COPD from non-COPD, but the performance of such models has varied (9-11). Sun et al. (12) used a weakly supervised DL method based on CT images to detect COPD. The model achieved an area under the receiver operating characteristic (ROC) curve (AUC) of 0.934 [95% confidence interval (CI): 0.903–0.961] on the internal test set, and 0.866 (95% CI: 0.805–0.928) on the external validation LDCT subset. Models that combine image features with AI have achieved good results in COPD detection. Tang et al. (13) examined the use of a DL approach based on residual networks to detect signs of COPD on LDCT, and the best model achieved an AUC of 0.889 (standard deviation: 0.017). They concluded that this approach provided a powerful technique for identifying patients within the general population. Li et al. (14) evaluated the role of two radiomics classification CT-based methods in the identification of COPD, and the models achieved AUCs of 0.970 (95% CI: 0.964–0.977) and 0.972 (95% CI: 0.969–0.975) in the test set, respectively. Another recent study (15) assessed the performance of radiomics features in COPD detection using CT images and reported an AUC of 0.90 (95% CI: 0.89–0.92) in the standard-dose CT model, and an AUC of 0.87 (95% CI: 0.83–0.91) in the LDCT model. Many investigations have developed approaches to predict COPD based on CT radiomics and AI; however, it is still unclear which approach has the best performance and could be most beneficial to apply as a clinical decision support system.
Therefore, we established and compared five prediction models, including models that employed CT images, lung density parameters, and demographic and clinical characteristics using DL and radiomics-based ML approaches, and determined the optimal models for the identification of COPD on LDCT images. We present this article in accordance with the TRIPOD reporting checklist (available at https://qims.amegroups.com/article/view/10.21037/qims-23-1307/rc).
Methods
Data set creation
Subjects
We conducted a population-based CT screening study for the early detection of lung cancer, COPD, and cardiovascular disease. Specifically, we retrospectively collected the data of consecutive subjects who were screened using LDCT for the big-three diseases (NELCIN-B3, ClinicalTrials.gov, and NCT03988322) from August 2018 to July 2021 at the Second Affiliated Hospital, Navy Medical University (Shanghai, China) (16). The epidemiological data of the subjects were collected through questionnaires. PFTs (HI-801 Chestgraph, CHEST M.I., Inc., Tokyo, Japan) were performed at the baseline screening. To balance the number of subjects among groups, the data of non-COPD subjects were collected until February 2019, and the data of the COPD subjects were collected until July 2021.
To be eligible for inclusion in this study, the patients had to meet the following inclusion criteria: have completed the questionnaires, PFT, and LDCT on the same day. Patients were excluded from the study if they met any of the following exclusion criteria: (I) had marked respiratory or heartbeat motion, or metal artifacts on their CT images; (II) had a CT image thickness >1 mm; (III) had an obvious lung disease, such as a lung mass, severe pulmonary interstitial fibrosis, or massive pulmonary infection; and/or (IV) had a thoracic deformity. The subjects were divided into the following two subgroups based on the PFT results: (I) the non-COPD group [forced expiratory volume in 1 second (FEV1)/forced vital capacity (FVC) ≥0.7]; and (II) the COPD group (FEV1/FVC <0.7). The inclusion and exclusion flowchart for the study is shown in Figure 1. In total, 1,024 subjects were included in this study. These subjects were further randomly divided into a training set, a validation set, and a test set at a ratio of approximately 6:1:3 (n=622 for the training set, n=104 for the validation set, and n=298 for the test set). The data from the training set were used for the initial model development, the data from the validation set were used to optimize the AI models, and the data from the test set were used for the model evaluation. In this study, the term “developmental set” refers to the data sets used during model development, including the training set and validation set. The patient demographics for the subjects in the developmental and test sets are shown in Table 1.
