The value of speckle-tracking stratified strain combined with myocardial work measurement in evaluating left ventricular function in patients with heart failure with preserved ejection fraction
Introduction
Heart failure with preserved ejection fraction (HFpEF) is a clinical syndrome characterized by elevated left ventricular (LV) filling pressure at rest or during exercise, typical symptoms and signs of heart failure, a “preserved” left ventricular ejection fraction (LVEF) of ≥50%, and LV diastolic dysfunction (1). Significant morbidity, an increased risk of hospitalization, short- and long-term mortality, and burdensome medical expenses make the high and rising prevalence of HFpEF a concerning public health issue (2-4). In contrast to heart failure with reduced ejection fraction, HFpEF has no effective targeted treatment due to its complex phenotype and heterogeneous pathophysiology (5). Although LV diastolic dysfunction is recognized as the core of the pathophysiology of HFpEF (6), previous studies have shown that abnormal LV systolic function is also part of the mechanism (7-10). LV systolic dysfunction refers to impaired pump function of the heart. LVEF is an indicator to evaluate LV systolic function, and LVEF <50% is defined as LV systolic dysfunction (1,11). Whether LV systolic function is in the normal range will affect decisions about clinical treatment (5). Early assessment of LV contractile performance in HFpEF patients may provide help for early clinical intervention and prevention of disease progression.
Early studies have used tissue Doppler and speckle-tracking imaging to measure LV systolic function in patients with HFpEF, mainly longitudinal systolic dysfunction (9,12). The LV myocardium has been analysed as a whole using both techniques. Nevertheless, the myocardial structure is heterogeneous (13,14), and the myocardial deformation parameters based on the overall wall thickness may reflect the average value of myocardial deformation across layers. Stratified strain and myocardial work (MW) measurement are novel ultrasound techniques derived from two-dimensional speckle-tracking imaging. The layer-specific strain technique can automatically divide the LV myocardium into subendocardial, mid-myocardial, and subepicardial layers, which can quantitatively, objectively, and accurately reflect myocardial mechanics (15). However, the stratified strain technique also has limitations inherent to speckle-tracking echocardiography, such as load dependence, when the LV afterload rises as the longitudinal strain declines (16), affecting the diagnostic accuracy with which myocardial function can be evaluated.
Suga et al. (17) demonstrated that an invasive LV pressure strain ring could reflect MW and oxygen consumption. Russell et al. (18) created a non-invasive left ventricular pressure strain loop (LVPSL) technique. Based on the assumption that the peak LV systolic pressure in echocardiographic study was equal to systolic blood pressure (SBP) measured by a cuff manometer, a patient-specific LV pressure curve was constructed by adjusting the standard LV pressure curve to the duration of the isovolumic and ejection periods defined by the time of valve events, and using strain parameters on two-dimensional speckle tracking imaging to represent myocardial displacement. Noninvasive and invasive LVPSL showed good correlation and consistency in dogs and patients with multiple cardiovascular diseases (r=0.96 and 0.99, respectively) (19-21). Several studies have demonstrated that MW parameters are significantly correlated with LVEF and global longitudinal strain (GLS), and the MW technique has emerged as a novel method to evaluate LV systolic function (19-21). Nevertheless, there have been few reports on applying LVPSL to evaluate LV systolic function and MW in HFpEF patients.
Herein, we applied stratified strain and MW techniques to evaluate LV systolic function and MW in patients with HFpEF and explored the diagnostic efficacy of parameters for HFpEF. This study hypothesized that patients with HFpEF had LV systolic dysfunction and that combining the two techniques could improve the diagnosis of HFpEF, which would have certain prospects for clinical application. We present this article in accordance with the STARD reporting checklist (available at https://qims.amegroups.com/article/view/10.21037/qims-23-1143/rc).
Methods
Study population
This is a prospective study. A total of 89 potentially eligible participants were identified based on signs and symptoms of heart failure (e.g., dyspnea, fatigue, exercise intolerance, and peripheral edema) as well as echocardiographic findings, but 11 patients were excluded because the brain natriuretic peptide (BNP) levels were not elevated, and six patients did not complete informed consent. Of the 72 eligible participants enrolled in the study, acute coronary syndrome (four patients), hypertrophic cardiomyopathy (three patients), significant valvular heart disease (three patients), severe arrhythmia (three patients), primary lung disease (three patients), and poor ultrasound window (seven patients) were excluded.
A total of 49 consecutive patients with de novo primitive HFpEF between 50 and 82 years of age (67.39±7.69 years) were prospectively admitted to the Fourth Affiliated Hospital of Harbin Medical University from December 2019 to May 2021. HFpEF was diagnosed by typical symptoms or signs of heart failure (e.g., dyspnea, fatigue, exercise intolerance, and peripheral edema), “preserved” LVEF (≥50%), increased BNP [BNP >35 ng/L or N-terminal proBNP (NT-proBNP) >125 ng/L], and objective evidence of elevated LV filling pressure at rest and/or with exercise as defined by the following: (I) cardiac structural alterations: left atrial enlargement and/or LV hypertrophy; (II) diastolic dysfunction: the ratio of early mitral inflow velocity to mitral annular early diastolic velocity (E/e') ≥13 and a mean e' of the septal and lateral wall <9 cm/s; and (III) invasively measured pulmonary capillary wedge pressure at rest >15 mmHg and/or with supine ergometry exercise ≥25 mmHg (1). The exclusion criteria were as follows: (I) haemodynamic instability such as acute coronary syndrome or shock; (II) severe congenital or valvular heart disease or pericardial disease; (III) invasive, restrictive or hypertrophic cardiomyopathy; (IV) severe arrhythmia; (V) primary lung, liver or kidney disease; and (VI) suboptimal acoustic image quality unsuitable for strain and MW analysis. The patient screening flow chart is shown in Figure 1.
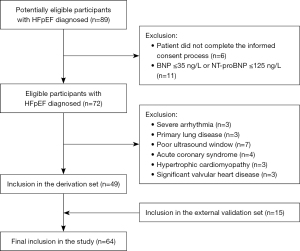
Gender- and age-matched 46 volunteers who visited the physical examination center, including 26 males and 20 females aged 41 to 79 (64.89±9.17) years, with normal echocardiography results, physical examinations, and biochemical indicators, were registered as controls during the same period.
The above 95 subjects (49 HFpEF patients and 46 controls) were used as the derivation set to develop and construct a model to identify HFpEF patients with LV systolic dysfunction. From May 2021 to August 2021, according to the same inclusion and exclusion criteria, 30 additional participants (15 HFpEF patients and 15 controls) were included as an external validation set to verify the model’s performance. The study was conducted in accordance with the Declaration of Helsinki (as revised in 2013). The study was approved by the Ethics Committee of the Fourth Affiliated Hospital of Harbin Medical University (No. 2020-ZWLLSC-02) and informed consent was taken from all individual participants.
General characteristics, including gender, age, height, weight, heart rate, blood pressure, NT-proBNP, baseline diseases (hypertension, coronary heart disease, diabetes, etc.), and New York Heart Association (NYHA) classification (22) were collected in all subjects. Body mass index (BMI) was calculated.
Conventional echocardiography
All participants underwent comprehensive transthoracic echocardiography (Vivid E95, GE) with a M5S 1.7–3.3 MHz phased-array transducer by experienced ultrasound physicians immediately on admission. Two-dimensional echocardiography and Doppler echocardiography were used to measure left atrial diameter (LAd), interventricular septal thick ness in diastole (IVSd), left ventricular posterior wall thickness in diastole (LVPWd), left ventricular end-diastolic diameter (LVEDd), the ratio of mitral valve peak early to late diastolic filling velocity (E/A), and the average E/e'. The left ventricular mass (LVM) was calculated as follows:
The body surface area (BSA) was calculated by the following formula:
The left ventricular mass index (LVMI) was obtained using the following formula:
Relative wall thickness (RWT) was calculated according to the following formula:
LV end-diastolic volume (EDV), LV end-systolic volume (ESV), and LVEF were measured by the biplane Simpson’s method.
Speckle-tracking stratified strain echocardiography
Two-dimensional dynamic images of apical four-, two- and three-chamber views were collected continuously for three cardiac cycles with consistent heart rates at frame rates between 50 and 80 frames/s. All images were stored in Digital Imaging and Communication in Medicine format and imported into the Echo PAC version 203 workstation for offline analysis. Q analysis mode and 2D strain were selected, manually delineating the endocardial boundary of each section at end-diastolic and adjusting the region of interest to cover the entire myocardium. According to the electrocardiogram, the system automatically selected the aortic valve closing time point. It automatically generated the subendocardial, mid-myocardial, and subepicardial GLS of 17 LV segments and a bull’s eye plot. The transmural gradient difference in GLS (ΔGLS = GLSendo − GLSepi) was calculated.
MW analysis
“Myocardial Work” was clicked in the “BE Layers” interface of the offline analysis software. The opening and closing times of the aortic and mitral valves were determined by the apical three-chamber view. The brachial systolic and diastolic blood pressure were input, and “Advanced” was selected. MW parameters were subsequently computed by the differentiation of the strain values over time multiplied by the instantaneous LV pressure. The system automatically obtained the following MW parameters:
- LVPSL: the relationship between pressure and strain in the LV considering the afterload.
- Global myocardial work index (GWI): the total work from mitral valve closure to opening, which is equal to the area of LVPSL.
- Global myocardial constructive work (GCW): the work promoting LV ejection shortening of cardiomyocytes during systole and elongation in the isovolumic relaxation phase.
- Global myocardial wasted work (GWW): the work preventing LV ejection stretching of cardiomyocytes during systole and shortening during the isovolumic relaxation phase.
- Global myocardial work efficiency (GWE): GCW divided by the sum of GCW and GWW.
All parameters were averaged over three measurements.
Statistical analysis
SPSS version 26.0 was applied for the Student’s t-test, Mann-Whitney U test, Chi-squared test, and Fisher exact probability method. Continuous variables for normally distributed data were expressed as the mean ± standard deviation, and Student’s t-test was used for comparisons between groups. Continuous variables for non-normally distributed data were reported as M (P25 – P75), and the Mann-Whitney U test was used. Categorical variables were represented as frequency counts and percentages using chi-square tests or Fisher’s exact tests for comparison.
For the binary classification question, this study applied R software to construct logistic regression model. The logistic regression was calculated as follows:
Variable Y is a binary variable with the value (0,1), where 1 represents the positive result and 0 represents the negative result. And there are m independent variables . represents the probability of a positive result under the action of m independent variables. Z represents the linear relationship of these m independent variables.
The univariate logistic regression model (ULRM) was used to evaluate the diagnostic performance of single technical parameters. When constructing the multivariate logistic regression model (MLRM), we used the principal component analysis method to reduce the influence of the collinearity of some parameters (Tables S1,S2). We transformed the original data into a set of variables that were not correlated with each other. Then, we used the converted data to construct MLRM. In the process of building the model, a 10-fold cross-validation method was performed. Data from the model building were divided into ten equally-sized subsets. Each subset was utilized as a training set, while the remaining subsets were employed as a test set. The reported values were obtained by averaging the results of 10-fold cross-validation, which was conducted to avoid overestimating the incremental values. External data verification was implemented to mitigate overfitting and enhance robustness.
The train function and “glm” method in the Caret package were used to construct the logistic regression model (23). Area under the curve (AUC), sensitivity, and specificity (pROC package, version 1.13.0) were used to assess the diagnostic performance of each model (24). The above statistical analyses were conducted using R version 4.2.2. All tests were two-sided, and P<0.05 was considered statistically significant.