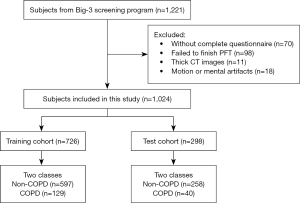
Table 1
Characteristics | Developmental set (n=726) | Test set (n=298) | |||||||||||||
---|---|---|---|---|---|---|---|---|---|---|---|---|---|---|---|
COPD (n=129) | Non-COPD (n=597) | P | COPD (n=40) | Non-COPD (n=258) | P | ||||||||||
Basic information | |||||||||||||||
Age (years) | 66 [61, 69] | 68 [65, 70] | <0.01 | 68 [65, 70.75] | 68 [65, 71] | 0.953 | |||||||||
Gender | <0.01 | 0.001 | |||||||||||||
Female | 46 | 348 | 12 | 147 | |||||||||||
Male | 83 | 249 | 28 | 111 | |||||||||||
Level of education | 0.218 | 0.012 | |||||||||||||
Never | 0 | 5 | 0 | 3 | |||||||||||
Primary school | 6 | 17 | 2 | 2 | |||||||||||
Junior school | 44 | 209 | 14 | 93 | |||||||||||
Senior high school | 50 | 218 | 20 | 94 | |||||||||||
College/junior college | 27 | 147 | 3 | 65 | |||||||||||
Graduate | 2 | 1 | 1 | 1 | |||||||||||
Behavior factors | |||||||||||||||
Smoking history | 0.001 | 0.016 | |||||||||||||
Never | 73 | 435 | 20 | 184 | |||||||||||
Ex-smoker | 12 | 37 | 6 | 18 | |||||||||||
Current smoker | 44 | 125 | 14 | 56 | |||||||||||
Average cigarettes per day | <0.001 | 0.008 | |||||||||||||
Never | 73 | 435 | 20 | 184 | |||||||||||
≤1 pack | 45 | 138 | 15 | 64 | |||||||||||
>1 pack | 11 | 24 | 5 | 10 | |||||||||||
Environmental factors | |||||||||||||||
Exposure to second-hand smoke at least 1 day per week for more than 15 minutes | 0.578 | 0.225 | |||||||||||||
No | 96 | 458 | 30 | 214 | |||||||||||
Yes | 33 | 139 | 10 | 44 | |||||||||||
Whether the kitchen filled with smoke during cooking | 0.093 | 0.213 | |||||||||||||
No smoke | 25 | 113 | 7 | 54 | |||||||||||
A little smoke | 96 | 400 | 29 | 176 | |||||||||||
Moderate smoke | 7 | 75 | 3 | 28 | |||||||||||
Heavy smoke | 1 | 9 | 1 | 0 | |||||||||||
The house is on the street or in the larger vehicle way | 0.478 | 0.325 | |||||||||||||
No | 87 | 383 | 23 | 169 | |||||||||||
Yes | 42 | 214 | 17 | 89 | |||||||||||
In the past year, did you use air purification equipment at least 3 times a week at home and at least 30 minutes at a time? | 0.325 | 0.803 | |||||||||||||
Yes | 9 | 63 | 3 | 25 | |||||||||||
Seldomly | 12 | 41 | 1 | 13 | |||||||||||
No | 108 | 493 | 36 | 220 | |||||||||||
Dust exposure | 0.796 | 1.00 | |||||||||||||
No | 124 | 576 | 39 | 249 | |||||||||||
Yes | 5 | 21 | 1 | 9 | |||||||||||
Family history | |||||||||||||||
Family history of lung cancer | 0.92 | 0.806 | |||||||||||||
No | 115 | 534 | 34 | 223 | |||||||||||
Yes | 14 | 63 | 6 | 35 | |||||||||||
Family history of emphysema | 0.465 | 0.03 | |||||||||||||
No | 113 | 536 | 31 | 231 | |||||||||||
Yes | 16 | 61 | 9 | 27 | |||||||||||
Disease symptoms | |||||||||||||||
Cough when weather changes | 0.005 | 0.448 | |||||||||||||
No | 83 | 456 | 27 | 189 | |||||||||||
Yes | 46 | 141 | 13 | 69 | |||||||||||
Frequent wheeze | 0.025 | 0.041 | |||||||||||||
Never | 77 | 417 | 23 | 189 | |||||||||||
Occasionally or often | 52 | 180 | 17 | 69 | |||||||||||
Allergic history | 0.803 | 0.645 | |||||||||||||
No | 106 | 496 | 31 | 208 | |||||||||||
Yes | 23 | 101 | 9 | 50 | |||||||||||
Frequent cough | 0.011 | 0.07 | |||||||||||||
No | 102 | 523 | 31 | 227 | |||||||||||
Yes | 27 | 74 | 9 | 31 | |||||||||||
Chronic respiratory disease | 0.02 | 0.739 | |||||||||||||
No | 115 | 565 | 37 | 241 | |||||||||||
Yes | 14 | 32 | 3 | 17 | |||||||||||
Subjective health status scale (100 represents the best state of health, and 0 represents the worst state of health) |
0.65 | 0.758 | |||||||||||||
Score ≥80 | 89 | 384 | 28 | 170 | |||||||||||
50≤ score <80 | 39 | 207 | 12 | 87 | |||||||||||
Score <50 | 1 | 6 | 0 | 1 | |||||||||||
Lung density parameters based on CT | |||||||||||||||
Whether LAA% ≥6 | <0.001 | <0.001 | |||||||||||||
No | 73 | 457 | 16 | 180 | |||||||||||
Yes | 56 | 140 | 24 | 78 | |||||||||||
Volume (mL) | 5,013.74 [4,084.59, 5,797.94] |
4,145.17 [3,468.51, 4,920.58] |
<0.01 | 5,083.58 [4,012.02, 6,037.80] |
4,219.98 [3,432.16, 5,060.94] |
<0.01 | |||||||||
Mean lung density (HU) | −849.20 [−862.16, −830.58] |
−835.23 [−849.93, −814.98] |
<0.01 | −852.03 [−866.11, −838.99] |
−837.98 [−852.00, −817.23] |
<0.01 | |||||||||
Skewness | 2.80 [2.49, 3.13] | 2.78 [2.47, 3.02] | 0.191 | 2.46 [2.35, 2.95] | 2.84 [2.45, 3.05] | 0.027 | |||||||||
Kurtosis | 13.45 [10.76, 15.74] | 13.19 [10.74, 14.85] | 0.32 | 10.52 [9.68, 14.64] | 13.65 [10.74, 15.11] | 0.01 | |||||||||