Reproducibility
Fifteen patients were randomly selected, and layer-specific strain and MW parameters were measured by two experienced ultrasound physicians blinded to the patients’ clinical data and each other’s results. Intra-observer concordance was acquired by having the same observer evaluate the same echocardiographic images at intervals of 2 weeks. Inter-observer concordance was evaluated by having another independent observer repeat the analysis of the same images.
Results
Characteristics of the study population
A total of 49 patients with HFpEF (including 33 patients with hypertension, 27 patients with coronary heart disease, 14 patients with diabetes, and nine patients with paroxysmal atrial fibrillation) were included in our study. The baseline clinical characteristics of the overall subjects are summarized in Table 1. Compared to the control group, the HFpEF group had significantly increased weight, BMI, SBP, diastolic blood pressure, and NT-proBNP. There were no significant differences in gender, age, height, or heart rate between the two groups (P>0.05).
Table 1
Variable | HFpEF group (n=49) | Control group (n=46) | P value |
---|---|---|---|
Age (years) | 67.39±7.69 | 64.89±9.17 | 0.15 |
Female gender | 28 [57] | 20 [43] | 0.18 |
Height (cm) | 165.82±6.97 | 166.89±5.35 | 0.40 |
Weight (kg) | 66.08±10.23 | 61.33±10.79 | 0.03 |
BMI (kg/m2) | 24.03±3.53 | 21.87±2.52 | 0.001 |
HR (beats/min) | 75.57±7.20 | 72.61±9.99 | 0.10 |
SBP (mmHg) | 142.00 [124–146] | 128 [121–132] | <0.001 |
DBP (mmHg) | 87.50 [74–94] | 77.00 [73–84] | 0.001 |
NT-proBNP (pg/mL) | 664.50 [425–1,148] | 63.50 [43–83] | <0.001 |
NYHA | |||
II | 21 [43] | – | – |
III | 23 [47] | – | – |
IV | 5 [10] | – | – |
Comorbidity | |||
Hypertension | 33 [67] | – | – |
Coronary artery disease | 27 [55] | – | – |
Diabetes mellitus | 14 [29] | – | – |
Paroxysmal atrial fibrillation | 9 [18] | – | – |
Data are expressed as the n [%], mean ± SD, or M [P25–P75]. 1 mmHg =0.133 kPa. HFpEF, heart failure with preserved ejection fraction; BMI, body mass index; HR, heart rate; SBP, systolic blood pressure; DBP, diastolic blood pressure; NT-proBNP, N-terminal-pro-brain natriuretic peptide; NYHA, New York Heart Association; BP, blood pressure.
Comparison of parameters of conventional echocardiography
Compared with the control subjects, the HFpEF group had significantly increased LAd, IVSd, LVPWd, RWT, LVMI, and average E/e' as well as significantly decreased E/A (P<0.05). No statistically significant difference in LVEDd, EDV, ESV, or LVEF was observed between the two groups, as shown in Table 2.
Table 2
Variable | HFpEF group (n=49) | Control group (n=46) | P value |
---|---|---|---|
LAd (mm) | 39.22±4.31 | 36.34±3.48 | 0.001 |
E/A | 0.77 (0.62–0.87) | 1.31 (1.24–1.40) | <0.001 |
The average E/e' | 15.26 (13.25–16.94) | 6.43 (5.15–7.65) | <0.001 |
IVSd (mm) | 11.02±1.18 | 8.96±1.08 | <0.001 |
LVPWd (mm) | 10.72±1.35 | 8.92±1.41 | <0.001 |
RWT (mm) | 0.46±0.05 | 0.39±0.06 | <0.001 |
LVMI (g/m2) | 109.90±17.52 | 84.64±17.77 | <0.001 |
LVEDd (mm) | 47.00±2.92 | 45.87±3.01 | 0.07 |
EDV (mL) | 104.63±13.84 | 99.89±14.39 | 0.11 |
ESV (mL) | 40.61±7.92 | 38.33±7.23 | 0.15 |
LVEF (%) | 60.18±4.04 | 61.46±3.66 | 0.11 |
Data are expressed as the mean ± SD or M (P25–P75). HFpEF, heart failure with preserved ejection fraction; LAd, left atrial diameter; E/A, the ratio of mitral valve peak early to late diastolic filling velocity; E/e', the ratio of early mitral inflow velocity to mitral annular early diastolic velocity; IVSd, interventricular septal thickness in diastole; LVPWd, left ventricular posterior wall thickness in diastole; RWT, relative wall thickness; LVMI, left ventricular mass index; LVEDd, left ventricular end-diastolic diameter; EDV, end-diastolic volume; ESV, end-systolic volume; LVEF, left ventricular ejection fraction.
Comparison of stratified strain and MW parameters
Transmural gradients of GLSendo, GLSmyo, and GLSepi, decreasing from the endocardium to the epicardium, existed in both the HFpEF group and the control group; patients with HFpEF had significantly lower GLSendo, GLSmyo, GLSepi, ΔGLS, GWI, GWE, and GCW as well as markedly higher GWW than controls (P<0.05), as reported in Table 3 and Figure 2.
Table 3
Variable | HFpEF group (n=49) | Control group (n=46) | P value |
---|---|---|---|
GLSendo (%) | −21.13±2.57 | −24.18±1.90 | <0.001 |
GLSmyo (%) | −18.52±2.31 | −20.46±1.67 | <0.001 |
GLSepi (%) | −16.37±1.38 | −17.59±0.96 | <0.001 |
ΔGLS (%) | −4.76±1.28 | −6.58±0.97 | <0.001 |
GWI (mmHg%) | 1,797.02±251 | 2,099.78±289 | <0.001 |
GCW (mmHg%) | 2,092.18±256 | 2,386.24±301 | <0.001 |
GWW (mmHg%) | 87.48 [66.23–121.50] | 60.50 [48.75–73.75] | <0.001 |
GWE (%) | 94 [92–96] | 98 [96.75–99] | <0.001 |
Data are expressed as the mean ± SD or M [P25–P75]. MW, myocardial work; HFpEF, heart failure with preserved ejection fraction; GLSendo, global longitudinal strain of subendocardium; GLSmyo, global longitudinal strain of myocardium; GLSepi, global longitudinal strain of subepicardium; ΔGLS, transmural gradient of global longitudinal strain; GWI, global myocardial work index; GCW, global myocardial constructive work; GWW, global myocardial wasted work; GWE, global myocardial work efficiency.
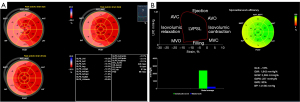
Performance of machine learning algorithms
We conducted univariate and multivariate logistic regression analyses on four stratified strain variables and four MW variables to explore their diagnostic performance for HFpEF. The MLRM based on layer-specific strain parameters had a mean AUC of 0.906 (95% CI: 0.76 to 1), achieving better diagnostic performance than any ULRMs. Among the ULRMs of layer-specific strain parameters, ΔGLS had the largest AUC, at 0.891 (Table S3). When MW parameters were input into the MLRM, the overall diagnostic performance of the model was lower than that of the stratified strain model, with a mean AUC of 0.883 (95% CI: 0.85 to 0.97). The ULRMs of MW parameters revealed that GWE had the largest AUC, at 0.882 (Table S3).
Due to the collinearity of some variables, we performed a principal component analysis on 8 variables. The top four independent principal components (PC1, PC2, PC3, PC4) were selected for subsequent modeling analysis. These four principal components’ variance explanation rates were 73.49%, 11.20%, 7.69%, and 5.93%, respectively, and the cumulative variance explanation rate was 98.31%.
As previously described, based on 10-fold cross-validation way, a logistic regression model was constructed. And the model parameters are displayed in detail in Table 4. The Z-value was calculated as follows:
Table 4
Variable | Estimate | Std.Error | t value | Pr (>|t|) |
---|---|---|---|---|
PC1 | 0.122 | 0.016 | 7.780 | 1.2e−11 |
PC2 | −0.173 | 0.040 | −4.320 | 4.0e−05 |
PC3 | −0.111 | 0.048 | −2.290 | 0.03 |
PC4 | −0.051 | 0.055 | −0.930 | 0.36 |
Intercept | 0.516 | 0.038 | 13.660 | <2e−16 |
PC, principal component.
A higher Z-value means that a sample is more likely to be diagnosed as HFpEF. The mean AUC for the optimal model in the derivation set was 0.966 (95% CI: 0.88 to 1), and participants with a predicted value greater than 0.569 were classified as HFpEF patients with LV systolic function impairment, with 87% sensitivity and 93% specificity (Table 5 and Figure 3). In 10-fold cross-validation, baseline characteristics, conventional ultrasound parameters, stratified longitudinal strain, and MW parameters of HFpEF and control patients were not significantly different between the derivation and validation sets (Table S4).
Table 5
Variable | Derivation set (n=95) | Validation set (n=30) | ||||
---|---|---|---|---|---|---|
Mean AUC (95% CI) | Cutoff value | AUC (95% CI) | Sensitivity (%) | Specificity (%) | ||
Combination of GLS | 0.906 (0.76–1) | 0.452 | 0.902 (0.78–0.99) | 100 | 73 | |
Combination of GMW | 0.883 (0.85–0.97) | 0.463 | 0.908 (0.84–1) | 100 | 87 | |
Combination of GLS & GMW | 0.966 (0.88–1) | 0.569 | 0.933 (0.85–1) | 87 | 93 |
MLRM, multivariate logistic regression model; LV, left ventricular; HFpEF, heart failure with preserved ejection fraction; AUC, area under the curve; CI, confidence interval; GLS, global longitudinal strain; GMW, global myocardial work.
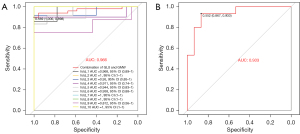
Repeatability test
All stratified strain and MW parameters exhibited excellent reliability and consistency, as summarized in Table 6. Intraclass correlation coefficient (ICC) values were higher for intra-observer variability when the same observer analyzed the same images at intervals. In contrast, the ICC was slightly lower but correlated well with interobserver variability.
Table 6
Variable | Intra-observer variability | Inter-observer variability | |||
---|---|---|---|---|---|
ICC | 95% CI | ICC | 95% CI | ||
GLSendo (%) | 0.915 | 0.766–0.971 | 0.828 | 0.563–0.939 | |
GLSmyo (%) | 0.941 | 0.835–0.980 | 0.882 | 0.686–0.959 | |
GLSepi (%) | 0.884 | 0.690–0.959 | 0.808 | 0.520–0.931 | |
GWI (mmHg%) | 0.915 | 0.766–0.971 | 0.894 | 0.713–0.963 | |
GCW (mmHg%) | 0.887 | 0.697–0.961 | 0.866 | 0.649–0.953 | |
GWW (mmHg%) | 0.873 | 0.663–0.955 | 0.852 | 0.616–0.948 | |
GWE (%) | 0.909 | 0.750–0.968 | 0.888 | 0.700–0.961 |
MW, myocardial work; ICC, intraclass correlation coefficient; CI, confidence interval; GLSendo, global longitudinal strain of subendocardium; GLSmyo, global longitudinal strain of myocardium; GLSepi, global longitudinal strain of subepicardium; GWI, global myocardial work index; GCW, global myocardial constructive work; GWW, global myocardial wasted work; GWE, global myocardial work efficiency.