Excess kurtosis | 10.34 [7.76, 12.74] | 10.19 [7.74, 11.85] | 0.32 | 7.52 [6.68, 11.64] | 10.65 [7.74, 12.11] | 0.01 | |||||||||
LAA volume (mL) | 260.52 [124.76, 535.54] | 138.78 [64.25, 271.86] | <0.01 | 318.61 [170.82, 928.94] | 155.10 [62.17, 335.29] | <0.01 | |||||||||
LAA% | 5.36 [2.50, 9.62] | 3.41 [1.76, 5.75] | <0.01 | 6.78 [2.97, 14.09] | 3.77 [1.76, 6.55] | <0.01 | |||||||||
PI-1 (HU) | −977 [−990.5, −96.5] | −971 [−980, −959] | <0.01 | −988 [−1,006.5, −968.25] | −972 [−982, −959] | <0.01 | |||||||||
PI-5 (HU) | −951 [−964, −937.5] | −942 [−952, −930] | <0.01 | −956.5 [−974.5, −940.25] | −944 [−955, −930] | <0.01 | |||||||||
PI-10 (HU) | −937 [−949, −924] | −928 [−938, −914] | <0.01 | −940.5 [−958.25, −924.25] | −929 [−941, −915] | <0.01 | |||||||||
PI-15 (HU) | −928 [−939.5, −912.5] | −918 [−929, −903] | <0.01 | −930 [−948, −915] | −918 [−931, −905] | <0.01 | |||||||||
PI-20 (HU) | −920 [−932, −904] | −909 [−921, −894] | <0.01 | −921.5 [−939, −907.25] | −910 [−924, −896.75] | <0.01 | |||||||||
PI-25 (HU) | −914 [−925, −897] | −902 [−914, −886] | <0.01 | −915 [−931.75, −900.5] | −903 [−917, −888.75] | <0.01 | |||||||||
PI-30 (HU) | −907 [−919, −890.5] | −895 [−908, −879] | <0.01 | −908 [−924.5, −894.25] | −896 [−910, −881.75] | <0.01 | |||||||||
PI-35 (HU) | −900 [−913, −884.5] | −889 [−902, −872] | <0.01 | −902 [−917.5, −888.25] | −890 [−903.25, −875] | <0.01 | |||||||||
PI-40 (HU) | −894 [−906, −877.5] | −882 [−896, −865] | <0.01 | −895.5 [−911.25, −882.25] | −884 [−897.25, −868] | 0.01 |
The data are presented as the median [interquartile range]. PI: CT attenuation values at a certain percentile of the CT histogram. COPD, chronic obstructive pulmonary disease; CT, computed tomography; LAA%, low attenuation area percentage; HU, Hounsfield units.
The study was conducted in accordance with the Declaration of Helsinki (as revised in 2013). The study was approved by the Ethics Committee of Changzheng Hospital, Naval Medical University, Shanghai, China, and the study was registered in the Chinese Clinical Trials Registry (http://www.chictr.org.cn/index.aspx; ChiCTR2000035283). All the subjects provided written informed consent for participating in this study.
Questionnaires
The questionnaire included questions related to basic information, behavior factors, environmental factors, family history, and disease symptoms. In total, 18 demographic and clinical characteristics were selected for the data analysis. The demographic and clinical characteristics included age, sex, education level, smoking history, and average cigarettes per day.
CT scanning
All the subjects were screened with a craniocaudal LDCT, lying supine with both arms raised. A 256-slice CT (Brilliance-iCT, Philips Healthcare, Eindhoven, The Netherlands) was used to obtain the CT images according to the NELCIN-B3 CT scan protocol. The subjects underwent breath-hold training before the CT scanning. No contrast-enhanced volumetric chest CT scanning was performed at the end of inspiration and expiration from the thoracic inlet to the diaphragm. The acquisition and reconstruction parameters for the chest CT scanning were as follows: collimation: 128×0.625 mm; tube energy: 120 kV; tube current modulation: Z-axial and 3D automatic; Doseright collimator (Philips Healthcare): on; reduced dose level: 3; pitch: 0.915; slice thickness: 1 mm; slice increment: 1 mm; field of view: 350 mm × 350 mm; matrix: 512×512; and algorithms: high and standard resolution.
CT lung density measurement
The lung density parameters were analyzed using commercial software (A-VIEW, Suhai Alderi Information Technology Ltd., Dubai, UAE). A lung parenchyma area with an attenuation value less than −950 Hounsfield units was defined as a low attenuation area (LAA). The percentage of the LAA of the whole lung relative to the total volume was automatically calculated as the LAA%. CT attenuation values at the 1st (PI-1), 5th (PI-5), 10th (PI-10), 15th (PI-15), 20th (PI-20), 25th (PI-25), 30th (PI-30), 35th (PI-35), and 40th (PI-40) percentiles of the CT histogram were automatically acquired by software. The volume of the whole lung, mean lung density, the skewness, kurtosis, and excess kurtosis of the CT attenuation values were also recorded. Ultimately, 17 lung density parameters were selected for the data analysis.