Discussion
The main findings can be summarized as follows: (I) compared with healthy individuals, HFpEF patients had subclinical changes in LV systolic function, manifested as decreased GLSendo, GLSmyo, GLSepi, ΔGLS, GWI, GWE, and GCW as well as increased GWW. (II) Both ΔGLS in stratified strain and GWE in MW are more effective predictors of LV systolic dysfunction in HFpEF patients. The combination of the two techniques can improve the diagnostic effect.
Patient characteristics and LV morphology
HFpEF, of which mortality risk is similar to that of other heart failure subtypes, has become a prevalent and increasing public health problem (25). Our results regarding the demographic characteristics of HFpEF patients were consistent with previous population-based epidemiologic studies demonstrating that HFpEF patients were older than healthy controls and, had a higher BMI and a higher percentage of women (55%), along with several comorbid diseases, such as hypertension, coronary heart disease, diabetes, and atrial fibrillation (26-28).
Conventional ultrasound parameters in this study showed that HFpEF patients had LV wall hypertrophy, left atrial enlargement, and LV diastolic dysfunction; these features were consistent with the results of previous studies (29). Zile et al. (30,31) revealed significant abnormalities in the active relaxation and passive stiffness of the LV in patients with HFpEF by means of pathophysiological mechanisms and cardiac catheterization. Higher LVMI and E/e' were independently associated with an increased risk of hospitalization for heart failure and with cardiovascular death in HFpEF patients (29).
Stratified strain analysis
LVEF is the percentage of stroke volume to LVEDV, the primary indicator to clinically evaluate LV systolic function. In this study, LVEF was measured by the biplane Simpson’s method. This study found no significant difference in conventional ultrasound parameters for evaluating LV systolic function between the two groups. Nonetheless, LVEF measured by the biplane Simpson’s method has limitations, for example, load dependence, geometric volume assumption, and poor sensitivity to slight LV systolic function impairment. Thus, our research considered more sensitive methods to detect myocardial contractile function. Speckle-tracking imaging tracks acoustic speckles frame-by-frame in the region of interest to quantitatively assess global or regional myocardial function. The myocardium has a unique anatomical structure: the oblique epicardial myocardium has a left-handed spiral arrangement, the middle myocardium has a circular configuration, and the oblique endocardial myocardium with a right-handed helical structure (13). In this study, GLS decreased progressively from the subendocardium to the subepicardium in both groups. This study was consistent with previous findings that the transmural strain gradient might be related to the differences in wall stress caused by the different curvature radii of the three layers of the myocardium (32,33). GLSendo, GLSmyo, GLSepi, and ΔGLS in the HFpEF group were lower than those in the control group, indicating that HFpEF patients had impaired LV longitudinal systolic function. According to the traditional pathophysiological model, HFpEF is the late-stage manifestation of hypertensive heart disease (34). Hypertension leads to increased LV afterload, and cardiomyocytes compensate through hypertrophy and interstitial fibrosis to ensure cardiac output. HFpEF is also often associated with obesity, diabetes, and coronary heart disease (26,27), resulting in microcirculation dysfunction (35) and decreased coronary blood flow reserve. The above mechanisms lead to increased myocardial oxygen demand and insufficient ventricular wall blood supply, thus impairing myocardial contraction performance. HFpEF patients have limited LV filling function, increased end-diastolic intracardiac pressure, increased wall stress in the subendocardium, and elevated energy demand. In addition, the subendocardium is perfused by the end branches of the coronary arteries, which are especially sensitive to early ischaemia. Under these circumstances, the subendocardium is more seriously damaged, and ΔGLS is significantly reduced. Previous studies have reported that normal torsion and circumferential strain in the subendocardium compensate for LV longitudinal systolic dysfunction (7,36), such that LVEF is maintained within the normal range. The sample size of this study was small, EDV and ESV were the same in both groups, and HFpEF had higher blood pressure than the control group, which may require enhanced torsion and circumferential strain to maintain LVEF.
MW analysis
MW measurement is a new ultrasound technology derived from two-dimensional speckle-tracking. It takes into account the influence of GLS and afterload, reflecting the requirements of MW and energy metabolism noninvasively and quantitatively (18). As mentioned above, the GLS of patients with HFpEF is reduced, the myocardial deformability is decreased, and the MW is impaired. The LV filling pressure of HFpEF patients is advanced, and the lengthening of the myocardium is affected during the isovolumic relaxation phase. Hence, GCW decreases and GWW increases. Ventricular systolic synchrony plays a vital role in maintaining MW within the normal range. In patients with HFpEF, LV dyssynchrony results in reduced, absent, or abnormal myocardial movement during ventricular ejection. Consequently, GWI, GCW, and GWE decrease while GWW increases (37). In line with recent research, there were significant differences in MW parameters between the HFpEF and control groups (38,39).
Machine learning model performance
A machine learning-based algorithm serves as a noninvasive diagnostic tool for HFpEF (38,40). The ULRMs of stratified strain demonstrated that ΔGLS had the optimal diagnostic performance for LV systolic function impairment in patients with HFpEF. Tanacli et al. (32) considered GLSepi to be superior to other GLS parameters in identifying HFpEF, but the technique used to detect multilayer myocardial strain was feature-tracking cardiac magnetic resonance. In the ULRMs of MW parameters, GWE is a sensitive predictor of changes in MW and LV systolic function in HFpEF patients. Resting GWE in HFpEF patients is closely associated with reduced exercise capacity, increased pulmonary congestion, and blunted LV contractile reserve during exertion (39). The GWE values showed distinct patterns in different cardiac pathologies (41). Wang et al. and Liu et al. suggested that GWE had better diagnostic performance than other MW indicators in patients with varying degrees of coronary artery stenosis and maintenance haemodialysis (42,43). Li et al. (38) revealed that GWI could play a complementary role to LVEF in the early diagnosis of HFpEF patients. The MLRM combined with stratified strain and MW techniques had the best combined diagnostic performance. The results indicated that the predictive ability of two combined diagnostic techniques was superior to that of any single diagnostic technique, which provided a new perspective for the early detection of LV systolic function changes in HFpEF patients. Previous diagnostic criteria for HFpEF include H2FPEF and HFA-PEFF. A H2FPEF score of 2–5 or a HFA-PEFF of 2–4 points classifies patients as having an intermediate likelihood of HFpEF, wherein further examinations are needed (44,45). However, invasive hemodynamic assessment is invasive, expensive, and risky. Exercise echocardiography is limited by the patient’s physical condition and operator experience. Therefore, this machine learning model may provide supplemental value and a new perspective for diagnosing HFpEF when the previous diagnostic criteria scores indicate intermediate possibilities.
Limitations
This study is a single-centre cross-sectional study with a small sample size, which may have selection bias and a lack of long-term follow-up of patients. Layer-specific technology has been developed in recent years, and we have few studies evaluating its clinical applicability. Therefore, future studies with larger sample sizes and a cohort or randomized controlled trial design are needed to provide a robust conclusion regarding LV systolic dysfunction in HFpEF patients. The layer-specific strain technique relies on postprocessing software that automatically divides the LV myocardium into three layers, which may differ from the actual myocardial fibre structure. There may be a few differences between brachial artery systolic pressure measured by a sphygmomanometer and the LV haemodynamic profile in the MW technique. Speckle-tracking technology requires high image quality with clear endocardial and epicardial boundaries; thus, patients need to be screened more strictly.
Conclusions
There are subclinical changes in LV myocardial function in patients with HFpEF. ΔGLS and GWE are good predictors of LV systolic function impairment in patients with HFpEF. The combination of speckle-tracking stratified strain and MW measurement may improve diagnostic performance and provide incremental value for the early identification of HFpEF.
Acknowledgments
Funding: This work was supported by
Footnote
Reporting Checklist: The authors have completed the STARD reporting checklist. Available at https://qims.amegroups.com/article/view/10.21037/qims-23-1143/rc
Conflicts of Interest: All authors have completed the ICMJE uniform disclosure form (available at https://qims.amegroups.com/article/view/10.21037/qims-23-1143/coif). The authors have no conflicts of interest to declare.
Ethical Statement: The authors are accountable for all aspects of the work in ensuring that questions related to the accuracy or integrity of any part of the work are appropriately investigated and resolved. The study was conducted in accordance with the Declaration of Helsinki (as revised in 2013). The study was approved by the Ethics Committee of the Fourth Affiliated Hospital of Harbin Medical University (No. 2020-ZWLLSC-02) and informed consent was taken from all individual participants.
Open Access Statement: This is an Open Access article distributed in accordance with the Creative Commons Attribution-NonCommercial-NoDerivs 4.0 International License (CC BY-NC-ND 4.0), which permits the non-commercial replication and distribution of the article with the strict proviso that no changes or edits are made and the original work is properly cited (including links to both the formal publication through the relevant DOI and the license). See: https://creativecommons.org/licenses/by-nc-nd/4.0/.
References
- Bozkurt B, Coats AJS, Tsutsui H, Abdelhamid CM, Adamopoulos S, Albert N, et al. Universal definition and classification of heart failure: a report of the Heart Failure Society of America, Heart Failure Association of the European Society of Cardiology, Japanese Heart Failure Society and Writing Committee of the Universal Definition of Heart Failure: Endorsed by the Canadian Heart Failure Society, Heart Failure Association of India, Cardiac Society of Australia and New Zealand, and Chinese Heart Failure Association. Eur J Heart Fail 2021;23:352-80. [Crossref] [PubMed]
- Schrutka L, Seirer B, Rettl R, Dachs TM, Binder C, Duca F, Dalos D, Badr-Eslam R, Kastner J, Hengstenberg C, Frommlet F, Bonderman D. Heart failure with preserved ejection fraction: Calculating the risk of future heart failure events and death. Front Cardiovasc Med 2022;9:921132. [Crossref] [PubMed]
- Pratley R, Guan X, Moro RJ, do Lago R. Chapter 1: The Burden of Heart Failure. Am J Med 2024;137:S3-8. [Crossref] [PubMed]
- Tomasoni D, Vitale C, Guidetti F, Benson L, Braunschweig F, Dahlström U, Melin M, Rosano GMC, Lund LH, Metra M, Savarese G. The role of multimorbidity in patients with heart failure across the left ventricular ejection fraction spectrum: Data from the Swedish Heart Failure Registry. Eur J Heart Fail 2023; Epub ahead of print. [Crossref]
- Borlaug BA. Evaluation and management of heart failure with preserved ejection fraction. Nat Rev Cardiol 2020;17:559-73. [Crossref] [PubMed]
- Chetrit M, Cremer PC, Klein AL. Imaging of Diastolic Dysfunction in Community-Based Epidemiological Studies and Randomized Controlled Trials of HFpEF. JACC Cardiovasc Imaging 2020;13:310-26. [Crossref] [PubMed]
- Wang J, Khoury DS, Yue Y, Torre-Amione G, Nagueh SF. Preserved left ventricular twist and circumferential deformation, but depressed longitudinal and radial deformation in patients with diastolic heart failure. Eur Heart J 2008;29:1283-9. [Crossref] [PubMed]
- Liu S, Guan Z, Zheng X, Meng P, Wang Y, Li Y, Zhang Y, Yang J, Jia D, Ma C. Impaired left atrial systolic function and inter-atrial dyssynchrony may contribute to symptoms of heart failure with preserved left ventricular ejection fraction: A comprehensive assessment by echocardiography. Int J Cardiol 2018;257:177-81. [Crossref] [PubMed]
- DeVore AD, McNulty S, Alenezi F, Ersboll M, Vader JM, Oh JK, Lin G, Redfield MM, Lewis G, Semigran MJ, Anstrom KJ, Hernandez AF, Velazquez EJ. Impaired left ventricular global longitudinal strain in patients with heart failure with preserved ejection fraction: insights from the RELAX trial. Eur J Heart Fail 2017;19:893-900. [Crossref] [PubMed]
- Morris DA, Ma XX, Belyavskiy E, Aravind Kumar R, Kropf M, Kraft R, Frydas A, Osmanoglou E, Marquez E, Donal E, Edelmann F, Tschöpe C, Pieske B, Pieske-Kraigher E. Left ventricular longitudinal systolic function analysed by 2D speckle-tracking echocardiography in heart failure with preserved ejection fraction: a meta-analysis. Open Heart 2017;4:e000630. [Crossref] [PubMed]
- Federmann M, Hess OM. Differentiation between systolic and diastolic dysfunction. Eur Heart J 1994;15 Suppl D:2-6.