Model development
All the ML and AL classification models were developed on the Python platform (Version 3.8.5, Python Software Foundation, USA) at a workstation equipped with an Intel Xeon Gold 5118 CPU and four NVIDIA GeForce RTX 2080 Ti GPUs. As stated above, all the AI models were trained with the training set data and optimized with the validation set data. As Figure 2 shows, a total of five AI models, including two ML models and three DL models, were developed in this study. The ML-based models included a model based solely on radiomics features and a model based on the combination of radiomics features and clinical features, while the DL-based models included a model based solely on CT images, a model integrating CT images and clinical and lung density features, and a model based on the combination of CT images, clinical and lung density features and radiomics features. Further details of these five models are provided below.
Extraction of radiomics features from the CT images
Before the radiomics image extraction, the lung region was segmented from the CT images. The radiomics features were extracted from the CT images using the Python package “pyradiomics” (17) (Version 3.0.1) on the Python programming platform. For each CT volume, 107 radiomics features, including 14 shape features, 18 first-order statistic features, 24 gray-level co-occurrence matrix features, 16 gray-level run length matrix features, 16 gray-level size zone matrix features, 14 gray-level dependence matrix features, and 5 neighboring gray-tone difference matrix features, were extracted from the segmented lung region. These radiomics features were further used to construct the radiomics-based ML classification models.
Radiomics-based ML methods for classification
The gradient boosting decision tree (GBDT) model (18) was adopted for the radiomics feature-based binary classification task. Two GBDT models were constructed using the “GradientBoostingClassifier” function from Scikit-Learn Toolkit (19) (Version 0.32.2): Model 1 employed 107 radiomics features only, while model 2 employed a combination of the 107 radiomics features, 17 lung density features, and 18 demographic and clinical characteristics based on the questionnaire administered to the subjects. During the training process, we used all the features without selection. To optimize the GBDT model, we used a grid search strategy to tune the hyperparameters in an empirical range. By grid searching, we constructed the optimal model with 40 decision trees (n_estimators =40), a learning rate of 0.01 (learning_rate =0.01), the number of features to consider when looking for the best split set as the logarithm to the base 2 of the feature numbers (max_features = ‘log2’), the maximum depth of the individual estimator of 5 (max_depth =5), the minimum number of samples required to be at a leaf node of 11 (min_samples_leaf =11), the fraction of samples to be used to fit the individual base learners of 0.85 (subsample =0.85), and the loss function of log loss (loss = ‘log_loss’). Further, given the class imbalance between the COPD and non-COPD samples, we put a weight of 0.8 on the COPD samples and a weight of 0.2 on the non-COPD samples during the training process, so that the classification model could better fit the minority class (i.e., the COPD samples).
Development of image-based DL models for COPD classification
In addition to the radiomics feature-based classification models, we also constructed three DL classification models based on CT images. Model 3 is based only on CT images; Model 4 is based on a combination of CT images, 17 lung density features, and 18 demographic and clinical characteristics from the questionnaire administered to the subjects; Model 5 is based on a combination of the CT images, 107 radiomics features, 17 lung density features, and 18 demographic and clinical characteristics. These three DL models were constructed using the DL framework PyTorch (20) (Version 1.10.2), and Model 3 is based on the ResNeXt50 (21) backbone, which is a CNN using group convolution strategy for improving model performance.
To use the lung density parameters, demographic and clinical characteristics, and/or radiomics features in the DL models, we developed a CNN called DualNet that integrates multimodal information, including the CT images, lung density parameters, demographic and clinical characteristics, and the selected radiomics features for the classification task. As Figure 3 shows, DualNet is a combination of the ResNet50 (22) and Ext-Attention (23) networks. ResNet50 encodes the CT image features, while the Ext-Attention network encodes the lung density parameters, demographic and clinical characteristics, and/or radiomics features. Both ResNet50 and Ext-Attention form a 1,024-dimentional eigenvector, and these eigenvectors are further concatenated and passed to the fully connected layer for the final classification. Models 4 and 5 were both constructed with the DualNet backbone.
Before the training process for the DL models, we preprocessed the images by first segmenting the lung region from the CT volumes, and then augmenting the segmented lung region images by random vertical and horizontal flipping and random rotation. During the training process, we consistently employed Focal Loss or its variants as the loss function for the DL models. Additionally, to address the class imbalance between the COPD and non-COPD data, we balanced the weights of the sample categories and mitigated the data imbalance effect by using a weighted random sampler during the data loading to achieve superior training effectiveness. Adam was employed as the optimizer during training, with exponential decay rates for parameters B1 and B2 set at 0.9 and 0.999, respectively. The epsilon parameter was fixed at 10e−8, and the initial learning rate was set to 0.0005. Before training, weight initialization in the CNN model was performed using Kaiming initialization. Subsequently, the data were randomly shuffled and fed into the CNN, with a batch size of 32 for each iteration.
Model evaluation
The classification performance of all our classification models was evaluated on the test set data. To evaluate the classification performance, we plotted ROC curves and calculated the areas under the ROC curves (AUCs) as the quantitative metrics. Under the optimal classification threshold (a.k.a. the operating point) where the Youden Index (sensitivity + specificity − 1) reaches the maximum, we calculated the accuracy, sensitivity, and specificity of the models in terms of classification. Calibration curves were used to evaluate the calibration of the prediction model with the best performance.