- Wenzelburger FW, Tan YT, Choudhary FJ, Lee ES, Leyva F, Sanderson JE. Mitral annular plane systolic excursion on exercise: a simple diagnostic tool for heart failure with preserved ejection fraction. Eur J Heart Fail 2011;13:953-60. [Crossref] [PubMed]
- Sengupta PP, Krishnamoorthy VK, Korinek J, Narula J, Vannan MA, Lester SJ, Tajik JA, Seward JB, Khandheria BK, Belohlavek M. Left ventricular form and function revisited: applied translational science to cardiovascular ultrasound imaging. J Am Soc Echocardiogr 2007;20:539-51. [Crossref] [PubMed]
- Ishizu T, Seo Y, Kameda Y, Kawamura R, Kimura T, Shimojo N, Xu D, Murakoshi N, Aonuma K. Left ventricular strain and transmural distribution of structural remodeling in hypertensive heart disease. Hypertension 2014;63:500-6. [Crossref] [PubMed]
- Ancedy Y, Ederhy S, Jean ML, Nhan P, Soulat-Dufour L, Adavane-Scheuble S, Chauvet-Droit M, Boccara F, Cohen A. Does layer-specific strain using speckle tracking echocardiography improve the assessment of left ventricular myocardial deformation? A review. Arch Cardiovasc Dis 2020;113:721-35. [Crossref] [PubMed]
- van der Bijl P, Kostyukevich M, El Mahdiui M, Hansen G, Samset E, Ajmone Marsan N, Bax JJ, Delgado V. A Roadmap to Assess Myocardial Work: From Theory to Clinical Practice. JACC Cardiovasc Imaging 2019;12:2549-54. [Crossref] [PubMed]
- Suga H. Total mechanical energy of a ventricle model and cardiac oxygen consumption. Am J Physiol 1979;236:H498-505. [Crossref] [PubMed]
- Russell K, Eriksen M, Aaberge L, Wilhelmsen N, Skulstad H, Remme EW, Haugaa KH, Opdahl A, Fjeld JG, Gjesdal O, Edvardsen T, Smiseth OA. A novel clinical method for quantification of regional left ventricular pressure-strain loop area: a non-invasive index of myocardial work. Eur Heart J 2012;33:724-33. [Crossref] [PubMed]
- Cui C, Liu L, Li Y, Liu Y, Huang D, Hu Y, Zhang L. Left Ventricular Pressure-Strain Loop-Based Quantitative Examination of the Global and Regional Myocardial Work of Patients with Dilated Cardiomyopathy. Ultrasound Med Biol 2020;46:2834-45. [Crossref] [PubMed]
- Li X, Chen H, Han M, Luo Y, Liu F, Chen L, Wang X, Zhao Y, Kang R, Wang C, Zhang C. Quantitative assessment of left ventricular systolic function in patients with systemic lupus erythematosus: a non-invasive pressure-strain loop technique. Quant Imaging Med Surg 2022;12:3170-83. [Crossref] [PubMed]
- Huang D, Cui C, Zheng Q, Li Y, Liu Y, Hu Y, Wang Y, Liu R, Liu L. Quantitative Analysis of Myocardial Work by Non-invasive Left Ventricular Pressure-Strain Loop in Patients With Type 2 Diabetes Mellitus. Front Cardiovasc Med 2021;8:733339. [Crossref] [PubMed]
- Dolgin M. Nomenclature and Criteria for Diagnosis of Diseases of the Heart and Great Vessels. 4th ed. New York: New York Tuberculosis & Health Association; 1940.
- Kuhn M. Building Predictive Models in R Using the caret Package. Journal of Statistical Software 2008;28:1-26.
- Robin X, Turck N, Hainard A, Tiberti N, Lisacek F, Sanchez JC, Müller M. pROC: an open-source package for R and S+ to analyze and compare ROC curves. BMC Bioinformatics 2011;12:77. [Crossref] [PubMed]
- Savarese G, Stolfo D, Sinagra G, Lund LH. Heart failure with mid-range or mildly reduced ejection fraction. Nat Rev Cardiol 2022;19:100-16.
- Linde C, Ekström M, Eriksson MJ, Maret E, Wallén H, Lyngå P, Wedén U, Cabrera C, Löfström U, Stenudd J, Lund LH, Persson B, Persson H, Hage C. Stockholm County/Karolinska Institutet 4D heart failure investigators. Baseline characteristics of 547 new onset heart failure patients in the PREFERS heart failure study. ESC Heart Fail 2022;9:2125-38. [Crossref] [PubMed]
- John JE, Claggett B, Skali H, Solomon SD, Cunningham JW, Matsushita K, Konety SH, Kitzman DW, Mosley TH, Clark D 3rd, Chang PP, Shah AM. Coronary Artery Disease and Heart Failure With Preserved Ejection Fraction: The ARIC Study. J Am Heart Assoc 2022;11:e021660. [Crossref] [PubMed]
- Amdahl MB, Sundaram V, Reddy YNV. Obesity in Heart Failure with Reduced Ejection Fraction: Time to Address the Elephant in the Room. Cardiol Clin 2023;41:537-44. [Crossref] [PubMed]
- Shah AM, Cikes M, Prasad N, Li G, Getchevski S, Claggett B, Rizkala A, Lukashevich I, O'Meara E, Ryan JJ, Shah SJ, Mullens W, Zile MR, Lam CSP, McMurray JJV, Solomon SD. Echocardiographic Features of Patients With Heart Failure and Preserved Left Ventricular Ejection Fraction. J Am Coll Cardiol 2019;74:2858-73. [Crossref] [PubMed]
- Zile MR, Baicu CF, Gaasch WH. Diastolic heart failure--abnormalities in active relaxation and passive stiffness of the left ventricle. N Engl J Med 2004;350:1953-9.
- Zile MR, Brutsaert DL. New concepts in diastolic dysfunction and diastolic heart failure: Part II: causal mechanisms and treatment. Circulation 2002;105:1503-8. [Crossref] [PubMed]
- Tanacli R, Hashemi D, Neye M, Motzkus LA, Blum M, Tahirovic E, Dordevic A, Kraft R, Zamani SM, Pieske B, Düngen HD, Kelle S. Multilayer myocardial strain improves the diagnosis of heart failure with preserved ejection fraction. ESC Heart Fail 2020;7:3240-5. [Crossref] [PubMed]
- Götte MJ, Germans T, Rüssel IK, Zwanenburg JJ, Marcus JT, van Rossum AC, van Veldhuisen DJ. Myocardial strain and torsion quantified by cardiovascular magnetic resonance tissue tagging: studies in normal and impaired left ventricular function. J Am Coll Cardiol 2006;48:2002-11. [Crossref] [PubMed]
- Redfield MM. Heart Failure with Preserved Ejection Fraction. N Engl J Med 2016;375:1868-77. [Crossref] [PubMed]
- Lin X, Wu G, Wang S, Huang J. The prevalence of coronary microvascular dysfunction (CMD) in heart failure with preserved ejection fraction (HFpEF): a systematic review and meta-analysis. Heart Fail Rev 2023; Epub ahead of print. [Crossref]
- Čelutkienė J, Plymen CM, Flachskampf FA, de Boer RA, Grapsa J, Manka R, Anderson L, Garbi M, Barberis V, Filardi PP, Gargiulo P, Zamorano JL, Lainscak M, Seferovic P, Ruschitzka F, Rosano GMC, Nihoyannopoulos P. Innovative imaging methods in heart failure: a shifting paradigm in cardiac assessment. Position statement on behalf of the Heart Failure Association of the European Society of Cardiology. Eur J Heart Fail 2018;20:1615-33. [Crossref] [PubMed]
- Santos AB, Kraigher-Krainer E, Bello N, Claggett B, Zile MR, Pieske B, Voors AA, McMurray JJ, Packer M, Bransford T, Lefkowitz M, Shah AM, Solomon SD. Left ventricular dyssynchrony in patients with heart failure and preserved ejection fraction. Eur Heart J 2014;35:42-7. [Crossref] [PubMed]
- Li Y, Zheng Q, Cui C, Liu Y, Hu Y, Huang D, Wang Y, Liu J, Liu L. Application value of myocardial work technology by non-invasive echocardiography in evaluating left ventricular function in patients with chronic heart failure. Quant Imaging Med Surg 2022;12:244-56. [Crossref] [PubMed]
- AbouEzzeddine OF, Kemp BJ, Borlaug BA, Mullan BP, Behfar A, Pislaru SV, Fudim M, Redfield MM, Chareonthaitawee P. Myocardial Energetics in Heart Failure With Preserved Ejection Fraction. Circ Heart Fail 2019;12:e006240. [Crossref] [PubMed]
- Ward M, Yeganegi A, Baicu CF, Bradshaw AD, Spinale FG, Zile MR, Richardson WJ. Ensemble machine learning model identifies patients with HFpEF from matrix-related plasma biomarkers. Am J Physiol Heart Circ Physiol 2022;322:H798-805. [Crossref] [PubMed]
- El Mahdiui M, van der Bijl P, Abou R, Ajmone Marsan N, Delgado V, Bax JJ. Global Left Ventricular Myocardial Work Efficiency in Healthy Individuals and Patients with Cardiovascular Disease. J Am Soc Echocardiogr 2019;32:1120-7. [Crossref] [PubMed]
- Wang RR, Tian T, Li SQ, Leng XP, Tian JW. Assessment of Left Ventricular Global Myocardial Work in Patients With Different Degrees of Coronary Artery Stenosis by Pressure-Strain Loops Analysis. Ultrasound Med Biol 2021;47:33-42. [Crossref] [PubMed]
- Liu C, Feng YP, Yan ZN, Fan L, Rui YF, Cui L. Value of quantitative analysis of left ventricular systolic function in patients on maintenance hemodialysis based on myocardial work technique. BMC Cardiovasc Disord 2021;21:76. [Crossref] [PubMed]
- Pieske B, Tschöpe C, de Boer RA, Fraser AG, Anker SD, Donal E, et al. How to diagnose heart failure with preserved ejection fraction: the HFA-PEFF diagnostic algorithm: a consensus recommendation from the Heart Failure Association (HFA) of the European Society of Cardiology (ESC). Eur J Heart Fail 2020;22:391-412. [Crossref] [PubMed]
- Reddy YNV, Carter RE, Obokata M, Redfield MM, Borlaug BA A. Simple, Evidence-Based Approach to Help Guide Diagnosis of Heart Failure With Preserved Ejection Fraction. Circulation 2018;138:861-70. [Crossref] [PubMed]