Statistical analysis
All the statistical analyses were performed using the R language platform (Version 4.0.0, R Foundation for Statistical Computing, Vienna, Austria). To compare the distribution of the categorical variables between the COPD and non-COPD data, we used the Chi-squared test. To compare the distribution of the quantitative variables between the COPD and non-COPD data, we first tested if the data distribution followed a normal distribution using the Shapiro-Wilk Test. If the distribution was normal (P>0.05 for the Shapiro-Wilk test), we used the Student’s t-test for further analysis; otherwise, the Mann-Whitney U test was used. In terms of the classification model performance, we compared the accuracy, sensitivity, specificity, the highest negative predictive value (NPV), positive predictive value (PPV), and AUC values of different models using the DeLong test. In all the statistical analysis, a P value less than 0.05 was considered statistically significant.
Results
COPD and non-COPD subjects’ demographic and clinical characteristics
A total of 1,024 subjects were included in this study, of whom 169 were COPD patients. The subjects were randomly divided into a developmental set (n=726, comprising a training set of 622 patients and a validation set of 104 patients) and a test set (n=298) for the model establishment and model evaluation, respectively. The patient demographics, clinical characteristics, and lung density parameters for the developmental set and the test set are listed in Table 1. The age of subjects in the non-COPD group was greater than that in the COPD group, and there was a significant difference between the two groups in terms of age (P<0.01) and gender (P<0.01) in the developmental set. However, no significant difference was found between the two groups in terms of age and gender in the test set (P=0.953). In addition, significant differences were observed between the COPD group and non-COPD group in terms of smoking history (P=0.001; P=0.016) and average cigarettes per day (P<0.001; P=0.008) for the developmental set and test set, respectively. Statistical differences were also observed between two groups in all the lung density parameters for the test set (all P<0.05).
Model performance for classification task
The performance results of our five classification models are set out in Table 2, and the corresponding ROC curves of these models are shown in Figure 4. In relation to the performance of the five models on the training set, Model 1 achieved the highest performance (AUC =0.95, 95% CI: 0.94–0.97) and performed significantly better than Model 3 (P<0.001), Model 4 (P<0.001), and Model 5 (P=0.004). There were no significant differences between Model 1 and Model 2 in terms of their classification performance (AUC =0.95, 95% CI: 0.94–0.97 vs. AUC =0.94, 95% CI: 0.92–0.97; P=0.13). Therefore, the classification performance of Models 1 and 2 was equal on the training set. In relation to the performance of the five models on the test set, the accuracy scores ranged from 0.64 to 0.81, the sensitivity scores ranged from 0.63 to 0.84, the specificity scores ranged from 0.50 to 0.73, and the AUC values ranged from 0.65 to 0.73. Model 2 had the highest AUC (0.73, 95% CI: 0.64–0.82), while Model 3 had the lowest AUC (0.65, 95% CI: 0.55–0.75). The AUC results showed that Model 2 performed significantly better than Model 1 (P=0.03). It also had the highest sensitivity (0.84), the highest accuracy (0.81), and the highest NPV (0.36) of the five models. The Hosmer-Lemeshow test results suggested Model 2 had adequate goodness of fit for predicting COPD in the training and test sets (P=0.322 and P=0.531, respectively).
Table 2
Model | Accuracy | Sensitivity | Specificity | NPV | PPV | AUC (95% CI) | P value† |
---|---|---|---|---|---|---|---|
Test set | |||||||
Model 1 | 0.72 | 0.74 | 0.60 | 0.27 | 0.92 | 0.66 (0.57–0.76) | 0.03* |
Model 2 | 0.81‡ | 0.84‡ | 0.58 | 0.36‡ | 0.93 | 0.73 (0.64–0.82)‡ | Reference |
Model 3 | 0.70 | 0.72 | 0.55 | 0.23 | 0.91 | 0.65 (0.55–0.75) | 0.26 |
Model 4 | 0.78 | 0.82 | 0.50 | 0.30 | 0.91 | 0.69 (0.59–0.78) | 0.52 |
Model 5 | 0.64 | 0.63 | 0.73‡ | 0.23 | 0.94‡ | 0.70 (0.61–0.79) | 0.61 |
Training set | |||||||
Model 1 | 0.82 | 0.79 | 0.97‡ | 0.49 | 0.99‡ | 0.95 (0.94–0.97)‡ | Reference |
Model 2 | 0.89‡ | 0.91‡ | 0.84 | 0.64‡ | 0.97 | 0.94 (0.92–0.97) | 0.13 |
Model 3 | 0.80 | 0.81 | 0.76 | 0.45 | 0.94 | 0.86 (0.82–0.90) | <0.001* |
Model 4 | 0.78 | 0.77 | 0.83 | 0.42 | 0.96 | 0.87 (0.84–0.90) | <0.001* |
Model 5 | 0.85 | 0.85 | 0.85 | 0.53 | 0.96 | 0.90 (0.87–0.93) | 0.004* |
†, the P value is for the DeLong test between the classification model and the reference model for AUC; ‡, the highest metric among all five models. *, P<0.05. NPV, negative predictive value; PPV, positive predictive value; AUC, area under the receiver operating characteristic curve; CI, confidence interval.
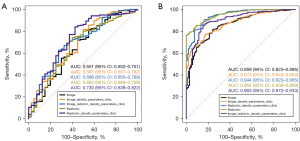
Discussion
In this study, we designed and evaluated five prediction models based on a radiomics-based ML method and a CT image-based DL method to detect COPD in a general population who underwent LDCT scans for the screening of three-big diseases. A COPD identification model with high sensitivity and specificity could effectively provide clinical treatment support for physicians. With the development of AI, several studies on COPD identification based on CT imaging have achieved satisfactory results; recently, some DL models trained from chest CT images and CT-based extracted radiomics features have been used to identify and classify COPD (24,25). However, to the best of our knowledge, few studies have compared the performance of DL models based on images to that of ML models based on lung radiomics features in identifying COPD.
The results of our experiments showed that Model 2, which employed a combination of CT-based radiomics features, lung density parameters, and demographic and clinical characteristics using a ML method, achieved an AUC of 0.73 (95% CI: 0.64–0.82) in the test set. It had the best performance among the five models. It also had the highest sensitivity (0.84), the highest accuracy (0.81), and the highest NPV (0.36). Conversely, Model 3, which used DL based on CT images, had the lowest AUC of 0.65 (95% CI: 0.55–0.75) in the test set. Among the five models, Model 2 had the best COPD identification capability. Therefore, compared with the DL models based on the chest LDCT images, the ML method based on the use of lung radiomics features had the best performing modality for COPD identification in this study.
There was a high-class imbalance among the patients included in our study. There were only 169 COPD subjects, while there were 855 non-COPD subjects. Such a class imbalance is common and typical in clinical scenarios, especially for COPD screening with LDCT. However, class imbalance can be a problem for AI model training, as it might result in a classification model biased to the majority class. Therefore, from a technical point of view, any such class imbalance needs to be handled with appropriate strategies to avoid classification bias. In this study, we used higher class weights for the minority class in the training process of the ML models, and we used a weighted random sampler during the data loading to ensure class balance in the training data batches, so that we could correct the model bias in the classification task. We also used the class imbalance insensitive metric of the AUC in the model evaluation, so that the models could be fairly evaluated despite the class imbalance.
Our results are consistent with those of Yang et al. (26), who found that the classification ability of lung radiomics features based on ML methods was better than that of chest high-resolution CT images based on classic CNNs for COPD stage classification. However, there were two differences between the two studies. First, while both studies compared the performance of ML and DL methods for COPD diagnostic applications, the present study explored the performance of COPD identification, while Yang et al. investigated COPD stage classification; Second, Yang et al. selected the best classifier from the different ML methods to construct a lung radiomics model to characterize COPD stage, while we constructed a lung radiomics model combined with lung density parameters, and demographic and clinical characteristics. In our study, we found that the identification and classification ability of the ML method based on the images was better than that of the DL method based on the lung radiomics features. There may be a number of reasons for this. First, DL requires a large number of labeled training data and rich experience, so it cannot achieve a satisfactory performance with a relatively small amount of training data, such as that in this study. Further, due to the “black box” feature of DL, the result may be interpreted inappropriately because it is unknown what was learned from the images. Second, lung radiomics can focus more on extracting the features from the lung region than the original chest CT images. Additionally, the lung density parameters were combined with the radiomics model to achieve better identification results in our study. This is because lung density can reflect parenchyma area abnormity to some extent. Therefore, when inputting the lung density parameters into the radiomics model (Model 2, AUC =0.73, 95% CI: 0.64–0.82), it achieved better COPD identification results than the radiomics-only model (Model 1, AUC =0.66, 95% CI: 0.57–0.76). A recent study of COPD identification by a ML support vector machine classifier had an AUC of 0.97 (95% CI: 0.964–0.977) in the test set (14). Another study for COPD detection by radiomics features showed that the radiomics features model had an AUC of 0.90 (95% CI: 0.89–0.92) in the standard-dose CT scans and an AUC of 0.87 (95% CI: 0.83–0.91) in the LDCT scans (15). The performance of the model was better than that of the models in our study. This may be because LDCT images and a different ML classifier were used in our study.
Many studies have confirmed that radiomics based on CT can improve COPD detection; however, the implementation of pyradiomics features in clinical chest CT scans is not yet practical. There may be a number of reasons for this (27,28). First, the feature selection step in ML approaches differs for heterogeneous scanning protocols and the resulting technical variabilities (e.g., different slice thicknesses, and the timings of contrast material administration) in the imaging data. Second, there is a lack of studies/development of any generalized model. Third, most of the current ML/DL algorithms are based on retrospective data, and very few ML/DL models have passed the clinical standard checkpoints. Finally, the performance of ML/DL models often diminish in uncontrolled real-world settings due to bias and a lack of generalization. Hence, such ML/DL models could face medio-legal issues.
The present study had a number of limitations that should be considered in the interpretation of the results. First, our models performed much better in the training set than the test set, which suggests a tendency of overfitting. Second, the sample sizes of 129 COPD patients in the development group and 40 COPD patients in the test group were not sufficient for the DL or radiomics-based COPD classification study, so studies with larger study populations need to be conducted. Third, this study did not include an external data set, and the applicability of models to the general population was unable to be evaluated, which should be studied further in the future. Further, spirometric triggering was not applied in the CT scan, therefore we cannot be completely sure that the imaging was obtained during maximum inspiration. Finally, as the presented models based on feature selection and segmentation choice were semi-automatic in nature, their performance is biased towards the particular choice of features or numeric values in the development phase. Therefore, the algorithm might perform well with retrospective data but fail to perform well with external data.
Conclusions
In conclusion, our results confirmed that CT image-based DL and radiomics-based ML models could be used to identify COPD on chest LDCT images and had acceptable performance. The ML methods based on CT-based radiomics features combined with lung density parameters, and demographic and clinical characteristics performed better than the chest CT image-based DL methods. Thus, these ML methods are more suitable and beneficial for COPD identification.
Acknowledgments
Funding: This work was supported by
Footnote
Reporting Checklist: The authors have completed the TRIPOD reporting checklist. Available at https://qims.amegroups.com/article/view/10.21037/qims-23-1307/rc
Conflicts of Interest: All authors have completed the ICMJE uniform disclosure form (available at https://qims.amegroups.com/article/view/10.21037/qims-23-1307/coif). Y.L., Xuebin Zheng, and C.H. are employees of Shanghai Aitrox Technology Corporation Limited, the AI company that assisted in the image analysis in this study. The other authors have no conflicts of interest to declare.
Ethical Statement: The authors are accountable for all aspects of the work in ensuring that questions related to the accuracy or integrity of any part of the work are appropriately investigated and resolved. The study was conducted in accordance with the Declaration of Helsinki (as revised in 2013). The study was approved by the Ethics Committee of Changzheng Hospital, Naval Medical University, Shanghai, China, and all the subjects provided written informed consent for participating in this study.
Open Access Statement: This is an Open Access article distributed in accordance with the Creative Commons Attribution-NonCommercial-NoDerivs 4.0 International License (CC BY-NC-ND 4.0), which permits the non-commercial replication and distribution of the article with the strict proviso that no changes or edits are made and the original work is properly cited (including links to both the formal publication through the relevant DOI and the license). See: https://creativecommons.org/licenses/by-nc-nd/4.0/.
References
- Global initiative for chronic obstructive lung disease [Internet]. Global strategy for the diagnosis, management, and prevention of chronic obstructive pulmonary disease (2024 REPORT). 2023 [cited 2023 Nov 15]. Available online: https://goldcopd.org/2024-gold-report/
- Rennard SI, Vestbo J. COPD: the dangerous underestimate of 15%. Lancet 2006;367:1216-9. [Crossref] [PubMed]
- Romei C, Castellana R, Conti B, Bemi P, Taliani A, Pistelli F, Karwoski RA, Carrozzi L, De Liperi A, Bartholmai B. Quantitative texture-based analysis of pulmonary parenchymal features on chest CT: comparison with densitometric indices and short-term effect of changes in smoking habit. Eur Respir J 2022;60:2102618. [Crossref] [PubMed]
- Park J, Hobbs BD, Crapo JD, Make BJ, Regan EA, Humphries S, Carey VJ, Lynch DA, Silverman EK. COPDGene Investigators. Subtyping COPD by Using Visual and Quantitative CT Imaging Features. Chest 2020;157:47-60. [Crossref] [PubMed]
- Kovacs G, Avian A, Bachmaier G, Troester N, Tornyos A, Douschan P, Foris V, Sassmann T, Zeder K, Lindenmann J, Brcic L, Fuchsjaeger M, Agusti A, Olschewski H. Severe Pulmonary Hypertension in COPD: Impact on Survival and Diagnostic Approach. Chest 2022;162:202-12. [Crossref] [PubMed]
- Zhou W, Cheng G, Zhang Z, Zhu L, Jaeger S, Lure FYM, Guo L. Deep learning-based pulmonary tuberculosis automated detection on chest radiography: large-scale independent testing. Quant Imaging Med Surg 2022;12:2344-55. [Crossref] [PubMed]
- Sun H, Ren G, Teng X, Song L, Li K, Yang J, Hu X, Zhan Y, Wan SBN, Wong MFE, Chan KK, Tsang HCH, Xu L, Wu TC, Kong FS, Wang YXJ, Qin J, Chan WCL, Ying M, Cai J. Artificial intelligence-assisted multistrategy image enhancement of chest X-rays for COVID-19 classification. Quant Imaging Med Surg 2023;13:394-416. [Crossref] [PubMed]
- Wang R, Huang C, Yang W, Wang C, Wang P, Guo L, Cao J, Huang L, Song H, Zhang C, Zhang Y, Shi G. Respiratory microbiota and radiomics features in the stable COPD patients. Respir Res 2023;24:131. [Crossref] [PubMed]
- Zhang L, Jiang B, Wisselink HJ, Vliegenthart R, Xie X. COPD identification and grading based on deep learning of lung parenchyma and bronchial wall in chest CT images. Br J Radiol 2022;95:20210637. [Crossref] [PubMed]
- Yang Y, Li W, Kang Y, Guo Y, Yang K, Li Q, Liu Y, Yang C, Chen R, Chen H, Li X, Cheng L. A novel lung radiomics feature for characterizing resting heart rate and COPD stage evolution based on radiomics feature combination strategy. Math Biosci Eng 2022;19:4145-65. [Crossref] [PubMed]
- Estépar RSJ. Artificial Intelligence in COPD: New Venues to Study a Complex Disease. Barc Respir Netw Rev 2020;6:144-60. [Crossref] [PubMed]
- Sun J, Liao X, Yan Y, Zhang X, Sun J, Tan W, Liu B, Wu J, Guo Q, Gao S, Li Z, Wang K, Li Q. Detection and staging of chronic obstructive pulmonary disease using a computed tomography-based weakly supervised deep learning approach. Eur Radiol 2022;32:5319-29. [Crossref] [PubMed]
- Tang LYW, Coxson HO, Lam S, Leipsic J, Tam RC, Sin DD. Towards large-scale case-finding: training and validation of residual networks for detection of chronic obstructive pulmonary disease using low-dose CT. Lancet Digit Health 2020;2:e259-67. [Crossref] [PubMed]
- Li Z, Liu L, Zhang Z, Yang X, Li X, Gao Y, Huang K. A Novel CT-Based Radiomics Features Analysis for Identification and Severity Staging of COPD. Acad Radiol 2022;29:663-73. [Crossref] [PubMed]
- Amudala Puchakayala PR, Sthanam VL, Nakhmani A, Chaudhary MFA, Kizhakke Puliyakote A, Reinhardt JM, Zhang C, Bhatt SP, Bodduluri S. Radiomics for Improved Detection of Chronic Obstructive Pulmonary Disease in Low-Dose and Standard-Dose Chest CT Scans. Radiology 2023;307:e222998. [Crossref] [PubMed]
- Du Y, Li Q, Sidorenkov G, Vonder M, Cai J, de Bock GH, et al. Computed Tomography Screening for Early Lung Cancer, COPD and Cardiovascular Disease in Shanghai: Rationale and Design of a Population-based Comparative Study. Acad Radiol 2021;28:36-45. [Crossref] [PubMed]
- van Griethuysen JJM, Fedorov A, Parmar C, Hosny A, Aucoin N, Narayan V, Beets-Tan RGH, Fillion-Robin JC, Pieper S, Aerts HJWL. Computational Radiomics System to Decode the Radiographic Phenotype. Cancer Res 2017;77:e104-7. [Crossref] [PubMed]
- Friedman JH. Greedy Function Approximation: A Gradient Boosting Machine. Ann Appl Stat 2001;29:1189-232.
- Pedregosa F, Varoquaux G, Gramfort A, Michel V, Thirion B, Grisel O, Blondel M, Prettenhofer P, Weiss R, Dubourg V, VanderPlas J, Passos A, Cournapeau D, Brucher M, Perrot M, Duchesnay E. Scikit-learn: Machine Learning in Python. Scikit-learn: Machine Learning in Python. J Mach Learn Res 2011;12:2825-30.
- Paszke A, Gross S, Massa F, Lerer A, Bradbury J, et al. PyTorch: An Imperative Style, High-Performance Deep Learning Library. arXiv:1912.01703 [Preprint]. 2019. Available online: https://doi.org/
10.48550 /arXiv.1912.01703 - Xie S, Girshick R, Dollár P, Tu Z, He K. Aggregated Residual Transformations for Deep Neural Networks. 2017 IEEE Conference on Computer Vision and Pattern Recognition (CVPR); 21-26 July 2017; Honolulu, HI, USA. IEEE: 2017:5987-95.
- He K, Zhang X, Ren S, Sun J. Deep residual learning for image recognition. 2016 IEEE Conference on Computer Vision and Pattern Recognition (CVPR); 27-30 June 2016; Las Vegas, NV, USA. IEEE; 2016;770-8.
- Guo MH, Liu ZN, Mu TJ, Hu SM. Beyond Self-Attention: External Attention Using Two Linear Layers for Visual Tasks. IEEE Trans Pattern Anal Mach Intell 2023;45:5436-47. [Crossref] [PubMed]
- Makimoto K, Au R, Moslemi A, Hogg JC, Bourbeau J, Tan WC, Kirby M. Comparison of Feature Selection Methods and Machine Learning Classifiers for Predicting Chronic Obstructive Pulmonary Disease Using Texture-Based CT Lung Radiomic Features. Acad Radiol 2023;30:900-10. [Crossref] [PubMed]
- Sun J, Liao X, Yan Y, Zhang X, Sun J, Tan W, Liu B, Wu J, Guo Q, Gao S, Li Z, Wang K, Li Q. Correction to: Detection and staging of chronic obstructive pulmonary disease using a computed tomography-based weakly supervised deep learning approach. Eur Radiol 2022;32:5785. [Crossref] [PubMed]
- Yang Y, Li W, Guo Y, Zeng N, Wang S, Chen Z, Liu Y, Chen H, Duan W, Li X, Zhao W, Chen R, Kang Y. Lung radiomics features for characterizing and classifying COPD stage based on feature combination strategy and multi-layer perceptron classifier. Math Biosci Eng 2022;19:7826-55. [Crossref] [PubMed]
- Röhrich S, Hofmanninger J, Prayer F, Müller H, Prosch H, Langs G. Prospects and Challenges of Radiomics by Using Nononcologic Routine Chest CT. Radiol Cardiothorac Imaging 2020;2:e190190. [Crossref] [PubMed]
- Bhowmik A, Eskreis-Winkler S. Deep learning in breast imaging. BJR Open 2022;4:20210060. [Crossref] [PubMed]